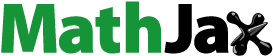
Abstract
Agricultural production is very risky, which can lead to significant shifts in income for individual farmers. De-risking input allocation due to production risk can change the level of technical efficiency. Therefore, the production risk component needs to be accounted for in the stochastic frontier model to produce unbiased estimates of technical efficiency. This study was conducted to assess the risks and efficiency of sesame production and to examine factors affecting farmers’ technical efficiency. A one-stage stochastic frontier model is used to estimate the production risk and technical inefficiency. The results show that the most suitable model for sesame production is the Cobb-Douglas function. The Cobb-Douglas model estimation shows that all agricultural inputs have a positive impact on sesame yield. Land and seeds are the most important factors in sesame production, followed by fertilizers, oxen, labour and chemicals. Fertilizers have been shown to reduce production risk, but oxen and chemicals are riskier. Sesame farmers have an average technical efficiency of 92.5%, indicating they can achieve 92.5% of their maximum production. A combination of farmer-specific factors could explain the differences in technical efficiency. Sesame farmers cannot achieve their planned production due to technical inefficiency and production risks. The study recommends that inputs for sesame production, such as fertilizers, should be easily accessible and inexpensive to increase technical efficiency and reduce production risk. Farmers should also be encouraged to adopt the best agronomic practices in sesame cultivation through improved support through extension services.
Reviewing editor:
1. Introduction
Sesame (Sesamum indicum L. Pedaliaceae) is an ancient oil crop of African origin (Dossa et al., Citation2017). The world’s leading sesame producers are Myanmar, India, and China, while Ethiopia is one of Africa’s largest sesame producers (Myint et al., Citation2020; Sharaby & Butovchenko, Citation2019). Sesame cultivation is mainly concentrated in the country’s lowlands, where drought and heat stress exist (Teklu et al., Citation2021). Sesame is a warm-season annual crop that prefers longer growing seasons and well-drained soils. Sesame grows well in nutrient-rich and well-drained soil, such as silty loam (Myint et al., Citation2020). Smallholders (95.5%) and medium- to large-scale commercial farmers are the main producers under rainfed conditions (Teklu et al., Citation2021). Sesame farming may be best suited to small-scale farmers because it fits their farming methods, which require a lot of human labour, and large-scale farmers have difficulty automating it properly (Saleh, Citation2020). Sesame, Ethiopia’s most important crop, contributes significantly to the country’s economy. It is the second largest source of foreign exchange earnings after coffee and millions of people rely on it for their livelihoods (Mekonnen, Citation2019).
Over the last decade, sesame production has increased in Ethiopia (Girmay, Citation2018; Hussaini et al., Citation2020; Rahman et al., Citation2020). Although the volume of sesame production is increasing over time, this does not mean that the country has reached its full production potential. Previous studies such as D. A. Ali et al. (Citation2017); Gebremichael (Citation2017); Myint et al. (Citation2020) found that the increase in sesame production is mainly due to extensive cultivation rather than intensive agricultural practices. This did not show how farmers have benefited from available technology, although increasing the volume of agricultural production, particularly high-value crops for export, remains a priority in the second growth and transformation plan. Closing the yield gap between actual and potential production is the same as catching up with the technological frontier represented by the best performers using best agricultural practices (González-Quintero et al., Citation2022; Yu et al., Citation2022). This enables farmers to increase the volume of sesame production with existing agricultural technologies, meaning they can produce more without necessarily increasing the number of production inputs (Gebremedhn et al., Citation2019).
The variability of sesame production is influenced by technical inefficiency and production risks, which prevent the producer from achieving the maximum production potential (Annette et al., Citation2017; Bhattarai & Conway, Citation2021). Technical efficiency refers to a firm’s ability to maximize output with minimal inputs (Ismail et al., Citation2014; Osamwonyi & Imafidon, Citation2016), while production risk refers to the variability of output due to input choices (Khan et al., Citation2021). Understanding the extent of inefficiency and its key determining factor could therefore help minimize the existing gap between actual and potential production. Production risk also prevents producers from getting the most from their crops, as they are affected by a variety of factors such as weeds, pests, viruses, and pathogens that can reduce yield (Elias et al., Citation2019; Fried et al., Citation2017). This type of risk can be offset by adjusting the inputs used in the production process, as they have a risk-reducing, risk-increasing, and risk-neutral effect on production. De-risking input allocation due to production risk can change the level of technical efficiency. Therefore, the production risk component needs to be considered in the stochastic frontier model to produce unbiased estimates of technical efficiency since farmers make production decisions in an inherently risky environment that influences their optimal input utilization behaviour.
When insurance and credit markets are underdeveloped or non-existent, the choice of agricultural production techniques and inputs, as well as the use of these processes and inputs, are heavily influenced by production risks (W. Ali et al., Citation2020; Mishra et al., Citation2018). Therefore, production risk is an important factor in agricultural production decisions. While agricultural inputs could increase farmers’ productivity and improve household living standards, they can also increase production risk by causing yields to fluctuate or increasing the likelihood of crop failures, or vice versa (Ogunniyi et al., Citation2017). Farmers’ decision to use agricultural inputs depends on how it affects their overall production and the level of risk to production (Vanlauwe et al., Citation2019). Thus, understanding the input relationships to output variance and examining the already existing technologies in terms of their extent of use can help farmers manage the variability in sesame production and efficiency levels.
