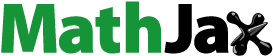
ABSTRACT
Evaluating the precision and applicability of high-quality precipitation products in the distinctive terrain and intricate climate of the Yunnan-Kweichow Plateau (YKP) is pivotal for climate research. This study comprehensively assesses four gridded precipitation datasets (AERA5-Asia, AIMERG, ERA5-Land, and IMERG-Final) using the China Meteorological Administration’s surface precipitation data. It employs eight statistical indicators and error decomposition methods at various spatiotemporal scales. The main findings are as follows: (1) AERA5-Asia, AIMERG, and IMERG-Final show similar precipitation patterns, with ERA5-Land overestimating. While all display minor seasonal variations, AERA5-Asia underestimates summer rain. ERA5-Land tends to overstate, whereas AIMERG and IMERG-Final are generally accurate but slightly undervalued in southern YKP. (2) Hourly analysis reveals AERA5-Asia leads in performance metrics (CC: 0.23, MAE: 0.49 mm/hour, RMSE: 0.18 mm/hour, CSI: 0.27). In contrast, ERA5-Land lags, marked by the lowest BIAS (35.39%), FAR (0.74), and FBI (2.85). AIMERG and IMERG-Final display comparable results but underperform in CC (0.16, 0.13), POD (0.31, 0.30), and CSI (0.19, 0.18). (3) False bias significantly contributes to the total bias of precipitation products. AERA5-Asia and AIMERG mitigate total bias and enhance false precipitation situations through calibration algorithms, albeit introducing missed bias in the central region of YKP. The study findings offer valuable insights into YKP precipitation, informing the development of grid-based fusion algorithms in the region’s complex terrain.
Introduction
Accurate precipitation data are paramount for efficient water resource allocation, early disaster warning, and the management of mountain flood risks and agricultural regulations (Irannezhad & Liu, Citation2022; Khan et al., Citation2023; Kim et al., Citation2022). However, the measurement of precipitation faces challenges due to its temporal and spatial variability influenced by climate change, geographical location, and topography (Peng et al., Citation2020; Yao et al., Citation2020). Acquiring high-quality precipitation data is vital to addressing climate change and formulating effective environmental protection policies (Lu et al., Citation2021; Prein & Gobiet, Citation2017; Zhang et al., Citation2022).
Recent advancements in earth observation technology and numerical modeling have yielded accurate and high-resolution precipitation datasets. These datasets are derived from ground-based rain gauges, weather radars, remote sensing satellites, and reanalysis datasets (Tang et al., Citation2023; Wu & Zhao, Citation2023). While ground-based instruments offer high accuracy, their coverage is limited by topography and requires regular maintenance, resulting in uneven distribution and limited representation of precipitation information (Le et al., Citation2023; Nawaz et al., Citation2020). Satellite-based sources use various sensors to generate high-resolution precipitation products through multisensor inversion and fusion correction (Wedajo et al., Citation2021). Satellite remote sensing technology, along with data inversion algorithms, facilitates in-depth research and application of satellite precipitation data, providing a better representation of regional precipitation’s spatial distribution with higher spatiotemporal resolution (Yu et al., Citation2020).
Despite these advantages, satellite observations are susceptible to errors, including cloud cover, surface characteristics, and data processing complexities (Xin et al., Citation2022). To address these limitations, various data quality improvement methods have been developed, such as CHIRPS (Funk et al., Citation2015), CMORPH (Joyce et al., Citation2004), PERSIANN-CDR (Ashouri et al., Citation2015), IMERG (Hou et al., Citation2014), FY-4A QPE (Li et al., Citation2021), and PECA-FY4A (S. Zhu & Ma, Citation2022), each combining different observational data sources. Model-based reanalysis precipitation products, generated through numerical models and assimilation of observational data, offer higher spatiotemporal resolution and accuracy (Baatz et al., Citation2021; Parker, Citation2016). However, uncertainties arise due to observational data, complex terrain, numerical models, and assimilation schemes, introducing errors in products like ERA-Interim (Dee et al., Citation2011), ERA5-Land (Muñoz-Sabater et al., Citation2021), MERRA-2 (Gelaro et al., Citation2017), JRA-55 (Kobayashi et al., Citation2015), and CRA-40 (Liu et al., Citation2023).
Gridded precipitation products, while valuable, exhibit biases and errors. Therefore, rigorous validation and evaluation are necessary (Chen et al., Citation2020; Soo et al., Citation2020; Zhao et al., Citation2022). Statistical indicators (Hoang et al., Citation2020; Torsri et al., Citation2022), hydrological models (Ji et al., Citation2022; Ren et al., Citation2018), and innovative methods like Triple Collocation (TC) (Duan et al., Citation2021; Tanim et al., Citation2021) and a double instrumental variable-based algorithm (IVd) (Dong et al., Citation2019) assess these products’ quality.
Many studies have explored the global/regional accuracy assessment of satellite remote sensing precipitation data, promoting the improvement and wider application of satellite precipitation products (Belete et al., Citation2020; Cooley et al., Citation2019). For instance, Xue et al. evaluated IMERG products and radar-based quantitative precipitation estimation (QPE), indicating IMERG’s superiority in capturing rainfall events, especially for light rain, compared to QPE (Xue Li et al., Citation2020). However, other studies revealed shortcomings of IMERG-Final, noting underestimation of heavy rainfall and errors in light rain estimation (Wang et al., Citation2017). Zhou et al. found that IMERG-Final underestimates precipitation in southern China but overestimates it in northern regions, particularly in the northwest (Zhou et al., Citation2020).
Additionally, research showed IMERG V06B’s good detection of light rain and snow in the Huang-Huai Plain but struggles in identifying heavy rainfall in summer and early autumn due to local climate and topography (Peng et al., Citation2020). Gomis-Cebolla et al. observed ERA5-Land/ERA5 overestimating low to moderate precipitation but underestimating heavy precipitation (Gomis-Cebolla et al., Citation2023). Furthermore, Xin et al.‘s comparison between ERA5-HRES and ERA5-Land highlighted stronger spatio-temporal variability in ERA5-Land due to inaccurate precipitation estimates at hourly and daily scales (Xin et al., Citation2021). These studies collectively emphasize uncertainty in the spatiotemporal distribution of precipitation products. Despite these efforts, discrepancies persist, and studies have highlighted the performance variations of products like IMERG, ERA5-Land, and others in different regions and climatic conditions.
Although global/regional-scale validation can reveal the macroscopic characteristics of various satellite precipitation products, different regions often exhibit varying performances among different products due to diverse properties such as terrain, climate, and land cover (Dahri et al., Citation2021; Nawaz et al., Citation2020). However, satellite-based precipitation entails notable uncertainties, limited temporal coverage, and relatively lower spatial resolutions, numerous researchers have diligently improved and applied various methodologies to address this issue (Michaelides et al., Citation2009). Particularly, the emergence of satellite remote sensing precipitation products and their integration using multiple approaches has significantly enhanced the quality of meteorological and hydrological precipitation data (Driscoll et al., Citation2000; Levizzani & Cattani, Citation2019). Some multi-source precipitation data fusion methods include Optimal Interpolation (OI) (Shen et al., Citation2014), Geographically Weighted Regression, GWR (Chao et al., Citation2018), Bayesian Model Averaging (BMA) (Sun et al., Citation2019), Region Probability Matching Method (RPMM) (Ayat et al., Citation2018), Long Short-Term Memory Network (LSTM) (Fang et al., Citation2019), Deep Belief Network (DBN) (Song et al., Citation2016), Generalized Regression Neural Network (GRNN) (Özerdem et al., Citation2017), and other methods.
For instance, Choi et al. use deep learning to enhance precipitation prediction. RAIN-F blends Radar, AWS ASOS, and IMERG Network for better forecasting. Using U-Net architecture, it outperforms radar-only forecasts, especially for heavy rainfall regions (Choi et al., Citation2021). 0Mo et al utilize data fusion methods (GWR, GDA, CDF) to combine station precipitation data and IMERG precipitation data, revealing IMERG data accuracy limitations. CDF-fused data quality exceeds others, aiding drought evaluation in the Chengbi River Basin (Mo et al., Citation2022). Ma et al. employed the innovative DTVCMDA fusion correction method, merging the ERA5-Land dataset with the high-quality APHRODITE dataset. The findings underscore AERA5-Asia’s remarkable superiority in resolution, consistency, and accuracy compared to gauge-based observations (Ma et al., Citation2022). Ma et al. improved daily AIMERG via new DSTDCA from APHRODITE/GPCC. mitigated systematic biases in IMERG, validated over China’s diverse terrain (Ma et al., Citation2020). These integration and correction methods have partially addressed potential drawbacks among various precipitation products, thereby enhancing overall product quality.
