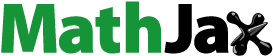
Abstract
Contract farming (CF) has been one of the strategies employed to enhance the production and productivity of malt barley and substitute imported malt barley in Ethiopia, in addition to addressing marketing challenges. The predicted results also include satisfying domestic malt barley demand, enhancing farmers’ welfare, and saving the nation’s foreign exchange. Hence, this study evaluated the production efficiency impact of malt barley CF in northwestern Ethiopia. The study was based on the data collected from 398 farmers in two districts, selected using multistage sampling techniques. The probit model showed that CF participation among malt barley farmers was positively correlated with frequency of extension contact, field day, training, cooperative membership, credit, and household size. The maximum likelihood estimator of SPF shows malt barley farm, fertilizer, and oxen power had positive effects on malt barley output. The stochastic cost frontier analysis demonstrated that the total cost of production was positively and significantly impacted by malt barley output and the cost of inputs (labor, oxen, farm, and seed). Propensity score matching (PSM) revealed that CF increased the TE, AE, and EE by 4.52%, 12.34%, and 11.55% for participants, respectively. The findings of this study demonstrate that CF can increase farm households’ TE, AE, and EE, which policymakers and other interested organizations may consider as a different development strategy as long as it is adjusted to local conditions.
PUBLIC INTEREST STATEMENT
The production of malt barley has become one of Ethiopia’s fastest-growing industries because of the establishment and expansion of malt and brewery plants supported by expanding beer consumption and a favorable investment. However, the domestic supply of the grain lags far behind the demand. The domestic supply accounts less than 50%. As a result, Ethiopia is a net importer of malt barley. This limited supply is caused mainly by low yield. Theoretically, this can be improved by enhancing efficiency and diffusing technology. In this regard, malt barley contract farming (CF) is introduced as a strategy to increase malt barley yield by enhancing farmers’ production efficiency through technical help and supplying improved technology through financing and price support. This study evaluated the impact of CF on production efficiency of malt barley farmers in northwestern Ethiopia. The finding revealed that CF increased the technical, allocative, and economic efficiencies of malt barley farmers by 4.52, 12.34, and 11.55%, respectively. Therefore, policy makers should introduce CF to non-participants is a better way to improve production efficiency to increase malt barley yield.
1. Introduction
Barley (Hordeum vulgare) is one of the major crops widely grown in different countries of the world. It ranks fourth in the world in terms of production after wheat, maize, and rice (Food and Agriculture Organization [FAO], Citation2021). Ethiopia is one of the top 10 barley producers in the world. As a potential producer, it also ranks first in Africa followed by Algeria, Morocco, Tunisia, and South Africa, which account for about 25% of the total barley production on the African continent (Food and Agriculture Organization [FAO], Citation2018).
In Ethiopia, among the top five kinds of cereal (teff, maize, wheat, and sorghum), barley ranks fifth in terms of area coverage and production contribution in 2019/20 (Central Statistical Agency [CSA], Citation2022). In 2020/21, maize, wheat, barley, and teff accounted for 64% of the cultivated area and 71% of total production. In this production year, barley is in the third rank. There are two types of barley namely food barley for consumption and malt barley for the brewery. Malt barley is one of the targeted crops for the selected commodities (Agricultural Transformation Institute [ATI], Citation2022). It is grown in a variety of conditions at altitudes between 1500 and 3500 meters above sea level (Senait Bekele et al., Citation2020).
Barley is a staple food and a source of cash income in Ethiopia. In this sector, more than 3.6 million smallholder farmers produced about 20.72 million quintals on 799,127.84 ha of land with a productivity of 25.93 qt/ha in 2022 (Central Statistical Agency [CSA], Citation2022). However, the productivity of Ethiopian barley is lower than that of best-performing African countries (for instance, Kenya and South Africa have average barley productivity of 32.6 and 29.3 qt/ha; respectively), and much lower than European countries (France and Germany produced more than 60 qt/ha of barley) (Food and Agriculture Organization [FAO], Citation2021). Its yield is also lower than its potential productivity ranges of 30 to 60 qt/ha (Bishaw & Molla, Citation2020). Hence, the productivity of barley is lower than its potential and global standards. The productivity of malt barley also follows a similar trend (Shiferaw et al., Citation2022). Moreover, the average barley productivity of Amhara region in 2021/22 was (22.54 qt/ha) is lower than the national (25.93 qt/ha) and Oromia Region (28.56 qt/ha) (Central Statistical Agency [CSA], Citation2022). In addition to low productivity, spatial productivity disparity reported among the potential barley producers zones of Amhara Region. According to the same source, the barley yield in 2021/22 for South Gondar, North Gondar, Central Gondar, North Wollo, South Wollo, North Shewa, East Gojjam, West Gojjam, Wghemra and Awi zones is 24.33, 21.65, 22.37, 21.29, 15.91, 25.73, 26.98, 25.66, 13.52 and 23.58qt/ha, respectively.
The production of malt barley has become one of Ethiopia’s fastest-growing industries because of the establishment of multinational brewery plants supported by expanding beer consumption and a favorable investment policy. According to International Center for Agricultural Research in the Dry Areas [ICARDA] (Citation2019), the demand is outpacing malt barley production due to its low productivity (Rashid et al., Citation2015) coupled with increasing demand for beer due to increasing per-capita incomes ($124.5 in 2000 and $855.76 in 2019) (World Bank [WB], Citation2021), population growth, and urbanization (Ethiopian agricultural transformation agency [ATA], Citation2017) as well as the construction of new malt factories ([Global Agriculture Information Network [GAIN], Citation2021). For instance, the average malt barley productivity in 2016/17 was 18.3 qt/ha for the Amhara region and 20.4 qt/ha for the country as a whole (Central Statistical Agency [CSA], Citation2017). Low productivity of malt barley in particular and barley in general are due to several factors, including production inefficiency (Shiferaw et al., Citation2022), a lack of improved technology (improved seed and fertilizers), limited market access, and a lack of technical expertise (Bishaw & Molla, Citation2020; Ferede & Demsie, Citation2020; Mpeta et al., Citation2018; Tadesse & Derso, Citation2019), among others. Theoretically and empirically, increasing efficiency and/or diffusing technology can increase production and productivity. Contract farming (CF) can achieve these objectives by providing new market opportunities, credit, farm inputs, new technology, and training (Mwambi et al., Citation2016). In other words, CF has the advantage of increasing agricultural productivity by enhancing farmers’ production efficiency through technical help and supplying improved technology through financing and price support. CF is an institutional arrangement in which a company contracts the production of agricultural products to farmers and guarantees a reliable supply of high-quality agricultural raw materials (Bellemare & Novak, Citation2017).
In Ethiopia, CF has been employed in the last 10 years as a solution for several agricultural commodities (Bezabeh et al., Citation2020; BIF, Citation2018, Biggeri et al., Citation2018; Yeshitila et al., Citation2020). Malt barley CF is one, which was introduced in 2013 by multinational malt and brewery firms in response to this need (Addisu, Citation2018; Business Innovation Facility [BIF], Citation2018; New Business Ethiopia [NBE], Citation2017; Rashid et al., Citation2015). Malt barley CF is mainly implemented in Oromia and the Amhara Region, with brewery factories to ensure local malt supply (Addisu, Citation2018; BIF [Business Innovation Facility], Citation2018). This is because both food and malt barley production is concentrated in the Oromia and Amhara Regions, which contribute 53% and 30% of national production, respectively (CSA [Central Statistical Agency], Citation2022). The same source also shows that the Oromia, Amhara, Tigray, and Southern Regions account for 99.5% of the country’s land and barley output among the current 11 regions. CF is employed by malt factories to regularly purchase malt at stable prices, and offer technologies (improved seed and fertilizer) and technical assistance to CF participants in exchange for a reliable supply of high-quality malt barley in the North Gondar Zone, and elsewhere in the nation. However, the demand for raw malt barley is unmet. As a result, in 2015 and 2018, the national supply of malt barley met only 35% (14,452 tons) and 44% (52,000 tons) of the domestic demand of the breweries, respectively (Addisu, Citation2018; Business Innovation Facility [BIF], Citation2018; National Bank of Ethiopia [NBE], Citation2017), with the remaining malt barley imported from abroad. The cost of malted barley imports in 2015 and 2018 was 51.97 million USD and 283.68 million USD, respectively (FAO, Citation2021). Moreover, the import cost of malt barley is expected to reach USD 420 million in 2025 (Rashid et al., Citation2015). This demonstrates the significance of CF for Ethiopia’s capacity to raise malt barley yield and production, to conserve hard currency, improve farmer welfare, and reduce transaction costs for the malt and beer industries. Therefore, improving malt barley yield and production is necessary to meet the growing demand for malt by augmenting the CF with empirical evidence. In North Gondar Zone, the study area, the Dashen beer factory introduced CF malt in 2014 with local sourcing of raw malt barley (BIF [Business Innovation Facility], Citation2018). Since then, the Gondar Malt Factory’s (GMF) demand for the grain has remained insufficient despite 25,025 farmers being actively involved in the cultivation of malt barley, of which 5570 (22.3%) were CF participants in 2021/22 (Beauro of Agriculture [BoA], Citation2020). According to the same source, the number of producers, including CF participants and non-participants, the volume of malt barley supplied, and the area covered, all demonstrate a declining tendency in the Amhara Region (2016/17 to 2020/21) (Table , Figures ). The yield and area allocated in the North Gondar Zone are both trending downward (Figure ). However, the Gondar malt factory’s demand for malt barley from the Oromia Region is increasing (Table , Figure ). The causes of the low productivity of malt barley in the region could be socioeconomic factors among others. This implies that empirical evidence is imperative to know the sources of low participation in CF and its impacts on production efficiency.
In general, in addition to overcoming marketing difficulties, CF has been one of the strategies used to improve the production and productivity of malt barley and substitute imported malt barley in Ethiopia. Besides meeting domestic malt barley demand, the expected outcomes include improving the welfare of smallholder farmers, and saving the foreign currency of the nation. In this regard, there have been numerous studies performed on the impacts of CF on the welfare of farmers. The majority of research demonstrates that CF has a favorable impact on income (Bellemare & Novak, Citation2017; Bezabeh et al., Citation2020; Biggeri et al, Citation2018; Mulatu et al., Citation2017; Tefera & Bijman, Citation2021), food security (Bellemare & Novak, Citation2017; Ganewo et al., Citation2022), productivity (Tefera & Bijman, Citation2021; Wendimu et al., Citation2016), production efficiency (Bidzakin et al., Citation2020; Chakraborty, Citation2009; Nguyen et al., Citation2018; Yeshitila et al., Citation2020), and asset (Michelson, Citation2013). For instance, Bidzakin et al. (Citation2020) and Yeshitila et al. (Citation2020) report that CF improves the economic efficiency (EE) of Indian rice, and Ethiopian sesame; respectively. However, the majority of these studies focus on income, while most of the impact studies of CF on production efficiency; mainly focused on technical efficiency (TE), are done on other commodities and elsewhere, except for Yeshitila et al. (Citation2020) who conducted on technical efficiency (TE), allocative efficiency (AE) and economic efficiency (EE) impact of sesame CF.
