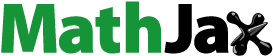
ABSTRACT
Instantaneous determinations of photosynthetically active radiation (PAR) over the sea from the Ocean and Land Color Instrument (OLCI) on Sentinel-3 are compared with in-situ measurements at the island of Lampedusa in the central Mediterranean Sea. Radiative transfer calculations show that the PAR measured at the island site is representative for open ocean conditions. Satellite data show a good agreement (5.2% positive bias, R2 = 0.97) with in-situ data, in line with similar analyses for other satellite sensors. Larger satellite-in situ differences are found during summer, and the possible role of aerosols in degrading PAR estimate has been investigated by comparing AOD values measured at Lampedusa and derived by OLCI. The relative difference between OLCI and in-situ PAR appears to be negatively correlated with the relative differences between OLCI and in-situ AOD, suggesting that a more accurate determination of AOD, in particular, for cases with AOD > 0.2, mostly related to Saharan dust, may lead to improved satellite PAR estimates.
Introduction
Photosynthetically active radiation (PAR) is defined as the solar radiation in the visible part of the spectrum, i.e. in the range between 400 and 700 nm, which is involved in photosynthesis (McCree, Citation1972) and plays a key role in terrestrial and marine primary production and carbon storage (Behrenfeld & Falkowski, Citation1997; Gregg & Rousseaux, Citation2019; Mercado et al., Citation2009). For example, equations for the determination of phytoplankton growth require PAR (e.g. Kiefer & Mitchell, Citation1983), and marine ecosystem models that utilize ocean colour satellite data for estimating primary production rely on PAR as input parameter (Friedrichs et al., Citation2009; Frouin et al., Citation2018; Saux Picart et al., Citation2014). The correct determination of PAR is, thus, essential to understand the behaviour of terrestrial and marine ecosystems and, among other processes, to quantify their role in the carbon cycle (e.g. Gregg & Rousseaux, Citation2019; Kwiatkowski et al., Citation2017).
Moreover, marine ecosystems can also affect ocean physics and eventually atmospheric dynamics (Frouin et al., Citation2018; Miller et al., Citation2003; Shell et al., Citation2003). With this respect, phytoplankton may affect ocean sunlight absorption and, thus, vertical heat distribution, with effects on marine mixed layer dynamics (e.g. Ballabrera-Poy et al., Citation2007; Nakamoto et al., Citation2000, Citation2001). Marine physical and biological interactions and related feedback processes are expected to affect future climate and are responsible for a large fraction of the uncertainties in future projections (Friedlingstein et al., Citation2006).
Local measurements of PAR, although very useful, do not allow to derive information on regional and global-scale processes. For this reason, efforts have been devoted to the determination of PAR from satellite observations (e.g. Frouin & Pinker, Citation1995; Harmel & Chami, Citation2016; Li et al., Citation2015; Liang et al., Citation2006) and to obtain information over large scales. Satellite sensors measure the upward scattered radiance, and the derivation of the downwelling PAR irradiance requires a series of radiative transfer calculations and assumptions on the atmospheric vertical structure and composition. Thus, satellite determination of PAR is somewhat indirect, and verification against high-quality surface data is needed to obtain reliable results. While PAR measurements over land are relatively common (e.g. at the U.S. Department of Agriculture UV sites, Bigelow et al., Citation1998; the National Oceanic and Atmospheric Administration Surface Radiation Budget network, SURFRAD; Augustine et al., Citation2000; FLUXNET; Baldocchi et al., Citation2001; and Integrated Carbon Observation System; Carrara et al., Citation2018, networks), very few datasets are available over the ocean, where validation of operational satellite retrievals is also needed (e.g. Gould et al., Citation2019; Somayajula et al., Citation2018).
A considerable effort has been dedicated to compare, improve and validate satellite PAR observations over land, with a particular interest in the fraction of absorbed PAR (FAPAR), which is a quantity of interest to monitor ecosystem (e.g. Tao et al., Citation2015, Citation2016; X. Zhang et al., Citation2014). According to the WMO, the absolute accuracy requirement for FAPAR products to be acceptable for agronomical and other applications is 0.05 (GCOS, Citation2011). Tao et al. (Citation2015), for example, compared and validated, using ground-based data, FAPAR data from five different sensors: MODIS (Moderate Resolution Imaging Spectroradiometer) and MISR (Multi-angle Imaging SpectroRadiometer) on the Terra satellite, MERIS (Medium Resolution Imaging Spectrometer) on Envisat, SeaWiFS (Sea-Viewing Wide Field-of-View Sensor) on SeaStar, and the GEOV1 products obtained from SPOT-VGT and PROBA-V. In the study, they found that satellite-derived FAPAR products have an uncertainty of 0.14 when validating with total FAPAR measurements and 0.09 when considering only green canopy FAPAR. These products are, thus, close but not fully compliant, to the WMO accuracy requirements for FAPAR. Su et al. (Citation2007) derived PAR using an algorithm primarily based on CERES (Clouds and the Earth’s Radiant Energy System) data and validated the results against data from SURFRAD sites. Z. Zhang et al. (Citation2020) estimated the gross primary production using OLCI FAPAR and MODIS FAPAR data and compared the results with ground-based data from different sites. The gross primary production obtained from the OLCI FAPAR data shows a better agreement with in-situ data than the estimate obtained from MODIS data, with an overall coefficient of determination R2 of 0.44 for MODIS-derived primary production and 0.55 for OLCI-derived primary production. In a different study, Ghayas et al. (Citation2022) compared the CERES PAR data from the Terra satellite with ground-based data in Delhi. PAR estimated from CERES is in a good agreement with in-situ data, with a −5.4% bias, a 14% root mean square difference (RMSD) and R2 = 0.74.
To the best of our knowledge, only a limited number of studies have been dedicated to the analysis and validation of satellite-based PAR estimations over oceanic regions. Vazyulya et al. (Citation2016) compared PAR data from MODIS on the Aqua satellite with measurements made during a research cruise from the Baltic Sea to the White Seas. They found a bias and a RMSD between satellite and ground-based data of up to 7.8% and 9.6%, respectively. Harmel and Chami (Citation2016) proposed an algorithm, tested on MERIS data in the north-western part of the Mediterranean Sea. The algorithm was aimed at obtaining better PAR retrievals by improving the aerosol estimate; the algorithm was planned to be applied to OLCI data (whose data were not operatively available at the time of the study).
Somayajula et al. (Citation2018) compared PAR estimates derived from different methods based on MODIS data with in-situ measurements in the western Mediterranean for both clear-sky and cloudy conditions. The best results were obtained with the NASA Ocean Biology Processing Group (OBPG; Frouin et al., Citation2003) model, that produced a bias of 6.6% and an RMSD of 19.7% between the satellite-derived PAR and the ground-measured values at daily scales.
Gould et al. (Citation2019) compared PAR data derived from MODIS and VIIRS (Visible Infrared Imaging Radiometer Suite), model data and ground-based observations in the Gulf of Mexico. They generally found a 5–7% difference between satellite and ground-based datasets.
Lastly, Tan et al. (Citation2020) investigated the accuracy of PAR retrieval from MERIS data against in-situ observations in different regions: the northwestern Mediterranean Sea, the northwestern Pacific Ocean, and the northeastern Atlantic Ocean. They compared daily (sunrise to sunset) mean radiative transfer model and in-situ data and corrected for possible acquisition and calibration errors using a Monte Carlo radiative transfer model. The correction on ground-based data was of the order of 6%. After the correction, they obtained a bias and a RMSD of 0.6% and 4.0% between MERIS and in-situ data, for clear sky and low aerosol conditions, respectively. When also cases with clouds occurring before/after the satellite overpass were taken into account, the bias and RMSD increased to 7.3% and 15.8%, respectively. As a result of a theoretical study with the 6S (Second Simulation of the
Satellite Signal in the Solar Spectrum; Vermote et al., Citation1997) model, Tan et al. (Citation2020) also suggested that aerosol strongly impacts daily PAR estimates, particularly for cases with aerosol optical depth (AOD) > 0.2. Indeed, with large aerosol loads, especially absorbing aerosol, they found a relative difference in PAR estimates up to 50% compared to clear-sky PAR with no aerosol. They also suggest that the performance of the discussed MERIS algorithm, which relies on a parameterization of the atmospheric transmittance based on AOD and Ångström exponent, degrades for large AOD, especially when dealing with absorbing aerosols.
