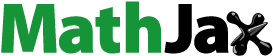
Abstract
In In this study, we set out to explore how various spatial patterns of travel demand drive the effectiveness of ride-pooling services. To do so, we generate a broad range of synthetic, yet plausible demand patterns. We experiment with the number of attraction centres, the dispersion of destinations around these centres, and the trip length distribution. We apply a strategic ride-pooling algorithm across the generated demand patterns to identify shareability potential using a series of metrics related to ridepooling. Our findings indicate that, under a fixed demand level, vehicle-hour reduction due to ride-pooling can range between 18 and 59%. These results depend on the concentration of travel destinations around the centre and the trip length distribution. Ride-pooling becomes more efficient when trips are longer and destinations are more concentrated. A shift from a monocentric to a polycentric demand pattern is found to have a limited impact on the prospects of ride-pooling.
1. Introduction
Ride-hailing services have become part of the urban mobility landscape across the world. It has been observed that there is an increased demand for these services for certain groups and in certain areas with low car ownership rates (Ghaffar, Mitra, and Hyland Citation2020), which can be interpreted as either a potential to substitute car rides or as an overall increment in car travel. There is a fervent debate on the impact of ride-hailing services, especially in relation to their competition with public transport services (Boisjoly et al. Citation2018; Etminani-Ghasrodashti and Hamidi Citation2019), or possible competing and complementing roles (Cats et al. Citation2022), and contribution to traffic congestion (Erhardt et al. Citation2019). In addition, ride-hailing also frequently happens between highly accessible areas (Marquet Citation2020) and depends on the density of different services (e.g. restaurants), which highlights the relevance of the urban environment composition in ride-hailing demand (Ghaffar, Mitra, and Hyland Citation2020).
Ride-pooling services are likely to be more consistent with policy goals and positively contribute towards accessibility and affordability as well as reducing congestion and related externalities. Passengers are incentivised to opt for a shared ride rather than a private ride by using a discount offered to compensate for the potentially induced discomfort and delay. From the perspective of service providers and drivers, ride-pooling may reduce the mileage which in turn increases the occupancy and reduces the operating costs. An analysis of the case of New York City estimated that the total demand served by 13,000 taxis could be served by 3,000 vehicles when allowing for en-route ride-sharing (Alonso-Mora et al. Citation2017). Moreover, attractive ride-pooling may increase the overall market share of ride-hailing and thus generate additional income. Notably, even though ride-hailing operations facilitate the emergence of ride-pooling services, their market shares remain low so far (W. Li et al. Citation2019; Young, Farber, and Palm Citation2020). Moreover, it is estimated that only about half of the trips that took place using the pooled service were actually shared in the case of Toronto (Young, Farber, and Palm Citation2020), before this service was discontinued due to the COVID-19 pandemic.
It is thus pertinent to identify under what circumstances ride-pooling is most likely to attain a significant market share and result in efficiency gains. In this study, we set out to explore how various spatial patterns of travel demand drive the effectiveness of ride-pooling services. We conduct an extensive series of experiments thereby contributing to the identification of the most promising spatial demand patterns for ride-pooling services. Namely, the ones in which shareability is most effective for travellers (short delays) and the service provider (short detours) and has the potential to attain a viable market size (when ride-hailing travellers decide to use pooled services).
For companies offering ride-pooling services, it is crucial to ensure that their trips can be pooled together into shared rides. This so-called trip shareability occurs among mutually compatible trip requests in terms of their spatial and temporal constraints so that they do not impose prohibitive detours or delays for any of the co-riders. Using taxi data from several cities, Tachet et al. (Citation2017) demonstrated that the likelihood that a trip can be matched correlates positively with demand levels, i.e. the higher the demand the more likely it is that trips can be matched. This relationship is also corroborated by Ke et al. (Citation2021), who found that matching probabilities increase with passenger demand following a negative-exponential distribution, implying an increasing return rate for additional demand. This has given rise to the notion of a critical mass that needs to be obtained for ride-pooling to become successful. However, users were assumed to be captive to the service and assigned to trips. Hence, the notion of shareability was studied without accounting for travellers’ ability to choose whether to share their trip, arguably key for assessing whether trips are in fact likely to be shared.
However, little is known about how the spatial structure of travel demand impacts ride-pooling. In fact, despite spatial variations being an inherent and key feature of travel demand, none of the abovementioned studies investigated the impacts of such. Daganzo, Ouyang, and Yang (Citation2020) underlined the importance of analysing the implications of alternative spatial demand patterns and analysing if and how ride-pooling results change when demand is not evenly distributed. Since the actual travel demand for ride-pooling typically accounts for a small share of urban mobility, it is likely to exhibit unique characteristics that are not representative of the overall demand pattern. Thus it is relevant to identify the relation between the spatial patterns of travel demand and their potential to be efficiently pooled. For instance, both Soria and Stathopoulos (Citation2021) and Zwick and Axhausen (Citation2022) identified that not only the population and workplace density are relevant in terms of ride-pooling demand but that the density of other services such as restaurants and cultural locations also play a role. In addition, several past studies have pointed to the importance of travel length (Dean and Kockelman Citation2021) in ride-pooling demand and travel demand directionality in explaining fleet utilisation (Narayan et al. Citation2021). Notwithstanding, even though demand distribution patterns are expected to greatly affect the potential for ride-pooling, their impact remains unknown.
In parallel, a stream of empirical studies investigated the relations between urban and road network structure, land-use distribution, travel demand and transport performance. Ewing and Cervero (Citation2010) performed a meta-analysis of studies analysing behaviour at the individual level from across the United States. Several studies conducted a regression analysis at the urban agglomeration level based on car travel (Ewing, Tian, and Lyons Citation2018) or mobile phone location data from the US (Bassolas et al. Citation2019) and location-based navigation service data for Chinese cities (Y. Li, Xiong, and Wang Citation2019), and public transport ridership data from across Europe (Ingvardson and Nielsen Citation2018). Hierarchical agglomerative clustering was applied to identify the relation between urban characteristics and mobility patterns for different clusters of global cities (Oke et al. Citation2019). The results of these studies confirm for instance that compact (as opposed to dispersed) city centres strongly affect the related travel demand patterns and, in turn, impact travel mileage and traffic congestion. Whether such a relation also holds true for ride-pooling remains unknown.