The Yunnan-Kweichow Plateau (YKP), with its unique topography and susceptibility to climate and geological disasters, requires precise precipitation data for effective socioeconomic planning and ecological conservation (Miao et al., Citation2007; Qiu et al., Citation2022). While previous studies have assessed specific satellite products on the YKP, a comprehensive analysis of multiple gridded precipitation products is lacking (Lu et al., Citation2020). This study aims to bridge this gap by evaluating four products (AERA5-Asia, AIMERG, ERA5-Land, and IMERG-Final) at various temporal scales against dense rain gauge observations. Additionally, the study employs error decomposition methods to understand the sources of errors in these products and their ability to detect precipitation events. The findings will contribute to improved water resource management, flood prevention, and drought mitigation on the YKP, offering valuable insights for future scientific research.
Study area and materials
Study area
The Yunnan-Kweichow Plateau (YKP), situated in southwestern China, extends between 100–111°E longitude and 22–30°N latitude (), representing one of China’s major plateaus. Encompassing the eastern section of Yunnan, the entirety of Guizhou, the northwestern expanse of the Guangxi Region, and bordering regions such as Sichuan, Hubei, and Hunan, the YKP is characterized by the convergence of mountain ranges aligning in both north-south and northeast-southwest directions across China (Hong et al., Citation2020; D. Zhu et al., Citation2022). The spatial depiction of the Digital Elevation Model (DEM) in illustrates the intricate topography of the YKP, featuring a gradual descent from northwest to southeast. The altitude ranges from 400 to 3500 m, with the majority of areas exceeding 1000 m. The terrain predominantly comprises hilly plateaus characterized by gentle slopes and undulating landscapes.
Figure 1. The spatial distribution of elevation and the network of 371 rain gauge stations across the Yunnan-Kweichow Plateau were utilized in the present study.

This study focuses on the time frame spanning 2010 to 2015. The YKP experiences a subtropical monsoon climate, marked by notable temperature and precipitation variations. Precipitation exhibits a general increase from south to north, with the rainy season, spanning April to October and constituting 85% to 95% of the total annual precipitation, witnessing frequent heavy precipitation events and ensuing floods. Conversely, the dry season, particularly the spring drought, is prolonged. The YKP receives an annual precipitation ranging between 600–2000 mm, showcasing a pronounced spatial variability. The eastern and southern regions receive higher precipitation levels, ranging from 1500–2000 mm, while the middle and northern areas witness a reduction to 500–600 mm. The rainy season prevails from May to October, contributing to approximately 80% of the annual precipitation (Rong & Zhang, Citation2023; Xiao et al., Citation2023).
Materials
Rain gauge measurements
Hourly rain gauge datasets from 371 national ground stations were utilized in this study, sourced from the China Meteorological Administration’s National Meteorological Information Center (NMIC) (http://data.cma.cn). These datasets, encompassing air temperature, air pressure, precipitation, wind, and other observations, underwent rigorous quality control, ensuring completeness and reliability illustrates the spatial distribution of ground stations in the Yunnan-Kweichow Plateau (YKP). While station density is relatively sparse in the northwest, it is more uniform in other areas, facilitating the investigation of precipitation distribution across the YKP.
AERA5-Asia data
AERA5-Asia, a high-quality precipitation dataset, integrates high temporal and spatial resolution with continuity considerations. This dataset employs the Daily Total Volume Controlled Merging and Disaggregation Algorithm (DTVCMDA). By combining continuous ERA5-Land data and the Asian Precipitation Highly Resolved Observational Data Integration Toward the Evaluation of Water Resources (APHRODITE) data, it generates a long-term series precipitation dataset for the Asian region. AERA5-Asia, available for download via the National Tibetan Platform/Third Pole Environment Data Center (https://data.tpdc.ac.cn/home), demonstrates superior performance in empirical evaluation indicators and regional extreme precipitation events (Ma et al., Citation2022). Detailed information on the satellite-based and model-based precipitation products used in this study is provided in .
Table 1. Summary of the gridded precipitation products used in this study.
AIMERG data
AIMERG, a high-quality, spatiotemporal resolution precipitation dataset for Asia, is published by the National Tibetan Platform/Third Pole Environment Data Center. Leveraging the Daily Spatio-Temporal Disaggregation Calibration Algorithm (DSTDCA), AIMERG calibrates GPM IMERG precipitation products using the APHRODITE ground observation product. This calibration results in high-quality precipitation datasets for the Asian region, outperforming IMERG in various space-time scales in the Chinese Mainland. AIMERG is accessible for download at https://data.tpdc.ac.cn/home (Ma et al., Citation2020).
ERA5-land data
ERA5-Land, a reanalysis dataset, provides high spatiotemporal resolution land variable evolution data derived from reproducing land components in the ECMWF ERA5 climate reanalysis. Offering dynamic data for various indicators, this dataset enables the study of land variable trends. It can be obtained through C3S climate data access (https://cds.climate.copernicus.eu/#!/home) (Muñoz-Sabater et al., Citation2021).
IMERG-final data
The IMERG dataset, a multi-satellite fusion inversion long-term time series based on Global Precipitation Measurement (GPM), incorporates satellite precipitation retrieval algorithms from the TRMM era. IMERG-Final, adjusted monthly using a spatially variable ratio, is widely applied in flood forecasting, agricultural drought, and climate change research. This data can be downloaded through GES DISC (https://disc.gsfc.nasa.gov/) (Hou et al., Citation2014).
Methodology
Evaluation metrics
The assessment of AERA5-Asia, AIMERG, ERA5-Land, and IMERG-Final products was conducted across various scales using a comprehensive set of validation metrics. These metrics include:
Correlation Coefficient (CC): Describing the consistency between precipitation products and rain gauge data.
Relative Bias (BIAS): Illustrating the deviation between precipitation products and rain gauge data.
Root Mean Square Error (RMSE): Representing the square root of the mean of the squared errors between predicted and actual observed values.
Mean Absolute Error (MAE): Signifying the mean absolute value of the errors between predicted and actual observed values.
Probability of Detection (POD): Expressing the ratio of correctly detected precipitation events by the satellite to all actual precipitation events.
False Alarm Ratio (FAR): Depicting the ratio of false alarm precipitation events to the detected precipitation events.
Critical Success Index (CSI): Reflecting the ratio of correctly detected precipitation events by the overall satellite.
Frequency Bias Index (FBI): Capturing the trend of overestimated or underestimated precipitation occurrence rates detected by satellites.
Error decomposition
The error decomposition method (Tian et al., Citation2009) was employed to analyze the error components of remote-sensing precipitation products. The total bias calculated was decomposed into three independent components:
Missed Bias (MB): Rain areas incorrectly determined as no-rain areas by precipitation products.
False Bias (FB): No-rain areas incorrectly determined as rain areas by precipitation products.
Hit Bias (HB): Rain areas are correctly determined by precipitation products but with inaccurately estimated precipitation intensity.
It is crucial to note that the threshold for judging the occurrence of precipitation events was set at 0.1 mm/h. The equations for all verification metrics and error composition schemes are provided in .
Table 2. List of the verification metrics and error decomposition method for evaluating precipitation products in this study.
Statistical analysis
In addition to the aforementioned metrics, a thorough statistical analysis was conducted to elucidate the strengths and weaknesses of each precipitation product. This analysis involved comparing their performance under varying temporal scales, such as yearly, seasonal, monthly, daily, and hourly, against dense rain gauge observations over the Yunnan-Kweichow Plateau. The results of this analysis offer valuable insights into the applicability and reliability of the precipitation products in capturing the complex spatiotemporal patterns of precipitation in the study region.
Results
Spatial patterns of precipitation over the YKP at the annual scale
The annual precipitation patterns for the year 2010 across the Yunnan-Kweichow Plateau (YKP) are illustrated in . To facilitate effective comparisons with other products, the inverse distance weighting (IDW) method is employed for interpolating annual average precipitation from rain gauges, generating a spatial continuity map.
Figure 2. Annual precipitation in 2010 (a) Rain gauge data, (b) Spatial-continuous rain gauge data based on the IDW interpolation method, (c) AERA5-Asia, (d) AIMERG, (e) ERA5-land, and (f) IMERG-Final over the YKP.

The spatial distribution reveals a discernible increasing trend from the northwest to the southeast over the YKP (). Regions exhibiting high precipitation (>2150 mm/year) are predominantly located in the southeast, contrasting with lower precipitation levels (<650 mm/year) in the west. AERA5-Asia, AIMERG, IMERG-Final, and rain gauge data demonstrate similar spatial distributions. AERA5-Asia () and rain gauge data exhibit comparable patterns, albeit with a slight underestimation in northern Guangxi and eastern Hunan (>1900 mm/year). AIMERG () slightly underestimates in most regions compared to ground observations and the other three precipitation products. This discrepancy is attributed to the utilization of APHRODITE in the fusion of AERA5-Asia and AIMERG, which tends to underestimate precipitation in mountainous lake areas (Prakash et al., Citation2015). ERA5-Land () significantly overestimates precipitation in the eastern part of the YKP (>2150 mm/year) and the southeastern part of Sichuan (>1900 mm/year) but underestimates in the eastern part of Yunnan (<650 mm/year). This aligns with previous findings indicating an overall overestimation of precipitation (Bandhauer et al., Citation2022). In contrast, IMERG-Final () better captures precipitation over the YKP but underestimates in western Guangxi and eastern Yunnan (<900 mm/year). Generally, compared to interpolated surface precipitation data, precipitation products offer a more comprehensive characterization of the precipitation structure. However, the complexity of terrain and climate in this region poses challenges to the effectiveness of precipitation products. Further algorithm adjustments are warranted to enhance alignment with actual ground precipitation values.