To our knowledge, there has been no previous validation of OLCI-derived PAR measurements over the ocean. The Sentinel-3 mission, which hosts the OLCI sensor, was designed to guarantee long-term and high-quality measurements. Specifically, OLCI was developed to guarantee continuity with MERIS.
Long-term calibration, validation and verification of Sentinel-3 data were a key aspect of the mission, which is expected to provide high-quality data over an extended time frame (Donlon et al., Citation2012). Thus, validation of OLCI observations is important for monitoring climate parameters over land and over the ocean.
In this study, we compare instantaneous PAR estimates obtained from the OLCI sensor on the Sentinel-3 satellite with measurements of PAR irradiance made on the island of Lampedusa, in the Central Mediterranean Sea, during the period 2016–2021. As far as we are aware, there has been no prior validation of satellite-derived PAR measurements conducted within this particular area of the Mediterranean Sea, which is characterized by frequent cloud-free conditions, variable aerosol properties, and oligotrophic waters (e.g. Liberti et al., Citation2020).
Due to the large role of atmospheric aerosol on the PAR retrieval (Harmel & Chami, Citation2016; Tan et al., Citation2020) we additionally compare OLCI and in-situ AOD measurements, with the aim of examining the influence of AOD on the satellite-based estimation of PAR.
Site, data and methods
Study site
Lampedusa (Italy) is a small island south of the Sicily Channel; the island is flat, with a surface area of about 20 km2. The closest continental region is Tunisia, about 130 km west of Lampedusa. The island is far from large pollution sources and is characterised by frequent cloud-free conditions, mainly in summer, and variable aerosols conditions (e.g. Pace et al., Citation2006). Cloud-free conditions occur in more than 60% of the cases at Lampedusa during June, July, and August, with peaks of 80%. The frequency is < 20% in February, November and December. On average, the area has a 37% overall probability of cloud-free occurrence; the interannual variability is relatively small (Trisolino et al., Citation2018).
Aerosol transport patterns and optical properties over Lampedusa have been extensively studied using ground-based measurements and satellite data (e.g. diSarra et al., Citation2015; Meloni et al., Citation2006, Citation2007; Pace et al., Citation2006). When not directly influenced by Saharan dust transport events, Lampedusa is characterized by low aerosol optical depth. When such events are not considered in the statistics the annual mean aerosol optical depth at 870 nm is about 0.09 (Liberti et al., Citation2020; Pace et al., Citation2006). About 75% of all daily average AOD values at 870 nm measured in the period 1999–2018 are below 0.15, while larger AOD values are measured during sporadic events of Saharan dust transport that typically last for 1 or 2 days (Meloni et al., Citation2007). The Saharan dust AOD at Lampedusa displays a clear annual cycle, with the largest values in spring and summer (e.g. DiIorio et al., Citation2009; diSarra et al., Citation2015; Pace et al., Citation2006). While dust transport occurring in the boundary layer does not display a clear seasonal cycle, the annual evolution of AOD is modulated by dust transport in the free troposphere (DiIorio et al., Citation2009; Marconi et al., Citation2014). During spring-summer daily AOD values associated with dust events may exceed 1 (e.g. diSarra et al., Citation2011), while monthly mean values often exceed 0.2 or 0.3 (diSarra et al., Citation2015). Cases of pollution transport from the European continent are infrequent: cases influenced by emissions from forest fires have been investigated (Pace et al., Citation2005), as well as aerosol originating from heavy fuel combustion processes (i.e. ship traffic or refineries; Becagli et al., Citation2012). The impact of emissions from Etna on AOD is very limited (Sellitto et al., Citation2017).
At surface sea salt represents the largest absolute and relative contribution to PM10 in all seasons, with a maximum (54%) in winter and minima (33%) in summer, while desert dust contributes by 17%–37%, with somewhat larger fractions in autumn (Calzolai et al., Citation2015).
The evolution of PAR is discussed by Trisolino et al. (Citation2018). The long-term mean values for global and diffuse PAR are 95 and 35 W·m−2 (corresponding to 432 and 159 μE·m−2·s−1, respectively), respectively. The mean annual cycle semi-amplitude is about 55% and 36% of the long-term mean, respectively, for the global and diffuse PAR. Clouds largely modulate PAR, although differences between all-sky conditions and cloud-free PAR are small in summer, due to high frequency of clear sky. The diffuse component of PAR for cloud-free conditions displays the largest variability during the months characterized by the largest AOD (April–July).
In-situ measurements
The in-situ measurements used in this study have been made at the Atmospheric Observatory (AO) of the Station for Climate Observation on the island of Lampedusa (35.5°N, 12.6°E; http://www.lampedusa.enea.it). shows the position of Lampedusa in the central Mediterranean, a satellite picture of the island, whose west-to-east size is about 10 km, and an aerial picture of the Atmospheric Observatory.
Figure 1. Geographical location of Lampedusa in the Central Mediterranean Sea (top left), satellite photography of Lampedusa (bottom image, image ISS026-E-21448) with the orange circle indicating the position of the atmospheric observatory, and aerial image of the atmospheric observatory (top right, picture kindly provided by Mirko Nobili).

The Lampedusa Atmospheric Observatory, which was set up in 1997, is placed on the north-eastern plateau of the island, and the measurements of downwelling radiation are made on the terrace of the main Observatory building, at a height of approximately 50 m above sea level. The instruments on the terrace have a free horizon, totally devoid of obstacles. All the radiometers are placed at the same altitude, with the instrument horizon above all surrounding obstacles. The different radiometers are mounted along the south-north axis to minimize mutual interference. The coastline is about 25 m north-east of the radiometer position. A large set of parameters to investigate climate processes is measured at Lampedusa (see, e.g. Liberti et al., Citation2020).
The PAR dataset has been constructed using direct measurements from PAR quantum sensors (Li-Cor model Li-190 R) and from the combination of signals from four narrowband channels of Multifilter Rotating Shadowband Radiometers (MFRSR).
The MFRSR radiometer (Harrison et al., Citation1994) has six 10 nm wide bands, centred approximately around 415, 500, 615, 673, 870 and 940 nm. Four of these fall into the PAR spectral interval. The instrument continuously performs measurements of global and diffuse irradiances with a time resolution of up to 15 s. The PAR irradiance is derived from MFRSR measurements by a linear combination of calibrated irradiances in the four bands; the coefficients of the combination are derived by comparing independent PAR irradiance observations using data from freshly calibrated Li-190 R PAR sensors.
The method for the derivation of PAR has been described by Trisolino et al. (Citation2016), while the dataset collected in the period 2002–2016 has been discussed by Trisolino et al. (Citation2018).
The Li-Cor sensors are calibrated at the factory using 200 W quartz tungsten halogen lamps traceable to the U.S. National Institute of Standards and Technology (NIST).
The absolute calibration error is lower than 5% (typically 3%), as reported on the sensor specifications (e.g. https://www.licor.com/env/products/light/quantum).
A factory calibration is recommended every 2 years, although various studies show that the responsivity of PAR irradiance sensors degrades rapidly during field use, especially in marine environments (e.g. Nunez et al., Citation2022). A better long-term stability is guaranteed by the method by Trisolino et al. (Citation2016), which uses MFRSR measurements over 4 narrow bands to derive PAR. After the initial comparison with the freshly calibrated Li-190 sensor, the long-term stability of subsequent data is guaranteed by frequent field calibrations of the MFRSR channels with the Langley plot method. A discussion of the Langley plot method can be found for example in Schmid and Wehrli (Citation1995). This method is applicable to the direct irradiances derived by the MFRSR as the difference between measurements of the global and the diffuse components. Frequent applications of the Langley plot method at Lampedusa are possible thanks to the high occurrence of cloud-free conditions and the negligible daily cycle (e.g. diSarra et al., Citation2015).
As discussed by Trisolino et al. (Citation2016), this method allows to obtain accurate long-term PAR data, which may be very useful to verify satellite retrievals. The uncertainty on the PAR irradiance measurements obtained with this method is 4–6%.