Analytical and simulation studies examined the relation between the underlying demand pattern and the performance of various transport modes. Analytical models estimated the macroscopic flow diagram properties for various concentric city scenarios (Tsekeris and Geroliminis Citation2013) or identified the public transport network structure for various urban structure characteristics such as trip dispersion, urban centre’s and sub-centre’s importance (Fielbaum, Jara-Diaz, and Gschwender Citation2016) as well as for the levels of compactness for a ring-radial city (Badia Citation2020). Network evolution models have demonstrated that structurally distinguished demand patterns facilitate the emergence of distinctive public transport networks for a ring-radial agglomeration (Cats et al. Citation2020). These studies offer a variety of modelling approaches and insights into the relation between travel demand patterns and service performance.
Notwithstanding, there is a lack of knowledge as to the ramifications of those for the prospects of ride-pooling. In a related study, Wang and Zhang (Citation2021) applied an agent-based shared model for various US cities and regressed how the pooling result correlates with urban characteristics. They found that job density and land-use diversity contribute to the percentage of pooled trips.
The research question addressed in this study is: how ‘shareable’ are various spatial demand patterns? To this end, we devise a set of experiments tailored to identify the most promising demand patterns for introducing ride-pooling services by applying the ExMAS algorithm – an offline, utility-based ride-pooling algorithm (Kucharski and Cats Citation2020) that bundles trip requests into attractive rides shared by up to eight travellers. To disentangle the critical mass effect needed to induce pooling from the spatial pattern significance, we keep the demand levels fixed through the experiments. To make simulations realistic, we keep the actual spatial pattern of origins in Amsterdam. We generate a broad range of synthetic demand patterns by varying the number of centres, the dispersion of destinations around each of these centres and the trip length distribution. We then apply ExMAS, which output includes detailed results of detours, delays and utility of sharing for travellers and drivers. Using a broad set of ride-pooling KPIs, we examine the consequences of alternative demand patterns on travellers’ choice for ride-pooling, its level of service and system performance.
The rest of this paper is organised as follows. In the next section, we detail the process of generating alternative demand patterns, the algorithms used for matching travel requests into rides and the indicators proposed for quantifying the shareability potential of a given demand pattern. Next, we describe the experimental set-up based on the Amsterdam case study and compare the results obtained for different scenarios as well as their spatial variations and distributional effects. We conclude with a discussion of the key findings and their implications as well as suggestions for further research.
2. Methods
In this section, we describe the sequence of steps that we undertake for generating spatial demand patterns and assess their shareability. First, we describe how we generate demand patterns. Second, we outline how we calculate the shareability potential of each of those scenarios, followed by the set of key performance indicators used for assessing the shareability potential ingrained in each of the demand patterns. An overview of applied methodology is illustrated in Figure .
2.1. Synthetic demand generation
We generate a series of synthetic scenarios that are designed to reflect a variety of demand patterns that might prevail in urban areas. We start with a fixed demand level (number of trip requests per hour) and the actual distribution of trip origins, for which we alter the spatial pattern of destinations. We propose characterising the urban demand distribution and generating variations thereof by determining the following design variables:
number of attraction centres,
density of destinations around each centre,
trip length distribution.
As illustrated in Figure , first we draw a given number of trip origins from the actual travel demand set. In the second step, we control for the number of centres, while the third and fourth steps are controlled by a set of parameters that jointly describe the spatial distribution. The procedure can be synthesised as follows:
We determine the number of centres around which we generate destinations with a given density (detailed in section 2.1.1 below).
We then place destinations around the centres with a given spatial distribution (detailed in section 2.1.2).
We assign destinations to the origins according to a predefined trip distribution length (detailed in section 2.1.3).
As a result, generated patterns cover urban areas consisting of one or more centres ranging from highly concentrated to uniformly dispersed destinations and ranging from low impedance (travellers likely to travel long distances) to high impedance (where travellers find destinations nearby). Note that in our synthetic demand generation process, travel origins are sampled from the actual demand pattern.
2.1.1. Centres of attraction
We place centres of attraction in a predefined set of locations. The centres remain in their fixed locations across the experiments and have a predefined hierarchy (i.e. we always start with the most important centre and gradually add secondary, tertiary and quaternary centres). By having the set of potential centres of attraction fixed, we obtain unbiased statistics, such as the average distance, and reduce the number of dimensions for analysis thereby allowing for a more direct interpretation of the experiment results. Here, we will experiment with up to four centres, virtually placed in potential hot-spots of Amsterdam.
2.1.2. Spatial distribution of destinations
Subsequently, potential destinations are generated around each centre. Their distance to the centre d follows a Gamma distribution with shape parameter kc and scale parameter sc, hence its probability density function is as follows:
(1)
(1) Where Γ(kc) corresponds to the Gamma function evaluated at the shape parameter kc:
(2)
(2) The scale parameter is fixed to represent the city under consideration and the shape parameter reflects the density. Gamma probability density function is selected as it properly describes the distance from a bivariate normal distributed coordinate to its centre. In addition, Gamma functions serve as good friction parameters in trip distribution models (e.g. Schiffer Citation2012) and have also been widely used in terms of travel time modelling (Guenthner and Hamat Citation1988; Kim and Mahmassani Citation2015). When the shape parameter is equal to one, it corresponds to an exponential distribution where the mean equals the scale of the distribution, and when it is larger than one, the distribution is skewed and varies with shape. Consequently, smaller shape parameter values represent the more concentrated destinations around each of the centres. Four specific distributions are illustrated in Figure a.