Risks have a significant impact on input selection and output production in various production processes, especially in agricultural production. A key feature is the variability in productivity across regions as biophysical constraints impact farmers’ operations. Measuring technical efficiency and production risk provides valuable insights into the competitiveness of farms and their ability to increase crop yield given available resources and technological advances (Rambe & Khaola, Citation2022). Examining elements that influence technical efficiency provides important information for policy makers to ensure optimal use of resources. Technical efficiency refers to a firm’s ability to produce greater output with the same inputs or the same output with fewer inputs without increasing other inputs (De la Fuente-Mella et al., Citation2020; Le et al., Citation2018). It is crucial to measure the efficiency of a company compared to other companies because it defines the relationship between actual production and ideal or future production. Therefore, efficiency measurement should provide policymakers with a meaningful assessment, and the stochastic production frontier approach (SPF) is a commonly used technique in agricultural economics. This technique eliminates random errors such as weather and disease, but as an econometric estimation tool, deviations must be avoided where possible to ensure accurate results (Achen, Citation2021).
In sub-Saharan Africa, particularly Ethiopia, some studies have been conducted to quantify the technical efficiency of sesame farmers. Studies on the technical efficiency of sesame farmers in Ethiopia by Gela et al. (Citation2019); Mezgebo et al. (Citation2021); Yeshitila et al. (Citation2020); and the technical efficiency of sesame production in Nigeria by Lawal et al. (Citation2017); Bed Elgader and Mohamed (Citation2018) also estimated the production function of the sesame crop in Sudan using time-series data. All prior studies employed Aigner et al. (Citation1977) typical estimate of stochastic frontier analysis, which failed to account for production risk in the production process. In Ethiopia, most previous studies were conducted to analyse only the technical efficiency of sesame crops without considering production risks (Gela et al., Citation2019; Gemeyida et al., Citation2021; Mezgebo et al., Citation2021). Therefore, the main aim of this study is to estimate the level of technical efficiency, estimate the risk of production input given certain input parameters, and investigate the factors affecting the technical efficiency of sesame farmers. The following critical questions were derived from the problems:
How efficient is the conversion of various farm inputs to output?
What production risks exist with regard to input use?
What is the technical efficiency of sesame production?
What factors influence the technical inefficiency of sesame production?
This study contributes to the body of knowledge in various ways. Estimating the productivity of input variables to produce outputs provides insights into the relationships between different input components and outputs. It also shows the change in output when all input factors are changed in the same proportion in the long run. These estimates help policymakers select the best input mix for higher production. The production risk component provides insight into how individual inputs affect output variability, with some variables potentially increasing output variation while others may not. It also uncovers aspects that could improve efficiency and provides important insights for those involved in programmed design and implementation. Improving technical efficiency will lead to higher production, which will affect farmers’ income and living standards. The results of the study will contribute to the literature on increasing technical efficiency in the sesame producing industry and reducing risks. It will also help in understanding the impact of input utilization on production efficiency. Finally, the content of the paper is structured as follows: in the second section, the methodology of the study is presented. The results and discussion are presented in Sections 3 and 4, respectively, and the conclusion, policy implications, limitations, and suggestions for further studies are presented in Section 5.
2. Methods
2.1. Theoretical framework
Inputs can have a significant impact on average output and the variation in output or production risk (Just & Pope, Citation1978). In the conventional stochastic frontier model, an input has the same influence on the mean and variance of the output. Production variables can have a favourable impact on production but have a negative impact on production variance. For example, insecticides, irrigation and seeds could help reduce yield fluctuations caused by bad weather, insects and plant diseases, while increasing the yield of some other production methods. Climate change is affecting crop production, making it vulnerable to droughts, and water shortages (Nhemachena et al., Citation2020). Climate change is likely to cause extreme weather events, particularly droughts, to become more severe and more frequent. By the end of the 21st century, the number of drought areas worldwide is expected to increase by 15–44%, resulting in more damaged areas and greater yield losses (Wang et al., Citation2019). Therefore, irrigation not only increases the level of crop output but also increases the stability of output from year to year by mitigating the adverse effects of extreme weather conditions.
Pesticides are also crucial in agricultural production, used for weed and insect control, leading to a significant increase in agricultural products (Tudi et al., Citation2021). The existing population growth relied on food production, with about a third of agricultural products produced through pesticide use (Sharma et al., Citation2019). Pesticides are essential for reducing diseases and increasing crop yields globally, as without them, fruit, vegetable, and grain productions would suffer significantly (Tudi et al., Citation2021). Besides, farming households face significant risks in crop production that hinder investment in technologies to increase productivity and efficiency. To help small farmers overcome these challenges, improved seed (stress-tolerant) varieties have been developed. These drought-tolerant seeds increase productivity and yield stability while reducing production risks (Simtowe et al., Citation2019). They also have desirable properties such as resistance to large biotic stresses, responsiveness to inputs and good nitrogen use efficiency (Ullah et al., Citation2019). These inputs were identified as risk-mitigating parameters. These inputs were identified as risk-mitigating parameters. However, prior empirical studies by Gela et al. (Citation2019); Lawal et al. (Citation2017); Yeshitila et al. (Citation2020) did not extend this to test the effects of inputs on output variance. The production function established by Just and Pope (Citation1978) is given by EquationEquation 1(1)
(1) .