In this study, the dataset discussed by Trisolino et al. (Citation2018) has been extended to 2018 to cover the period of OLCI observations. Li-Cor Li-190 R data are used for the period 2019–2021. Few interruptions in the ground-based observations, due to technical problems, are present in the dataset.
In-situ measurements of PAR from MFRSR are derived in W/m2, and these values are converted to μE·m−2·s−1 by dividing by 0.22 W·s·µE−1 (Ross & Sulev, Citation2000). The Li-190 R measurements are obtained directly in μE·m−2·s−1.
MFRSR measurements of broadband global and diffuse irradiances are also used to identify cloud-free periods, as will be discussed in the Method section. In addition to MFRSR data, all-sky camera images are used to characterize cloud distribution when MFRSR data are not available. All-sky camera images are routinely acquired at 1–min time resolution at AO.
As will be discussed below, also measurements of AOD made at Lampedusa in the same period are used. The AOD values used in this study are obtained with Cimel Sun photometer part of AERONET (Holben et al., Citation1998), as well as with MFRSR when AERONET data are not available. The spectral AOD from AERONET and MFRSR is obtained at various wavelengths; in the present study, we use the AOD at 500 and 870 nm. Instantaneous level 2.0 AERONET data are used; the estimated uncertainty on level 2.0 AOD is about 0.015 (Barreto et al., Citation2016). AOD determinations by MFRSR are corrected for the influence of the forward scattering produced by large-sized aerosol, as described by diSarra et al. (Citation2015). The estimated uncertainty on the derived MFRSR AOD is about 0.02.
From the AOD at 500 and 870 nm the Ångström exponent is calculated and is used to characterize particle size and type.
OLCI measurements
In this study, we use data from the Ocean and Land Colour Instrument (OLCI) that was launched on the Sentinel-3A satellite in 2016. OLCI is a medium-resolution push-broom imaging spectrometer with 21 bands in the range 0.4–1.2 μm, a spatial resolution of up to 300 m, and a swath of 1270 km.
OLCI L2 data, downloaded from the EUMETSAT data store, are remapped on the equirectangular grid covering the regions of interest (i.e. 32.5–39°N and 10–18°E), with 1 km spatial resolution, once all the flagged pixels have been removed. Flags selected to clean up the data are those suggested by EUMETSAT (https://www-cdn.eumetsat.int/files/2022–05/S3%20PN-OLCI-L2M_003_02%20-%20Sentinel-3%20Product%20Notice%20%E2%80%93%20OLCI%20Level-2%20Ocean%20Colour_new.pdf): CLOUD, CLOUD_AMBIGUOUS, CLOUD_MARGIN, INVALID, COSMETIC, SATURATED, SUSPECT, HISOLZEN, HIGHGLINT, SNOW_ICE, AC_FAIL, WHITECAPS, ADJAC, RWNEG_O2, RWNEG_O3, RWNEG_O4, RWNEG_O5, RWNEG_O6, RWNEG_O7, RWNEG_O8, PAR_ FAIL.
As reported in the OLCI PAR Algorithm Theoretical Basis Document (Lavender, Citation2010), OLCI estimates of the PAR reaching the ocean surface are based on the MERIS PAR algorithm, which relies on the Gregg and Carder (Citation1990) model for clear sky conditions, adapted using Frouin et al. (Citation2003).
The effect of aerosol in the algorithm is taken into account using the aerosol optical depth obtained from the OLCI band at 865 nm.
The resulting product is an instantaneous value, in μE·m−2·s−1, obtained from calculations based on a plane-parallel model atmosphere and assuming a decoupling of the effects of clouds and clear sky.
Thus, the PAR estimates are obtained at the satellite overpass over Lampedusa, which takes place approximately between 8.30 and 9.30 UTC. Consequently, the solar illumination conditions change considerably throughout the year, with solar zenith angles ranging from about 70° in winter and 25° in summer. shows the histogram of the number of occurrences of the solar zenith angle values at OLCI overpass time in Lampedusa for the period covered by the present study.
Statistical parameters
Four statistical indices were employed to assess the quality of the OLCI estimates: bias, root mean squared difference (RMSD), unbiased RMSD (ubRMSD) and coefficient of determination (R2). The intercept, slope, and p-value of the fit have been also calculated. The unbiased RMSD describes the root mean distance of the OLCI with respect to in-situ data, regardless of the average bias between the two distributions. The introduced quantities are defined as follows:
In these formulas, yi are the PAR values derived from OLCI observations, xi are the in situ PAR observations and N is the total number of data pairs. The variables with subscript m identify the corresponding averages. The coefficient of determination is computed as the square of the Pearson correlation coefficient.
Methods
The OLCI dataset is composed of instantaneous PAR values at the OLCI overpass over Lampedusa, covering the period from April 2016 to December 2021. OLCI PAR measurements are obtained considering the value of one of the pixels closest to AO without land contamination, chosen about 4 km east of the measurement site.
The in-situ PAR dataset is obtained, for each day, considering the instantaneous in-situ data closest in time to the OLCI overpass time, within a maximum time difference of 6 min. The maximum time difference between satellite and ground-based AOD measurements is set to 10 min.
Due to the application of cloud-specific flags (i.e. CLOUD, CLOUD_AMBIGUOUS, CLOUD_MARGIN) the influence of cloudy pixels is expected to be minimal in the OLCI data. The resulting dataset for quasi-clear sky conditions when satellite and ground-based measurements are present is composed of 352 data pairs. A second check aimed at identifying cloud-free data selected on the basis of ground-based measurements has been also implemented. Previous studies have shown that determinations of cloud cover from space and from the surface may differ, especially in the case of partial cloud cover, due to different factors and in particular the different spatial resolution (e.g. Werkmeister et al., Citation2015; Wielicki & Parker, Citation1992). Cloud screening based on ground-based data is performed using two methods. Whenever MFRSR data are available, the algorithm described by Meloni et al. (Citation2007), is used. This algorithm is an adaptation of the method by Long and Ackermann (Long & Ackerman, Citation2000) and is based on the simultaneous measurement of global and diffuse broadband solar irradiances. This method allows to identify conditions with cloud cover < 2 oktas and sun unobstructed by clouds. When MFRSR observations are not available, a visual inspection of all-sky camera images has been carried out, and cloud-contaminated images are discarded.
The cloud screening procedure leads to the elimination of many data, and the remaining dataset comprises 93 data pairs.
As discussed above, AO is located at about 50 m above sea level, over a rocky terrain, close to the northeastern promontory of the island. Thus, the measurements used in the comparison are not obtained directly over the ocean, although they benefit from stable levelling and regular cleaning. Previous studies have shown that there is a very good correspondence, with a small bias, between measurements of broadband solar irradiance carried out at AO and those made at the Oceanographic Observatory, which is an open ocean instrumented buoy located about 15 km South-West of AO (diSarra et al., Citation2019). In this study, the bias induced by differences between AO and the ocean surface, due to both altitude and albedo, is estimated through radiative transfer model calculations.
The MODerate-resolution atmospheric radiance and TRANsmittance model (MODTRAN, version 6.0; Berk et al., Citation2014) was run to simulate the downwelling PAR irradiance at the AO and over the sea. The model has been initialized using, as input, parameters measured during a field campaign in July 2013. The model used 93 vertical layers with higher resolution in the lowest troposphere. The downwelling PAR irradiance has been estimated for varying values of the solar zenith angle between 12° (the minimum value reached at Lampedusa) and 85°, assuming no aerosol, and taking into account differences in surface albedo and height. The spectral surface albedo at the AO was estimated as in Meloni et al. (Citation2015), as a combination of land (barren/desert surface type included in the MODTRAN model) and sea albedo with a proportion of 72% and 28%, respectively. The sea albedo from Jin et al. (Citation2004) has been used. Although water vapour plays a very limited role in regulating PAR, variations of column water vapour associated with the altitude change are included in the model, based on data from radiosonde launches made in July 2013. The total column water vapour used in the model is 3.0 cm. No changes in the total ozone column, which was assumed to be 305 Dobson units (value measured on 3 July 2013, which corresponds to the annual mean in Lampedusa), were considered in the simulations. The ozone profile measured by aircraft in July 2013 was used in the simulations. Additional details on the model setup are given by Meloni et al. (Citation2018). The differences between calculated PAR at sea level and at 50 m are always smaller than 0.75% (PAR at sea level smaller than at AO) for all values of the solar zenith angle. The relative difference slightly increases with the solar zenith angle.