2.1.3. Matching origins to destinations
Finally, we match destinations with origins. In this study, we use the existing and fixed spatial coordinates of trip origins. To create trip requests, origins are matched with synthetically generated destinations following the specified trip length distribution. Similarly to the second stage, this trip length distribution parameter corresponds to the shape parameter of the Gamma distribution, for which its scale parameter has been fixed. As before, we define the distance of these generated trips, d, to follow a Gamma distribution with shape parameter kt and scale parameter st. Lower values of parameter kt imply trip destinations closer to origins (shorter trips), while greater values represent longer trips. The probability density function is thus defined as follows:
(3)
(3) Four different distributions used in experiments are illustrated in Figure b.
Matching trip origins with destinations is performed as follows. For each origin, we calculate the distance d to each of the potential destinations from the previous step. We then calculate the probability to select that destination based on the probability density function described in (3). We then sample the destination for that particular origin by employing those probabilities as weights and thereby obtain an unconstrained model where trip lengths follow a predefined distribution (unlike a doubly constrained gravity model where an iterative balancing procedure needs to be applied (O’Kelly and Niedzielski Citation2008))
Consequently, after following the aforementioned procedure, we obtain a synthetic set of requests where each origin is matched with a destination in such a way that the resulting demand patterns follow the desired spatial distributions.
2.2. Quantifying shareability potential
We are interested in quantifying the shareability potential of each of the generated synthetic travel demand patterns. To this end, we apply the ExMAS algorithm (Kucharski and Cats Citation2020) which pools single trips into attractive shared rides. This algorithm is suited for strategic planning purposes and focuses on the demand side, rather than operational service considerations. It uses as input the demand pattern, i.e. set of trip requests with their origin, destination and departure time – such as the one introduced in the previous section. ExMAS pools rides for given sets of behavioural parameters (value-of-time and willingness to share) and design variables (e.g. trip fare and discount offered for pooled rides). Based on those, ExMAS identifies attractive pooled rides for which the detour and delay are at least compensated by the reduced fare for each pooled traveller.
ExMAS evaluates the attractiveness of each candidate pooled ride using a utility formula. The pooled ride is considered attractive if the utility of pooling is greater than the utility of a private ride for all travellers involved. The utility associated with a private trip is composed of travel time and trip fare, while pooling utility is extended with the detour, delay and the so-called willingness-to-share – which are negative costs of pooling that shall at least be compensated by the discount offered for pooling. ExMAS considers all the feasible and attractive pooled rides composed of up to eight travellers and performs the optimal matching in the bi-partitioned graph consisting of individual trips on the one hand and (private or shared) rides on the other hand. By narrowing the solution space to attractive rides only, ExMAS can exhaustively assess all attractive shared rides. In the matching phase, ExMAS optimally assigns each traveller/trip to an attractive ride to minimise the global objective function value. In this study, the assignment made as part of the matching process aims at minimising the total vehicle hours (note that each pooled ride in the search space is already guaranteed to be attractive for all travellers therein). Importantly, the ExMAS solution is not necessarily composed solely of pooled rides since the traveller may still be assigned to a private (non-pooled) ride. This may happen when a) her/his trip request is not compatible with any other request (i.e. the private alternative ride is more attractive than any of the shared ride options) or b) when it is beneficial for the global matching solution. For more details and utility formulas, we refer the reader to the ExMAS paper (Kucharski and Cats Citation2020) and the open-source python package with reproducible examplesFootnote1, including its incorporation in a simulation model of two-sided mobility-on-demand platforms (Kucharski and Cats Citation2022).
The offline and deterministic algorithm of ExMAS allows computing shareability metrics for relatively large-scale demand patterns using compensatory behavioural decision-making rules. Contrary to most of the algorithms reported in the literature, ExMAS does not focus on real-time operations and fleet management. Instead, it is utility-based and takes a user-centric approach. Thus, it allows the identification of the actual ride-pooling potential inherent to the demand pattern itself.
The output of ExMAS (trip requests optimally matched into attractive pooled rides) allows for a comprehensive assessment of ride-pooling efficiency. In particular, we measure the shareability potential using the following metrics:
Total vehicle hours, T – total time spent with travellers on-board to satisfy the demand (deadheading and other empty vehicle trips are not considered). This allows for the assessment of the efficiency of ride-pooling from the supplier’s (platform operator or service provider) perspective. In principle, the total vehicle hours shall be reduced due to pooling.
Total passenger cost, C – cost of all travellers, which combines the disutility of time (in-vehicle and waiting) with the monetary terms (fare, possibly reduced due to pooling). In principle, the pooling shall reduce the passenger costs due to the explicit consideration of utility constraints in ExMAS.
Occupancy, O – ratio of vehicle hours (T) to passenger hours (Tpass), supports investigating how compactly the travel demand is pooled. We use it as a key indicator for studying the efficiency attained by pooling as it integrates information from both the supply- and demand-side effects.
For vehicle hours and passenger costs, we compare the values obtained when allowing for pooling versus the case where a ride-pooling service is not available. We report the relative differences that result from pooling ΔC, ΔT as follows:
(4)
(4) The above set of metrics allows for the sound assessment of the shareability potential associated with each of the demand patterns.
3. Application
In this section, we first describe the experimental set-up devised to address our research question. We then present the results, first in terms of the resulting synthetic demand patterns and then followed by the aggregate and distributional shareability indicators.
3.1. Experimental set-up
The experiments are configured around the case of Amsterdam, the Netherlands, where 1,000 trip requests are generated during a one-hour period. This aims to replicate ride-hailing operations in the city, as it starts with origins sampled from the actual Amsterdam demand pattern extracted from an updated version of the nationwide synthetic demand model (Arentze and Timmermans Citation2004) and keeps them fixed across the experiments. As explained in the previous section, the first step consists of defining the number of centres around which the synthetic demand is pivoted. We consider up to four possible centres, namely Dam Square, Station Zuid, Concertgebouw (Museumplein) and Sloterdijk (their locations can be seen in Figure ). These four centres constitute major travel attraction areas during the morning peak hour and we therefore choose to use them in our experiments as the anchors for demand attraction. In addition, these four centres are employed in the abovementioned order, meaning that when we simulate a monocentric city, we always consider Dam Square as the sole centre of attraction, whereas when considering two centres, we consider Dam Square and Station Zuid, and so forth.