On the contrary, a few researchers have verified that CF is viewed as a tool for agricultural businesses to exploit farmers’ resources, hence limiting household gain from CF (Abdulai & Al-Hassan, Citation2016; Mpeta et al., Citation2018; Olounlade et al., Citation2020; Ragasa et al., Citation2018; Wendimu et al., Citation2016). For instance, CF reduces the TE of small-scale sunflower production in Tanzania (Mpeta et al., Citation2018). Similar study note that CF has reduced the asset stock and revenue of Ethiopian sugarcane growers (Wendimu et al., Citation2016). Olounlade et al. (Citation2020) claim that CF in Benin also has a detrimental effect on the livelihood and security of rice producers. Similarly, CF reduced the income of soybean producers in Ghana (Abdulai & Al-Hassan, Citation2016). According to a study by Ragasa et al. (Citation2018), CF reduces the income of Ghanaian maize farmers. This implies that CF has a negative effect on TE, assets, income, and food security.
Furthermore, the majority of previous studies not considered the selection bias in favor of a single production frontier model assuming that CF participants and non-participants have equal access to technology and share the same production frontier (for instance Shiferaw et al., Citation2022). Others, such as Yeshitila et al. (Citation2020), employed propensity score matching to examine the impact of CF on sesame production efficiency by controlling selection bias from observable factors. However, research on the effects of various interventions on farm productivity has argued for the importance of taking unobserved heterogeneities into account. These studies examined the effects of natural resource management projects (Bravo-Ureta et al., Citation2012), CF (Bidzakin et al., Citation2020; Mpeta et al., Citation2018), improved management (Rahman et al., Citation2020), agricultural cooperative (Chen et al., Citation2020), credit (Koricho & Ahmed, Citation2022), and cooperative (Olagunju et al., Citation2021) on farm TE and showed not accounting for the selection bias from unobserved characteristics. These studies demonstrated that ignoring the selection bias from unobserved variables may lead to an incorrect estimate.
Therefore, in contrast to prior studies, this analysis seeks to adjust for both observed and unobserved heterogeneity in CF participation decisions and production efficiency. Accordingly, PSM and sample selection model was used to control bias stemming from observable and unobservable factors (Greene, Citation2010). Hence, the objective of this study was to identify the determinants of CF participation and evaluate the impact of CF on TE, AE and EE. This empirical study will therefore be valuable for planners and policymakers to enhance and increase malt barley and other commodities productivity by CF.
2. Methodology
2.1. Description of the study area
The study area, North Gondar Zone is found in Amhara National Regional State, located in the northwestern part of Ethiopia. Debark is the main town of the North Gondar Zone, which is located 817 km and 90 Km from the capital Addis Ababa and Gondar, respectively. It has six rural Districts and two urban administrations covering an area of 38,685.79 square kilometers. It has a total population of 914,266, of whom 452,922 are men and 461,344 women (North Gondar Zone [NGZ], Citation2020). It is bordered on the south by East Belesa, on the west by Tegede, Tach Armachiho, and Wogera Districts, on the north by the Tigray region, and on the east by Wag Hemra Zone. The mixed farming system; livestock rearing and crop production is mainstream in the study area. The livestock population was 888,938.30 TLU (tropical livestock unit). The main crops are cereals such as teff, maize, wheat, millet, barley, and sorghum (North Gondar Zone [NGZ], Citation2020).
The highland parts of the Zone have the potential for both food and malt barley production. Malt barley is cultivated in Beyeda, Janamora, Debark, and Dabat districts. The average malt barley productivity of this Zone, Debark, and Dabat districts was 18.39 qt/ha, 18.96 qt/ha and 17.93 qt/ha; respectively in 2020 (Gondar Malt factory [GMF], Citation2020). This productivity is below the potential and international standards. According to the same source, malt barley CF was introduced in 2014 by the Dashen brewery factory with the objective of local sourcing of malt barley. The total number of malt barley producers in North Gondar zone was 5170; of which 2234 (43.2%) were CF participants. According to the Zone Department of Agriculture report, malt barley is the third most important crop among cereals. This indicates that malt barley is among the main cereal crops in the Zone. Figure below indicates the study area; which is the North Gondar Zone of Debark and Dabat districts.
2.2. Sample size and sampling procedures
A multistage sampling procedure was implemented to draw the targeted sample of malt barley farmers who are CF participants and non-participants. In the first stage, among CF participants and non-participants malt barley producing districts, Debark, and Dabat districts were selected purposively among four malt barley growing districts since both malt barley CF participants and non-participants are found in these districts. In the second stage, four kebeles namely Abtera, Dabat Zuria, Woken Zuraia, and Chena from the Dabat district; and five kebeles such as Arginjona, Miligebsa, Miqara, Yekirar, and Gomiya from Debark district were taken by following simple random sampling. Thirdly, the farmers were stratified into malt barley CF participants and non-participants by taking CF engagement as the criterion. Then, to make the sample size of each stratum comparable and have enough matching groups for the treatment group (CF participants), 189 malt barley CF participants and 209 non-participants were selected using simple random sampling based on probability proportional to their size by having the fresh lists of the farmers from the respective kebeles and districts.
2.3. Data types, sources, and method of data collection
Pretested and revised semi-structured interviews were used to collect primary data on the output, inputs and costs of malt barley, demographic, socioeconomic, resource endowments, and environmental factors from a sample of CF participant and non-participant malt barley farmers. The interview schedule was prepared in English and it was translated into the local language, Amharic. Secondary data were collected from Government and non-government reports, as well as online data sources like FAOSTAT.
2.4. Data analysis method
2.4.1. Descriptive statistics
The study used descriptive statistics (mean, standard deviation, frequency, and percentage) and inferential statistics (chi-square test and t-test). The t-test and chi-square test were used to determine statistical mean differences and the association between CF participants and non-participants on continuous and discrete variables; respectively.
2.4.2. Econometric model
The probit model was employed to identify the determinants of CF participation and predict propensity score and PSM was used to measure the impacts of CF on TE, AE and EE. All the data analysis was done by STATA version 17 and NLOGIT 5. The estimation of conventional stochastic production and cost frontier for both unmatched and matched samples and PSM was generated using STATA version 17 software. NLOGIT version 5 was used to estimate the sample selection stochastic production and cost frontier (SPF and SCF) models.
2.4.2.1. Malt barley contract farming participation decision
Farmers CF participation decision can be guided by different choice theories. Decision/choice theory is the study of the reasoning underlying an agent’s choices. The main choice theories are constant utility theory and random utility theory (RUM). However, constant utility theory fails to account the imperfection of CF due to “bound rationality and opportunistic behaviour” of the decision making unit (Ben-Akiva & Lerman, Citation1985).
The most well-known theoretical paradigm is transaction cost economics (TCE), which evolved from the pioneering works of (Coase, Citation1937) and (Williamson, Citation1989). It is the main theoretical foundation for CF and a component of New Institutional Economics (NIE). The premise of NIE and TCE is that all interactions between economic actors have transaction costs. According to TCE, economic agents have rationally bounded behavior and a propensity for opportunism. The asset specificity, uncertainty, and exchange frequency characteristics of transactions have an impact on the level of transaction costs (Bijman, Citation2008; Minot, Citation2011).
Asset specificity is caused by an investment that the farmer or buyer makes that is unique to a given transaction and has little to no value for a different application (Hobbs, Citation1996). The motivation to enter into a CF to safeguard such assets rather than using the spot market increases with the degree of asset specificity. Factors that are challenging and costly to predict are said to as uncertain. The main source of uncertainty is incomplete and asymmetrical information. Making successful transactions is difficult due to the lack of knowledge regarding the market circumstances for farmers and the quality of the product for buyers (Bijman, Citation2008). Finally, if transaction frequency is low, transaction costs will be high, and vice versa.
The firm favors vertical integration when transaction costs on the spot market are too high. The “hybrid” that exists between the extremes of spot markets and vertical integration is CF. The firm will then choose the organization that minimizes transaction costs. This implies that when transaction costs diminish, households become more efficient, and more capital and labor are freed to produce wealth (asset). In this context, CF can reduce the negative effects of transaction costs due to an imperfect market by granting malt barley farmers access to agricultural technologies (improved seed and fertilizers), access to finance, a guaranteed price for the output, and technical support (extension services: advice, training, field demonstration).
According to transaction cost economic theory, the benefits of CF in addressing market imperfections caused by farmers’ behavior (bound rationality and inclination for opportunism) and the nature of the commodity (asset specificity, uncertainty, and transaction frequency) must be measured. Hence, following Ben-Akiva and Lerman, (Citation1985), Louviere et al. (Citation2000), and (Khonje et al. (Citation2015), the theoretical framework for participation decisions in CF of the farmers is the random utility model where farmers choose a strategy that provides the highest utility among the given alternatives. The link it provides to microeconomics is another important advantage of the RUM in the study of choice. Different behavioral paradigms can be incorporated into models to account for the potential that an agent’s behavior is uncertain or unpredictable in itself due to bound rationality and opportunistic behaviour (Stephane & Nesha, Citation2012). And this utility is not directly observed, rather it is observed through the farmers’ choice. Suppose represents the latent variable of the expected utility that the ith households drives by participating in CF compared with that of non-participating
. By comparing the costs and benefits of participating in CF, the household participates in CF only when the net benefits are greater than the costs:
. The net benefit
, therefore, is a latent variable determined by explanatory variables: demographic, socioeconomic, institutional, and environmental factors, and the random term.
Where Ii is binary and equals 1 if farmers participated in CF and 0 otherwise, xi stands for explanatory variables, β represents unknown parameters that need to be estimated, and is the error term with a normal distribution i.e
. Thus, the likelihood of participating in CF is presented as:
where F is the cumulative distribution function for εi. EquationEquation (2)(2)
(2) is estimated by using the probit model (Wooldridge, Citation2010). EquationEquation (3)
(3)
(3) represents the model to calculate the likelihood of observing a farmer participating in CF:
where Pr is the probability of Yi being the binary choice variable (1 for participants and 0 for non-participants in CF), Z=Xβ and others are as defined above.
The log-likelihood function of the probit model is specified as
where F, Pr, X, Y, and β are defined above.
Empirically, the probit model used to compute farmers’ CF participation choices is shown as follows:
Where ui is a random error term and xi reflects the factors influencing the decision of farmers to participate in CF.This model is estimated using the maximum likelihood (ML) estimator.
For interpretation of the probit model result, the marginal effect is preferred and used in this study. Hence, the marginal effect is:
2.4.2.2. Production efficiency estimation
Production efficiency of malt barley includes Technical efficiency (TE), Allocative Efficiency (AE) and Economic efficiency (EE). Efficiency is the production possibilities that compare observed and optimal values of its output and input. It is classified into technical and allocative efficiency (Farrell, Citation1957). TE refers to the ability of a decision-making unit (DMU) to produce the maximum feasible output from a given bundle of inputs (Chen et al., Citation2005; Farrell, Citation1957). AE refers to the ability to combine inputs and outputs in optimal proportions in the light of prevailing prices. EE is the product of TE and AE. Any deviation from this maximal output (minimum cost) is considered inefficiency (Coelli et al., Citation2005). To measure production efficiency, either parametric or non-parametric methods can be used (Simar & Wilson, Citation2015). The non-parametric technique, like Data Envelopment Analysis, assumes that the DMUs have complete control over the production process and that any departures from the frontier are indicative of inefficiency (Coelli, Citation1995; Kumbhakar & Lovell, Citation2000). However, because of the prevalence of factors outside of a farmer’s control in agriculture, it is difficult to agree that all deviations from the frontier are inefficient (Bauman & Jablonski, Citation2016). Thus, the parametric stochastic production frontier was used. Aigner et al. (Citation1977), and Meeusen and van Den Broeck (Citation1977) both independently proposed the stochastic production/cost frontier function to measure TE, AE and EE.