(1)
(1)
In the model, the mean output and output risk functions are expressed by and
, respectively, while the input variables are denoted by
.
are the parameters in the mean output and output risk functions that are estimated, respectively, and
are the random noise effect in the model. Löffler and Raupach (Citation2018) expand the model further to distinguish between systemic risk and idiosyncratic components of production risk, as described below in EquationEquation 2
(2)
(2) .
(2)
(2)
A vector D, which estimates the spatial effect of annual weather conditions on production variation for the whole sample, captures systemic risk. The idiosyncratic component of production risk is given by .
Given that the mean output function is and the output variance is
, the marginal production risk, which estimates how inputs influence production risk, is calculated as follows using EquationEquation 3
(3)
(3) :
(3)
(3)
The sign of and
determine the marginal risk, where the latter is the partial derivative of
with respect to input j. A positive marginal risk indicates that the input positively impacts the output risk, whereas a negative sign indicates that the input negatively influences the output risk. A risk of zero implies that the input is risk-free (Just & Pope, Citation1978). There are three forms of the model, depending on how the inefficiency effect is included. This study used a more flexible method that includes an extra function of
to describe the inefficiency effect (Kumbhakar, Citation2002), as stated below in EquationEquation 4
(4)
(4) .
(4)
(4)
The mean production function is denoted by , while the production risk function is represented by
. The vector of mean production parameters is
, while the vector of output risk parameters is
. Under certain distributional assumptions, the stochastic term,
, is assumed to be
whereas
is the non-negative inefficiency variable. The input variables
explain variations in technical efficiency, while
explain variations in technical inefficiency. An expansion of the additive model to account for the variable in technical efficiency is shown in EquationEquation 4
(4)
(4) above. Technical efficiency is described in EquationEquation 5
(5)
(5) as the ratio of observed output to frontier production, given input values and inefficiency effects (Khan et al., Citation2021).
(5)
(5)
The technical inefficiency (TI) is represented as:
(6)
(6)
In the absence of inefficiencies, there is a positive relationship between technical inefficiency and production risk and a negative relationship with average output, as shown in EquationEquation 6(6)
(6) . It makes sense to assume that the environment for agriculture is uncertain and risky, and the stochastic frontier model should take this risk into account. However, when using the standard stochastic frontier model, technical efficiency is determined entirely by one-sided random errors.
2.2. The technical inefficiency models
Understanding the differences in technical efficiency due to external variables can help policymakers determine the marginal output for a given technology and input level (Dagar et al., Citation2021). Exogenous influences could include the farmer’s age, education level, financial availability, work position, gender and other characteristics. Academics use these characteristics to explain how farmers succeed when their yields change, potentially changing the production frontier used to evaluate producer efficiency. This may affect the production frontier against which the efficiency of sesame farmers is estimated, which can be presented below:
(7)
(7)
Where
This technique has the disadvantage that, although it better portrays production possibilities, it fails to explain inefficiency effects in an acceptable production model since they are seen as uncorrelated (Carlucci et al., Citation2018). Exogenous variables are incorporated into the model differently, and they are thought to be related to inefficiency effects. This procedure is carried out in two stages. As shown in EquationEquation 8(8)
(8) , the first step estimates a stochastic frontier model with the predicted technical inefficiencies, and in the second stage (EquationEquation 9
(9)
(9) ), the exogenous variables are regressed on the inefficiency effects using ordinary least squares (OLS). However, this formulation gives a distorted portrayal of the production frontier due to the lack of external influences in the first stage. As a result, the projected technological parameters are warped, leading to inaccurate estimates of the underlying technical inefficiency. Furthermore, the second stage regression poses a significant econometric difficulty since it contradicts the premise that technical inefficiency effects are uncorrelated with social variables (Mhlanga, Citation2019).
(8)
(8)
(9)
(9)
Introducing exogenous factors into the production process shown in EquationEquation 10(10)
(10) advances previous techniques from a deterministic standpoint.