Assessment of multiple precipitation products at monthly and daily scale
illustrates the monthly mean precipitation in the Yunnan-Kweichow Plateau (YKP) from 2010 to 2015 using AERA5-Asia, AIMERG, ERA5-Land, IMERG-Final, and ground observation datasets. The time series trends of AERA5-Asia, AIMERG, and IMERG-Final closely mirror each other, with IMERG-Final showing strong alignment with ground station observations. Conversely, ERA5-Land consistently overestimates precipitation, particularly during the summer months. Seasonally, in winter and spring, AERA5-Asia, AIMERG, and IMERG-Final closely resemble observed rainfall data from rain gauges, but discrepancies become more pronounced in summer and autumn. IMERG-Final, in comparison to rain gauge data, exhibits a closer alignment with ground observations over time. AERA5-Asia and AIMERG tend to underestimate summer precipitation, especially in high-altitude areas. Notably, ERA5-Land displays significant fluctuations and exhibits the poorest temporal distribution characteristics. Overall, while the datasets are generally consistent with the broader YKP pattern and ground data, systematic errors in precipitation products emerge over longer time scales. These disparities can be attributed to substantial altitude differences, complex terrain conditions, and the influence of the monsoon climate in Southeast Asia (Loo et al., Citation2015).
Figure 3. Temporal variations of the area mean monthly precipitation over the YKP estimated by AERA5-Asia, AIMERG, ERA5-land, IMERG-Final, and ground observation datasets from 2010 to 2015.

To evaluate the performance of AERA5-Asia, AIMERG, ERA5-Land, and IMERG-Final precipitation products, individual validations against rain gauge data were conducted. presents the validation results for June, July, and August 2010.
Figure 4. Validations of (a) AERA5-Asia, (b) AIMERG, (c) ERA5-land, and (d) IMERG-Final data against ground observations at a monthly scale over the YKP in the summer of 2010.

During the summer, AERA5-Asia demonstrates excellent performance, surpassing others with a high correlation coefficient (CC) of 0.87 and a low mean absolute error (MAE) of 44.85 mm/month. However, concerning the root mean square error (RMSE), IMERG-Final exhibits the lowest RMSE, while ERA5-Land performs less favorably with a higher value of 91.93 mm/month.
In comparison to ground observations, AERA5-Asia and AIMERG slightly overestimate precipitation at 64.08 mm/month and 68.76 mm/month, respectively. It’s noteworthy that some higher observed values in each scatter map result from challenges in accurately estimating precipitation from these products in the northwest of the YKP.
These findings underline the nuanced performance of each product, emphasizing the need for a comprehensive understanding of their strengths and limitations, particularly in regions with challenging climatic and topographic conditions.
illustrates the spatial patterns of the four evaluated products against ground observations at the daily scale over the YKP in 2010, focusing on correlation coefficient (CC), bias, root mean square error (RMSE), and mean absolute error (MAE). AERA5-Asia and AIMERG exhibit similar and commendable performance across various daily indicators. AERA5-Asia, surpassing the other products with a CC exceeding 0.6 in most parts of the YKP, outperforms in terms of correlation. Conversely, ERA5-Land demonstrates the weakest correlation, particularly in mountainous and northwest parts of Sichuan, showing a weak correlation with observed rainfall gauge data. In terms of bias indicators, IMERG-Final generally performs well, while AERA5-Asia and AIMERG show moderate performance, with a deviation between −30% and 15%. Notably, ERA5-Land exhibits the poorest performance, especially in southeast Sichuan, northeast Yunnan, and northern Guangxi provinces. Regarding RMSE and MAE, AERA5-Asia and AIMERG perform better in the west of the YKP but worse in the east. Conversely, ERA5-Land and IMERG-Final struggle, particularly in Guangxi and western Hunan, due to the complex terrain of the western YKP, including high-altitude mountains, hills, watersheds, and basins. Despite providing more spatial details, ERA5-Land is less effective in providing accurate daily precipitation information compared to other products. In summary, AERA5-Asia and AIMERG demonstrate better overall performance, albeit with challenges in accurately capturing heavy precipitation in the western YKP influenced by complex terrain.
Assessment of multiple precipitation products at an hourly scale
Spatial patterns of performance metrics for AERA5-Asia, AIMERG, ERA5-Land, and IMERG-Final at the hourly scale over the YKP from 2010 to 2015 are presented in . The products exhibit consistent performance at both hourly and daily scales, revealing similar spatial patterns. For the correlation coefficient (CC) indicator, spatial distribution is generally consistent, averaging between 0.2 and 0.35, increasing from the bottom left to the top right. However, notable performances include AERA5-Asia and ERA5-Land excelling in the northwest of Hunan and Chongqing (CC > 0.4), while AIMERG and IMERG-Final exhibit weaker performance in some areas, particularly in parts of southern Chongqing and eastern Sichuan (CC < 0.2). In terms of bias indicators, ERA5-Land demonstrates the highest bias, exceeding 60% in YKP. AERA5-Asia and AIMERG indicate comparatively poorer performance (−35% to 0%), while IMERG-Final shows better overall performance with deviations in specific areas. Concerning root mean square error (RMSE), all products consistently demonstrate spatial performance ranging from 0.7 mm to 1.7 mm. AERA5-Asia performs best, especially in eastern Yunnan (<0.7 mm). For mean absolute error (MAE), AERA5-Asia, AIMERG, and IMERG-Final perform similarly, excelling in the west of the YKP (<0.19 mm). However, ERA5-Land performs less effectively (0.19 mm to 0.34 mm), particularly in the southeast of Guangxi (>0.34 mm).
Figure 6. Spatial patterns of performance indicators (CC, bias, RMSE, MAE) for AERA5-Asia, AIMERG, ERA5-land, and IMERG-Final at the hourly scale over the YKP from 2010 to 2015.

Spatial patterns of hourly scale probability of detection (POD), false alarm ratio (FAR), critical success index (CSI), and frequency bias index (FBI) are presented in . AERA5-Asia and ERA5-Land perform well in POD, FAR, CSI, and FBI, with AERA5-Asia showing high CSI indicators (>0.35). ERA5-Land excels in POD (>0.7) in both the western and eastern parts of YKP, while IMERG-Final and AIMERG exhibit lower POD values, particularly in Guizhou, eastern Sichuan, and western Chongqing. In FAR indicators, AERA5-Asia, AIMERG, and IMERG-Final perform well in mountainous areas, with FAR generally below 0.65. AIMERG exhibits the best performance (<0.55), while ERA5-Land performs the worst (0.65 to 0.75), especially in the western part of YKP. For CSI and FBI indicators, AERA5-Asia and ERA5-Land show superior performance, with CSI indicators showing a gradually increasing trend from the bottom left to the top right. IMERG-Final and AIMERG exhibit lower CSI values (<0.35) in the western and eastern regions of YKP, while ERA5-Land’s FBI indicators are higher (>2.1) in certain regions, particularly in southern Sichuan and Yunnan. AERA5-Asia’s FBI is mostly in a higher range (from 1.2 to 2.1), while IMERG-Final and AIMERG’s FBI are mainly below 1.2.
Figure 7. Spatial patterns of performance indicators (POD, FAR, CSI, FBI) for AERA5-Asia, AIMERG, ERA5-land, and IMERG-Final at the hourly scale over the YKP from 2010 to 2015.

illustrates the numerical distributions of verification metrics for AERA5-Asia, AIMERG, ERA5-Land, and IMERG-Final at an hourly scale. Among the four precipitation products, AERA5-Asia performed the best, followed by AIMERG and IMERG-Final, with ERA5-Land performing the worst. AERA5-Asia demonstrated superior performance in CC (0.23), RMSE (0.49 mm/hour), MAE (0.18 mm/hour), and CSI (0.27), highlighting its accuracy in capturing precipitation. IMERG-Final exhibited the best average BIAS value at 5.56%, indicating a small deviation in precipitation estimation. In contrast, AERA5-Asia, AIMERG, and ERA5-Land showed average BIAS values of −12.44%, −19.14%, and 35.39%, respectively. ERA5-Land exhibited poor performance with data fluctuations and numerous outliers, suggesting overestimation of precipitation events and inconsistency. AIMERG and IMERG-Final performed similarly in POD and FBI, with average POD values of approximately 0.31 and 0.30, respectively, while AERA5-Asia and ERA5-Land had average POD values of approximately 0.61 and 0.71, indicating that reanalysis data and fusion correction data based on it can accurately capture actual precipitation events. For FBI, the average values of AERA5-Asia and ERA5-Land were both large and greater than 1, indicating that these two products overestimated precipitation events on an hourly scale, while AIMERG and IMERG-Final tended to underestimate precipitation events. In FAR, AIMERG averaged about 0.65, indicating fewer false alarms for reporting precipitation events. Conversely, ERA5-Land averaged about 0.74, displaying poor performance in false precipitation event reporting. Regarding CSI, AERA5-Asia excelled with an average value of 0.27, indicating a strong ability to detect precipitation events correctly. AIMERG and IMERG-Final averaged 0.19 and 0.18, respectively. At the hourly resolution, all four products showcased good statistical indicators, although variations were notable across different evaluation criteria.