The production efficiency of malt barley was defined as:
Where: f(.) is the Cobb-Douglas functional form- the results of the generalized likelihood ratio (LR) test performed in this study favored the Cobb—Douglas production function over the translog specification, Yi is the actual malt barley output of the ith farmer, xi is a vector of inputs used by the ith farmer, β is a vector of unknown parameters, vi is a random variable beyond the farmer’s control, measurement errors as well as other statistical noise that are assumed to be and it is independent of ui, and ui is a nonnegative random variable assumed to account for production inefficiency.
Given the level of input, the TE of the ith malt barley farmers is the ratio of the observed output to the maximum potential output (frontier output):
The dual cost frontier Cobb-Douglas production was specified in Equationequation(9)
(9)
Where represents total production cost (minimum cost incurred), g is Cobb-Douglas functional form,
represents output produced,
represents the cost of input (land, seed, labor, oxen, and fertilizer),
represents the parameters of the cost function,
is a nonnegative random variable assumed to account for cost inefficiency and
is as defined above.
The cost efficiency of an individual farm is defined in terms of the ratio of the observed cost (C) to the corresponding minimum cost ( given the available technology. That is, cost efficiency
):
The individual farmer’s economic efficiency is the product of the individual farmer’s TE and AE. i.e. EE=TE*AE
Finally, in this study, the Cobb-Douglas functional forms for the CF participant and non-participant malt barley producers’ TE, AE, and EE, were estimated independently by using two-stage estimating processes and a maximum likelihood estimator because we failed to estimate simultaneously the determinants of economic efficiency in single stage estimation procedure in stata software (Bravo-Ureta & Rieger, Citation1991; Wana & Sori, Citation2018).
2.4.2.3. Production efficiency estimation with bias correction
A combination of approaches is utilized to control for biases from both observed and unobserved variables to evaluate the impact of CF on the production efficiency of malt barley. The PSM technique is paired with the Greene (Citation2010) frontier model to correct for biases from observed characteristics and selectivity bias coming from unobserved variables, respectively. This is done by following the works of Bravo-Ureta et al. (Citation2012) and González-Flores et al. (Citation2014). PSM is not an appropriate technique if unobservable characteristics affect the outcome variable (Khonje et al., Citation2015; Takahashi & Barrett, Citation2014). Hence, the Greene (Citation2010) frontier model is adopted to control for selectivity bias arising from unobserved variables within the SPF formulation. This model can be expressed as follows:
where is a binary variable equal to one for CF participants and zero for non-participants,
is the amount of malt barley yield (total cost incurred) by the ith farmers, z is a vector of covariates included in the sample selection equation, and xi is a vector of inputs (costs and output) in the production (cost) frontier used by the ith farmers;
and
are parameters to be estimated while
denotes the characters in the error structure corresponding to the typical characterization of a stochastic frontier model and
captures the presence or absence of selectivity bias. However, the homogeneity test after matching shows that there was no bias due to unobservable factors. Hence, we proceed with PSM to evaluate the impact of CF on EE.
2.4.2.4. Impact of contract farming on production efficiency of malt barley farmers
The propensity scores matching method (PSM) was used to evaluate the impact of malt barley CF on the economic efficiency (EE) of smallholder farmers. It is the most widespread method of impact evaluation (Bezabeh et al., Citation2020; Ganewo et al., Citation2022; Yeshitila et al., Citation2020). It is chosen from among the nonexperimental methods (quasi-experimental method) because it does not require baseline data and is considered the second-best alternative to experimental design in minimizing selection biases (Caliendo & Kopeinig, Citation2008). PSM was used to estimate if there is a significant difference in the mean values of outcome indicators (TE, AE, and EE scores) between CF participants and non-participants. Firstly, the propensity score was predicted using the probit model (treated = 1 and untreated = 0) in the PSM method. CF participant malt barley farmers were considered as treatment groups while the controls were non-participant malt barley farmers. According to Caliendo & Kopeinig (Citation2008), the average treatment effects on the treated (ATT) were estimated by PSM. ATT is the mean difference between the expected outcome values with and without the treatment for those who participated in the intervention (Caliendo & Kopeinig, Citation2008). Particularly, assume that there are two expected outcomes, Yo and Y11. Y1. refers to the outcome of CF participants and Yo denotes the outcome of non-participants. The average effect of participation in CF on the participants’ outcome (production efficiency) is the difference between their expected outcome when participating and the expected outcomes if they had not been participating in the CF. Therefore, ATT is specified as:
Where ATT refers to the average effect of participation in CF on the malt barley farmers’ production efficiency (TE, AE, and EE), X denotes the vector of observed characteristics of the household that affect the decision in participating in CF and/or expected outcome (TE, AE, and EE) of farmers and used as independent variables, P refers to the probability to participate in CF (p = 1, if households participate in CF, and p = 0, otherwise).
The problem arising when estimating ATT given Equationequation (12)(12)
(12) is that it could not be measured
since it is not observed. The fact that it could not be observed simultaneously the outcome in the case of non-participation for the same person who participated in the CF. This problem may be solved by replacing unobserved outcome values (missing) of CF participants’
with the expected outcome values of matched non-participant who has similar observable characteristics to the CF participants
called counterfactual outcome. Hence, Equationequation (12)
(12)
(12) could be rearranged as follows:
The propensity score, which is the individual probability of participating given the observable variables, might be condensed as the vector X to verify the similarity of characteristics in a dimension (Ito et al., Citation2012). The impact of CF on malt barley producers’ production efficiency was therefore estimated using EquationEquation (13)(13)
(13) . Two key assumptions must be satisfied for PSM to be successful in estimating ATT. Firstly, the conditional independence assumption (CIA) of expected results and selection into the treatment given observable variables (Rosenbaum & Rubin, Citation1983). In other words, all factors influencing the choice of treatment and the expected results must be considered (Caliendo & Kopeinig, Citation2008). The overlap or common support condition, which guarantees that there exist control subjects with the same observable variables for each treated subject, is the second condition (Nannicini, Citation2007). To evaluate ATT using the PSM approach, a five-step process was modified (Nhan, Citation2019), including choosing the variables that were utilized for matching, estimating the propensity scores, selecting the matching algorithm, matching based on the estimated propensity score, checking and evaluating the balance for confounders before and after matching, and estimating the average treatment effects from the matched data.
Finally, sensitivity analysis was made to check the quality of comparison matching between CF participants and non-participants with observed covariates. It is used mainly to check robustness for unobserved covariates and the outcome. Following Caliendo and Kopeinig (Citation2008), it is assumed that the participation probability πi is determined by observable factors (Xi) and an unobservable component (ui):
Where Xi is the observed characteristics for individual malt barley farmer i, is the unobserved variable and
is the effect of
on the participation decision. If unobserved variables do not affect, then
will be zero and the participation probability is solely determined by x.
2.5. Operational definition of variables
Having a review of the literature, and the researcher’s observation, the input variables to determine the status of TE, and cost of inputs and malt barley output to compute AE were defined as follows in Table , and the covariates that were used to predict the propensity score and identify the factors that affect CF participation are summarized in Table .
Table 1. Variables definitions of inputs and cost of malt barley to determine TE and AE status
Table 2. Covariates used in probit model and predict propensity score for PSM
2.5.1. Dependent variable
Participation in contract farming is the participation status of the sample household in malt barley CF in 2021/22. It is a dummy that assumed one if the farm household heads CF participate in the 2021/22 production period, and otherwise zero.
Malt barley output(kg/ha) is the amount of malt barley output produced by the ith farmers in the 2021/22 production years measured in kg per ha and it was in log form that was used to compute the score of TE.
Total cost (Birr/ha): is the sum of the cost of the inputs incurred for malt barley production by ith farmers in 2021/22 that is measured in birr per ha and was used to compute the AE score.
Outcome: the TE, AE, and EE scores were used as an outcome variable to compute the impact of CF.
2.5.2. Independent variables for stochastic frontier technical and allocative efficiency analysis
Malt barley productivity is influenced by the levels of inputs used in the production process. The yield response of malt barley at various levels of inputs applied in the production process was evaluated in the smallholder production system. The inputs per season, namely, land, labor, seed, fertilizer, and oxen were employed; which was employed in the stochastic frontier Cobb-Douglas production function analysis. In the stochastic frontier production efficiency analysis, the inputs are the independent variables; which are operationally defined as follows.
Production inputs: previous studies have shown that the amount of land allotted, labor used (family, hired, exchange, or gift), seed, fertilizer, chemicals, and oxen labor are expected to have a positive impact on output in the production function (Bekele & Regasa, Citation2019; Getachew et al., Citation2018; Koye et al., Citation2022; Shiferaw et al., Citation2022).
Plot size refers to the total area (including owned, contractual rent, and land obtained on a share crop basis) of farms allotted to malt barley cultivation during the 2021/22 production period. During the data collection process, it was measured in timad (a quarter of a hectare) that was converted into a hectare. The land is the main factor of production; thus, the expected coefficient was hypothesized to be positive.
Labor refers to the total amount of pre-harvest labor (the supply of household labor force and external labor, such as hiring and exchange labor) used for different agronomic practices of malt barley production, such as land preparation, sowing, input application, and weeding. Since harvesting does not affect the level of output, unless there is unexpected rainfall, disease, etc., these will destroy the production of malt barley. According to the conversion coefficients of the working classes of different sex and ages participating in the production process, this was converted into man-days equivalent (Emana & Storck, Citation1992). Labor in man-days equivalent per ha was employed. It was hypothesized that the available labor is positively related to production efficiency.
Seed refers to the amount of malt barley seeds used for the cultivation of malt barley in the 2021/22 season, in kilograms per ha. Seeds are a universal input in all crop-based agricultural systems and affect crop productivity in two ways. Due to high competition for nutrients, too high seed density will lead to low yield, and very low seed density will also lead to low yield due to insufficient land use. Therefore, its influence on malt barley production efficiency was expected to be indeterminate.
Fertilizer: refers to the kilograms per ha of NPS (nitrogen, phosphorous, and sulfur), DAP, and urea used to grow malt barley in 2021/22. On the malt barley plot, urea is applied either once or twice, whereas NPS is often applied when the crop is being sown. NPS and urea in malt barley should be added in concentrations of 121 kg/ha and 100 kg/ha, respectively (Bishaw & Molla, Citation2020; Zewdie et al., Citation2021). Although the amount of fertilizer used is anticipated to have a favorable impact on output, using too much fertilizer could lead to low yield or even a complete loss of harvest. Therefore, it was anticipated that using chemical fertilizer at the appropriate application rate and time would improve farmers’ productivity.
Oxen labor the quantity of oxen work (measured in pairs of oxen days) utilized for malt barley cultivation is referred to as “oxen labor.” It is one of the primary inputs of production due to small-scale farmers and less mechanized agricultural activities. For plowing, oxen are employed as power. To quantify oxen power, the total number of oxen days per ha allotted for various malt barley production tasks (such as plowing and sowing/harrowing) during the 2021/22 production season was employed. Therefore, it was assumed that farmers with more oxen would be more productive than other farmers.