The calculated results are consistent with similar calculations made by diSarra et al. (Citation2019) for broadband solar irradiance; they found an average difference always < 1%.
Being the combined effect of altitude and albedo on PAR much smaller than the associated measurement uncertainty, we have assumed that PAR observations at AO may be taken as representative of ocean surface measurements, and no correction for these effects was applied to the data.
Results and discussion
shows the time series of in situ 24-h average PAR and daily mean AOD measurements at Lampedusa. The main characteristics of the PAR and AOD seasonal cycles appear evident in the figure. The PAR reaches its peak in June, when the minima of solar zenith angle are reached, and gradually decreases to its minimum in December. The large impact of clouds on the downwelling irradiance is evident in the figure, mainly in winter. As discussed above, AOD peaks are generally associated with Saharan dust events, which are more frequent in spring and summer and last 1–2 days. In this figure, the aerosol impact on PAR is masked by the dominating cloud effect.
Figure 3. Time series of in-situ 24-h mean PAR (orange) and AOD at 870 nm (grey) for the period 2016–2021.

The daily course of instantaneous PAR (iPAR) irradiance on 2 days in different seasons is shown in , together with the iPAR value derived from OLCI. On both days cloud-free conditions are dominant, and an overestimate of PAR by OLCI appears evident.
Figure 4. Daily evolution of instantaneous in-situ PAR for a summer day (2016-07-09, left) and for a winter day (2021-11-12, right). The green triangles are the OLCI instantaneous PAR irradiances at the sentinel-3 overpass.

shows the time series of OLCI and in-situ determinations of iPAR for quasi-clear-sky conditions determined from the OLCI data flags. A 6% uncertainty on ground-based PAR measurements is shown in the graph. The uncertainty on OLCI PAR data is not available in the data files and has not been included in the graph.
Figure 5. Time series of instantaneous OLCI (orange) and in-situ (grey) PAR data. Data are selected based on different cloud flags of OLCI data (see text). An estimated 6% uncertainty (vertical bars) has been associated with the in-situ PAR data. The red dots are the relative difference between OLCI and in-situ data, normalised with respect to the in-situ values.

The iPAR seasonal change is very evident and is mainly due to the seasonal evolution of the solar zenith angle at the OLCI overpass time. The distribution of solar zenith angle number of occurrences is shown in . The OLCI iPAR estimation effectively reproduces the seasonal evolution observed in the corresponding ground-based measurements.
reports some statistical parameters for the two datasets. In general, OLCI tends to provide slightly higher estimates of PAR compared to in-situ measurements, with a bias of 83.2 μE·m−2·s−1 and RMSD of 141.3 μE·m−2·s−1. These values represent 5.7% and 9.7% of the mean measured iPAR, respectively. This result is consistent with findings from previous studies (e.g. Gould et al., Citation2019; Tan et al., Citation2020; Vazyulya et al., Citation2016). The unbiased RMSD is 114.5 μE·m−2·s−1 (8%), which is compatible with results from Somayajula et al. (Citation2018).
Table 1. Statistical indices for PAR with and without cloud selection based on ground-based data.
displays the scatter plot between OLCI and in-situ iPAR measurements. The plot reveals a good agreement between the two datasets, with a relatively small number of points deviating from the diagonal line. The coefficient of determination, R2, is 0.87, confirming a strong correlation, while the slope of the fit is 0.93. The p-value associated with the calculated R2 is considerably lower than 0.05.
Figure 6. Scatterplot between OLCI and in-situ instantaneous PAR data selected based on different cloud flags of OLCI data (see text). The grey line is the bisector and the orange line is the linear fit to the data.

As discussed above, due to the possible large effect of residual clouds, the comparison was carried out also with the reduced dataset, obtained by applying the cloud screening based on ground observations.
shows the time series of cloud-screened ground-based and satellite instantaneous PAR. The agreement between OLCI and in-situ data is better for the cloud-screened than for the previous dataset. The bias reduces to 78.0 μE·m−2·s−1 (5.2%) and the RMSD to 97.8 μE·m−2·s−1 (6.6%), whereas the ubRMSD becomes 58.9 μE·m−2·s−1 (4%). Statistical parameters for this case are also reported in .
Figure 7. Time series of instantaneous OLCI (orange) and in-situ (grey) PAR for cloud-free days selected from ground-based data. An estimated 6% uncertainty (vertical bars) has been associated with the in-situ PAR data. The red dots show the difference between OLCI and in-situ data, normalised with respect to the in-situ values.

reports statistical parameters calculated for different seasons (winter DJF, spring MAM, summer JJA, autumn SON) for the dataset without ground-based cloud screening. reports the same quantities for the ground-based cloud-screened dataset. It is interesting to note that the cases with the largest differences, in terms of absolute bias and RMSD, occur in summer for both datasets. In particular, the ratio between RMSD and mean iPAR (but also between bias and mean iPAR) is largest during summer, when R2 becomes much smaller than in the other seasons.
Table 2. Seasonal statistical indices for PAR without cloud selection based on ground-based data.
Table 3. Seasonal statistical indices for PAR with cloud selection based on ground-based data.
shows the scatter plot between the cloud-screened datasets. It can be noted that most of the off-diagonal data points have disappeared, confirming that the largest deviations found in were due to residual clouds. Also in this case, OLCI overestimates in-situ data. R2 has risen to 0.97, indicating a robust correlation between OLCI and in-situ data. The scatter plot linear fit slope is 1.02, compared with 0.93 for the dataset without cloud screening based on ground-based observations. In line with these findings, the p-value remains substantially below 0.05.
Figure 8. Scatterplot of OLCI vs in-situ instantaneous PAR data, selected for cloud-free conditions from ground-based observations (see text). The grey line is the bisector and the orange line is the linear fit to the data.

It can also be noted that most of the data in lie on a line which is approximately parallel with respect to the 1:1 line, with the largest deviations occurring for PAR values above about 1400 μE·m−2·s−1. Thus, to investigate the cause of the deviation, the ground-based cloud-screened PAR dataset has been divided into two subsets, above and below a threshold of 1450 μE·m−2·s−1. This threshold value essentially separates autumn-winter from spring-summer observations (see also ). shows the distribution of the number of occurrences of the difference between OLCI and in-situ PAR data for the two subsets. Differences between OLCI and in-situ data are centred at about 75 μE·m−2·s−1 in autumn-winter, when the influence of high AOD cases is small. Two peaks can be found in spring-summer, one approximately around 75 μE·m−2·s−1, which is present also in the autumn-winter subset, and one approximately around 150 μE·m−2·s−1.
Figure 9. Histogram of the number of occurrences of the differences between OLCI and in-situ iPAR values. The ground-based cloud-screened dataset is divided into two subsets considering in-situ iPAR data below (orange histogram with black contour lines) and above (blue histogram with no contour lines) a threshold of 1450 μE·m−2·s−1, respectively.

Thus, differences around 75 μE·m−2·s−1 are present throughout the year and may suggest the presence of an overall bias between OLCI and in-situ PAR determinations. The spring and summer peak around 150 μE·m−2·s−1 may be linked to specific conditions, limited in time, which may produce larger differences. Large AOD values may be found at Lampedusa in spring and summer, when they are generally associated with intrusions of Saharan dust. We may thus investigate the possibility that high AOD cases are those producing the largest differences in PAR estimates.
AOD impact on PAR differences
shows the coincident OLCI-in-situ AOD observations corresponding with simultaneous iPAR determinations, and the distribution of the corresponding Ångström exponent values obtained from the ground-based observations. Although the number of selected data is limited, similarly to ground-based data, the OLCI AOD displays larger values in spring and summer, with a peak around 0.5. Most AOD observations (73%) have an Ångström exponent < 1, while for many cases it is smaller than 0.5. This value of the Ångström exponent has been used to identify Saharan dust cases at Lampedusa. Cases with Ångström exponent > 1.5 have been attributed to transport of small-sized particles from urban-industrial activities or forest fires in continental Europe (Pace et al., Citation2006), and display a low frequency of occurrence. The OLCI retrieval algorithm takes into account the AOD effect, and we expect that inaccuracies in the determination of AOD may influence the PAR determination. It must also be pointed out that the OLCI algorithm uses a single band (outside the PAR spectral interval) to retrieve AOD, and this may affect the estimation of the aerosol effect at shorter wavelengths. Due to the AOD seasonal evolution at Lampedusa, we expect that the largest differences in the retrieved OLCI AOD occur in the summer, as well as the largest differences in the PAR determinations.