Error components analysis of precipitation products
The present study employs error component analysis to elucidate the overall bias of four precipitation products (AERA5-Asia, AIMERG, ERA5-Land, and IMERG-Final) in the YKP. This method provides a nuanced understanding of the main components contributing to precipitation product errors, aiding researchers in product selection and guiding future development. illustrates the spatial distribution of the overall error and three independent error components at the hourly scale over the YKP for these four precipitation products from 2010 to 2015, shedding light on the diverse error sources influencing bias. The total bias of the four precipitation products and the three independent biases exhibit similar spatial distribution characteristics. Notably, false bias emerges as the predominant error component in the YKP for all four precipitation products, constituting a substantial portion of the total bias. Despite this, the overall bias in the region remains relatively low due to a balance between positive false bias, negative hit biases, and missed biases. This balance may create a misleading perception of minimal overall error and underscores the importance of utilizing error decomposition methods for accurate analysis of precipitation product errors. In direct comparison, AERA5-Asia and AIMERG demonstrate more favorable total bias performance (ranging from 0% to −30%). IMERG-Final registers a total bias index between −10% and 20%, while ERA5-Land exhibits the poorest performance, especially in the western YKP, where total bias exceeds 40%. Concerning hit bias, all precipitation products exhibit negative values. IMERG-Final and AIMERG perform well (ranging from 0% to 20%), while ERA5-Land performs less effectively (ranging from −20% to −35%). AERA5-Asia exhibits the poorest hit bias performance (ranging from −25% to −60%), indicating accurate capture of precipitation events but requiring improvements in detecting precipitation intensity. In terms of missed bias, ERA5-Land and AERA5-Asia perform well (ranging from −25% to 10%), while AERA5-Asia displays poorer missed bias values in the central and southern regions of YKP. The false bias component is significant, with the false bias index ranging from 30% to 90% for all four precipitation products. AERA5-Asia and AIMERG exhibit more favorable false bias values (ranging from 20% to 60%).
Figure 9. Spatial patterns of error components in precipitation estimates relative to ground observations data over the YKP at the hourly scale from 2010 to 2015.

illustrates the time series changes of total bias and three independent error components of the four precipitation products in YKP in 2010. To enhance clarity and mitigate visual interference, a 10-day moving average method was applied. Overall, all four precipitation products exhibit distinct seasonal variations. In summer, the patterns remain stable, indicating efficient detection of warm-season precipitation. However, during winter, higher amplitudes and weaker detection capability lead to increased errors. AERA5-Asia and AIMERG show similar temporal variations in total bias and its error components. Their temporal changes are relatively stable, with significant seasonal differences, particularly in summer and winter. The missed bias indicator increases, while the false bias decreases significantly, leading to a smoother overall trend. ERA5-Land exhibits high values for total bias, hit bias, and false bias indicators, while the missed bias indicator is close to 0 and consistent with the spatial distribution. It shows inferior performance compared to satellite products, especially during winter, highlighting a deterioration in detection capability and higher errors. IMERG-Final demonstrates larger overall variations, with significant fluctuations, especially in summer when errors in the other two corrected products remain relatively stable. The magnitude of false precipitation slightly exceeds that of missed precipitation, and they largely offset each other. False bias plays a decisive role in total bias, and YKP, particularly in the eastern region, exhibits remarkable temporal changes with distinct seasonal dependence in error characteristics.
Discussion
Accurate and high-spatiotemporal resolution precipitation data are vital for regional climate studies, water resource management, and agricultural practices (Aksu & Akgül, Citation2020; Reda et al., Citation2021). The present study conducts a comprehensive evaluation and error decomposition analysis of precipitation products, utilizing ground station data from 2010 to 2015 in the YKP. The findings unveil nuanced insights into the performance of AERA5-Asia, AIMERG, ERA5-Land, and IMERG-Final, shedding light on their strengths and limitations in capturing precipitation dynamics over this complex terrain.
Summer precipitation underestimation
A noteworthy observation is the underestimation of summer precipitation in the YKP by AERA5-Asia and AIMERG. This tendency may be linked to the underestimation of monsoonal rainfall, especially in high-altitude areas, by the APHRODITE dataset (Luo et al., Citation2020). Conversely, IMERG-Final exhibits better alignment with ground observations during summer, possibly attributed to the calibration of GPCC monthly data and subsequent adjustments using spatially varying ratios (Jackson et al., Citation2018). ERA5-Land, however, displays pronounced fluctuations and poorer representation of temporal distribution characteristics, resulting in an overestimation of precipitation in humid and topographically complex regions (Jiang et al., Citation2021).
Impact of complex terrain on performance
In the complex terrain of the western YKP, both ERA5-Land and IMERG-Final face challenges in high-altitude mountains, hills, watersheds, and basins (Xie et al., Citation2022). IMERG-Final’s accuracy in these regions is influenced by topography, moisture flux (Kirstetter, Citation2022), and landform types (Xin et al., Citation2022). While ERA5-Land provides more spatial details, it proves less effective in delivering accurate daily-scale precipitation information compared to other products. AERA5-Asia and AIMERG, incorporating corrections through APHRODITE fusion, face challenges in accuracy due to sparse ground observation stations and the influence of complex terrain in the western region (Luo et al., Citation2020; Mannig et al., Citation2013).
Performance comparison among products
Compared to ground observations, IMERG-Final generally outperforms ERA5-Land, particularly in terms of BIAS and MAE, highlighting the advantage of satellite-based precipitation products over model-based ones, especially in mid-latitudes (Xu et al., Citation2022). AERA5-Asia and AIMERG exhibit similar performance indicators, with limited calibration effects in local areas due to inadequate observational data in mountainous regions. AIMERG, with the introduction of APHRODITE for calibration, outperforms IMERG-Final in bias at different temporal and spatial scales (Ma et al., Citation2020). However, limitations such as terrain, climate, and ground calibration stations hinder IMERG-Final’s ability to accurately capture precipitation events in the YKP (Gan et al., Citation2022; Nan et al., Citation2021). ERA5-Land simulates land fields with high spatial resolution but tends to overestimate hourly and daily precipitation, showing significant deviations in capturing precipitation events (Xie et al., Citation2022).
Error decomposition insights
The error decomposition method, widely used in precipitation error analysis studies, provides additional insights (Ning et al., Citation2017; Tong et al., Citation2014; Zhang et al., Citation2021; Zhou et al., Citation2020). AIMERG and IMERG-Final exhibit little variation in hit bias and missed bias in the YKP, primarily due to the overall improvement of false precipitation in AIMERG. ERA5-Land tends to overestimate precipitation in central and western YKP, primarily due to false bias. AIMERG improves the underestimation of precipitation and hit precipitation events in the central part of the YKP, enhancing hit bias. AERA5-Asia and ERA5-Land demonstrate differences in the spatial distribution of total bias and its components. False bias accounts for a significant portion, but hit bias and missed bias should not be neglected. Calibration algorithms contribute to reducing these errors in pure satellite and model-based products. ERA5-Land effectively corrects high false bias, compensating for false precipitation and improving AERA5-Asia’s total bias.
Seasonal variations and calibration impact
The four precipitation products exhibit significant seasonal differences, with relatively smooth patterns during the summer and larger errors during the winter. These variations may stem from the efficiency of satellite sensors, complex terrain, and regional climatic features. AERA5-Asia and AIMERG show stable performance with smaller error variations, while ERA5-Land and IMERG-Final exhibit larger fluctuations, especially during the winter. The introduction of calibration algorithms and ground station data positively contributes to error improvements. However, the performance varies across regions and seasons, influenced by factors such as climate variations, terrain conditions, and the uncertainty of atmospheric variable inputs.
Considerations for product evaluation and application
In evaluating and applying these precipitation products, it is essential to consider their seasonal differences, spatial distribution characteristics, and the strengths and limitations of each product. The distinct responses to seasonal variations and varying performance in different terrains underscore the need for a nuanced understanding when employing these products in climatological and hydrological research on the YKP.