(10)
(10)
This significantly modifies the efficiency scores since it explains the inefficiency effects, solves the independence problem, and accurately portrays the nature of the production settings from which they were derived. However, a fundamental shortcoming of this technique is that it does not account for the influence of random noise in the production process, as shown in EquationEquation 9(9)
(9) . Umenberger et al. (Citation2018) used the maximum likelihood estimation method to account for the random noise effect in the model and solve the problems of variable omission and interdependence in a one-stage estimation procedure under different distribution assumptions of inefficiency effects. The non-neutral stochastic frontier model, proposed by Huang and Liu (Citation1994), allows for interaction between exogenous and conventional input variables. The model of non-neutral technical inefficiency is defined as follows;
(11)
(11)
2.3. Empirical model specification
The functional form used to estimate a stochastic production frontier with risk is critical for consistent parameter estimates. The model is given in the following translog form of the anticipated production function for the deterministic sector of the production frontier:
(12)
(12)
The observed farm production in kilograms per hectare, normalized by dividing it by the sample mean, is denoted by .
signifies the estimated technical efficiency parameters, and
represents the input variables per hectare, which are standardized by farm size and normalized by dividing them by their respective sample averages before being log-transformed. The trans-log stochastic frontier model simplifies to the Cobb-Douglas model if
as follows:
(13)
(13)
The composed error term includes the production risk and technical inefficiency functions, which are given as follows:
(14)
(14)
The estimated coefficients of the input variables are interpreted as their elasticity to output in the Cobb-Douglas model. In the trans-log model, the first-order coefficients of the input variables are seen as the output elasticity because they are divided by the sample mean before being log-transformed. The estimated scale elasticity is the percentage change in output caused by a one-percentage-point change in all input components.
When constant returns to scale (CRS),
decreasing returns to scale (DRS), and
increasing returns to scale (IRS).
For the linear production risk function, the equation is as follows:
(15)
(15)
Where denoted the input variables,
the estimated risk function parameters, and
the pure noise effects. The marginal production risks of separate inputs are denoted by
, and if it is positive, the input is risk-increasing. When
becomes negative, it indicates that the input increases output variance, and when it is zero, the input has no influence on production risk. The effects of exogenous factors on technical inefficiency are assumed to follow a left-truncated normal distribution with constant variance and are given as follows:
(16)
(16)
The computed coefficients of the technical inefficiency model are , whereas the exogenous explanatory variables are
. The unknown parameters β,
, and δ were associated with marginal products, marginal input risk, and the inefficiency impacts of EquationEquations 8–10 are estimated using the loglikelihood function (Kumbhakar & Lovell, Citation2003):
(17)
(17)
Where is the standard normal distribution. Following Battese and Coelli (Citation1988), the technical efficiency of each firm is calculated as
, and the production risk for each firm is determined by the exponential function of the variable specified in the risk function.
(18)
(18)
where
is the total output variance,
is production risk, and
is the variance of inefficiency in the case of a half-normal distribution. Inefficiency and production risk may have a considerable impact on overall observed output variability. The study uses EquationEquation 14
(14)
(14) to estimate the share of variation due to total production variance for each farm input in order to break down the contribution (Chen et al., Citation2017; Tiedemann & Latacz-Lohmann, Citation2013).
2.4. Data source and description of variables
2.4.1. Data sources
This study was conducted in western Ethiopia in the regional states of Amhara and Tigray, which accounted for the largest share of sesame production. The study was based on the data collected at the household level throughout the 2018–2019 agricultural season. The Ethiopian Central Statistics Authority collaborated with the World Bank’s Living Standards Measurement Study-Integrated Surveys on Agriculture (LSMS-ISA) team to collect the data. Sesame cultivation is an important source of foreign exchange income for the Ethiopian government. A total of 436 sesame producing households were included in the analysis.
2.4.2. Description of variables, measurement units, and hypothesized sign
The comprehensive description of the variables, measurement units and their predicted direction of effect is presented in (see Appendix A).
3. Results
3.1. Descriptive statistics of farmers’ socioeconomic characteristics
The age of the sesame farmers surveyed varied between 23 and 72 years, with the average being 47 years. The majority of sesame farmers have completed elementary school or higher. The average years of schooling for sesame farmers is 4.55 years, ranging from a minimum of no schooling to a maximum of 9 years. Only 4% of farmers had never attended school, while 70% had completed primary school. Ninety percent of farmers in this study were male and only 10% were female. The average number of extensions visits farmers received during the production year was 6.48, with a range of 2 to 12 visits per year. In tropical livestock units, the average number of livestock holdings per farmer was 2.31, with a minimum of 2 and a maximum of 12. Only 25% of farm households had access to irrigation services, while the remaining 75% had no access at all. The study also found that 64% of farmers had access to news and information through various media, compared to 36% who did not have access to media or information. 79% of farmers did not avail credit during the harvest season, while 21% had access to credit. Furthermore, 67% of sesame farmers had suffered a health shock, while the other 33% had not suffered a health shock (see ).
Table 1. Descriptive statistics of farmers’ socioeconomic characteristics.
4.2. Summary statistics of input-output variables
The study area has an average sesame yield of 630 kg/ha (). The lowest harvested sesame yield per hectare was 264 kg, while the highest produced yield was 2000 kg/ha. The average land size was 1.25 hectares, with 65% owning less, indicating that the majority of farms are smallholder. The average amount of sesame seeds that farmers use for production is 39.1 kg/ha, ranging from the smallest amount used of 6 kg/ha to the highest amount of 46 kg/ha. An average of 32.4 person-days of labour were used during the production period. This number varied between 11 and 135 person-days for the sample. The average fertilizer rate in sesame cultivation is 43.3 kg/ha, with the lowest application rate being 12 kg/ha and the highest application rate being 388 kg/ha. The lowest amount of chemicals used is nothing, and the highest amount is 25 l/ha. The average number of ox days spent harvesting sesame was 11.2 days, varying from a minimum of 3 days to a maximum of 12 days (see ).