In terms of the other two attributes, namely kt and kc, we define them around the existing origin-destination pattern in Amsterdam. To obtain sensible baseline values for these parameters, we considered Amsterdam as a monocentric city, meaning destinations are concentrated around Dam Square as the sole centre of gravity. By fitting two Gamma distributions to the trip length distribution and the destination density around the centre, we obtain a kt parameter value of approximately 1.5 and a kc parameter value of around 2.5, respectively. Since, in reality, destinations in Amsterdam and elsewhere radiate around a multiplicity of centres, we experiment with increasing the travel time distribution parameter kt and decreasing the density parameter around centres kc. For the sake of thoroughness, we also consider additional scenarios with a lower kt and a higher kc than those observed for Amsterdam in reality (yielding spatial distributions as illustrated in Figure ).
Consequently, the experiments are run over the following grid of parameter values, i.e. all possible combinations of values from the following sets:
n = 1, 2, 3, 4,
kt = 1, 1.5, 2, 3,
kc = 1, 1.5, 2, 2.5, 3,
Our experimental design results in a total of 80 (4 × 4×5) scenarios to be simulated. Since each scenario is associated with a random demand generation (whereas the ExMAS ride-pooling algorithm is deterministic), we replicate scenarios to obtain statistically significant results. Based on the distribution of the output metrics, 10 replications per scenario were found sufficient to obtain statistically sound results. Each replication took about 7 min to run on a PC: 2 min to generate the demand patterns and 5 min to perform the matching and to quantify the shareability potential. By parallelising these calculations, it was possible to run the entire set of scenarios within a reasonable amount of time.
3.2. Results
In this section, we first report the demand patterns generated for the study and then explore their shareability prospects. Figure presents an illustrative example in terms of how the different parameter variables affect each scenario. While the lengths of the black dashed lines are controlled by kc, the probability of matching an origin to a destination (red lines in the figure) is controlled by the trip length distribution parameter, kt.
3.2.1. Demand patterns description
Each simulation is specified based on the number of centres and a combination of the two shape parameters: of the distance between the potential destinations and each centre, kc, and of the trip length distribution, kt. In the following, we first report the characteristics of the demand patterns generated to better understand the underlying causes of the shareability findings and the spatial variations thereof that are reported in subsequent sections.
We first analyse the distance of each potential destination generated in a monocentric city to its centre (Figure , left), followed by the trip length distribution (Figure , right). As expected, the boxplots – the lines spanning from the 10th to the 90th percentile and from the 25th to the 75th percentile define the box limits, the median is represented by the middle line and the dot represents the average value – illustrate that destinations are more dispersed when increasing kt; the mean distance from the centre and its variability are increasing (Figure , left). In terms of the distribution of potential destinations, we see that the average distance to the centroid increases with kc, especially when kt = 3. As for the average trip distance, we see that it significantly increases when increasing kt, as expected, and at a lower rate when increasing kc (Figure , right).
Figure 5. Distance between generated destinations and a single centre (Dam Square) (left), and trip length distribution (right).

In addition, we display heatmaps of the geographical distribution of the generated demands. In Figure , the four different kc values are presented for a fixed kt. One can observe how increasing this parameter increases the spread of the generated destinations across the city. We carry out the same analysis for the four different kt values and a fixed kc, as presented in Figure . We observe a similar trend to the previous heatmaps, albeit less pronounced. We also see a larger number of trips heading to the peripheral areas of the city due to the increase in trip length distribution parameter. Finally, we conduct a similar analysis for a specific combination of kt and kc (both equal to 1.5) but for different numbers of centres of attraction, presented in Figure . As expected, we observe how increasing the number of centres increases the spread of the demand destinations generated, yet it is still visibly concentrated around the respective centres (marked with purple triangles).
Figure 6. Synthetic demand patterns. Spatial distribution of destinations of various concentrations around centres (kc). One centre (Dam Square) and kt = 1.5.

3.2.2. Aggregate shareability results
The demand pattern generation scenarios not only yield substantially different trip characteristics (as reported in the previous subsection), but they also lead to significantly different shareability prospects as we report in Table . Recall that all of the results presented are averaged over 10 scenario replications, each of which involves sampling from the respective demand distribution settings. The occupancy ranges between 1.25 in the least shareable scenario and 1.75 in the most shareable one. Vehicle hours can be reduced by 18% in the least shareable scenario and up to almost 60% in the most shareable scenarios. The impact of introducing ride-pooling on passenger costs ranges between a reduction of 2.1% and 2.9%.
Table 1. Shareability indicators for various demand patterns.
Notably, not all the parameters of the demand pattern distribution have the same impact. While destinations’ density kc and the number of centres have a relatively limited impact, we find that all the indicators are most sensitive to kt. For a monocentric demand with kc = 1, the shareability increases with kt as reflected across all performance metrics. The reduction in vehicle hours more than doubles when kt increases from 1 to 3 and trip lengths thereby increase. Keep in mind that vehicle hours in private (non-pooled) scenarios also double when kt increases from 1 to 3. We find that kc and the number of centres are negatively associated with travel time and thus with shareability. Changing kc from 1 to 3 changes the total travel time from 118.3 h to 111.1 h, which is accompanied by the travel time reduction due to pooling dropping from 23% to 19%. Similarly, passenger cost reduction diminishes from 2.27% to 2.09%. Shareability metrics are overall stable in relation to changes in the number of centres where the total travel time reductions do not fall below 20% and passenger cost reductions remain greater than 2.15% even for scenarios with four centres.