Figure 10. Left: time series of instantaneous AOD determinations from OLCI (orange) and in-situ measurements (grey). The estimated uncertainty associated with ground-based observations is 0.015. Right: histogram with the distribution of Ångström exponent values; the Ångström exponent is derived from the multi-wavelength ground-based observations.

Thus, we have compared AOD determinations by OLCI with ground-based measurements at Lampedusa at 870 nm. Ground-based AOD data closest in time to the OLCI overpass, within a 10-min interval, are used in the comparison. The AOD dataset is composed of 64 data pairs.
shows the scatter plot between OLCI and in-situ AOD. AODs from Cimel and MFRSR measurements reach larger values (0.5) than OLCI (0.35).
Figure 11. OLCI vs in-situ aerosol optical depth. The grey line is the bisector, the orange line is the linear fit considering the whole dataset, the red line is the linear fit obtained considering only the in-situ AOD below 0.2 and the blue line is the linear fit for in-situ AOD above 0.2. Elements circled in red and grey correspond to, respectively, Ångström exponent below and above 0.5.

A good agreement is found for AOD below 0.2, even if some outliers are present, with OLCI overestimating in situ AOD. A degradation of the agreement between OLCI and in situ AOD determinations is evident, notwithstanding the small number of cases, for AOD > 0.2.
reports a summary of statistical indices for the AOD datasets. Due to the different behaviour, these indices were also calculated separately for values of surface AOD, respectively, less than and greater than 0.2.
Table 4. Summary of the statistical indices for in-situ and OLCI AOD.
The bias between OLCI and ground-based data is small when considering the entire dataset (0.0083, corresponding to 7.5%). RMSD and ubRMSD are, however, relatively high, respectively, 0.051 (46%) and 0.051 (45%).
When the dataset is divided into values below and above 0.2, a positive bias (0.025, 38% of the observed mean with AOD < 0.2) is found for AOD < 0.2, and a negative one for AOD > 0.2 (bias of −0.057, 19% of the observed mean with AOD > 0.2). The low values of R2 and the slope of the fit suggest a poor agreement for AOD > 0.2. The over- and underestimate effects for the two ranges of AOD appear to offset each other when the full dataset is considered. A small bias, although with a large RMSD, is found for the full dataset, together with a relatively large value of R2. The R2 values shown in are characterized by a p-value substantially below 0.05.
It must be noted, however, that this behaviour is driven by few data points, and the availability of a wider dataset appears necessary to draw final conclusions.
The data points colour shows the corresponding value of the Ångström exponent, and it is possible to note that most of the data points which lie far from the 1:1 line are characterized by a small exponent.
To simplify the visualization, in , data with Ångström coefficient < 0.5 are circled in red.
To investigate the impact of the AOD differences on the OLCI PAR estimates, we calculated the relative deviation between OLCI and in-situ PAR and between OLCI and in-situ AOD determinations. shows the scatter plot between the PAR and AOD relative deviations. Despite the modest correlation (R2 = 0.18, p < 0.05) and the spread in the data, a negative correlation appears, suggesting that an underestimate of the AOD leads to an overestimate of PAR.
Figure 12. Relative difference between OLCI and in-situ PAR vs relative difference between OLCI and in-situ AOD. The grey line is the linear fit. Elements circled in red and grey correspond to, respectively, Ångström exponent below and above 0.5.

also shows, in colours, the value of the Ångström exponent, with data circled in red representing Ångström exponent < 0.5. As shown for example by diSarra et al. (Citation2008), the downwelling solar irradiance decreases for increasing AOD due to aerosol absorption and scattering. Thus, we expect that the calculated PAR irradiance estimated from the OLCI data is overestimated when the AOD is underestimated (i.e. there is an underestimate of the downwelling radiation reduction due to aerosols). The effect shown in with a iPAR overestimate when AOD is underestimated is coherent with what is expected by an erroneous correction for the aerosol influence, and a better determination of AOD, in particular for cases of large particles, is expected to lead to an improvement of the PAR determination. Alternatively, the use of more accurate in-situ AOD determination, where available, might be used at specific sites in the satellite PAR retrieval to obtain more accurate PAR retrievals.
Conclusions
A comparison between OLCI and in-situ determination of photosynthetically active radiation over the ocean has been carried out at Lampedusa, in the central Mediterranean Sea.
Instantaneous ground-based and satellite data covering the period of May 2016–December 2021 have been used. PAR measurements at Lampedusa are taken at the Atmospheric Observatory, which resides on the northeastern promontory of the island, at about 50 m above sea level.
Radiative transfer calculations performed with the MODTRAN radiative transfer model show that the observations on the island of Lampedusa well represent open ocean conditions.
In order to reduce the variability of PAR irradiance due to clouds, only clear sky days are selected. A first dataset comprises data screened using the OLCI cloud flags.
The comparison of instantaneous data shows a good correlation (R2 = 0.88) and values of bias and RMSD (5.7% and 9.7%, respectively) consistent with similar analyses made for PAR derived from other satellite sensors.
A second dataset is obtained using a cloud screening algorithm based on surface observations. The use of this algorithm leads to a strong reduction of the dataset, at the same time significantly improving the agreement between OLCI and in-situ measurements.
The analysis of the second dataset leads to the elimination of all the outliers and to a very good agreement between OLCI and in situ observations, with a smaller RMSD (6.6%) and higher R2 (0.97). The bias remains essentially unchanged (5.2%), with OLCI overestimating ground-based iPAR. The bias and RMSD are similar to those obtained over the ocean in previous comparisons with respect to MODIS, SeaWIFS and VIIRS. Cases with somewhat larger overestimates occur in summer. It is worth noting that the bias found between OLCI and ground-based iPAR estimates is comparable with the uncertainty associated with ground-based PAR measurements (which is in the order of 4–6%). Thus, it is not possible to exclude that part of the bias may be due to a systematic component of the uncertainty.
The possible influence of aerosol on the differences between OLCI and in-situ PAR determinations has been investigated by comparing the satellite and ground-based AOD estimates, and by relating the PAR differences with AOD differences.
The comparison between the OLCI AOD retrieved on band 16 at 865 nm and in situ measurements at Lampedusa displays a good agreement for AOD < 0.2 and a significant underestimate by OLCI for larger AOD values.
Although, in general, the agreement between PAR by OLCI and in-situ is good, larger overestimates of PAR correspond with AOD underestimates, suggesting that an improvement of the AOD determinations may lead to better results. This seems to be particularly important for cases with moderate and large aerosol load, i.e. AOD > 0.2, which appears to be related to Saharan dust events. The impact of AOD on PAR estimates found in the present study, especially for AOD > 0.2, agrees with what was found in the theoretical study by Tan et al. (Citation2020) in Northwestern Mediterranean for daily mean PAR.As discussed in the introduction, PAR data over the ocean are needed, among other things, to estimate primary production. High-quality data are crucial to understand the evolution of the marine ecosystem, also in relation with the carbon cycle. This study suggests that an improvement in the AOD determination might help in reducing uncertainties in cases of high AOD. However, due to the limited statistics, it appears evident that a dedicated study on a more extended dataset would be necessary to have a robust assessment of the uncertainties associated with the OLCI AOD retrieval.
The synergistic use of ground-based AOD and Ångström exponent data at specific sites, with a dedicated correction for the aerosol effect, might also lead to improvement.
Acknowledgments
Contributions by Pamela Trisolino, Fabrizio Anello, and Salvatore Piacentino are gratefully acknowledged.
The authors thank EUMETSAT and the EU Copernicus service for making available the OLCI data and the University of Valladolid and AERONET staff for the provision of Cimel photometer calibration and processing.
Mirko Nobili is thanked for the Lampedusa aerial picture shown in .
The International Space Station picture of is courtesy of the Earth Science and Remote Sensing Unit, NASA Johnson Space Center (https://eol.jsc.nasa.gov).