While satellite-derived precipitation data has demonstrated potential application in forecasting and generally aligns with measured data, using solely satellite-derived precipitation data for forecasting studies, given current technological limitations, often falls short in meeting the precision requirements at smaller time scales in scientific research and production (Michaelides et al., Citation2009). Therefore, incorporating ground-based observed precipitation data for calibration remains necessary to mitigate the impact of errors in satellite-derived precipitation data on forecasts. Moreover, further fusion and calibration processes of satellite precipitation data are essential to improve data accuracy and enhance the applicability of satellite remote sensing precipitation data.
Impact of uncertainties in meteorological observations
Meteorological observations, essential for accurate weather assessments, confront inherent uncertainties demanding meticulous handling. The variability in instrument calibration, especially impacting precipitation measurements, underscores the crucial need for regular calibration. Addressing these uncertainties not only improves observation accuracy but also enhances the reliability of weather predictions. Exploring uncertainties in observational precipitation data, covering measurement accuracy and validation procedures, is indispensable for comprehensive reliability assessments.
Observational uncertainties markedly impact climate analyses, notably in the spatial and temporal variability of precipitation records. Regions with high latitudes, mountainous terrain, and sparse data face pronounced uncertainties, including sampling errors tied to station density and influenced by topography, seasonality, temporal resolution, and precipitation type (Schneider et al., Citation2014). Interpolating irregular rain-gauge measurements onto grids presents challenges and advantages, enabling direct climate model evaluation and region-based averaging. However, it introduces difficulties like spatial smoothing and potential underestimation of extremes (Hofstra et al., Citation2010). Inhomogeneities in precipitation records, especially in high latitudes and mountainous regions, significantly affect climate change analyses (Prein & Gobiet, Citation2017). Addressing these uncertainties in climate model evaluation and bias correction is crucial for enhancing overall meteorological observation accuracy.
Conclusion
This study comprehensively evaluated four precipitation products – AERA5-Asia, AIMERG, ERA5-Land, and IMERG-Final – using precipitation data from the YKP provided by CMA spanning 2010 to 2015. Comparisons with rain gauge data revealed distinctive performance characteristics among the evaluated products at different temporal and spatial scales. Key conclusions drawn from this study include, but are not limited to, the following:
Spatial and temporal patterns
Analyzing the spatial and temporal dynamics of precipitation across the YKP reveals distinctive patterns in various products. AERA5-Asia, AIMERG, and IMERG-Final align closely with YKP’s spatiotemporal variations, showing nuanced seasonal changes. AERA5-Asia mirrors ground stations but tends to underestimate precipitation in mountainous regions, while ERA5-Land consistently overestimates, especially in the eastern YKP during summer and autumn. Understanding these intricacies is crucial for precise precipitation assessments.
Performance across metrics
Comprehensive evaluation across multiple metrics underscores the absence of a single product excelling in all indicators. AERA5-Asia stands out with superior correlation coefficients (CC) and critical success index (CSI), along with lower mean absolute error (MAE) and root mean square error (RMSE). In contrast, AIMERG and IMERG-Final exhibit suboptimal performance in specific regions, and ERA5-Land ranks lower in Bias and MAE but excels in other indicators. This diverse metric assessment aids in product selection based on specific evaluation criteria.
Error sources and mitigation
Managing false precipitation remains a significant challenge in various products, creating a delicate task for calibration algorithms to strike a balance between minimizing false events and avoiding missed events. While the APHRODITE correction enhances precipitation event capture, it introduces potential missed errors in specific regions. The spatial distribution of three distinct error components plays a crucial role in shaping the perception of total bias. Future efforts should prioritize advanced calibration algorithms and refined correction methodologies, aiming for an optimal balance between minimizing false events and accurately capturing precipitation.
Integrated insights and future prospects
This study provides a nuanced analysis of error distribution and uncertainties in precipitation datasets over the YKP, offering valuable insights for meteorological and hydrological dataset selection. Future research can build upon these findings, addressing specific challenges in each product, such as underestimation and missed events in AERA5-Asia and AIMERG, overestimation in ERA5-Land, and erroneous events in IMERG-Final, especially in central and western YKP.
Spatial and various multi-source precipitation fusion products exhibit deficiencies arising from disparities in regional precipitation monitoring capabilities, reliance on inferred ground-based measurements, and seasonal accuracy variations. Strengthening multi-sensor joint inversion algorithms is imperative for enhancing satellite-based precipitation fusion products. Applicability analyses based on local climatic conditions are essential for optimal product selection. The integration of surface data and mainstream hydrological models, such as VIC, enhances accuracy, offering a comprehensive understanding of precipitation product strengths and limitations in the YKP.
Satellite remote sensing is pivotal for evaluating precipitation in challenging terrains like the Qinghai-Tibet Plateau, Himalayas, and oceans, thereby improving extreme event forecasting. While tracking storm precipitation evolution, algorithms deriving rainfall from high-resolution satellite data may yield false positives and negatives, necessitating careful scrutiny. Bridging disparities between satellite and ground data is vital for precision, requiring a thorough exploration of observational precipitation uncertainties, biases, and limitations. Furthermore, evaluating GPM IMERG and SM2RAIN-ASCAT over the Qinghai-Tibet Plateau, coupled with examining global datasets like IMERG and ERA5, offers valuable insights for future research.
Author contributions
Conceptualization, H. L.; Data curation, Y. Y., X. L., and S. Y.; Formal analysis, T. L.; Funding acquisition, H. L.; Methodology, T. L.; Project administration, Q. X.; Resources, J. R. and J. H.; Supervision, H. L.; Validation, T. L.; Visualization, T. L.; Writing – original draft, T. L.; Writing – review & editing, H. L. and Z. Z. All authors have read and agreed to the published version of the manuscript.
Acknowledgments
We express our gratitude to the providers of operational precipitation products and rain gauge measurements for generously making their data available to us.
Disclosure statement
No potential conflict of interest was reported by the author(s).
Data availability statement
We express our sincere appreciation to the data providers, particularly the CMPA provider (http://data.cma.cn). The AERA5-Asia products are accessible for download at https://data.tpdc.ac.cn/zh-hans/data/7bb5feae-c1c9–4677-87d6-5c64dba659cd/, last accessed on 5 January 2023. Likewise, the AIMERG products can be obtained from https://data.tpdc.ac.cn/zh-hans/data/1090dead-dbba-44c8-980c-ca4c631d3d5c, accessed on the same date. For the IMERG-Final products, they are available for download at https://disc.gsfc.nasa.gov/datasets/GPM_3IMERGHH_06/summary?keywords=%22IMERG%20final%22, accessed on 5 January 2023. Furthermore, the ERA5-Land reanalysis datasets can be acquired from https://cds.climate.copernicus.eu/cdsapp#!/dataset/reanalysis-era5-land?tab=overview, accessed on the same date. Our profound gratitude extends to these organizations for generously providing the data indispensable to this study.