Table 2. Summary statistics of input-output variables.
The hypothesis tests (see ) and the results of the econometric estimations are presented in the following sections. First, the mean production function is estimated using ordinary least squares (OLS) to test production risk. Based on the OLS results, the Breusch-Pagan test was also performed. At the 1% significance level, the test showed that the constant variance hypothesis was rejected (Prob > chi2 = 0.0000), showing that there is a production risk in sesame production. A maximum likelihood ratio test was then performed to test the suitability of the Cobb-Douglass function, the presence of production risks and technical inefficiency in the model, and the relevance of the technical inefficiency model. The first hypothesis test result confirms the fit of the Cobb-Douglas production function for the data (). The alternative hypothesis that the trans-log production function is appropriate for the data was rejected at a 1% significance level in favour of the Cobb-Douglas production function. The second hypothesis, which states that there is no production risk in sesame production, is rejected at a significance level of 1%. The result confirms that production risk depends on input intensity independent of climate and environmental factors and supports the functional specification of the mode. The third hypothesis, the stochastic limit model, outweighs the deterministic limit model because the null hypothesis of lambda is equal to zero (: λ=0) is rejected, which means that the calculated test statistic is much more meaningful than the critical value. This indicates that changes in observed performance at the boundary are related to technical inefficiency and random noise, but that the fluctuations due to technical inefficiency are more significant than those due to pure noise. The final hypothesis states that external factors do not explain the technical inefficiency model. This hypothesis was proven false at the 5% significance level. This suggests that sesame farmers are technically inefficient and a variety of exogenous factors contribute to this.
Table 3. Testing of hypotheses.
3.2. Estimates of the frontier
shows the parameter estimates of the Cobb-Douglas production function, understood as the output elasticity of the input variables. Land, labour, fertilizer, seeds, oxen, and chemicals have production elasticities of 0.013%, 0.236%, 0.128%, and 0.007%, respectively. All input variables have a positive and statistically significant effect on the output. Land is the most important production factor, followed by seeds with the greatest elasticity with a value of 0.236%. Chemicals used for sesame production, on the other hand, contribute the least. also shows an elasticity of scale of 0.616, indicating that returns to scale are decreasing for sesame farms in the region.
Table 4. Estimates of the Frontier.
3.3. Production risk of sesame
The estimates from the production risk function in the stochastic frontier model with flexible risk properties are shown in . The results show that fertilizers have a negative and statistically significant impact on production variability, while seeds increase production variability, although seeds are not statistically significant. Furthermore, oxen and chemical use have a positive and statistically significant influence on yield variability.
Table 5. Production risk estimates.
3.4. Distribution of technical efficiency scores
Technical efficiency estimates range from 75.7% to 100%. The average technical efficiency is 92.5%. This high average technical efficiency may be due to the fact that the study area is known and suitable for sesame cultivation and that the households included in the study are smallholder farmers with lifelong experience in agriculture. This points to the premise that small farmers are ‘poor but efficient’ (C. Ubabukoh & Imai, Citation2021; C. L. Ubabukoh & Imai, Citation2023). The distribution of technical efficiency in the study area is skewed to the right, as shown in . The majority of respondents, representing 61.7%, have technical efficiency scores between 91% and 100%. While 6.6% of sesame farmers have efficiency scores less than or equal to 80% and 31.7% of them have efficiency scores between 81% and 85% (see ).
Table 6. Distribution of technical efficiency scores.
3.5. Estimates of technical inefficiency
In , the age of the household head was added to the model to show how age affects a farmer’s technical inefficiency. The results showed that age had a significant and positive influence on technical inefficiency. The educational status variable of household heads had a negative and statistically significant influence on technical inefficiency. Access to credit also has a positive and statistically significant effect of 5% on technical inefficiency. Technical inefficiency is directly related to health shocks, and this relationship is statistically significant at the 1 percent level. Crop yields were less successful when households were headed by people with poor health. A health shock changes the amount of labour available for agriculture and the planning horizon in response to the shock, negatively affecting production efficiency. The study also confirmed that farmers who owned more livestock were less efficient than farmers who owned fewer livestock herds. Technical inefficiency is negatively related to media access and the relationship is significant at the 10% level. Farmers’ exposure to diverse media can improve their ability to understand, adapt and use productivity-enhancing inputs and technologies. Farmers who have access to the media can use the suggested sesame production practices to increase the efficiency of their farms.
Table 7. Estimates of the technical inefficiency Model.