The reviewers are thanked for their valuable suggestions and contributions.
Disclosure statement
No potential conflict of interest was reported by the author(s).
Data availability statement
Data available on request from the authors.
Additional information
Funding
References
- Augustine, J. A., DeLuisi, J. J., & Long, C. N. (2000). SURFRAD–A National Surface Radiation Budget Network for Atmospheric Research. Bulletin of the American Meteorological Society, 81, 2341–18. https://doi.org/10.1175/1520-0477(2000)081%3C2341:SANSRB%3E2.3.CO;2
- Baldocchi, D., Falge, E., Gu, L., Olson, R., Hollinger, D., Running, S., Anthoni, P., Bernhofer, C., Davis, K., Evans, R., Fuentes, J., Goldstein, A., Katul, G., Law, B., Lee, X., Malhi, Y., Meyers, T., Munger, W., … Wofsy, S. (2001). FLUXNET: A New Tool to Study the Temporal and Spatial Variability of Ecosystem-Scale Carbon Dioxide, Water Vapor, and Energy Flux Densities. Bulletin of the American Meteorological Society, 82(11), 2415–2434. https://doi.org/10.1175/1520-0477(2001)082<2415:FANTTS>2.3.CO;2
- Ballabrera-Poy, J., Murtugudde, R., Zhang, R.-H., & Busalacchi, A. J. (2007). Coupled ocean–atmosphere response to seasonal modulation of ocean color: Impact on interannual climate simulations in the Tropical Pacific. Journal of Climate, 20(2), 353–374. https://doi.org/10.1175/JCLI3958.1
- Barreto, Á., Cuevas, E., Granados-Muñoz, M.-J., Alados-Arboledas, L., Romero, P. M., Gröbner, J., Kouremeti, N., Almansa, A. F., Stone, T., Toledano, C., Román, R., Sorokin, M., Holben, B., Canini, M., & Yela, M. (2016). The new sun-sky-lunar cimel CE318-T multiband photometer – a comprehensive performance evaluation. Atmospheric Meaurement Techniques, 9(2), 631–654. https://doi.org/10.5194/amt-9-631-2016
- Becagli, S., Sferlazzo, D. M., Pace, G., diSarra, A., Bommarito, C., Calzolai, G., Ghedini, C., Lucarelli, F., Meloni, D., Monteleone, F., Severi, M., Traversi, R., & Udisti, R. (2012). Evidence for heavy fuel oil combustion aerosols from chemical analyses at the island of Lampedusa: A possible large role of ships emissions in the mediterranean. Atmospheric Chemistry and Physics, 12(7), 3479–3492. https://doi.org/10.5194/acp-12-3479-2012
- Behrenfeld, M. J., & Falkowski, P. G. (1997). RA consumer’s guide to phytoplankton primary productivity models. Limnology and Oceanography, 42(7), 1479–1491. https://doi.org/10.4319/lo.1997.42.7.1479
- Berk, A., Conforti, P., Kennett, R., Perkins, T., Hawes, F., & van den Bosch, J. (2014). MODTRAN6: A major upgrade of the MODTRAN radiative transfer code. Proceedings of SPIE, Algorithms and Technologies for Multispectral, Hyperspectral, and Ultraspectral Imagery XX, 90880H. https://doi.org/10.1117/12.2050433
- Bigelow, D. S., Slusser, J. R., Beaubien, A. F., & Gibson, J. H. (1998). The USDA ultraviolet radiation monitoring program. Bulletin of the American Meteorological Society, 79(4), 601–616. https://doi.org/10.1175/1520-0477(1998)079<0601:TUURMP>2.0.CO;2
- Calzolai, G., Nava, S., Lucarelli, F., Chiari, M., Giannoni, M., Becagli, S., Traversi, R., Marconi, M., Frosini, D., Severi, M., Udisti, R., diSarra, A., Pace, G., Meloni, D., Bommarito, C., Monteleone, F., Anello, F., & Sferlazzo, D. M. (2015). Characterization of PM 10 sources in the central mediterranean. Atmospheric Chemistry and Physics, 15(24), 13939–13955. https://doi.org/10.5194/acp-15-13939-2015
- Carrara, A., Kolari, P., Op de Beeck, M., Arriga, N., Berveiller, D., Dengel, S., Ibrom, A., Merbold, L., Rebmann, C., Sabbatini, S., Serrano-Ortíz, P., & Biraud, S. C. (2018). Radiation measurements at ICOS ecosystem stations. International Agrophysics, 32(4), 589–605. https://doi.org/10.1515/intag-2017-0049
- DiIorio, T., A di Sarra, A., Sferlazzo, D. M., Cacciani, M., Meloni, D., Monteleone, F., Fuà, D., & Fiocco, G. (2009). Seasonal evolution of the tropospheric aerosol vertical profile in the central mediterranean and role of desert dust. Journal of Geophysical Research: Atmospheres, 114(D2). https://doi.org/10.1029/2008JD010593
- diSarra, A., Bommarito, C., Anello, F., DiIorio, T., Meloni, D., Monteleone, F., Pace, G., Piacentino, S., & Sferlazzo, D. (2019). Assessing the quality of shortwave and longwave radiation observations over the ocean: One year of high time resolution measurements at the lampedusa oceanographic observatory. Journal of Atmospheric and Oceanic Technology, 36(12), 2383–2400. https://doi.org/10.1175/JTECH-D-19-0018.1
- diSarra, A., DiBiagio, C., Meloni, D., Monteleone, F., Pace, G., Pugnaghi, S., & Sferlazzo, D. (2011). Shortwave and longwave radiative effects of the intense saharan dust event of 25–26 March, 2010, at lampedusa (Mediterranean sea). Journal of Geophysical Research: Atmospheres, 116(D23), D23209. https://doi.org/10.1029/2011JD016238
- diSarra, A., Pace, G., Meloni, D., De Silvestri, L., Piacentino, S., & Monteleone, F. (2008). Surface shortwave radiative forcing of different aerosol types in the central mediterranean. Geophysical Research Letters, 35(2), L02714. https://doi.org/10.1029/2007GL032395
- diSarra, A., Sferlazzo, D., Meloni, D., Anello, F., Bommarito, C., Corradini, S., De Silvestri, L., DiIorio, T., Monteleone, F., Pace, G., Piacentino, S., & Pugnaghi, S. (2015). Empirical correction of multifilter rotating shadowband radiometer (MFRSR) aerosol optical depths for the aerosol forward scattering and development of a long-term integrated MFRSR-Cimel dataset at Lampedusa. Applied Optics, 54(10), 2725–2737. https://doi.org/10.1364/AO.54.002725
- Donlon, C., Berruti, B., Buongiorno, A., Ferreira, M.-H., Féménias, P., Frerick, J., Goryl, P., Klein, U., Laur, H., Mavrocordatos, C., Nieke, J., Rebhan, H., Seitz, B., Stroede, J., & Sciarra, R. (2012). The global monitoring for environment and security (GMES) sentinel-3 mission. Remote Sensing of Environment, 120, 37–57. https://doi.org/10.1016/j.rse.2011.07.024
- Friedlingstein, P., Cox, P., Betts, R., Bopp, L., von Bloh, W., Brovkin, V., Cadule, P., Doney, S., Eby, M., Fung, I., Bala, G., John, J., Jones, C., Joos, F., Kato, T., Kawamiya, M., Knorr, W., Lindsay, K., … Zeng, N. (2006). Climate–carbon cycle feedback analysis: Results from the C4MIP model intercomparison. Journal of Climate, 19(14), 3337–3353. https://doi.org/10.1175/JCLI3800.1
- Friedrichs, M. A. M., Carr, M.-E., Barber, R. T., Scardi, M., Antoine, D., Armstrong, R. A., Asanuma, I., Behrenfeld, M. J., Buitenhuis, E. T., Chai, F., Christian, J. R., Ciotti, A. M., Doney, S. C., Dowell, M., Dunne, J., Gentili, B., Gregg, W., Hoepffner, N., … Winguth, A. (2009). Assessing the uncertainties of model estimates of primary productivity in the tropical Pacific Ocean. Journal of Marine Systems, 76(1–2), 113–133 . https://doi.org/10.1016/j.jmarsys.2008.05.010
- Frouin, R., Franz, B., & Wang, M. (2003). Algorithm to estimate PAR from SeaWiFS data. Version 1.2 – documentation. National Aeronautics and Space Administration. https://oceancolor.gsfc.nasa.gov/atbd/par/seawifs_par_wfigs.pdf
- Frouin, R., & Pinker, R. T. (1995). Estimating photosynthetically active radiation (PAR) at the Earth’s surface from satellite observations. Remote Sensing of Environment, 51(1), 98–107. https://doi.org/10.1016/0034-4257(94)00068-X
- Frouin, R., Ramon, D., Boss, E., Jolivet, D., Compiègne, M., Tan, J., Bouman, H., Jackson, T., Franz, B., Platt, T., & Sathyendranath, S. (2018). Satellite radiation products for Ocean Biology and biogeochemistry: Needs, state-of-the-art, gaps, development priorities, and opportunities. Frontiers in Marine Science, 5. https://doi.org/10.3389/fmars.2018.00003
- GCOS. (2011). Systematic observation requirements for satellite-based data products for climate—2011 update. WMO.