We further investigate the underlying relations between demand generation parameters and the shareability indicators by plotting related trends. In Figure , we show how the three shareability metrics – O, T and C – change under various demand configurations for a monocentric city. While for lower values of impedance (kt < 3) increasing dispersion from the centres has a negative impact on all metrics, for kt = 3 the trend is inverted for occupancy and vehicle hours reduction. For instance, changing kc from 1 to 3 barely changes the occupancy and vehicle hours reduction, whereas changing kt from 1 to 3 changes it substantially; occupancy rises from 1.25–1.8 and the reduction in vehicle hours increases from 15% to almost 40%. The trends are consistent with the exception of passenger costs (right panel in Figure ), where for high values of kt (2 and 3), the positive impact of pooling on passenger costs first increases and then decreases. The spacings between the lines (values of kt) are similar for occupancy and vehicle hours reduction, with kt = 3 being a clear outlier. This different trend occurs because of the combination of the high preference for longer trips, increasing the dispersion of destinations around the centre and the resulting higher discount (in absolute, monetary terms) which makes shared rides more attractive. Furthermore, for passenger costs, kt = 1 is the outlier with the least positive impact (differences between kt = 2 and kt = 3 are smaller than those between kt = 1 and kt = 1.5).
Figure 9. Occupancy (left), reduction in vehicle hours (middle) and reduction in passenger costs (right) in a monocentric city with varying distance from centres and trip length distribution.

Results for more polycentric cities may significantly vary from those reported above for monocentric ones. We report here the changes in the shareability metrics for different numbers of centres, first for shorter (kt = 1) and longer trips (kt = 3) in Figure . When the trips are short, results are less sensitive to kc the more centres that are present. While for a monocentric city vehicle hour reduction varies with kc from 15% to 19.5%, for three or more centres the difference narrows by almost twice, from 15.5% to 17.5%. Nonetheless, in the case of short trips, a monocentric city typically provides the best results if kc < 2 and introducing new centres limits the benefits of pooling. In contrast, trends are not that clear for kc ≥ 2. For instance, with kc = 2.5, introducing a second centre increases passenger benefits, while the introduction of a third or a fourth centre reduces the passenger benefits attained. Conversely, the reduction in vehicle hours declines after introducing the second centre when kc = 2.5 and then improves when a third or a fourth centre is introduced.
Figure 10. Occupancy (left), reduction in vehicle hours (middle) and reduction in passenger costs (right) for urban areas with a different number of centres and destination density under kt = 1 (above) and kt = 3 (below).

When travellers perform longer trips, the benefits stemming from pooling are in general greater (see also Table ). However, the changes with respect to the number of centres differ in various ways. Cities with four centres yield the greatest reductions in vehicle hours and the highest occupancy, while duo-centric cities yield the lowest benefits. Introducing a second centre worsens both occupancy and vehicle hour reductions for all cases except for when kc = 1. For cities with four centres and kc ≥ 2, passenger benefits are lowest and drop significantly when the number of centres changes from 2 to 4, while introducing a second centre increases passenger benefits. Unlike for kt = 1, where trends across the three metrics are consistent, contradicting trends can be observed under kt = 3. For instance, when kc = 2, both occupancy and vehicle hour reductions are greatest for cities with two centres, while it is the opposite for passenger costs, and the worst results are yielded when there are two prime centres.
3.2.3. Distributional effects of shareability
In the previous subsection, we analysed the overall relations between demand pattern parameters and our shareability metrics. Next, we investigate how the shareability potential ingrained in each of the demand scenarios varies among users and manifests itself spatially as well as the extent to which spatial disparities are exhibited. The analysis thereby sheds light on the distributional effects associated with the introduction of ride-pooling. In the following, we present and discuss the histogram of each of the performance indicators as well as display average values calculated over trip origins for each grid cell by overlaying a grid of 1.33 square km over the case study area. We start by examining the impact that kt has in a monocentric city, i.e. how much it affects longer travel distances, and then follow with an analysis of the impact of having a certain number of centres of attraction.
The distributions of the shareability metrics are presented in Figure . Instead of studying the occupancy as in the previous sections, we analyse the share of trips that have been successfully matched with at least one co-traveller. This is done as it is not possible to account for the passenger and vehicle times for each trip request (i.e. the nominator and denominator of occupancy, O, respectively) in a consistent way. The histograms present the distributions of each metric across the grid cells, varying from 0 if none of the trips originating from the cell were shared, up to 100% where each traveller finds a match. As discussed in the previous subsection, we see a clear increase in the extent to which people share their rides when increasing the travelled distance. The longer the distance, the higher the likelihood that (parts of) the trips can be shared. In addition, we see that there is also a higher variability, both at a ride level and at the geographical grid cell level, when kt is lower. In this scenario, we observe that a higher travel distance distribution also leads to more homogeneous (and higher) shareability levels across the city and across users.
Figure 11. Distributional analysis of the percentage of trips shared in a monocentric city under two travel distance distribution scenarios: with short trips (top) and long trips (bottom).

When considering the differences in total vehicle hours (Figure ), we observe a similar trend in the distribution. This is expected, as there is a direct relation between travel distance and resulting vehicle hours. However, in this case, we see a higher variability for the case of higher kt. As more people are willing to travel to the centre of attraction and travel distances are longer, the extent of the detour becomes more variable. Keep in mind that passengers experience no detour when either starting close enough to their destination in a shared ride or when they did not find a match and travelled using private ride-hailing.
Figure 12. Distributional analysis of the increase in vehicle hours following the introduction of ride-pooling in a monocentric city under two travel distance distribution scenarios.

To consider the two aforementioned effects jointly, we analyse the distributional effect of the total passenger costs in Figure . Overall, we observe a similar trend as above, meaning an increase in the shareability indicator value when kt = 3. However, the differences in this scenario are considerably smaller because of the discount benefits being compensated by the prolonged travel times when more people are inclined to travel longer distances. In this case, variability is less pronounced and the differences between the two cases are modest.