4. Discussion
The majority of sesame farmers in the study area are elderly and have a high school education or higher. Compared to previous studies, these numbers show that most sesame farmers have received better education. This improves sesame farmers’ ability to make farm-level decisions, understand modern agricultural innovations and utilize them to achieve peak production levels; It also helps farmers rationalize input use, which can help increase output (Bati et al., Citation2017; Paltasingh & Goyari, Citation2018). The results suggest that the majority of sesame producers were men. This could be because most women are unwilling to devote even a tiny portion of their resources to sesame production because cultivation requires a lot of time and money. Extension services are essential for achieving maximum yields as they enable farmers to use the most efficient agricultural methods. This shows that farmers practiced mixed farming, predominantly in the north of the country. The proportion of irrigation users is well above the national average. However, additional efforts need to be made to increase the proportion beyond this figure - irrigation contributes to crop development, landscape management and soil greening. This increases production efficiency when sesame farmers make information accessible through various media. This finding shows that there is a low supply of formal and informal loans in the study area.
The average sesame yield of the study area is below the national average of 700 kg/ha (Tilasto, Citation2019). Labour, land, seeds, fertilizer, oxen, and chemicals are all necessary inputs to the sesame production process. The Cobb-Douglas production function was found to be suitable for the data. However, the alternative hypothesis of the trans-log production function is refuted. The hypothesis that there is no production risk in sesame production was rejected, meaning that production risk depends on input intensity in addition to climatic and environmental factors. The stochastic frontier model outweighs the deterministic limit model because the null hypothesis that lambda is equal to zero is rejected. This suggests that the deviations in actual performance from the limit are due to both technical inefficiency and random noise, rather than pure noise. Finally, the hypothesis that external factors do not contribute to technical inefficiency was also refuted. This suggests that sesame farmers are technically inefficient and that exogenous factors are causing the problems.
Both production risks and inefficiencies exist in sesame cultivation, so the study used maximum likelihood estimation to determine the production function as well as the risk and inefficiency functions. The estimation of the Cobb-Douglas model shows that the production elasticity of inputs such as land, labour, fertilizers, seeds, oxen and chemicals have a positive and statistically significant influence on production. Land is the most important factor of production, followed by seeds, which have the greatest elasticity. In contrast, the contribution of the chemicals used for production is the lowest Gemeyida et al. (Citation2021) found that seed, fertilizer and labour elasticity in sesame production are positive and statistically significant. Land is the most important input component in rice cultivation, followed by fertilizers and labour (Chandio et al., Citation2019). Sesame production is most sensitive to the seed, followed by the labour and fertilizer involved in production. Obianefo et al. (Citation2021) also found that fertilizers, seeds, and labour each contribute 0.17% to production in terms of elasticity, but are not equally important to their own production.
The elasticity of the various input variables in this study is comparable to the results of Ng’ombe (Citation2017) who estimated the elasticity using the Cobb-Douglas production function. Land area has the greatest influence on average production, while labour flexibility has the lowest. The scale elasticity of sesame production shows a decreasing rate of returns to scale. This shows that if all input variables grew by 1%, output would increase by less than 1%. In other words, the proportional increase in output is smaller than the corresponding increase in all input variables combined.
The input parameters that provide information about the output variability in the production process characterize the production risk. Some inputs minimize risk while others increase it. This is important information for stabilizing crop yields through inputs. Fertilizer is therefore a risk-reducing input in sesame production. This implies that the use of fertilizers as an input component could improve soil fertility while increasing sesame production. Farmers who are risk averse and want to reduce their risk should use more risk-reducing inputs such as fertilizers to reduce the output variance. However, seeds increase production variation, although seeds are not statistically significant. Seeds can cause yield fluctuations with low germination rates or seedlings that cannot withstand the extreme climatic conditions in the field, resulting in a loss of plant density per hectare and a decrease in yield per hectare. Adlaida et al. (Citation2019) showed that seeds represent a risk-increasing input for rice growth. Highly germinating seeds fed in a timely manner to produce live seedlings that begin healthy growth and withstand harsh field weather conditions can reduce production risk. Seeds with poor germination rates that do not receive adequate nutrients and do not develop into healthy seedlings may be more susceptible to disease. Oxen and chemical inputs increase the risk of sesame production. Akhtar et al. (Citation2021); Mehri et al. (Citation2020) found a positive relationship between chemicals and production variance. Chemicals can positively influence production variation if they can increase the occurrence of risk-causing factors in the production process. On average, sesame farmers have the opportunity to increase their production by 7.5% in the short term by adopting best agricultural practices. Thus, on average, 7.5% of potential sesame production is lost due to technical inefficiency. The best techniques of agricultural practices such as use of highly productive inputs, timely application of crop production practices including row planting technique, timely weeding, fertilizer application and harvesting, among others, can effectively contribute to achieving marginal yield. As a result, the increase in production is due to the improvement in technical efficiency rather than the use of agricultural methods, which is associated with the use of more land to increase production. This increase in production leads to an increase in income, which improves living standards. Ebukiba et al. (Citation2020) in a study on small-scale sesame production, found that the average technical efficiency was 58%, with a range of 17%–99%. In a related study in Ethiopia by Lema et al. (Citation2017), the lowest technical efficiency is 12% and the highest technical efficiency is 88%. The average technical efficiency is 53%. This implies that the sesame producers who participated in this study are relatively technically more efficient than the previous studies, but the efficiency of sesame producers may vary depending on the region. The distribution of technical efficiency in the study region is skewed to the right as the majority of respondents are found to have technical efficiency scores above 95%.