To compute allocative, the opportunity cost of daily labor wages, the rental value per ha of land, and the rental value pair of oxen per day were employed to measure the cost of labor, land, and oxen; respectively. Instead; the market prices were employed per kg of malt barley seed and per kg of fertilizers. Besides, malt barley output was measured in kg per ha as defined above. Generally, malt barley output and the cost of all inputs used in malt barley production are the independent variables that determine cost efficiency. The cost of land was the annual rental value (in Birr) per ha of land expressed for all plots of land used in malt barley production in 2021/22. Labor cost is the opportunity cost of daily labor wages; which was used to measure the cost of all sources of labor. The cost was calculated considering the cost of hired labor, family labor, and exchange labor for all agronomics practices (pre-harvest practices) for malt barley production in 2021/22. This cost was taken in Ethiopian Birr (ETB) per ha. The cost of oxen power was estimated by multiplying the rental value pair of oxen per day by the total oxen days used in the malt barley production process (plowing and sowing or harrowing) in 2021/22. It was converted into ETB per ha to compute cost efficiency. The cost of fertilizer refers to the total cost of NPS and Urea paid for the production of malt barley in 2021/22 measured in ETB per ha. The cost of seed was the amount of ETB per kg paid by the farmers to purchased seed and converted in to ETB per ha.
2.5.3. Covariates for malt barley contract farming participation and PSM
Sex: refers to the sex of the household head, if the household head is male, then it is one and zero otherwise. Sex is expected to have a positive influence on the participation of CF positively since a male-headed household has better access to information, control resources, and access to credit than a female-headed household (Wendimu et al., Citation2016). Hence, sex was expected to be positively related to participation in malt barley CF.
The age of the household head: is a continuous variable in years; which is a proxy for experiences. It is learning by doing and improving the decision-making power of the household head. This means that older farm households have greater access to productive resources such as land and labor for use in the production process. As age increases, farmers also accumulate more experience and thus, be more participants in CF. According to reports, age is positively related to participation in CF (Bellemare & Novak, Citation2017; Bezabeh et al., Citation2020). However; the farmer at a certain level of age may get physically weaker and ineffective in agronomic practices and lack access to information that has a negative influence on participation in CF. Studies have shown that age has an inverse impact on participation in CF (Maertens & Vande Velde, Citation2017; Olounlade et al., Citation2020). Hence, age was expected to have an indeterminate effect on malt barley CF participation.
Household size: is a continuous variable measured in the count of who is living within the household. It is directly related to CF participation (Olounlade et al., Citation2020) since CF is expected to be labor-intensive rather than capital-intensive. It is hypothesized that if labor contributions dominate consumption, the large family-sized household is expected to have better participation in malt barley CF. Therefore, household size was expected to have an indeterminate influence on malt barley CF participation.
Level of Education: refers to the level of education of the household head, which was measured by years of schooling. Education facilitates the management capacity, and ability of farmers to make informed decisions. It enhances the ability of the household head to gather, analyze and use relevant information, which is expected to have a direct relationship with the participation in malt barley CF. Studies indicated that the level of education is positively related to CF (Tefera et al., Citation2020). In this study, the level of education was expected to have a positive effect on the likelihood of participation in malt barley CF.
Total land holding of the household: -is a continuous variable measured in hectares (ha) under the control of the household head. In the current scenario, it has a positive correlation with participation in malt barley CF (Ganewo et al., Citation2022). Hence, it was hypothesized that land size was expected to have a direct relationship with CF.
Off-farm is a dummy variable that takes a value of one if the household participated in the off-farm activities and zero otherwise. It refers to the involvement of the household members in off-farm activities; which is a proxy variable for off-farm income that may have negative and positive effects on the participation in malt barley CF. That is to say, a household head who is engaged in off-farm activities may not manage his/her malt barley farm timely due to labor competition (Tefera et al., Citation2020), while as Olounlade et al. (Citation2020) reported off-farm income can be used to purchase inputs such as improved inputs (seed, fertilizer, chemicals) and labor for farm management activities. It is assumed that this variable was expected to have indeterminate effects on participation in malt barley CF. Frequency of extension contact: refers to the number of days contacted between the household head and extension experts per malt barley production season. The extension improves the knowledge, attitude towards technology, and skills of household heads through consultation, demonstration, training, and field visits of model farmers. It was expected to have a direct relationship with the participation in malt barley CF because extension experts advise about the benefits of malt barley CF on market security and other supports (Ganewo et al., Citation2022; Tefera et al., Citation2020). Hence, the more extension contacts the household head had likely participated in malt barley CF. Participation in training is a dummy variable, if the household head participated in training; it would be one and zero otherwise. Training access can promote the knowledge and decision-making skills of the household head. Training and participation in malt barley CF were found to be directly related (Dube, Citation2019). So, it was expected that having access to training would have a positive influence on the household head’s participation in the malt barley CF. Experience in malt barley production and marketing: the number of years the household head has been involved in the production of malt barley. According to Ganewo et al. (Citation2022), more experienced farmers participated in CF than less experienced ones. Hence, it was expected that malt barley production experience positively determines participation in malt barley CF. Mobile ownership is a dummy variable that takes one if the household head had a mobile and zero otherwise. It refers to having a mobile to improve the ability of the household head to obtain information. Owning a mobile increases the likelihood of farmers participating in malt barley CF by improving information access. It was also found that mobile ownership has a direct effect on CF participation (Tefera et al., Citation2020). Hence, having a mobile allows household heads to participate in malt barley CF. Credit access: it was a dummy variable that takes one for those who received credit from formal lending institutions in 2020/21 and zero otherwise. It gives the input purchasing power to the household heads. Credit access is directly related to CF participation (Bezabeh et al., Citation2020; Tefera et al., Citation2020). Hence, credit access was hypothesized to encourage household heads to participate in malt barley CF.
Distance to market: is a continuous variable measured in walking minutes. It is a proxy variable for market access for malt barley producers. Market access encourages household heads to purchase and sell inputs and outputs; respectively (Bezabeh et al., Citation2020; Ganewo et al., Citation2022; Tefera et al., Citation2020). In this study, distance from the market was hypothesized to have an inverse relationship with participation in malt barley CF. Distance to FTC: is a continuous variable measured in walking minutes. The household heads nearest to the farmers’ training center (FTC) have the opportunity to observe the demonstration at the FTC, and, pieces of training, information, and advocacy services are easier to obtain than those who are far from the FTC. It is inversely related to participation in CF (Bezabeh et al., Citation2020; Ganewo et al., Citation2022; Tefera et al., Citation2020). It was expected to have a negative influence on malt barley CF participation decision of the household head. Participation in social affairs refers to the leadership position in formal (Gote, village, and kebele) or informal organization (edir, equb, and mahiber) in the community. It is a dummy variable that takes the value of one if the household head participated at least in one of the various social statuses and zero otherwise. It was expected to have an indeterminate effect on participation in CF. For instance, this participation might have a positive effect in the sense that the household head may have information access, experience sharing, and labor as well as other resources sharing to participate in CF (Rondhi et al., Citation2020). Contrarily, taking part in social activities may compete with the family’s resources. Hence, the influence of participation in social affairs of the household head on CF participation was indeterminate. Field day participation: is a dummy variable that takes one if the household head participated in the field day and zero otherwise. The households who had field day participation experiences were expected to participate in malt barley CF because seeing is believing. It is of course among the extension approaches to disseminating technologies through sharing the best experiences from their model peers and on-farm and on-station demonstration sites. According to Yami and Bekele (Citation2013) and Mathewos et al. (Citation2021) field day participation has a direct relationship with technology adoption. Hence, participation in the field day was expected to motivate household heads to participate in malt barely CF.
Cooperative membership is a dummy variable that takes one if the household is a member of the cooperative and zero otherwise. Cooperatives are expected to offer malt barley producers viable information about production technologies, deliver improved inputs, and arrange ways of easy access to credit services to encourage farmers (Bezabeh et al., Citation2020). Hence, cooperative members are more likely to participate in malt barley CF.
3. Result and discussion
3.1. Characterization of malt barley farmers
The equality means and association tests of continuous and discrete variables, respectively, are shown in Tables by CF participation.
Table 3. Summary statistics of contract farming participants and non-participants
Table 4. Association tests for contract farming participants
Regarding the continuous variables, there was a significant difference in average age, farming experiences, household size, land size, and frequency of extension contact between CF participants and non-participants at 1 and 5% significance levels. The household size of both participants and non-participants was more than the national (6.23) and the regional average (4.53) (Central Statistical Agency [CSA], Citation2021). The average land holding per household of both participants and non-participants was more than the national (0.84 ha) and less than the regional average (1.15 ha) (Central Statistical Agency [CSA], Citation2021).
Table further reveals that, aside from seed, which was statistically insignificant, participants allocated more cropland, and used more fertilizer, oxen power, and human labor than non-participants for the cultivation of malt barley. Moreover, malt barley yields were about 17.96 qt/ha and 16.34 qt/ha, respectively, for participants and non-participants, which is statistically significant at a 1% significance level. It is less than the national (20.4 qt/ha), regional (18.3 qt/ha), and zonal (18.39 qt/ha) yields in 2021 (Central Statistical Agency [CSA], Citation2021). This figure is also lower than what Tefera and Bijman (Citation2021) found in the Arsi zone of Oromia, where the average malt barley yield for samples, CF participants, and non-participants is 20.03qt/ha, 21.67qt/ha, and 18.8qt/ha, respectively.This may be due to the farmers’ low adoption of malt barley technology packages (Feyisa, Citation2020; Mekuria & Tadesse, Citation2015), the existence of production inefficiency and the unability to reallocate available resources (Bidzakin et al., Citation2018,Shiferaw et al., Citation2022; Yeshitila et al., Citation2020). Other than the cost of malt barley seed, it was found that participants’ malt barley total costs, which included labor, oxen power, fertilizer, and farmland were greater than those of their counterparts and statistically significant.
Regarding the discrete variables in Table , participation in CF is statistically significant at a 1% significance level and has a positive association with training, membership in a cooperative, participation in field days, and access to credit. These indicate that, in comparison to their counterparts, most CF participants had more access to credit, took part in training and field days, and were cooperative members. This is essential for improving the yield and production of malt barley, which in turn ultimately raises farmers’ standard of living.
3.2. Determinants of contract farming participation
Table displays the findings from the probit model on the variables affecting malt barley farmers’ CF participation. The probit model was employed for the estimation of the propensity scores as it best fits the data compared to the logit model. For the goodness-of-fit (gof) tests after probit and logit, the logit model’s Pearson chi-square is significant at 10%, suggesting that the errors in the logistic regression are not logistically distributed (Table ). The Wald chi-square (LR χ2(16) = 88.51, Prob > χ2 = 0.000) test showed statistical significance at the 1% probability level, indicating that the null hypothesis that the model had no explanatory power was strongly rejected. There was no systematic variation in the distribution of variables between CF participants and non- participants, according to the pseudo-R2, which is moderately low at 0.2029. Among sixteen covariates, six of them namely frequency of extension contacts, training participation, cooperative membership, household size, participation in field day, and access to credit were discovered to statistically significantly predict malt barley CF participation with expected signs. The probit model indicates that 45.5% of farmers were likely to participate in CF. This implies that the rate of malt barley participation was found to be below 50%. Household size and CF participation were positively and significantly correlated. It had a 3% marginal effect and was significant at a 5% probability level. This implies as the household size increase by one, the probability of malt barley farmers participation in CF will be 3%. This finding agrees with those made by Ganewo et al. (Citation2022). They claim that household size has a positive influence on CF participation.