Figure 13. Distributional analysis of the reduction in passenger costs following the introduction of ride-pooling in a monocentric city under two travel distance distribution scenarios.

Finally, we consider the scenario of a simulated polycentric city with four centres of attraction and its effects on shareability (Figure ). When compared to the heatmap and histogram shown in Figure , we see that the share of shared trips is lower in this scenario than in the corresponding monocentric scenario. This is expected as destinations are concentrated in four different areas instead of just one, which reduces the chances of passengers having mutually compatible rides. In addition, we see that there is a higher heterogeneity across the city, which is related to the proximity of each origin to the potential destinations. People in the peripheral areas now have a reduced chance to share their rides because (i) the chance of finding a feasible pooling option is lower and (ii) the detour penalty is higher for those, resulting in less attractive matches and hence the ride-pooling alternative is less likely to be selected over private rides.
Figure 14. Distributional analysis of the percentage of trips shared in a polycentric urban area with four centres under two travel distance distribution scenarios.

In summary, the main insights from our experiment are as follows:
As trip distance increases, shared rides are characterised by higher occupancies and larger reductions in vehicle hours and passenger costs.
The higher the concentration of destinations around centres, the higher the vehicle occupancy, vehicle hours and passenger cost reductions, except for when trip distances are particularly long.
Increasing the number of centres of attraction has a limited impact on vehicle occupancy, vehicle hours and passenger costs.
Shorter travel distances lead to greater spatial variability in the degree of shared rides across the city.
Longer travel distances are associated with longer detours and a higher variability of detour length across the city.
Increasing the number of centres reduces the number of attractive shared rides, especially for those living in the peripheral areas of the city.
4. Discussion and conclusions
Shared rides are often considered to be a promising travel alternative that could efficiently pool people together while offering a door-to-door service. Despite this premise, when examining large-scale ride-pooling services in large urban areas, they are either not yet available or have not yet claimed a significant market share. For ride-hailing service providers to offer a ride-pooling alternative, it is of utmost importance to ensure that demand levels are sufficiently high so that there is a non-negligible probability that trips could be matched. Otherwise, the service provider bears the risk of offering considerable discounts to passengers who end up travelling on their own (even though they signed up for a shared ride service) without saving on driver commissions. The likelihood that trip requests can be matched depends on their mutual compatibility in terms of the trip’s origin, destination and departure time. While this mutual compatibility is an essential prerequisite, it is by no means sufficient. Hence, when matching trips with rides in our study, we not only ensure their mutual compatibility in time and space, we also guarantee that shared rides are only composed of travellers who find the ride-pooling offer to be more attractive than the private ride-hailing alternative given the trade-offs between travel time, fare and discomfort (i.e. unwillingness to share).
Our analysis sheds light on the consequences of the spatial demand pattern on the resulting prospects for a ride-pooling service. On one hand, a demand pattern that is more concentrated in space is likely to offer more opportunities for matching similar trips without inducing substantial detours. On the other hand, the incentive to share a ride is greater for longer rides due to the per-kilometre discount offered. There is, therefore, a non-trivial relation between demand pattern characteristics and its potential shareability, which calls for a detailed empirical assessment. Our findings indicate that introducing a ride-pooling service can yield a reduction in vehicle hours ranging between 18 and 59%, depending on the concentration of travel destinations around the centre and the trip length distribution. System efficiency correlates positively with both dimensions; highly concentrated destinations and longer trips produce the highest reduction in vehicle hours. The respective on-board occupancy level ranges between 1.25 and 1.74. Passenger cost savings hover between 2-3% for all scenarios. These gains are attained by passengers who shift from private to shared rides because they find that the discount more than compensates for the detour and discomfort experienced (otherwise they would not have opted for the ride-pooling alternative). Interestingly, the number of major centres of attraction across the city does not have a considerable impact, everything else being equal.
The results suggest that cities characterised by (i) compact centre(s) of attraction and (ii) where travellers are inclined to use ride-hailing for those trips where destinations are located further away from their origins, i.e. in our case study of Amsterdam this corresponds to an average trip distance of 5-8 km, offer the most fertile ground for attracting users to share their rides and result in an increased efficiency in on-demand transport services. Our analysis of the distributional effects in shareability levels under different demand patterns also reveals that those patterns that induce the most ride-pooling are also those that result in the most even distribution of service performance – both across the population as well as across different geographical parts of the urban area. This demonstrates that the impacts of ride-pooling are not limited to select localised effects but are rather well distributed because the efficiency gains are made possible by matching individuals with a diverse set of origins and destinations (in contrast to a shuttle service).
Further research may extend our analysis by sampling travel origins and examining the impacts of alternative trip generation and attraction patterns. This will allow for analysing more complex spatial relations that extend beyond the (mono or poly)centric patterns assumed in this study. In particular, how different centres of attraction are distributed in polycentric cities might also be relevant for further research. Similarly, the daily temporal variations in the demand patterns analysed in this study have been specified based on the travel demand profile data for Amsterdam. Future research may also examine how different temporal and spatial profiles, based on travel patterns observed for other cities, may impact the potential of ride-pooling services. In addition, it would be interesting to compare empirical shareability measurements and their spatial distribution to validate the results presented in this study. Future research should address the lack of benchmarking in the domain of matching algorithms for ride-pooling services. This calls for a comparison of alternative (exact and heuristic) algorithms for various (pre-booked, en-route) on-demand services in terms of system performance, computational efficiency and their impact on shareability potential under different demand patterns.