- Ghayas, H., Radhakrishnan, S. R., Sehgal, V. K., & Singh, S. (2022). Measurement and comparison of photosynthetically active radiation by different methods at Delhi. Theoretical and Applied Climatology, 150(3–4), 1559–1571. https://doi.org/10.1007/s00704-022-04252-9
- Gould, R. W., Jr., Ko, D. S., Ladner, S. D., Lawson, T. A., & MacDonald, C. P. (2019). Comparison of satellite, model, and in situ values of photosynthetically available radiation (PAR). Journal of Atmospheric and Oceanic Technology, 36(4), 535–555. https://doi.org/10.1175/JTECH-D-18-0096.1
- Gregg, W. W., & Carder, K. L. (1990). A simple spectral solar irradiance model for cloudless marine atmospheres. Limnology and Oceanography, 35(8), 1657–1675. https://doi.org/10.4319/lo.1990.35.8.1657
- Gregg, W. W., & Rousseaux, C. S. (2019). Global ocean primary production trends in the modern ocean color satellite record (1998–2015). Environmental Research Letters, 14(12), 124011. https://doi.org/10.1088/1748-9326/ab4667
- Harmel, T., & Chami, M. (2016). Estimation of daily photosynthetically active radiation (PAR) in presence of low to high aerosol loads: Application to OLCI-like satellite data. Optics Express, 24(22), A1390–A1407. https://doi.org/10.1364/OE.24.0A1390
- Harrison, L., Michalsky, J., & Berndt, J. (1994). Automated multifilter rotating shadow-band radiometer: An instrument for optical depth and radiation measurements. Applied Optics, 33(22), 5118–5125. https://doi.org/10.1364/AO.33.005118
- Holben, B. N., Eck, T. F., Slutsker, I., Tanré, D., Buis, J. P., Setzer, A., Vermote, E., Reagan, J. A., Kaufman, Y. J., Nakajima, T., Lavenu, F., Jankowiak, I., & Smirnov, A. (1998). AERONET—A Federated Instrument Network and data archive for aerosol characterization. Remote Sensing of Environment, 66(1), 1–16. https://doi.org/10.1016/S0034-4257(98)00031-5
- Jin, Z., Charlock, T. P., Smith, W. L., Jr., & Rutledge, K. (2004). A parameterization of ocean surface albedo. Geophysical Research Letters: Oceans, 31(22), L22301. https://doi.org/10.1029/2004GL021180
- Kiefer, D. A., & Mitchell, B. G. (1983). A simple, steady state description of phytoplankton growth based on absorption cross section and quantum efficiency. Limnology and Oceanography, 28, 770–776. https://doi.org/10.4319/lo.1983.28.4.0770
- Kwiatkowski, L., Bopp, L., Aumont, O., Ciais, P., Cox, P., Laufkötter, C., Li Yand Séférian, Y., & Séférian, R. (2017). Emergent constraints on projections of declining primary production in the tropical oceans. Nature Climate Change, 7(5), 355–358. https://doi.org/10.1038/nclimate3265
- Lavender, S., (2010). OLCI level 2 algorithm theoretical basis document, photosynthetically active Radiation, SENTINEL-3 optical products and algorithm definition, S3-L2-SD-03-C12-ARG-ATBD, 1–15, https://www.eumetsat.int/media/38637
- Liang, S., Zheng, T., Liu, R., Fang, H., Tsay, S., & Running, S. (2006). Estimation of incident photosynthetically active radiation from moderate resolution imaging spectrometer data. Journal of Geophysical Research: Atmospheres, 111(D15), 1–15. https://doi.org/10.1029/2005JD006730
- Liberti, G. L., D’Alimonte, D., diSarra, A., Mazeran, C., Voss, K., Yarbrough, M., Bozzano, R., Cavaleri, L., Colella, S., Cesarini, C., Kajiyama, T., Meloni, D., Pomaro, A., Volpe, G., Yang, C., Zagolski, F., & Santoleri, R. (2020). European radiometry buoy and infrastructure (EURYBIA): A contribution to the design of the European Copernicus infrastructure for Ocean colour system vicarious calibration. Remote Sensing, 12(7), 1178. https://doi.org/10.3390/rs12071178
- Li, L., Xin, X., Zhang, H., Yu, J., Liu, Q., Yu, S., & Wen, J. (2015). A method for estimating hourly photosynthetically active radiation (PAR) in China by combining geostationary and polar-orbiting satellite data. Remote Sensensing of Environment, 165, 14–26. https://doi.org/10.1016/j.rse.2015.03.034
- Long, C. N., & Ackerman, T. P. (2000). Identification of clear skies from broadband pyranometer measurements and calculation of downwelling shortwave cloud effects. Journal of Geophysical Research: Atmospheres, 105(D12), 15609–15626. https://doi.org/10.1029/2000JD900077
- Marconi, M., Sferlazzo, D. M., Becagli, S., Bommarito, C., Calzolai, G., Chiari, M., diSarra, A., Ghedini, C., Gómez-Amo, J. L., Lucarelli, F., Meloni, D., Monteleone, F., Nava, S., Pace, G., Piacentino, S., Rugi, F., Severi, M., Traversi, R., & Udisti, R. (2014). Saharan dust aerosol over the central Mediterranean sea: PM10 chemical composition and concentration versus optical columnar measurements. Atmospheric Chemistry and Physics, 14(4), 2039–2054. https://doi.org/10.5194/acp-14-2039-2014
- McCree, K. J. (1972). Test of current definitions of photosynthetically active radiation against leaf photosynthesis data. Agricultural Meteorolgy, 10, 443–453. https://doi.org/10.1016/0002-1571(72)90045-3
- Meloni, D., diSarra, A., Biavati, G., DeLuisi, J. J., Monteleone, F., Pace, G., Piacentino, S., & Sferlazzo, D. M. (2007). Seasonal behaviour of Saharan dust events at the mediterranean island of Lampedusa in the period 1999–2005. Atmospheric Environment, 41(14), 3041–3056. https://doi.org/10.1016/j.atmosenv.2006.12.001
- Meloni, D., diSarra, A., Brogniez, G., Denjean, C., De Silvestri, L., DiIorio, T., Formenti, P., Gómez-Amo, J. L., Gröbner, J., Kouremeti, N., Liuzzi, G., Mallet, M., Pace, G., & Sferlazzo, D. (2018). Determining the infrared radiative effects of saharan dust: A radiative transfer modelling study based on vertically resolved measurements at Lampedusa. Atmospheric Chemistry and Physics, 18(6), 4377–4401. https://doi.org/10.5194/acp-18-4377-2018
- Meloni, D., diSarra, A., Pace, G., & Monteleone, F. (2006). Aerosol optical properties at Lampedusa (central mediterranean). 2. Determination of single scattering albedo at two wavelengths for different aerosol types. Atmospheric Chemistry and Physics, 6(3), 715–727. https://doi.org/10.5194/acp-6-715-2006
- Meloni, D., Junkermann, W., diSarra, A., Cacciani, M., De Silvestri, L., DiIorio, T., Estellés, V., Gómez-Amo, J. L., Pace, G., & Sferlazzo, D. M. (2015). Altitude-resolved shortwave and longwave radiative effects of desert dust in the mediterranean during the GAMARF campaign: Indications of a net daily cooling in the dust layer. Journal of Geophysical Research: Atmospheres, 120(8), 3386–3407. https://doi.org/10.1002/2014JD022312
- Mercado, L. M., Bellouin, N., Sitch, S., Boucher, O., Huntingford, C., Wild, P. M., & Cox, P. M. (2009). Impact of changes in diffuse radiation on the global land carbon sink. Nature, 458(7241), 1014–1017. https://doi.org/10.1038/nature07949