Table 5. Probit model results of determinants of contract farming participation
Access to credit had a positive influence on malt barley CF participation. It was statistically significant at a 1% probability level and had a marginal effect of 26%. This finding is consistent with findings made by (Tefera & Bijman, Citation2021), and (Ganewo et al., Citation2022).
Field day participation positively and statistically significantly affected malt barley farmers’ participation in CF, as was hypothesized. This implies that field day participants are more likely to involve in CF than those who did not have it. Its marginal effect was found to be 45.1% and statistically significant at a 1% probability level. This finding is in agreement with that of Abdurehman and Abdi (Citation2021), and Mathewos et al. (Citation2021), who revealed a direct relationship between field day participation and technology adoption.
The frequency of extension contact had a significant influence on CF participation at a 5% level. The frequency of extension contact has a 3% marginal effect. Tefera et al. (Citation2020), Tefera and Bijman (Citation2021), and Ganewo et al. (Citation2022) reported similar findings.
Cooperative membership had a positive and statistically significant effect on CF participation at a 10% level of probability as expected. Its marginal effect was found to be 14%. This finding is consistent with prior research (Bellemare & Novak, Citation2017; Bezabeh et al., Citation2020). While CF for broilers in Indonesia has a negative relationship with cooperative service (Rondhi et al., Citation2020).
Training participation was positively and statistically significant at a 1% probability level related to CF participation as was expected. This implies that the farmers who had at least a single training access were more likely to participate in CF than those who had no training access. The marginal effect of training participation was found to be 21%. This result is in line with research that shows that training access is positively related to participation in the CF (Dube, Citation2019).
3.3. Estimates and estimation of production efficiency
3.3.1. Preliminary tests
Before the estimation of the stochastic production frontier, various hypothesis tests were conducted and presented in Table . The existence of the inefficiency component of the composed error term of the stochastic frontier model is the first null hypothesis that is tested. This is made to decide whether the traditional average production function (OLS) best fits the data set as compared to the stochastic frontier model (SFM) selected for this study. The hypothesis tests were conducted based on the generalized log-likelihood ratio test: LR=−2×(ln(H0)-ln(H1) where ln(H0) and ln(H1) are the log-likelihood values under the null hypothesis H0 (OLS, which is the value at zero iteration) and alternative hypothesis H1(SPM), respectively. LR=−2×[−169.69137-(−169.69137)] = 66.42. This value is greater than the critical value Χ2 (1%, 1) value of 5.412 at 1 degree of freedom. Furthermore, the sigma-u, and lambda (λ) was statistically significant at a 1% level of probability; proving the presence of inefficiencies components in malt barley production (Table ). On top of this, the rejection of the null hypothesis of no technical inefficiency confirmed the appropriateness of SPF over the OLS.
Table 6. Hypothesis tests for production efficiency models
Table 7. The maximum likelihood estimates of the parametric stochastic production frontier
The next hypothesis test was choosing the proper stochastic production function specification form. The hypothesis tests were conducted based on the generalized log-likelihood ratio test LR= −2×(ln(H0) -ln(H1) = −2×[−162.488-(−153.5178)] = 17.94, where ln(H0) and ln(H1) are the log-likelihood values under the null hypothesis H0 (Cobb Douglas function) and alternative hypothesis H1(TL function), respectively, with a value of the degree of freedom computed based on the number of restrictions in the test. For that reason, as the calculated LR value is less than the critical value, the null hypothesis was not rejected that the Cobb Douglas production functional form adequately represents the data. Moreover, the AIC value for the Cobb Douglas production function (325) is relatively lower than the AIC value of the Translog production function (342), implying CD is more appropriate in representing the data (Table ). Hence, for its consistent results, Cobb Douglas specification has been uniformly applied in all of the estimations.
The next test about the distributional assumptions of the inefficiency terms (μi). Following Belotti et al. (Citation2013), the generalized LR test was used besides AIC. The LR test indicated that the normal/truncated normal distribution was found to be the best fit for inefficiency distribution for the data sets (Table ). The AIC also favored the truncated normal (343) than half-normal (355) implying that truncated normal has a lower AIC value (Table ). Hence, the normal-truncated normal was found to be the best fit for inefficiency distribution for the data sets. Note that the results from half-normal and exponential distribution are almost similar (Belete, Citation2020; Girma, Citation2019; Jondrow et al., Citation1982). Hence, no comparison was made between half-normal and exponential distributions as well as an exponential and truncated normal distribution.
The fourth test is to determine if a single frontier or separate frontier is suitable for the data set. A generalized LR test and the inclusion of CF participation as a regressor with the inputs in the deterministic production frontier were both used as crucial verification procedures. The conventional SPF LR [chi2 (5) = 33.25] test of unmatched samples showed a significant result at 1% probability level confirming that there was selection bias though CF had insignificant effect on the conventional SPF for the pooled sample. The degree of freedom is the difference between the number of parameters estimated under the pooled and the parameters estimated in the two groups: CF participants and non-participants (i.e. df = 12–7 = 5) (Table ). The same source also showed that the SPF correlation coefficients, or rho (w, v), under the sample selection frontier, were significant for unmatched samples of participants. Generally speaking, the LR test and rho (w, v) were statistically significant, which supported the existence of sample selection bias in the SPF for unmatched samples. This is also true for SCF (Table ). These all signify the use of the sample-selection correction stochastic production frontier model for unmatched samples. However, the calculated LR [chi2 (5) = 8.21] (Table ) and the sample selection SPF correction value [rho (w, v)] were insignificant at a 10% probability level for matched samples (Table ). This demonstrates that following matching, the sample selection bias caused by unobservable factors is eliminated for matched samples. As a result, PSM was used to evaluate the impact of CF on production efficiency.
Following the test for homogeneous production technology, the last test was to check whether or not the inefficiency effect model does not affect the inefficiency of smallholder farmers in the study area. To test this hypothesis, LR (the inefficiency effect) was calculated using the values of the generalized Log-Likelihood functions under the stochastic production function model (a model without explanatory variables of inefficiency effects: H0) and the full frontier model (a model with explanatory variables that are supposed to determine inefficiency of each: H1). The computed LR value of 41.8 was higher than the critical value of 29.141 at 14 degrees of freedom, indicating that the null hypothesis (H0) that the explanatory variables are both equal to zero was rejected at the 1% level of significance. All of the hypothesis tests confirm the specification of stochastic production frontier was found to be appropriate in estimating the efficiency of scores. Moreover, the Wald chi-square value [Χ2 = 72.83 (p = 0.0000)] shows the model fits the data well for the pooled, indicating the absence of wrong functional form specification (Table ). In this Table, the Wald chi-square value also statistically significantly 1% probability levels for CF participants and non-participants. Hence, the Cobb-Douglass parametric stochastic frontier (normal/truncated normal) production and cost function was used for the efficiency analysis.
3.3.2. Estimates of technical efficiency of unmatched samples
Table depicts the maximum likelihood estimate of the stochastic Cobb-Douglas production function. The estimated value of gamma (γ) in all of the frontiers was found to be greater than 0.70 in all frontiers. This indicates that the inefficiency effects are likely to be highly significant in the analysis of the value of the farm households (Battese & Coelli, Citation1995). In this study, hence, more than 70% of the total variation in the output is primarily attributed to the existence of technical inefficiency. Accordingly, the production of malt barley was influenced by oxen power, fertilizer, and malt barley farm size with expected signs. A similar finding is reported by Shiferaw et al. (Citation2022). In all frontiers, oxen power and fertilizer had positive effects on malt barley production at various probability levels. By the same token, the production of barley malt was significantly and positively related to farm size in both the pooled and non-participant frontiers. Oxen power had the highest elasticity value for pooled samples and participants, whereas the elasticity of malt barley farm size was the leading for non-participants. Note that the level of influence of each significant parameter depends on the numerical value of the coefficient. For instance, a 1% increase in oxen power will increase malt barley output for the pooled, participants and non-participants by 0.209%, 0.27%, and 0.14%, respectively. We can interpret the significant variables similarly.
The sum of all partial production elasticities inputs, i.e. returns to scale (RTS), for the pooled, participants and non-participants are consistently less than one, showing decreasing returns to scale. The return to scale of malt barley pooled, CF participants and non-participants were 0.474, 0.655, and 0.43; respectively. CF participants had a better return to scale than the pooled and non-participants though all of them were operated at decreasing returns to scale. This is because a 1% increase in all the specified production inputs will increase malt barley output by 0.474%, 0.655%, and 0.43% for the pooled, participants and non-participants; respectively. This finding is in line with Dessale (Citation2019), Bekele and Regasa (Citation2019), Beyene et al. (Citation2020), Bidzakin et al. (Citation2020), Yeshitila et al. (Citation2020), Alulu et al. (Citation2021), Argaw et al. (Citation2021) and Haile et al. (Citation2018),who found that the production of barley, wheat, malt barley, rice, sesame, haricot beans, soya beans, and sorghum, respectively, has decreasing returns to scale. In contrast, Adane et al. (Citation2021), Lema et al. (Citation2022) and Shiferaw et al. (Citation2022) discover that malt barley production in Southern Ethiopia, North Shewa, and Oromia Region follows an increasing return to scales.
3.3.3. Estimates of allocative efficiency for unmatched samples
The allocative efficiency of the households was also analyzed using a stochastic (parametric) Cobb-Douglas type cost function (normal/truncated normal). The results of the stochastic cost frontier model of unmatched samples are shown in Table . The values of gamma for pooled, CF participants, and non-participants were 82.6%, 83.3%, and 82.4% the variations in the total cost of malt barley production; respectively due to cost inefficiency level. The Wald-test statistics that measure the overall fitness of the frontier model were significant at a 1% probability level revealing the high explanatory power of the independent variables in the model for all frontiers. Accordingly, the malt barley output, wage for labor, cost of seed, the rental value of oxen, and the rental value of the malt barley farm determined the total cost of malt barley with the expected sign. This finding is consistent with Shiferaw et al. (Citation2022) that shows the minimum cost of malt barley is positively influenced by the opportunity cost of labor, oxen, and farmland as well as the amount of malt barley output. In all frontiers, the malt barley output, and the rental value of malt barley farm and oxen had positive effects on the total cost of malt barley at various probability levels. The wage for labor was positively and statistically significantly related to the total cost of malt barley production in pooled and participants’ frontiers. The rental value of the malt barley farm had the highest elasticity value for all frontiers. Note that the interpretation of the coefficient is based on the concept of elasticity. For instance, a 1% increase in the annual rental value of oxen will increase the total cost of malt barley for the pooled, participants and non-participants by 0.065%, 0.075%, and 0.041%, respectively. Similar interpretation can be followed for the rest significant variables.