Additional information
Funding
References
- Aksu, H., & Akgül, M. (2020). Performance evaluation of CHIRPS satellite precipitation estimates over Turkey. Theoretical and Applied Climatology, 142(1–2), 71–19. https://doi.org/10.1007/s00704-020-03301-5
- Ashouri, H., Hsu, K.-L., Sorooshian, S., Braithwaite, D. K., Knapp, K. R., Cecil, L. D., Nelson, B. R., & Prat, O. P. (2015). PERSIANN-CDR: Daily Precipitation Climate Data Record from Multisatellite Observations for Hydrological and Climate Studies. Bulletin of the American Meteorological Society, 96(1), 69–83. https://doi.org/10.1175/BAMS-D-13-00068.1
- Ayat, H., Reza Kavianpour, M., Moazami, S., Hong, Y., & Ghaemi, E. (2018). Calibration of weather radar using region probability matching method (RPMM). Theoretical and Applied Climatology, 134(1), 165–176. https://doi.org/10.1007/s00704-017-2266-7
- Baatz, R., Hendricks Franssen, H. J., Euskirchen, E., Sihi, D., Dietze, M., Ciavatta, S., Fennel, K., Beck, H., De Lannoy, G., Pauwels, V. R. N., Raiho, A., Montzka, C., Williams, M., Mishra, U., Poppe, C., Zacharias, S., Lausch, A., Samaniego, L. … Vereecken, H. (2021). Reanalysis in earth system science: Toward terrestrial ecosystem reanalysis. Reviews of Geophysics, 59(3), e2020RG000715. https://doi.org/10.1029/2020RG000715
- Bandhauer, M., Isotta, F., Lakatos, M., Lussana, C., Båserud, L., Izsák, B., Szentes, O., Tveito, O. E., & Frei, C. (2022). Evaluation of daily precipitation analyses in E-OBS (v19.0e) and ERA5 by comparison to regional high-resolution datasets in European regions. International Journal of Climatology, 42(2), 727–747. https://doi.org/10.1002/joc.7269
- Belete, M., Deng, J., Wang, K., Zhou, M., Zhu, E., Shifaw, E., & Bayissa, Y. (2020). Evaluation of satellite rainfall products for modeling water yield over the source region of Blue Nile Basin. Science of the Total Environment, 708, 134834. https://doi.org/10.1016/j.scitotenv.2019.134834
- Chao, L., Zhang, K., Li, Z., Zhu, Y., Wang, J., & Yu, Z. (2018). Geographically weighted regression based methods for merging satellite and gauge precipitation. Journal of Hydrology, 558, 275–289. https://doi.org/10.1016/j.jhydrol.2018.01.042
- Chen, C., Chen, Q., Qin, B., Zhao, S., & Duan, Z. (2020). Comparison of different methods for spatial downscaling of GPM IMERG V06B satellite precipitation product over a typical arid to semi-arid area. Frontiers in Earth Science, 8, 8. https://doi.org/10.3389/feart.2020.536337
- Choi, Y., Cha, K., Back, M., Choi, H., & Jeon, T.(2021). Rain-F: A fusion dataset for rainfall prediction using convolutional neural network. Proceedings of the 2021 IEEE International Geoscience and Remote Sensing Symposium IGARSS. https://doi.org/10.1109/igarss47720.2021.9555094
- Cooley, S. S., Williams, C. A., Fisher, J. B., Halverson, G. H., Perret, J., & Lee, C. M. (2019). Assessing regional drought impacts on vegetation and evapotranspiration: A case study in Guanacaste, Costa Rica. Ecological Applications, 29(2), e01834. https://doi.org/10.1002/eap.1834
- Dahri, Z. H., Ludwig, F., Moors, E., Ahmad, S., Ahmad, B., Shoaib, M., Ali, I., Iqbal, M. S., Pomee, M. S., Mangrio, A. G., Ahmad, M. M., & Kabat, P. (2021). Spatio-temporal evaluation of gridded precipitation products for the high-altitude indus basin. International Journal of Climatology: A Journal of the Royal Meteorological Society, 41(8), 4283–4306. https://doi.org/10.1002/joc.7073
- Dee, D. P., Uppala, S. M., Simmons, A. J., Berrisford, P., Poli, P., Kobayashi, S., Andrae, U., Balmaseda, M. A., Balsamo, G., Bauer, P., Bechtold, P., Beljaars, A. C. M., van de Berg, L., Bidlot, J., Bormann, N., Delsol, C., Dragani, R., Fuentes, M. … Vitart, F. (2011). The ERA-Interim reanalysis: Configuration and performance of the data assimilation system. Quarterly Journal of the Royal Meteorological Society, 137(656), 553–597. https://doi.org/10.1002/qj.828
- Dong, J., Crow, W. T., Duan, Z., Wei, L., & Lu, Y. (2019). A double instrumental variable method for geophysical product error estimation. Remote Sensing of Environment, 225, 217–228. https://doi.org/10.1016/j.rse.2019.03.003
- Driscoll, S. J., Harrison, D. L., & Kitchen, M. (2000). Improving precipitation estimates from weather radar using quality control and correction techniques. Meteorological Applications, 7(2), 135–144. https://doi.org/10.1017/S1350482700001468
- Duan, Z., Duggan, E., Chen, C., Gao, H., Dong, J., & Liu, J. (2021). Comparison of Traditional Method and Triple Collocation analysis for Evaluation of multiple gridded precipitation products across Germany. Journal of Hydrometeorology, 22(11), 2983–2999. https://doi.org/10.1175/JHM-D-21-0049.1
- Fang, K., Pan, M., & Shen, C. (2019). The value of SMAP for long-term soil moisture estimation with the help of deep learning. IEEE Transactions on Geoscience and Remote Sensing, 57(4), 2221–2233. https://doi.org/10.1109/TGRS.2018.2872131
- Funk, C., Peterson, P., Landsfeld, M., Pedreros, D., Verdin, J., Shukla, S., Husak, G., Rowland, J., Harrison, L., Hoell, A., & Michaelsen, J. (2015). The climate hazards infrared precipitation with stations—A new environmental record for monitoring extremes. Scientific Data, 2(1), 150066. https://doi.org/10.1038/sdata.2015.66
- Gan, F., Chen, S., Gao, Y., & Li, Y. (2022). An evaluation of the performance of IMERG hourly precipitation estimates in a geographical sub-region with complex terrain and climate conditions: A case study in the upper Red River Basin. Remote Sensing Letters, 13(3), 301–310. https://doi.org/10.1080/2150704X.2021.2014076
- Gelaro, R., McCarty, W., Suárez, M. J., Todling, R., Molod, A., Takacs, L., Randles, C. A., Darmenov, A., Bosilovich, M. G., Reichle, R., Wargan, K., Coy, L., Cullather, R., Draper, C., Akella, S., Buchard, V., Conaty, A., Silva, A. M. D. … Zhao, B. (2017). The Modern-era retrospective analysis for research and applications, version 2 (MERRA-2). Journal of Climate, 30(14), 5419–5454. https://doi.org/10.1175/JCLI-D-16-0758.1
- Gomis-Cebolla, J., Rattayova, V., Salazar-Galán, S., & Francés, F. (2023). Evaluation of ERA5 and ERA5-land reanalysis precipitation datasets over Spain (1951–2020). Atmospheric Research, 284, 106606. https://doi.org/10.1016/j.atmosres.2023.106606
- Hoang, T., Manomaiphiboon, K., Singhrattna, N., & Assareh, N. (2020). Evaluation of multiple sub-daily satellite precipitation products for Thailand. Journal of Sustainable Energy & Environment, 11(2020), 81–91. https://www.semanticscholar.org/paper/Evaluation-of-multiple-sub-daily-satellite-products-Trang-Manomaiphiboon/aee14b4e1ce4818ad4e226c159b2720c58702f66
- Hofstra, N., New, M., & McSweeney, C. (2010). The influence of interpolation and station network density on the distributions and trends of climate variables in gridded daily data. Climate Dynamics, 35(5), 841–858. https://doi.org/10.1007/s00382-009-0698-1
- Hong, L., Huang, Y., Peng, S., & Hewitt, J. (2020). Monitoring the trends of water-erosion desertification on the Yunnan-Guizhou Plateau, China from 1989 to 2016 using time-series Landsat images. Public Library of Science ONE, 15(2), e0227498. https://doi.org/10.1371/journal.pone.0227498
- Hou, A. Y., Kakar, R. K., Neeck, S., Azarbarzin, A. A., Kummerow, C. D., Kojima, M., Oki, R., Nakamura, K., & Iguchi, T. (2014). The global precipitation measurement mission. Bulletin of the American Meteorological Society, 95(5), 701–722. https://doi.org/10.1175/BAMS-D-13-00164.1
- Irannezhad, M., & Liu, J. (2022). Evaluation of six gauge-based gridded climate products for analyzing long-term historical precipitation patterns across the Lancang-Mekong River Basin. Geography and Sustainability, 3(1), 85–103. https://doi.org/10.1016/j.geosus.2022.03.002
- Jackson, G., Kirschbaum, D., Petersen, W., Huffman, G., Kidd, C., Stocker, E., & Kakar, R. (2018). The global precipitation measurement (GPM) mission’s scientific achievements and societal contributions: Reviewing four years of advanced rain and snow observations. Quarterly Journal of the Royal Meteorological Society, 144(S1), 27–48. https://doi.org/10.1002/qj.3313