- Miller, A. J., Alexander, M. A., Boer, G. J., Chai, F., Denman, K., Erickson, D. J., Frouin, R., Gabric, A. J., Laws, E. A., Lewis, M. R., Liu, Z., Murtugudde, R., Nakamoto, S., Neilson, D. J., Norris, J. R., Ohlmann, J. C., Perry, R. I., Schneider, N., Shell, K. M., & Timmermann, A. (2003). Potential feedbacks between Pacific Ocean ecosystems and interdecadal climate variations. Bulletin of the American Meteorological Society, 84(5), 617–634. https://doi.org/10.1175/BAMS-84-5-617
- Nakamoto, S., Prasana Kumar, S., Oberhuber, J. M., Muneyama, K., & Frouin, R. (2000). Chlorophyll control of sea surface temperature in the Arabian sea in a mixed layer isopycnal general circulation model. Geophysical Research Letters, 27(6), 747–750. https://doi.org/10.1029/1999GL002371
- Nakamoto, S., Prasanna Kumar, S., Oberhuber, J. M., Ishizaka, J., Muneyama, K., & Frouin, R. (2001). Response of the equatorial Pacific to chlorophyll pigments in a mixed layer isopycnal ocean general circulation model. Geophysical Research Letters, 28(10), 2021–2024. https://doi.org/10.1029/2000GL012494
- Nunez, M., Cantin, N., Steinberg, C., van Dongen-Vogels, V., & Bainbridge, S. (2022). Correcting PAR data from photovoltaic quantum sensors on remote weather stations on the great barrier reef. Journal of Atmospheric and Oceanic Technology, 39(4), 425–448. https://doi.org/10.1175/JTECH-D-21-0095.1
- Pace, G., diSarra, A., Meloni, D., Piacentino, S., & Chamard, P. (2006). Aerosol optical properties at lampedusa (Central Mediterranean). 1. Influence of transport and identification of different aerosol types. Atmospheric Chemistry and Physics, 6(3), 697–713. https://doi.org/10.5194/acp-6-697-2006
- Pace, G., Meloni, D., & diSarra, A. (2005). Forest fire aerosol over the mediterranean basin during summer 2003. Journal of Geophysical Research Atmospheres, 110, D21202. https://doi.org/10.1029/2005JD00598
- Ross, J., & Sulev, M. (2000). Sources of errors in measurements of PAR. Agricultural and Forest Meteorology, 100(2–3), 103–125. https://doi.org/10.1016/S0168-1923(99)00144-6
- Saux Picart, S., Sathyendranath, S., Dowell, M., Moore, T., & Platt, T. (2014). Remote sensing of assimilation number for marine phytoplankton. Remote Sensing of Environment, 146, 87–96. https://doi.org/10.1016/j.rse.2013.10.032
- Schmid, B., & Wehrli, C. (1995). Comparison of sun photometer calibration by use of the langley technique and the standard lamp. Applied Optics, 34(18), 4500–4512. https://doi.org/10.1364/AO.34.004500
- Sellitto, P., Zanetel, C., diSarra, A., Salerno, G., Tapparo, A., Meloni, D., Pace, G., Caltabiano, T., Briole, P., & Legras, B. (2017). The impact of mount Etna sulfur emissions on the atmospheric composition and aerosol properties in the central mediterranean: A statistical analysis over the period 2000-2013 based on observations and lagrangian modelling. Atmospheric Environment, 148, 77–88. https://doi.org/10.1016/j.atmosenv.2016.10.032
- Shell, K. M., Frouin, R., Nakamoto, S., & Somerville, R. C. J. (2003). Atmospheric response to solar radiation absorbed by phytoplankton. Journal of Geophysical Research: Atmospheres, 108(D15). https://doi.org/10.1029/2003JD003440
- Somayajula, S. A., Devred, E., Bélanger, S., Antoine, D., Vellucci, V., & Babin, M. (2018). Evaluation of sea-surface photosynthetically available radiation algorithms under various sky conditions and solar elevations. Applied Optics, 57(12), 3088–3105. https://doi.org/10.1364/AO.57.003088
- Su, W., Charlock, T. P., Rose, F. G., & Rutan, D. (2007). Photosynthetically active radiation from clouds and the Earth’s radiant energy system (CERES) products. Journal of Geophysical Research: Biogeosciences, 112(G2), G02022. https://doi.org/10.1029/2006JG000290
- Tan, J., Frouin, R., Jolivet, D., Compiègne, M., & Ramon, D. (2020). Evaluation of the NASA OBPG MERIS ocean surface PAR product in clear sky conditions. Optics Express, 28(22), 33157–33175. https://doi.org/10.1364/OE.396066
- Tao, X., Liang, S., He, T., & Jin, H. (2016). Estimation of fraction of absorbed photosynthetically active radiation from multiple satellite data: model development and validation. Remote Sensing of Environment, 184, 539–557. https://doi.org/10.1016/j.rse.2016.07.036
- Tao, X., Liang, S., & Wang, D. (2015). Assessment of five global satellite products of fraction of absorbed photosynthetically active radiation: Intercomparison and direct validation against ground-based data. Remote Sensing of Environment, 163, 270–285. https://doi.org/10.1016/j.rse.2015.03.025
- Trisolino, P., diSarra, A., Anello, F., Bommarito, C., DiIorio, T., Meloni, D., Monteleone, F., Pace, G., Piacentino, S., & Sferlazzo, D. (2018). A long-term time series of global and diffuse photosynthetically active radiation in the Mediterranean: Interannual variability and cloud effects. Atmospheric Chemistry and Physics, 18(11), 7985–8000. https://doi.org/10.5194/acp-18-7985-2018
- Trisolino, P., diSarra, A., Meloni, D., & Pace, G. (2016). Determination of global and diffuse photosynthetically active radiation from multi-filter shadowband radiometer (MFRSR. Applied Optics, 55(29), 8280–8286. https://doi.org/10.1364/AO.55.008280
- Vazyulya, S. V., Kopelevich, O. V., Sheberstov, S. V., & Artemiev, V. A. (2016). Estimation of sea surface solar radiation at 400-700 nm using satellite ocean color data, and its validation by ship data. Optics Express, 24(6), A604–A611. https://doi.org/10.1364/OE.24.00A604
- Vermote, E. F., Tanré, D., Deuzé, J. L., Herman, M., & Morcrette, J. J. (1997). Second simulation of the satellite signal in the solar spectrum, 6s: An overview. IEEE Transactions on Geoscience and Remote Sensing, 35(3), 675–686. https://doi.org/10.1109/36.581987
- Werkmeister, A., Lockhoff, M., Schrempf, M., Tohsing, K., Liley, B., & Seckmeye, G. (2015). Comparing satellite- to ground-based automated and manual cloud coverage observations – a case study. Atmospheric Measurement Techniques, 8(5), 2001–2015. https://doi.org/10.5194/amt-8-2001-2015
- Wielicki, B. A., & Parker, L. (1992). On the determination of cloud cover from satellite sensors: The effect of sensor spatial resolution. Journal of Geophysical Research Atmospheres, 97:(D12), 12799–12823. https://doi.org/10.1029/92JD01061
- Zhang, X., Liang, S., Zhou, G., Wu, H., & Zhao, X. (2014). Generating global land surface satellite incident shortwave radiation and photosynthetically active radiation products from multiple satellite data. Remote Sensing of Environment, 152, 318–332. https://doi.org/10.1016/j.rse.2014.07.003
- Zhang, Z., Zhao, L., & Lin, A. (2020). Evaluating the performance of sentinel-3A OLCI land products for gross primary productivity estimation using AmeriFlux data. Remote Sensing, 12(12), 1927. https://doi.org/10.3390/rs12121927