Table 8. The maximum likelihood estimates of the parametric stochastic cost frontier
The sum of the coefficient estimates in the cost function was 0.521, 0.633, and 0.508 for pooled, participants and non-participants, respectively. This implies indicating an economy of size. That is, each additional unit of input adds less and less cost to the total cost of production of malt barley. Even though both groups are experiencing declining RTS, participants had higher RTS than non-participants. This result is consistent with the study conducted by Bidzakin et al. (Citation2020) and Yeshitila et al. (Citation2020) who reported that both CF participants and non-participant rice and sesame farmers in India and Ethiopia, respectively are operating in decreasing return to scale.
3.4. Impact of contract farming on production efficiency
3.4.1. Predicting propensity score and imposing common support region
The propensity scores were predicted, and the common support zone was also imposed once the probit model was estimated. The minimum of the maximum and maximum of the minimum comparisons were made in setting the common support conditions. Table shows the predicted propensity scores used to match participants with non-participants ranging from 0.0329 to 0.8944. For CF participants, the computed propensity scores ranged from 0.0426 to 0.8944 with a mean of 0.5616, while for the control groups, the values ranged from 0.0329 to 0.8688 with a mean of 0.3957. Consequently, the range of 0.0426 to 0.8688 was identified as the common support region. The chosen kernel estimator (bandwidth 0.1) found 187 treatment and 194 comparison-matched groups with a total of 381 observations for the analysis. This suggests that 95.73% of participants and non-participants were in the common support region, demonstrating significant overlap between the two groups. Besides the areas of off and on common support, Figure also displays the common support zone derived from the distribution of propensity scores for each group.
Table 9. Summary of propensity scores for CF participants and non-participants
3.4.2. Choice of the matching estimator
After determining the common support region and estimating the propensity score, the subsequent step is to find an appropriate matching estimator (algorithm) (Table ). The alternative matching estimators (algorithms) were searched to match the participant and non-participant households in the common support region. Based on the stated criteria, the best estimator was kernel matching (bandwidth of 0.1), which balances all covariates (i.e., all covariates are insignificant), has a lower pseudo R2, yielded a large matched sample, and has a smaller mean bias. Furthermore, it was noted in the literature that many researchers favored using kernel matching since it weighs the contribution of each member of the comparison group, allowing more weight to be given to those comparators that offer a better match. For instance, Heckman et al. (Citation1998) claimed that kernel matching outperforms other matching algorithms in lowering the standardized mean bias between treated and control groups. Additionally, this approach makes use of more information and has a smaller variance than they claimed. Therefore, a kernel matching approach (estimator) with a bandwidth of 0.1 was used to estimate the average treatment effect on the treated (ATT) by CF participation.
Table 10. Performance of different match algorithm by selection criteria
3.4.3. Matching quality
As emphasized by Rosenbaum and Rubin (Citation1983), ensuring the balancing condition is a crucial issue in PSM as it reduces the influence of confounding variables. Table presented the matching quality test or the covariate balancing test and the standardized bias declined of covariates after matching. Accordingly, all covariates were insignificant and the standardized bias were declined after matching. Almost all variables had a standardized difference in covariates after matching that ranged from 0.6% to 15.8% in absolute value, which is below the critical level of 20% suggested by Rosenbaum and Rubin (Citation1985) while the standardized difference in covariates before matching ranged from 2.8% to 81.3% in absolute value. After matching, the joint significant test in Table showed that the mean standardized bias decreased from 20.2 to 4, Pseudo-R2 decreased from 0.094 to 0.019, and the likelihood ratio became insignificant. The total bias was also reduced significantly via the matching process. Hence, the specification of the propensity score estimation process using kernel (0.1) was successful in balancing the distribution of covariates between participants and non-participants in malt barley CF.
Table 11. Balancing test of the covariates after matching
Table 12. Results of the joint significant test (kernel bandwidth (0.1))
3.4.4. Impact of CF on the production efficiency of malt barley farmers
Table shows kernel (0.1) estimates of the impact of CF on TE, AE, and EE after assuring the common support area and checking the PSM model’s matching quality. The participants’ TE, AE, and EE increased by 4.52%, 12.34%, and 11.55%, respectively. The TE, AE, and EE differences between participants and non-participants are significant at 1%. This shows that participants were more technically, allocatively, and economically efficient than their counterparts though both operating below the frontier. That is to say, CF participants will benefit from this treatment more than non-participants. The positive effect of CF on efficiency is that, in addition to providing inputs such as fertilizer and improved seed on a credit base, the malt factory has extension agents who make farm visits and provide technical assistance and training to improve production techniques. This finding is in line with Bidzakin et al. (Citation2020) and Yeshitila et al. (Citation2020). According to Bidzakin et al. (Citation2020), CF improves rice TE, AE and EE by 21%, 23%, and 26%; respectively in Ghana. Similarly, CF improves the TE, AE and EE of sesame farmers by 11%, 8%, and 8.7% (Yeshitila et al., Citation2020).
Table 13. Impacts of malt barley contract farming on production efficiency
3.4.5. Sensitivity analysis of hidden bias for the impact of CF on production efficiency
The sensitivity test is used to investigate whether the causal effect estimated from the PSM is susceptible to the influence of unobserved covariates. The legitimacy of propensity score analysis assumes of strongly ignorable treatment assignment that assumes all relevant covariates are employed in the treatment assignment and the bias due to the unmeasured covariates is ignorable. This test helps to explore how sustainable the treatment effect is to the potential effect of unmeasured covariates. If the estimated treatment effect is sensitive to the presence of unmeasured covariates, or in other words, the estimated treatment effect is possibly washed away with the unmeasured covariates, the treatment effect may be due to the bias of unobserved covariates rather than a true effect.
Table demonstrated that despite allowing participants and non-participants to have treatment odds that varied by up to eγ = 7 in terms of unobserved variables, the conclusion for the impact of malt barley on TE, AE and EE remained unchanged since the p-critical values are significant at all levels of the critical value of gamma. We can thus conclude that our impact estimate (ATT of TE, AE, and EE) is insensitive to unobserved selection bias, being pure impacts of malt barley CF. This study’s main contribution was identifying the pure impact of CF on TE, AE and EE that previous research had not considered. To analyze the pure impact of CF on TE, AE and EE, the PSM and sample selection model were employed to control bias resulting from observable and unobservable components, respectively. The LR test showed that there is no hidden bias resulting from unobserved factors after matching. The sensitivity analysis also proved that the outcomes such as TE, AE and EE are not affected by unobserved factors after matching. Consequently, the PSM result showed that the participants’ TE, AE, and EE increased by 4.52%, 12.34%, and 11.55%, respectively, in contrast to non-participant counterparts. This result is in line with the transaction cost economic theory.
Table 14. Sensitivity analysis of hidden bias for the production efficiency impact of CF
4. Conclusion and recommendation
Contract farming (CF) is a strategy used in Ethiopia to commercialize malt barley; however, participation is limited. Using information gathered from 398 smallholder farmers, this study attempted to investigate the variables influencing CF participation and its impact on the production efficiency (TE, AE, and EE) of malt barley production in northwestern Ethiopia. The inferential statistics shows significant mean difference and association between CF participants and non-participants. The probit model results demonstrate that household size, cooperative membership, frequency of extension contacts, field-day participation, training, and formal credit access positively and statistically significantly determined CF participation of malt barley farmers. Moreover, the PSM test estimated the CF participation impacts on TE, AE, and EE in the matched sample by t-statistic. The results revealed that CF participation statistically significantly contributes to the improvement of TE, AE, and EE of malt barley farmers. More precisely, CF participants are more technically, allocatively, and economically efficient than non-participants in the production of malt barley. This implies that involvement in CF improves malt barley farmers’ production efficiency and enables them to produce the maximum amount possible with the available technology. Therefore, introducing CF to non-participants is a better way to improve production efficiency. Hence, to exploit the contribution of CF on the TE, AE and EE enhancement of malt barley farmers, government and non-governmental organizations with other concerned bodies should scale up the CF participation of malt barley farmers, thereby improving the welfare of potential malt barley production areas in general and the study area in particular by accounting the context of the area. Furthermore, spillover effects of CF on other crops and non-participants should be considered to check whether it has negative or positive external effects.
Authors’ contribution
All authors contribute to the design of the study, data collecting, analysis, and critical review, and they all offer feedback on the paper’s content and organization. The final manuscript has been reviewed and approved by all authors.
Availability of data and materials
All authors declare that the data sets used in this manuscript are fully available upon request from the corresponding author.
Informed consent
Ethical approval and consent to participate does not apply to our study.
Acknowledgments
We expressed our gratitude to the Ministry of Education for funding this study, the North Gondar Agricultural Office for providing general information, the Agricultural Offices of the Dabat and Debark Districts for facilitating data collection and the provision of secondary data, and the farmers who provide primary data.
Disclosure statement
No potential conflict of interest was reported by the author(s).
Additional information
Funding
Notes on contributors

Abebe Dagnew
Abebe Dagnew is a lecturer and researcher at University of Gondar. Currently, he is a PhD student of Agricultural Economics at School of Agricultural Economics and Agribusiness, Haramaya University, Ethiopia. He obtained BSc and MSc in agricultural Extension and Agricultural Economics, respectively from Haramaya University. He has teaching and socioeconomic research experiences. He has been also head, department of Agricultural economics (2011-2016) and exam center Coordinator (2016-2019) of University of Gondar, Ethiopia. He has published 13 articles in reputable journals. His research interests include adoption of technology, food security and poverty, production efficiency, and impacts evaluations.
References
- Abdulai, Y., & Al-Hassan, S. (2016). Effects of contract farming on small-holder soybean farmers’ income in the eastern corridor of the Northern region, Ghana. Journal of Economics & Sustainable Development, 7(2). www.iiste.org
- Abdurehman, M., & Abdi, M. (2021). Determinants of farmers adoption decision of improved crop varieties in Ethiopia: Systematic review. African Journal of Agricultural Research, 17(7), 953–37. https://doi.org/10.5897/ajar2020.15197
- Adane, E. S., Tefera, T., & Gidey, G. (2021). Technical efficiency of malt barley production in Malga district of Southern Ethiopia. Innovative Systems Design and Engineering, 12(1), 1621. https://doi.org/10.7176/isde/12-1-03
- Addisu, B. A. (2018). Malt barley commercialization through contract farming scheme: A systematic review of experiences and prospects in Ethiopia. African Journal of Agricultural Research, 13(53), 2957–2971. https://doi.org/10.5897/ajar2018.13071
- Agricultural Transformation Institute. (2022). Increasing agricultural productivity across SSA. Our world in data. Accessed on February 10, 2023 at https://unctad.org/system/files/non-official-document/aldc_2022_pdsd_eth_pdw_3-4_mar_ppt_yifru_tafesse_eng.pdf
- Aigner, D., Lovell, C. A. K., & Schmidt, P. (1977). Formulation and estimation of stochastic frontier production function models. Journal of Econometrics, 6(1), 21–37. 6(1 https://doi.org/10.1016/0304-4076(77)90052-5
- Alulu, J., Jakinda Otieno, D., Oluoch-Kosura, W., & Ochieng, J. (2021). Comparison of technical efficiency and technology gaps between contracted and non-contracted vegetable farmers in Western Kenya. Cogent Food & Agriculture, 7(1). https://doi.org/10.1080/23311932.2021.1910156
- Argaw, B., Haji, H., & Tegegne, B. (2021). Economic efficiency soybean production: The case of smallholder farmers in Pawe Woreda, Ethiopia: Parametric approach. Journal of Economics & Sustainable Development. https://doi.org/10.7176/jesd/12-15-01
- ATA (Ethiopian agricultural transformation agency). (2017). Transforming Agriculture in Ethiopia 2016/17. Annual report, Addis Ababa, Ethiopia.