The approach adopted in this study can be coupled with models of innovation diffusion and supply evolution (de Ruijter et al. Citation2022) to identify the relevant conditions for obtaining a critical mass of users and drivers in a two-sided on-demand transport platform. Moreover, embedding our method in a travel demand model that includes a feedback loop to modal choices will allow treating the total demand for on-demand transport services as an endogenous variable and hence conclude on the ability of ride-pooling services to attract users who, in its absence, will use alternative means of travel other than private ride-hailing.
Acknowledgements
This research was supported by the CriticalMaaS project (grant number 804469), which is financed by the European Research Council and the Amsterdam Institute for Advanced Metropolitan Solutions.
Disclosure statement
No potential conflict of interest was reported by the author(s).
Notes
References
- Alonso-Mora, J., S. Samaranayake, A. Wallar, E. Frazzoli, and D. Rus. 2017. “On-Demand High-Capacity Ride-Sharing Via Dynamic Trip-Vehicle Assignment.” Proceedings of the National Academy of Sciences of the United States of America 114 (3): 462–467. doi:10.1073/pnas.1611675114.
- Arentze, T. A., and H. J. P. Timmermans. 2004. “A Learning-Based Transportation Oriented Simulation System.” Transportation Research Part B: Methodological 38 (7): 613–633. doi:10.1016/j.trb.2002.10.001.
- Badia, H. 2020. “Comparison of Bus Network Structures in Face of Urban Dispersion for a Ring-Radial City.” Networks and Spatial Economics 20: 233–271. doi:10.1007/s11067-019-09474-5.
- Bassolas, A., H. Barbosa-Filho, B. Dickinson, X. Dotiwalla, P. Eastham, R. Gallotti, and J. J. Ramasco. 2019. “Hierarchical Organization of Urban Mobility and Its Connection with City Livability.” Nature Communications 10 (1): 1–10. doi:10.1038/s41467-019-12809-y.
- Boisjoly, G., E. Grisé, M. Maguire, M. P. Veillette, R. Deboosere, E. Berrebi, and A. El-Geneidy. 2018. “Invest in the Ride: A 14 Year Longitudinal Analysis of the Determinants of Public Transport Ridership in 25 North American Cities.” Transportation Research Part A: Policy and Practice 116 (May): 434–445. doi:10.1016/j.tra.2018.07.005.
- Cats, O., R. Kucharski, S. R. Danda, and M. Yap. 2022. “Beyond the Dichotomy: How Ride-Hailing Competes with and Complements Public Transport.” PLoS ONE 17 (1 January 2022). https://journals.plos.org/plosone/article/citation?id=10.1371/journal.pone.0262496.
- Cats, O., A. Vermeulen, M. Warnier, and H. van Lint. 2020. “Modelling Growth Principles of Metropolitan Public Transport Networks.” Journal of Transport Geography 82 (October 2019): 102567. doi:10.1016/j.jtrangeo.2019.102567.
- Daganzo, C. F., Y. Ouyang, and H. Yang. 2020. “Analysis of Ride-Sharing with Service Time and Detour Guarantees.” Transportation Research Part B: Methodological 140: 130–150. doi:10.1016/j.trb.2020.07.005.
- Dean, M. D., and K. M. Kockelman. 2021. “Spatial Variation in Shared Ride-Hail Trip Demand and Factors Contributing to Sharing: Lessons from Chicago.” Journal of Transport Geography 91. https://www.sciencedirect.com/science/article/pii/S0966692320310218?casa_token=0hZ9CylXD38AAAAA:yncfqba54yDwm_n5Lnh41KVRdFZxdXFwa5VQ8rr7kh4ve6KvtxgIWTptEO5J2-pvhGpaRKwN8g.
- de Ruijter, A., O. Cats, R. Kucharski, and H. van Lint. 2022. “Evolution of Labour Supply in Ridesourcing.” Transportmetrica B 10 (1): 599–626. doi:10.1080/21680566.2021.2024917.
- Erhardt, G. D., S. Roy, D. Cooper, B. Sana, M. Chen, and J. Castiglione. 2019. “Do Transportation Network Companies Decrease or Increase Congestion?” Science Advances 5 (5). doi:10.1126/sciadv.aau2670.
- Etminani-Ghasrodashti, R., and S. Hamidi. 2019. “Individuals’ Demand for Ride-Hailing Services: Investigating the Combined Effects of Attitudinal Factors, Land Use, and Travel Attributes on Demand for App-Based Taxis in Tehran.” Iran. Sustainability (Switzerland) 11 (20). https://www.mdpi.com/2071-1050/11/20/5755#cite.
- Ewing, R., and R. Cervero. 2010. “Travel and the Built Environment.” Journal of the American Planning Association 76 (3): 265–294. doi:10.1080/01944361003766766.
- Ewing, R., G. Tian, and T. Lyons. 2018. “Does Compact Development Increase or Reduce Traffic Congestion?” Cities 72 (August 2017): 94–101. doi:10.1016/j.cities.2017.08.010.
- Fielbaum, A., S. Jara-Diaz, and A. Gschwender. 2016. “Optimal Public Transport Networks for a General Urban Structure.” Transportation Research Part B: Methodological 94: 298–313. doi:10.1016/j.trb.2016.10.003.
- Ghaffar, A., S. Mitra, and M. Hyland. 2020. “Modeling Determinants of Ridesourcing Usage: A Census Tract-Level Analysis of Chicago.” Transportation Research Part C: Emerging Technologies 119. https://www.sciencedirect.com/science/article/pii/S0968090X2030680X?casa_token=9eRgrfrnzB4AAAAA:1YdcDnZDaFlerweE4c2_Inrf7QLVLrN5g2A5d5YPOYK8ELsnRyY1YwUaBlt7niXCxk69m7rJMQ.
- Guenthner, R. P., and K. Hamat. 1988. “Distribution of Bus Transit On-Time Performance.” Transportation Research Record 1202: 1–8.
- Ingvardson, J. B., and O. A. Nielsen. 2018. “How Urban Density, Network Topology and Socio-Economy Influence Public Transport Ridership: Empirical Evidence from 48 European Metropolitan Areas.” Journal of Transport Geography 72 (July): 50–63. doi:10.1016/j.jtrangeo.2018.07.002.