- Jiang, Q., Li, W., Fan, Z., He, X., Sun, W., Chen, S., Wen, J., Gao, J., & Wang, J. (2021). Evaluation of the ERA5 reanalysis precipitation dataset over Chinese mainland. Journal of Hydrology, 595, 125660. https://doi.org/10.1016/j.jhydrol.2020.125660
- Ji, H., Peng, D., Gu, Y., Liang, Y., & Luo, X. (2022). Evaluation of multiple satellite precipitation products and their potential utilities in the Yarlung Zangbo River Basin. Scientific Reports, 12(1), 13334. https://doi.org/10.1038/s41598-022-17551-y
- Joyce, R. J., Janowiak, J. E., Arkin, P. A., & Xie, P. (2004). CMORPH: A method that produces global precipitation estimates from passive microwave and infrared data at high spatial and temporal resolution. Journal of Hydrometeorology, 5(3), 487–503. https://doi.org/10.1175/1525-7541(2004)005<0487:CAMTPG>2.0.CO;2
- Khan, M. K. U., Iqbal, M. F., Mahmood, I., Shahzad, M. I., Zafar, Q., & Khalid, B. (2023). Evaluation of precipitation products over different climatic zones of Pakistan. Theoretical and Applied Climatology, 151(3), 1301–1321. https://doi.org/10.1007/s00704-022-04355-3
- Kim, S., Sharma, A., Wasko, C., & Nathan, R. (2022). Linking total precipitable water to precipitation extremes globally. Earth’s Future, 10(2), e2021EF002473. https://doi.org/10.1029/2021EF002473
- Kirstetter, P. E. (2022). Evaluation of IMERG over CONUS complex terrain using environmental variables. Geophysical Research Letters, 49(19), e2022GL100186. https://doi.org/10.1029/2022GL100186
- Kobayashi, S., Ota, Y., Harada, Y., Ebita, A., Moriya, M., Onoda, H., Onogi, K., Kamahori, H., Kobayashi, C., Endo, H., Miyaoka, K., & Takahashi, K. (2015). The JRA-55 reanalysis: General specifications and basic characteristics. Journal of the Meteorological Society of Japan Ser II, 93(1), 5–48. https://doi.org/10.2151/jmsj.2015-001
- Le, X.-H., Nguyen Van, L., Hai Nguyen, D., Nguyen, G. V., Jung, S., & Lee, G. (2023). Comparison of bias-corrected multisatellite precipitation products by deep learning framework. International Journal of Applied Earth Observation and Geoinformation, 116, 103177. https://doi.org/10.1016/j.jag.2022.103177
- Levizzani, V., & Cattani, E. (2019). Satellite remote sensing of precipitation and the Terrestrial Water Cycle in a changing climate. Remote Sensing, 11(19), 2301. https://doi.org/10.3390/rs11192301
- Li, X., Chen, Y., Wang, H., & Zhang, Y. (2020). Assessment of GPM IMERG and radar quantitative precipitation estimation (QPE) products using dense rain gauge observations in the Guangdong-Hong Kong-Macao Greater Bay area, China. Atmospheric Research, 236, 104834. https://doi.org/10.1016/j.atmosres.2019.104834
- Liu, Z., Jiang, L., Shi, C., Zhang, T., Zhou, Z., Liao, J., Yao, S., Liu, J., Wang, M., Wang, H., Liang, X., Zhang, Z., Yao, Y., Zhu, T., Chen, Z., Xu, W., Cao, L., Jiang, H., & Hu, K. (2023). CRA-40/Atmosphere—The first-generation Chinese Atmospheric reanalysis (1979–2018): System description and performance evaluation. Journal of Meteorological Research, 37(1), 1–19. https://doi.org/10.1007/s13351-023-2086-x
- Li, X., Yang, Y., Mi, J., Bi, X., Zhao, Y., Huang, Z., Liu, C., Zong, L., & Li, W. (2021). Leveraging machine learning for quantitative precipitation estimation from fengyun-4 geostationary observations and ground meteorological measurements. Atmospheric Measurement Techniques, 14(11), 7007–7023. https://doi.org/10.5194/amt-14-7007-2021
- Loo, Y. Y., Billa, L., & Singh, A. (2015). Effect of climate change on seasonal monsoon in Asia and its impact on the variability of monsoon rainfall in Southeast Asia. Geoscience Frontiers, 6(6), 817–823. https://doi.org/10.1016/j.gsf.2014.02.009
- Lu, H., Ding, L., Ma, Z., Li, H., Lu, T., Su, M., & Xu, J. (2020). Spatiotemporal assessments on the satellite-based precipitation products from Fengyun and GPM over the Yunnan-Kweichow Plateau, China. Earth and Space Science, 7(1), e2019EA000857. https://doi.org/10.1029/2019EA000857
- Lu, H., Huang, Z., Ding, L., Lu, T., & Yuan, Y. (2021). Calibrating FY4A QPE using CMPA over Yunnan–Kweichow Plateau in summer 2019. European Journal of Remote Sensing, 54(1), 476–486. https://doi.org/10.1080/22797254.2021.1960202
- Luo, X., Fan, X., Ji, X., & Li, Y. (2020). Evaluation of corrected APHRODITE estimates for hydrological simulation in the Yarlung Tsangpo–Brahmaputra River Basin. International Journal of Climatology, 40(9), 4158–4170. https://doi.org/10.1002/joc.6449
- Mannig, B., Müller, M., Starke, E., Merkenschlager, C., Mao, W., Zhi, X., Podzun, R., Jacob, D., & Paeth, H. (2013). Dynamical downscaling of climate change in central asia. Global and Planetary Change, 110, 26–39. https://doi.org/10.1016/j.gloplacha.2013.05.008
- Ma, Z., Xu, J., Ma, Y., Zhu, S., He, K., Zhang, S., Ma, W., & Xu, X. (2022). AERA5-Asia: A long-term Asian precipitation dataset (0.1°, 1-hourly, 1951–2015, Asia) anchoring the ERA5-land under the total volume control by APHRODITE. Bulletin of the American Meteorological Society, 103(4), E1146–E1171. https://doi.org/10.1175/BAMS-D-20-0328.1
- Ma, Z., Xu, J., Zhu, S., Yang, J., Tang, G., Yang, Y., Shi, Z., & Hong, Y. (2020). AIMERG: A new Asian precipitation dataset (0.1°/half-hourly, 2000–2015) by calibrating the GPM-era IMERG at a daily scale using APHRODITE. Earth System Science Data, 12(3), 1525–1544. https://doi.org/10.5194/essd-12-1525-2020
- Miao, Q., Yuan, S., Shi, L., & Gu, X. (2007). Study on the spring drought rule in the karst region of Yunnan and Guizhou Plateau in China. Proceedings of SPIE - The International Society for Optical Engineering, 6790. https://doi.org/10.1117/12.746860
- Michaelides, S., Levizzani, V., Anagnostou, E., Bauer, P., Kasparis, T., & Lane, J. E. (2009). Precipitation: Measurement, remote sensing, climatology and modeling. Atmospheric Research, 94(4), 512–533. https://doi.org/10.1016/j.atmosres.2009.08.017
- Mo, C., Meng, X., Ruan, Y., Wang, Y., Lei, X., Xing, Z., & Lai, S. (2022). Drought assessment based on fused satellite and station precipitation data: An example from the Chengbi River Basin, China. ISPRS International Journal of Geo-Information, 11(1), 48. https://doi.org/10.3390/ijgi11010048
- Muñoz-Sabater, J., Dutra, E., Agustí-Panareda, A., Albergel, C., Arduini, G., Balsamo, G., Boussetta, S., Choulga, M., Harrigan, S., Hersbach, H., Martens, B., Miralles, D. G., Piles, M., Rodríguez-Fernández, N. J., Zsoter, E., Buontempo, C., & Thépaut, J.-N. (2021). ERA5-land: A state-of-the-art global reanalysis dataset for land applications. Earth System Science Data, 13(9), 4349–4383. https://doi.org/10.5194/essd-13-4349-2021
- Nan, L., Yang, M., Wang, H., Xiang, Z., & Hao, S. (2021). Comprehensive Evaluation of Global Precipitation Measurement Mission (GPM) IMERG Precipitation products over Mainland China. Water, 13(23), 3381. https://doi.org/10.3390/w13233381
- Nawaz, Z., Li, X., Chen, Y., Nawaz, N., Gull, R., & Elnashar, A. (2020). Spatio-temporal assessment of Global precipitation products over the largest agriculture region in Pakistan. Remote Sensing, 12(21), 3650. https://doi.org/10.3390/rs12213650
- Ning, S., Song, F., Udmale, P., Jin, J., Thapa, B., & Ishidaira, H. (2017). Error analysis and evaluation of the latest GSMap and IMERG precipitation products over Eastern China. Advances in Meteorology, 2017, 1–16. https://doi.org/10.1155/2017/1803492
- Özerdem, M. S., Acar, E., & Ekinci, R. (2017). Soil moisture estimation over vegetated agricultural areas: Tigris Basin, Turkey from radarsat-2 data by Polarimetric Decomposition Models and a Generalized Regression Neural Network. Remote Sensing, 9(4), 395. https://doi.org/10.3390/rs9040395
- Parker, W. S. (2016). Reanalyses and observations: What’s the difference? Bulletin of the American Meteorological Society, 97(9), 1565–1572. https://doi.org/10.1175/BAMS-D-14-00226.1
- Peng, F., Zhao, S., Chen, C., Cong, D., Wang, Y., & Ouyang, H. (2020). Evaluation and comparison of the precipitation detection ability of multiple satellite products in a typical agriculture area of China. Atmospheric Research, 236, 104814. https://doi.org/10.1016/j.atmosres.2019.104814
- Prakash, S., Mitra, A. K., Momin, I. M., Rajagopal, E. N., Basu, S., Collins, M., Turner, A. G., Achuta Rao, K., & Ashok, K. (2015). Seasonal intercomparison of observational rainfall datasets over India during the southwest monsoon season. International Journal of Climatology, 35(9), 2326–2338. https://doi.org/10.1002/joc.4129