- Battese, G. E., & Coelli, T. J. (1995). A model for technical inefficiency effects in a stochastic frontier production function for Panel data. Empirical Economics, 20(2), 325–332. https://doi.org/10.1007/BF01205442
- Bauman, A., & Jablonski, B. B. R. (2016). Evaluating scale and technical efficiency among farms and ranches with a local market orientation. Renewable Agriculture and Food Systems, 34(3), 198–206. https://doi.org/10.1017/S1742170517000680
- Bekele, Y., & Regasa, G. (2019). Technical efficiency of smallholder malt barley producers in Tiyo district (Ethiopia). RUDN Journal of Economics, 27(3), 525–535. https://doi.org/10.22363/2313-2329-2019-27-3-525-535
- Belete, A. S. (2020). Analysis of technical efficiency in maize production in Guji Zone: Stochastic frontier model. Agriculture & Food Security, 9(1). https://doi.org/10.1186/s40066-020-00270-w
- Bellemare, M. F., & Novak, L. (2017). Contract farming and food security. American Journal of Agricultural Economics, 99(2), 357–378. https://doi.org/10.1093/ajae/aaw053
- Belotti, F., Daidone, S., Ilardi, G., & Atella, V. (2013). Stochastic frontier analysis using Stata. The Stata Journal: Promoting Communications on Statistics & Stata, 13(4), 719–758. https://doi.org/10.1177/1536867X1301300404
- Ben-Akiva, M. E., & Lerman, S. R. (1985). Discrete choice analysis: Theory and application to Travel demand. MIT Press.
- Beyene, T., Mulugeta, W., Merra, T., & Wong, W.-K. (2020). Technical efficiency and impact of improved farm inputs adoption on the yield of haricot bean producer in Hadiya zone, SNNP region, Ethiopia. Cogent Economics & Finance, 8(1), 1833503. https://doi.org/10.1080/23322039.2020.1833503
- Bezabeh, A., Beyene, F., Haji, J., Lemma, T., & Yildiz, F. (2020). Impact of contract farming on income of smallholder malt barley farmers in Arsi and West Arsi zones of Oromia region, Ethiopia. Cogent Food & Agriculture, 6(1), 1834662. https://doi.org/10.1080/23311932.2020.1834662
- Bidzakin, J. K., Fialor, S. C., Awunyo-Vitor, D., & Yahaya, I. (2020). Contract farming and rice production efficiency in Ghana. Journal of Agribusiness in Developing and Emerging Economies, 10(3), 269–284. https://doi.org/10.1108/JADEE-11-2018-0160
- Bidzakin, J., Fialor, S., & Yahaya, I. (2018). Production efficiency of smallholder rice farms under contract farming scheme in Ghana. Asian Journal of Agricultural Extension, Economics & Sociology, 25(1), 1–12. https://doi.org/10.9734/ajaees/2018/41057
- Biggeri, M., Burchic, F., Ciania, F., & Herrmann, R.(2018). Linking small-scale farmers to the durum wheat value chain in Ethiopia: Assessing the effects on production and well-being. Food Policy, 79(c), 77–91. https://doi.org/10.1016/j.foodpol.2018.06.001
- Bijman, J. (2008). Contract farming in developing countries: An overview working paper.
- Bishaw, Z., & Molla, A. (2020). Deployment of malt barley technologies in Ethiopia achievements and lessons learned.
- BoA (Beauro of Agriculture). (2020). Annual Report. Bahir Dar,
- Bravo-Ureta, B. E., Greene, W., & Solís, D. (2012). Technical efficiency analysis correcting for biases from observed and unobserved variables: An application to a natural resource management project. Empirical Economics, 43(1), 55–72. https://doi.org/10.1007/s00181-011-0491-y
- Bravo-Ureta, & Rieger. (1991). Dairy farm efficiency measurement using stochastic frontiers and neoclassical duality?. American Journal of Agricultural Economics, 41(2), 215–226. https://doi.org/10.2307/1242726
- Business Innovation Facility. (2018). Assessment of the malting barley market System in Ethiopia. Accessed on January 20, 2023 at http://www.ifpri.org/publication/barley-value-chain-ethiopia
- Caliendo, M., & Kopeinig, S. (2008). Some practical guidance for the implementation of propensity score matching. Journal of Economic Surveys, 22(1), 31–72. https://doi.org/10.1111/j.1467-6419.2007.00527.x
- Chakraborty, D. (2009). Contract farming in India. Review of Market Integration, 1(1), 83–102. https://doi.org/10.1177/097492920900100105
- Chen, J., Qu, R., Wu, Y. D., Jones, G., Li, W., Jin, S., Chang, Q., Cao, Y., Yang, G., Li, Z. J., & Frewer, L. (2020). Effects of Agricultural Cooperative Society on Farmers’ Technical Efficiency: Evidence from stochastic frontier analysis. Sustainability, 12, 1–13.
- Chen, X., Skully, M., & BROWN, K. (2005). Banking efficiency in China: Application of DEA to pre- and post-deregulation eras: 1993–2000. China Economic Review, 16(3), 229–245. https://doi.org/10.1016/j.chieco.2005.02.001
- Coase, R. (1937). The nature of the firm. Economica, 4(16), 386–405. https://doi.org/10.1111/j.1468-0335.1937.tb00002.x
- Coelli, T. (1995). Estimators and hypothesis tests for a stochastic frontier function: A Monte Carlo analysis. Journal of Productivity Analysis, 6(3), 247–268. https://doi.org/10.1007/BF01076978
- Coelli, T. J., Rao, D. S. P., O'Donnell, C. J., & Battese, G. E.(2005). An introduction to efficiency and productivity analysis. Springer Science & Business Media. https://doi.org/10.1007/978-1-4615-5493-6
- CSA (Central Statistical Agency). (2017). Report on area and production of major crops by private peasant holdings in 2016/2017 meher season, CSA, Addis Ababa,
- CSA (Central Statistical Agency). (2021). The federal democratic republic of Ethiopia central statistical agency farm management practices (private peasant holdings, meher season) statistical bulletin.
- CSA (Central Statistical Agency). (2022). Report on area and production of major crops by private peasant holdings in 2019/2020 meher season, CSA, Addis Ababa, Ethiopia retrieved on June 20/2023 from http://www.statsethiopia.gov.et/agriculture-2/
- Dessale, M. (2019). Analysis of technical efficiency of small holder wheat-growing farmers of Jamma district, Ethiopia. Agriculture & Food Security, 8(1). https://doi.org/10.1186/s40066-018-0250-9
- Dube, E. G. (2019). Analysis of farmers resource use efficiency on malt barley production and its impact on Food security in Lemuna-Bilbilo Wereda.
- Emana, B., & Storck, H. (1992). Improvement strategies for farming systems in the Eastern Highlands of Ethiopia. Agricultural Economics, 8(1), 57–77. https://doi.org/10.1111/j.1574-0862.1992.tb00231.x
- Farrell, M. J. (1957). The measurement of productive efficiency. Source: Journal of the Royal Statistical Society: Series A (General), 120(3), 253. https://doi.org/10.2307/2343100
- Ferede, M., & Demsie, Z. (2020). Participatory evaluation of malt barley (Hordium disticum L.) varieties in barley-growing highland areas of northwestern Ethiopia. Cogent Food & Agriculture, 6(1), 1–15. https://doi.org/10.1080/23311932.2020.1756142
- Feyisa, B. W. (2020). Determinants of agricultural technology adoption in Ethiopia: A meta-analysis. Cogent Food & Agriculture, 6(1), 1–10. https://doi.org/10.1080/23311932.2020.1855817
- Food and Agriculture Organization. (2021). FAOSTAT Database.
- Food and Agriculture Organization of the United Nations. (2018). FAOSTAT Database. Retrieved July from www.faostat.org
- GAIN (Global Agriculture Information Network). (2021). Assessments of commodity and trade issues made by USDA staff and not necessarily statements of official U.S. Government policy.
- Ganewo, Z., Balguda, T., Alemu, A., Mulugeta, M., Legesse, T., Kaske, D., & Ashebir, A. (2022). Are smallholder farmers benefiting from malt barley contract farming engagement in Ethiopia? Agriculture & Food Security, 11(1). https://doi.org/10.1186/s40066-022-00396-z
- Getachew, W., Lemma, Z., & Bosena, T. (2018). Economic efficiency of smallholder farmers in barley production in Meket district, Ethiopia. Journal of Development and Agricultural Economics, 10(10), 328–338. https://doi.org/10.5897/jdae2018.0960
- Girma, H. (2019). Estimation of technical efficiency of dairy farms in central zone of Tigray national regional state. Heliyon, 5(3), e01322. https://doi.org/10.1016/j.heliyon.2019.e01322
- Gondar Malt Factory (2020). Annual report. Bahir Dar.
- González-Flores, M., Bravo-Ureta, B. E., Solís, D., & Winters, P. (2014). The impact of high value markets on smallholder productivity in the Ecuadorean Sierra: A stochastic production frontier approach correcting for selectivity bias. Food Policy, 44, 237–247. https://doi.org/10.1016/j.foodpol.2013.09.014
- Greene, W.(2010). A stochastic frontier model with correction for sample selection. Journal of Productivity Analysis, 34(1), 15–24.
- Haile, K., Mohammed, J. H., Bahir, B. T., Haji, J., & Tegegne, B. (2018). Technical efficiency of sorghum production: The case of smallholder farmers in Konso district, Southern Ethiopia. Journal of Agriculture and Crops, 80, 50–62. www.iiste.org/
- Heckman, J., Ichimura, H., Smith, J., & Todd, P. (1998). Characterizing Selection Bias Using Experimental Data. Econometrica, 66(5), 1017. https://doi.org/10.2307/2999630
- Hika, W., & Sori, O. (2018). Analysis of economic efficiency of sesame (sesamum indicum L) production in Babogambel district of West Wollega zone, Oromia region, Ethiopia. Food Science and Quality Management, 76(1), 47–61.
- Hobbs, J. E. (1996). A transaction cost approach to supply chain management. Supply Chain Management: An International Journal, 1(2), 15–27. https://doi.org/10.1108/13598549610155260
- ICARDA (International Center for Agricultural Research in the Dry Areas). (2019). Seed info: Official newsletter of West Asia and North Africa (WANA) seed network. issue no. 56, seed Section. ICARDA.