The result confirmed that as household heads get older, their technical inefficiency will increase. This means that aging can be accompanied by a loss of physical strength, leading to a reduction in efficient performance in various activities. This finding is consistent with Degefa et al. (Citation2017), who found that older household heads may become less technologically efficient. The educational level of household heads also helps to minimize the technical inefficiency of sesame farmers. This is because well-educated farmers have access to a wider range of optimal resource allocations and are therefore more likely to focus their knowledge on selecting successful sesame production practices. This means that farmers with a higher level of schooling are more efficient in sesame production. Gela et al. (Citation2019); Gemeyida et al. (Citation2021); Mezgebo et al. (Citation2021) who found that older household heads may become less technologically efficient. The educational level of household heads also helps to minimize the technical inefficiency of sesame farmers. This is because well-educated farmers have access to a wider range of optimal resource allocations and are therefore more likely to focus their knowledge on selecting successful sesame production practices. This means that farmers with a higher level of schooling are more efficient in sesame production. Mwalupaso et al. (Citation2019) also show that education plays an important role in improving technical efficiency. Credit is essential to reduce sesame farmers’ technical inefficiency, the finding confirmed. This means that farmers who receive credit in the form of cash or in-kind are more efficient than farmers who do not receive access to credit. Access to credit enables farmers to adopt the best farming and agricultural technologies. In addition, technical inefficiency is directly related to damage to health. This suggested that health shocks such as illness or death of household heads significantly increase the technical inefficiency of sesame farmers. I. Ali et al. (Citation2019) found that crop yields were less successful when households were headed by individuals with poor health. A health shock affects the amount of labour available for agriculture and the planning horizon in response to illness or death of household heads, which negatively affects production efficiency. Osei-Akoto et al. (Citation2013) also explained how idiosyncratic health shocks impact crop efficiency and result in significant production losses. The study also confirmed that farmers who owned more livestock were less efficient than farmers who owned fewer livestock herds. This could be because diverting resources to different activities could reduce the efficiency of sesame farmers. This finding is consistent with the findings of Chube (Citation2019). Additionally, farmers’ exposure to various media can improve their ability to understand, adapt, and use productivity-enhancing inputs and technologies. Farmers who have access to the media can use the suggested sesame production practices to increase the efficiency of their farms. In this context, Ebers et al. (Citation2017) showed that farmers’ access to the media improves their ability to achieve marginal yield in sesame production.
5. Conclusions and policy recommendations
5.1. Conclusions
There is a paucity of literature on production risk and efficiency within a single framework for sesame production. Production risk is an essential feature of the agricultural production process. Since production risks and risk preferences can alter the extent of technical efficiency, the study must consider the production risk component in the stochastic frontier model to produce unbiased estimates of technical efficiency. While production risk has been identified as a possible cause of sesame production fluctuations, little is known about the impact this has on yields. This article contributes to the literature by examining the production risk and technical inefficiency of sesame cultivation. We also find out what leads to fluctuations in production and how sesame cultivation can be made technically more efficient. The Cobb-Douglas production function is shown to be the best fitting model for the mean output function compared to the trans-log function. Household-specific characteristics explain technical inefficiency effects. All production inputs such as labour, land, fertilizers, oxen, and chemicals are positively related to sesame marginal production. The production practices of sesame farms exhibit diminishing returns to scale. However, there is an opportunity to increase sesame production through better utilization of inputs and technology. The results show that there are significant production risks and inefficiencies in the sesame production system. Therefore, both factors are important in explaining production variability. If the goal is to reduce production variability between farmers, measures should be taken to reduce production risk. Inputs have either risk-increasing or risk-reducing effects; By reducing the use of operating resources that have risk-increasing effects, such as: B. oxen (ploughing) and chemicals, the production risk is reduced. However, limiting these inputs leads to lower production risk but also a reduction in productivity. The elasticity of oxen and chemicals is statistically significant; Reducing these inputs will affect farmers’ productivity. An alternative strategy is to focus farmers’ attention on the input factors that both increase production and reduce risk. The results showed that increased fertilizer use reduces production risk. Fertilizers are the most important risk-reducing factor. The government can reduce the risk of sesame production by subsidizing fertilizer costs.
5.2. Policy implications
The results provide useful information for all stakeholders involved in the design and implementation of programs and policies to improve sesame production. Improving the technical efficiency of sesame production will obviously lead to an increase in production, which will ultimately have a significant impact on farmers’ income and also improve their living standards. Therefore, the following recommendations can contribute to progress in the technical efficiency of sesame production.
Farmers can increase sesame production through the use of labour, fertilizers, seeds, oxen and chemicals. Proper planting density, improved seeds and use of inorganic fertilizers can increase potential sesame yield. To increase labour productivity, establish a schedule early in the season and apply chemicals at the right time to reduce weed growth. However, in order to stabilize sesame production, compliance with scientifically recommended use levels is crucial, as this has an impact on production risk and technical efficiency.
The study highlights the need to address technical inefficiencies in sesame production to increase sesame yield. This means that policymakers should prioritize efficiency drivers such as credit and access to media and support agricultural associations and educational activities to promote the timely and effective use of productivity-enhancing inputs to increase sesame production.