- Ke, J., Z. Zheng, H. Yang, and J. Ye. 2021. “Data-Driven Analysis on Matching Probability, Routing Distance and Detour Distance in Ride-Pooling Services.” Transportation Research Part C: Emerging Technologies 124. https://www.sciencedirect.com/science/article/pii/S0968090X20308214?casa_token=s-jJpqWCb14AAAAA:nu_4v6Pw9pFSLOd2ZIP-rj4FHfr7T2Q9m2AP7fagOaJL_ea08i2PJd92Rxf4YuVb1PxHMnUs1Q.
- Kim, J., and H. S. Mahmassani. 2015. “Compound Gamma Representation for Modeling Travel Time Variability in a Traffic Network.” Transportation Research Part B: Methodological 80: 40–63. doi:10.1016/j.trb.2015.06.011.
- Kucharski, R., and O. Cats. 2020. “Exact Matching of Attractive Shared Rides (ExMAS) for System-Wide Strategic Evaluations.” Transportation Research Part B: Methodological 139: 285–310. doi:10.1016/j.trb.2020.06.006.
- Kucharski, R., and O. Cats. 2022. “Simulating Two-Sided Mobility Platforms with MaaSSim.” PLOS ONE 17 (6): e0269682. doi:10.1371/journal.pone.0269682.
- Li, W., Z. Pu, Y. Li, and X. (Jeff) Ban. 2019. “Characterization of Ridesplitting Based on Observed Data: A Case Study of Chengdu, China.” Transportation Research Part C: Emerging Technologies 100 (January): 330–353. doi:10.1016/j.trc.2019.01.030.
- Li, Y., W. Xiong, and X. Wang. 2019. “Does Polycentric and Compact Development Alleviate Urban Traffic Congestion? A Case Study of 98 Chinese Cities.” Cities 88 (January): 100–111. doi:10.1016/j.cities.2019.01.017.
- Marquet, O. 2020. “Spatial Distribution of Ride-Hailing Trip Demand and Its Association with Walkability and Neighborhood Characteristics.” Cities 106. https://www.sciencedirect.com/science/article/pii/S0264275120312749?casa_token=3v-Y0c1Pfq0AAAAA:2mGgx81GyF3aYO1Bd4nHpb2sX80m2Qf543n52XXpvf2gQ6B5WikIu2IAAUAa-xoFLwvDpdjhFQ.
- Narayan, J., O. Cats, N. van Oort, and S. Hoogendoorn. 2021. “On the Scalability of Private and Pooled On-Demand Services for Urban Mobility in Amsterdam.” Under Review.
- Oke, J. B., Y. M. Aboutaleb, A. Akkinepally, C. L. Azevedo, Y. Han, P. C. Zegras, and M. E. Ben-Akiva. 2019. “A novel Global Urban Typology Framework for Sustainable Mobility Futures.” Environmental Research Letters 14 (9). https://iopscience.iop.org/article/10.1088/1748-9326/ab22c7/meta.
- O’Kelly, M. E., and M. A. Niedzielski. 2008. “Efficient Spatial Interaction: Attainable Reductions in Metropolitan Average Trip Length.” Journal of Transport Geography 16 (5): 313–323. doi:10.1016/j.jtrangeo.2007.11.003.
- Schiffer, R. G. 2012. “Long-Distance and Rural Travel Transferable Parameters for Statewide Travel Forecasting Models.” Long-Distance and Rural Travel Transferable Parameters for Statewide Travel Forecasting Models. https://books.google.nl/books?hl=en&lr=&id=_p0ilf0HizgC&oi=fnd&pg=PP1&dq=Long-Distance+and+Rural+Travel+Transferable+Parameters+for+Statewide+Travel+Forecasting+Models.+&ots=gRoq2ksx0z&sig=stgbwsdhAgdSkntiwtSADefZIh8&redir_esc=y#v=onepage&q=Long-Distance%20and%20Rural%20Travel%20Transferable%20Parameters%20for%20Statewide%20Travel%20Forecasting%20Models.&f=false.
- Soria, J., and A. Stathopoulos. 2021. “Investigating Socio-Spatial Differences between Solo Ridehailing and Pooled Rides in Diverse Communities.” Journal of Transport Geography 95. https://www.sciencedirect.com/science/article/pii/S0966692321002015?casa_token=yPqENkLEkNwAAAAA:LXXFa-3RVC5qYhQ-LqopD8dh42ZaG9dgZB8rwFdHz5r-uL6uWey94HENruPdqGieMJp2R3mcQw.
- Tachet, R., O. Sagarra, P. Santi, G. Resta, M. Szell, S. H. Strogatz, and C. Ratti. 2017. “Scaling Law of Urban Ride Sharing.” Scientific Reports 7 (October 2016): 1–6. doi:10.1038/srep42868.
- Tsekeris, T., and N. Geroliminis. 2013. “City Size, Network Structure and Traffic Congestion.” Journal of Urban Economics 76 (1): 1–14. doi:10.1016/j.jue.2013.01.002.
- Wang, K., and W. Zhang. 2021. “The Role of Urban Form in the Performance of Shared Automated Vehicles.” Transportation Research Part D: Transport and Environment 93 (March): 102744. doi:10.1016/j.trd.2021.102744.
- Young, M., S. Farber, and M. Palm. 2020. “The True Cost of Sharing: A Detour Penalty Analysis between UberPool and UberX Trips in Toronto.” Transportation Research Part D: Transport and Environment 87 (September): 102540. doi:10.1016/j.trd.2020.102540.
- Zwick, F., and K. W. Axhausen. 2022. “Ride-pooling Demand Prediction: A Spatiotemporal Assessment in Germany.” Journal of Transport Geography 100. https://www.sciencedirect.com/science/article/pii/S0966692322000308.