- Prein, A. F., & Gobiet, A. (2017). Impacts of uncertainties in European gridded precipitation observations on regional climate analysis. International Journal of Climatology, 37(1), 305–327. https://doi.org/10.1002/joc.4706
- Qiu, S., Peng, J., Zheng, H., Xu, Z., & Meersmans, J. (2022). How can massive ecological restoration programs interplay with social-ecological systems? A review of research in the South China karst region. Science of the Total Environment, 807, 150723. https://doi.org/10.1016/j.scitotenv.2021.150723
- Reda, K., Liu, X., Haile, G., Sun, S., & Tang, Q. (2021). Hydrological evaluation of satellite and Reanalysis-based Rainfall Estimates Over the Upper Tekeze Basin, Ethiopia. https://doi.org/10.22541/au.161494395.50246119/v1
- Ren, P., Li, J., Feng, P., Guo, Y., & Ma, Q. (2018). Evaluation of multiple satellite precipitation products and their use in hydrological modelling over the Luanhe River Basin, China. Water, 10(6), 677. https://doi.org/10.3390/w10060677
- Rong, G., & Zhang, J.(2023). Risk assessment of extreme precipitation-geological hazard chains in the Yunnan-Guizhou Plateau. Advances in Economics, Business and Management Research, 18–25. https://doi.org/10.2991/978-94-6463-194-4_4
- Schneider, U., Becker, A., Finger, P., Meyer-Christoffer, A., Ziese, M., & Rudolf, B. (2014). GPCC’s new land surface precipitation climatology based on quality-controlled in situ data and its role in quantifying the global water cycle. Theoretical and Applied Climatology, 115(1–2), 15–40. https://doi.org/10.1007/s00704-013-0860-x
- Shen, Y., Zhao, P., Pan, Y., & Yu, J. (2014). A high spatiotemporal gauge‐satellite merged precipitation analysis over China. Journal of Geophysical Research: Atmospheres, 119(6), 3063–3075. https://doi.org/10.1002/2013JD020686
- Song, X., Zhang, G., Liu, F., Li, D., Zhao, Y., & Yang, J. (2016). Modeling spatio-temporal distribution of soil moisture by deep learning-based cellular automata model. Journal of Arid Land, 8(5), 734–748. https://doi.org/10.1007/s40333-016-0049-0
- Soo, E. Z. X., Wan Jaafar, W. Z., Lai, S. H., Othman, F., Elshafie, A., Islam, T., Srivastava, P., & Othman Hadi, H. S. (2020). Precision of raw and bias-adjusted satellite precipitation estimations (TRMM, IMERG, CMORPH, and PERSIANN) over extreme flood events: Case study in Langat river basin, Malaysia. Journal of Water and Climate Change, 11(S1), 322–342. https://doi.org/10.2166/wcc.2020.180
- Sun, H., Yang, Y., Wu, R., Gui, D., Xue, J., Liu, Y., & Yan, D. (2019). Improving estimation of cropland evapotranspiration by the bayesian Model averaging method with surface energy balance models. Atmosphere, 10(4), 188. https://doi.org/10.3390/atmos10040188
- Tang, X., Li, H., Qin, G., Huang, Y., & Qi, Y. (2023). Evaluation of satellite-based precipitation products over complex topography in Mountainous Southwestern China. Remote Sensing, 15(2), 473. https://doi.org/10.3390/rs15020473
- Tanim, A. H., Mullick, M. R. A., & Sikdar, M. S. (2021). Evaluation of spatial rainfall products in sparsely gauged region using copula uncertainty modeling with triple collocation. Journal of Hydrologic Engineering, 26(4), 04021004. https://doi.org/10.1061/(ASCE)HE.1943-5584.0002071
- Tian, Y., Peters-Lidard, C. D., Eylander, J. B., Joyce, R. J., Huffman, G. J., Adler, R. F., Hsu, K.-L., Turk, F. J., Garcia, M., & Zeng, J. (2009). Component analysis of errors in satellite-based precipitation estimates. Journal of Geophysical Research: Atmospheres, 114(D24). https://doi.org/10.1029/2009JD011949
- Tong, K., Su, F., Yang, D., & Hao, Z. (2014). Evaluation of satellite precipitation retrievals and their potential utilities in hydrologic modeling over the Tibetan Plateau. Journal of Hydrology, 519, 423–437. https://doi.org/10.1016/j.jhydrol.2014.07.044
- Torsri, K., Lin, Z., Dike, V., Thodsan, T., & Wongsaming, P. (2022). Evaluation of Spatial-Temporal Characteristics of Rainfall Variations over Thailand inferred from different gridded datasets. Water, 14(9), 1359. https://doi.org/10.3390/w14091359
- Wang, R., Chen, J., & Wang, X. (2017). Comparison of IMERG level-3 and TMPA 3B42V7 in estimating typhoon-related heavy rain. Water, 9(4), 276. https://doi.org/10.3390/w9040276
- Wedajo, G. K., Muleta, M. K., & Awoke, B. G. (2021). Performance evaluation of multiple satellite rainfall products for Dhidhessa River Basin (DRB), Ethiopia. Atmospheric Measurement Techniques, 14(3), 2299–2316. https://doi.org/10.5194/amt-14-2299-2021
- Wu, X., & Zhao, N. (2023). Evaluation and comparison of six high-resolution daily precipitation products in Mainland China. Remote Sensing, 15(1), 223. https://doi.org/10.3390/rs15010223
- Xiao, X., Liu, X., Gao, Y., Zhang, S., & Xu, W. (2023). Evaluation of Regional Drought in Yunnan–Guizhou Plateau of China. Proceedings of the nternational Conference on Computational & Experimental Engineering and Sciences (pp. 345–359). https://doi.org/10.1007/978-3-031-42515-8_23.
- Xie, W., Yi, S., Leng, C., Xia, D., Li, M., Zhong, Z., & Ye, J. (2022). The evaluation of IMERG and ERA5-land daily precipitation over China with considering the influence of gauge data bias. Scientific Reports, 12(1), 8085. https://doi.org/10.1038/s41598-022-12307-0
- Xin, Y., Lu, N., Jiang, H., Liu, Y., & Yao, L. (2021). Performance of ERA5 reanalysis precipitation products in the Guangdong-Hong Kong-Macao greater Bay area, China. Journal of Hydrology, 602, 126791. https://doi.org/10.1016/j.jhydrol.2021.126791
- Xin, Y., Yang, Y., Chen, X., Yue, X., Liu, Y., & Yin, C. (2022). Evaluation of IMERG and ERA5 precipitation products over the Mongolian Plateau. Scientific Reports, 12(1), 21776. https://doi.org/10.1038/s41598-022-26047-8
- Xu, J., Ma, Z., Yan, S., & Peng, J. (2022). Do ERA5 and ERA5-land precipitation estimates outperform satellite-based precipitation products? A comprehensive comparison between state-of-the-art model-based and satellite-based precipitation products over mainland China. Journal of Hydrology, 605, 127353. https://doi.org/10.1016/j.jhydrol.2021.127353
- Yao, J., Chen, Y., Yu, X., Zhao, Y., Guan, X., & Yang, L. (2020). Evaluation of multiple gridded precipitation datasets for the arid region of northwestern China. Atmospheric Research, 236, 104818. https://doi.org/10.1016/j.atmosres.2019.104818
- Yu, C., Hu, D., Liu, M., Wang, S., & Di, Y. (2020). Spatio-temporal accuracy evaluation of three high-resolution satellite precipitation products in China area. Atmospheric Research, 241, 104952. https://doi.org/10.1016/j.atmosres.2020.104952
- Zhang, Y., Ye, A., Nguyen, P., Analui, B., Sorooshian, S., & Hsu, K. (2021). New insights into error decomposition for precipitation products. Geophysical Research Letters, 48(17), e2021GL094092. https://doi.org/10.1029/2021GL094092
- Zhang, Y., Ye, A., Nguyen, P., Analui, B., Sorooshian, S., & Hsu, K. (2022). QRF4P-NRT: Probabilistic Post-Processing of Near-Real-Time Satellite Precipitation Estimates Using Quantile Regression Forests. Water Resources Research, 58(5), e2022WR032117. https://doi.org/10.1029/2022WR032117
- Zhao, Y., Xu, K., Dong, N., & Wang, H. (2022). Optimally integrating multi-source products for improving long series precipitation precision by using machine learning methods. Journal of Hydrology, 609, 127707. https://doi.org/10.1016/j.jhydrol.2022.127707
- Zhou, Z., Guo, B., Xing, W., Zhou, J., Xu, F., & Xu, Y. (2020). Comprehensive evaluation of latest GPM era IMERG and GSMaP precipitation products over mainland China. Atmospheric Research, 246, 105132. https://doi.org/10.1016/j.atmosres.2020.105132
- Zhu, S., & Ma, Z. (2022). PECA-FY4A: Precipitation estimation using Chromatographic analysis methodology for full-disc multispectral observations from FengYun-4A/AGRI. Remote Sensing of Environment, 282, 113234. https://doi.org/10.1016/j.rse.2022.113234
- Zhu, D., Yang, Q., Xiong, K., & Xiao, H. (2022). Spatiotemporal variations in daytime and night-time precipitation on the Yunnan–Guizhou Plateau from 1960 to 2017. Atmosphere, 13(3), 415. https://doi.org/10.3390/atmos13030415