- Ito, J., Bao, Z., & Su, Q. (2012). Distributional effects of agricultural cooperatives in China: Exclusion of smallholders and potential gains on participation. Food Policy, 37(6), 700–709. https://doi.org/10.1016/j.foodpol.2012.07.009
- Jondrow, J., Lovell, C. A. K., Materov, I. S., & Schmidt, P. (1982). On the estimation of technical inefficiency in the stochastic frontier production function model. Journal of Econometrics, 19(2–3), 233–238. https://doi.org/10.1016/0304-4076(82)90004-5
- Khonje, M., Manda, J., Alene, A. D., & Kassie, M. (2015). Analysis of adoption and impacts of improved maize varieties in Eastern Zambia. World Development, 66, 695–706. https://doi.org/10.1016/j.worlddev.2014.09.008
- Koricho, M. G., & Ahmed, M. H. (2022). The impact of credit on the technical efficiency of food crop producing smallholder farmers in Ethiopia. Agricultural Finance Review, 82(5), 847–856. https://doi.org/10.1108/AFR-08-2021-0108
- Koye, T. D., Koye, A. D., & Mekie, T. M. (2022). Analysis of technical efficiency of irrigated tomato production in North Gondar Zone of Amhara regional state, Ethiopia. Letters in Spatial and Resource Sciences, 15(3), 599–620. https://doi.org/10.1007/s12076-022-00314-8
- Kumbhakar, S. C., & Lovell, K. (2000). Stochastic frontier analysis: An Econometric approach. https://www.researchgate.net/publication/233765662
- Lema, T. Z., Masresha, S. E., & Neway, M. M. (2022). Analysis of the technical efficiency of barley production in North Shewa Zone of Amhara regional state, Ethiopia. Cogent Economics & Finance, 10(1). https://doi.org/10.1080/23322039.2022.2043509
- Louviere, J. J., Hensher, D. A., Swait, J. D., & Adamowicz, W. (2000). Stated choice methods. Cambridge University Press. https://doi.org/10.1017/CBO9780511753831
- Maertens, M., & Vande Velde, K. (2017). Contract-farming in staple Food chains: The case of rice in Benin. World Development, 95, 73–87. https://doi.org/10.1016/j.worlddev.2017.02.011
- Mathewos, T., Temesgen, D., Hamza, D., & Fesseha, H. (2021). Determinants of smallholder farmers’ participation in improved sheep production: The case of Doyogena district, Kembata Tembaro Zone, Southern Ethiopia. Advances in Agriculture, 2021, 1–17. https://doi.org/10.1155/2021/5514315
- Meeusen, W., & van Den Broeck, J. (1977). Efficiency estimation from Cobb-Douglas production functions with composed error. Source: International Economic Review, 18(2), 435. https://doi.org/10.2307/2525757
- Mekuria, W., & Tadesse, D. (2015). Determinants affecting adoption of malt-barley technology: Evidence from North Gondar Ethiopia. Journal of Food Security, 3(3), 75–81. https://doi.org/10.12691/jfs-3-3-2
- Michelson, H. (2013). Small farmers, NGOs, and a Walmart World: Welfare effects of supermarkets operating in Nicaragua. American Journal of Agricultural Economics, 95(3), 628–649. https://doi.org/10.1093/ajae/aas139
- Minot, N. (2011). Food security in Africa: Market and Trade policy for Staple Foods in Eastern and Southern Africa. European Review of Agricultural Economics, 38(2), 289–291. https://doi.org/10.1093/erae/jbr019
- Mpeta, D., Kuzilwa, J., & Sebyiga, B. (2018). ANALYSIS OF CONTRACT FARMING EFFECTS ON EFFICIENCY AND PRODUCTIVITY OF SMALL-SCALE SUNFLOWER FARMERS IN TANZANIA – A PROPENSITY SCORE METHOD APPROACH. Acta Scientiarum Polonorum Oeconomia, 17(1), 75–84. https://doi.org/10.22630/ASPE.2018.17.1.9
- Mulatu, G., Haji, J., Legese, B., & Ketema, M. (2017). Impact of participation in vegetables’ contract farming on household’s income in the central rift valley of Ethiopia. American Journal of Rural Development, 5(4), 90–96.
- Mwambi, M. M., Oduol, J., Mshenga, P., & Saidi, M. (2016). Does contract farming improve smallholder income? The case of avocado farmers in Kenya. Journal of Agribusiness in Developing and Emerging Economies, 6(1), 2–20. https://doi.org/10.1108/JADEE-05-2013-0019
- Nannicini, T. (2007). Simulation-based sensitivity analysis for matching estimators. The Stata Journal: Promoting Communications on Statistics & Stata, 7(3), 334–350. https://doi.org/10.1177/1536867X0700700303
- NBE (National Bank of Ethiopia). (2017). National Bank of Ethiopia 2016 2017 Annual report. http://www.nbe.gov.et
- Nguyen, A. T., Dzator, J., & Nadolny, A. (2018). Contract farming, Agriculture productivity and poverty reduction: Evidence from tea estates in Viet Nam. Asia-Pacific Sustainable Development Journal, 25(1), 109–145. https://doi.org/10.18356/b18dd784-en
- NGZ (north Gondar Zone). (2020). Annual agricultural production performance report,
- Nhan, T. Q. (2019). Effect of private-led contract farming on rice growers’ yield, cost, selling price and return: Evidence from Vietnam’s central Mekong Delta. International Food and Agribusiness Management Review, 22(5), 731–746. https://doi.org/10.22434/IFAMR2018.0093
- Olagunju, K. O., Ogunniyi, A. I., Oyetunde Usman, Z., Omotayo, A. O., Awotide, B. A., & Martínez-Paz, J. M. (2021). Does agricultural cooperative membership impact technical efficiency of maize production in Nigeria: An analysis correcting for biases from observed and unobserved attributes. PLoS One, 16(1), e0245426. https://doi.org/10.1371/journal.pone.0245426
- Olounlade, O. A., Li, G. C., Kokoye, S. E. H., Dossouhoui, F. V., Akpa, K. A. A., Anshiso, D., & Biaou, G. (2020). Impact of participation in contract farming on smallholder farmers’ income and food security in rural Benin: PSM and LATE parameter combined. Sustainability, 12(3), 901. https://doi.org/10.3390/su12030901
- Ragasa, C., Lambrecht, I., & Kufoalor, D. S. (2018). Limitations of contract farming as a pro-poor strategy: The case of maize out-grower schemes in Upper West Ghana. World Development, 102, 30–56. https://doi.org/10.1016/j.worlddev.2017.09.008
- Rahman, M. S., Mizanul, M., Kazal, H., & Rayhan, S. J. (2020). Improved management practices adoption and technical efficiency of shrimp farmers in Bangladesh: A sample selection stochastic production frontier approach. The Bangladesh Journal of Agricultural Economics, 41(1). http://ageconsearch.umn.edu
- Rashid, S., Abate, G. T., Lemma, S., Warner, J., Kasa, L., & Minot, N. (2015). The barley value chain in Ethiopia. Accessed on Decemeber 15, 2022 at http://ebrary.ifpri.org/cdm/ref/collection/p15738coll2/id/130012
- Rondhi, M., Aji, J. M. M., Khasan, A. F., & Yanuarti, R. (2020). Factors affecting farmers’ participation in contract farming: The case of broiler sector in Indonesia. Tropical Animal Science Journal, 43(2), 183–190. https://doi.org/10.5398/TASJ.2020.43.2.183
- Rosenbaum, P. R., & Rubin, D. B. (1983). The Central role of the propensity score in observational studies for causal effects. Biometrika, 70(1), 41–55. https://doi.org/10.1093/biomet/70.1.41
- Rosenbaum, P. R., & Rubin, D. B. (1985). Constructing a control group using multivariate matched sampling methods that incorporate the propensity score. American Statistician, 39(1), 33. https://doi.org/10.1080/00031305.1985.10479383
- Senait Bekele, S. B., Yoseph, T., & Ayalew, T. (2020). Growth, protein content, yield and yield components of malt barley (hordeum vulgare; L.) varieties in response to seeding rate at Sinana district, Southeast Ethiopia. International Journal of Applied Agricultural Sciences, 6(4), 61. https://doi.org/10.11648/j.ijaas.20200604.12
- Shiferaw, S., Haji, J., Ketema, M., & Sileshi, M. (2022). Technical, allocative and economic efficiency of malt barley producers in Arsi zone, Ethiopia. Cogent Food & Agriculture, 8(1), 1–17. https://doi.org/10.1080/23311932.2022.2115669
- Simar, L., & Wilson, P. W. (2015). Statistical approaches for non-parametric frontier models: A guided tour. International Statistical Review, 83(1), 77–110. https://doi.org/10.1111/insr.12056
- Stephane, H., & Nesha, B. B.(2012). Accounting for latent attitudes in willingness-to-pay studies: The case of coastal water quality improvements in Tobago. Environmental & Resource Economics, 52(1), 109–131.
- Tadesse, D., & Derso, B. (2019). The status and constraints of food barley production in the North Gondar highlands, North Western Ethiopia. Agriculture & Food Security, 8(1), 1–7. https://doi.org/10.1186/s40066-018-0248-3
- Takahashi, K., & Barrett, C. B. (2014). The system of rice intensification and its impacts on household income and child schooling: Evidence from rural Indonesia. American Journal of Agricultural Economics, 96(1), 269–289. https://doi.org/10.1093/ajae/aat086
- Tefera, D. A., & Bijman, J. (2021). Economics of contracts in African food systems: Evidence from the malt barley sector in Ethiopia. Agricultural and Food Economics, 9(1), 1–21. https://doi.org/10.1186/s40100-021-00198-0
- Tefera, D. A., Bijman, J., & Slingerland, M. A. (2020). Multinationals and modernisation of domestic value chains in Africa: Case studies from Ethiopia. The Journal of Development Studies, 56(3), 596–612. https://doi.org/10.1080/00220388.2019.1590551
- WB (World Bank). (2021) . World bank data. Retrieved from GDP per capita (current US$) - Ethiopia |. Data (worldbank.org).
- Wendimu, M. A., Henningsen, A., & Gibbon, P. (2016). Sugarcane out-growers in Ethiopia: “forced” to remain poor? World Development, 83, 84–97. https://doi.org/10.1016/j.worlddev.2016.03.002
- Williamson, O. E. (1989). Chapter 3 transaction cost economics. In Handbook of industrial organization (Vol. 1). https://doi.org/10.1016/S1573-448X(89)01006-X
- Wooldridge, J. M. (2010). Econometric analysis of cross Section and Panel data. MIT press.
- Yami, M., & Bekele, A. (2013). Determinants of farmers’ participation decision on local seed multiplication in Amhara region, Ethiopia: A Double hurdle approach. Article in International Journal of Science and Research, 2(1). www.ijsr.net
- Yeshitila, M., Isriya, B., Prapinwadee, S., & Auttapol, S. (2020). The impact of contract farming on technical efficiency in Ethiopia’s smallholder sesame production. Journal of the Austrian Society of Agricultural Economics, 16(4), 57–66.
- Zewdie, M. C., Moretti, M., Tenessa, D. B., Ayele, Z. A., Nyssen, J., Tsegaye, E. A., Minale, A. S., & Van Passel, S. (2021). Agricultural technical efficiency of smallholder farmers in Ethiopia: A stochastic frontier approach. Land, 10(3), 1–17. https://doi.org/10.3390/land10030246
Appendix
Table A1. Amhara region’s malt barley supply, demand, area coverage, and beneficiaries
Table A2. Post-estimation tests of the probit and logit model (n = 398)
Table A3. Conventional and selection SPF based on unmatched observation
Table A4. Conventional and selection stochastic production frontier (SPF) based on matched observation
Table A5. Conventional and selection stochastic cost frontier based on unmatched observation
Table A6. Likelihood-ratio test for technical inefficiency distribution assumption
Table A7. Comparison of production frontier models for TE analysis