5.3. Suggestion for further research
The study uses a stochastic production frontier model to examine the factors that influence yield variability and farmers’ ability to maximize sesame production, thereby enabling policy interventions to reduce the yield gap and increase productivity. The study was conducted using household-level data. Future studies should focus on plot-level analysis to examine both household- and plot-specific characteristics that influence sesame production efficiency, as well as other environmental factors in efficiency studies. Labour can also be considered disaggregated because the labour of children, women and men can have different influences on technical efficiency.
Authors contributions
All authors conceived of the original study concept and design. MA led the analysis of the collected data and prepared the first draft of the manuscript and made extensive revisions to the manuscript.
Disclosure statement
No potential conflict of interest was reported by the author(s).
Data availability statement
The dataset generated and analysed for the current study is not publicly available due to privacy and licensing regulations. However, data for this study are available from the corresponding author upon reasonable request.
Additional information
Notes on contributors
Mohammed Adem
Mohammed Adem is an assistant professor of economics at Bahir Dar University in Ethiopia. His research interests include food security, poverty, impact assessment, resource and environmental economics, production economics, and technology adoption using econometric models.
References
- Achen, C. H. (2021). The statistical analysis of quasi-experiments. University of California Press.
- Adlaida, M., Sa’Ad, M., & Saidin, N. (2019). The study of risk production in increasing of farming rice productivity at Kerinci district of Jambi province, Indonesia. Russian Journal of Agricultural and Socio-Economic Sciences, 95(11), 1–15.
- Aigner, D., Lovell, C. K., & Schmidt, P. (1977). Formulation and estimation of stochastic frontier production function models. Journal of Econometrics, 6(1), 1–15. https://doi.org/10.1016/0304-4076(77)90052-5
- Akhtar, S., Abbas, A., Iqbal, M. A., Rizwan, M., Samie, A., Faisal, M., & Sahito, J. G. M. (2021). What determines the uptake of multiple tools to mitigate agricultural risks among hybrid maize growers in Pakistan? Findings from field-level data. Agriculture, 11(7), 578. https://doi.org/10.3390/agriculture11070578
- Ali, W., Abdulai, A., & Mishra, A. K. (2020). Recent advances in the analyses of demand for agricultural insurance in developing and emerging countries. Annual Review of Resource Economics, 12(1), 411–430. https://doi.org/10.1146/annurev-resource-110119-025306
- Ali, D. A., Deininger, K., & Harris, A. (2017). Using national statistics to increase transparency of large land acquisition: Evidence from Ethiopia. World Development, 93, 62–74. https://doi.org/10.1016/j.worlddev.2016.12.027
- Ali, I., Huo, X-x., Khan, I., Ali, H., Khan, B., & Khan, S. U. (2019). Technical efficiency of hybrid maize growers: A stochastic frontier model approach. Journal of Integrative Agriculture, 18(10), 2408–2421. https://doi.org/10.1016/S2095-3119(19)62743-7
- Annette, K., Francis, M., Ijjo, A. T., & Isaac, M. B. S. (2017). An assessment of Uganda’s agricultural production, climate change, agricultural trade and food security. https://www.climdev-africa.org/sites/default/files/DocumentAttachments/WP25_%20Scoping%20study%20Uganda.pdf
- Bati, M., Mulugeta Tilahun, D., & Parabathina, R. K. (2017). Economic efficiency in maize production in Ilu Ababor zone, Ethiopia. Research Journal of Agriculture and Forestry Sciences, 2320, 6063.
- Battese, G. E., & Coelli, T. J. (1988). Prediction of firm-level technical efficiencies with a generalized frontier production function and panel data. Journal of Econometrics, 38(3), 387–399. https://doi.org/10.1016/0304-4076(88)90053-X
- Bed Elgader, A., & Mohamed, R. A. (2018). Estimation of production function of sesame in Sudan: A case study of El Gadaref State (1990-2015). University of Gezira.
- Bhattarai, K., & Conway, D. (2021). Agriculture and environment. In Contemporary environmental problems in Nepal (pp. 335–445). Springer.
- Carlucci, F., Cirà, A., & Coccorese, P. (2018). Measuring and explaining airport efficiency and sustainability: Evidence from Italy. Sustainability, 10(2), 400. https://doi.org/10.3390/su10020400
- Chandio, A. A., Jiang, Y., Gessesse, A. T., & Dunya, R. (2019). The nexus of agricultural credit, farm size and technical efficiency in Sindh, Pakistan: A stochastic production frontier approach. Journal of the Saudi Society of Agricultural Sciences, 18(3), 348–354. https://doi.org/10.1016/j.jssas.2017.11.001
- Chen, C., Restuccia, D., & Santaeulalia-Llopis, R. (2017). Land misallocation and productivity. https://www.nber.org/system/files/working_papers/w23128/w23128.pdf
- Chube, B. (2019). Analysis of production risk and technical efficiency amongst smallholder livestock farmers in Botswana. https://stud.epsilon.slu.se/14349/7/chube_b_190314.pdf
- Dagar, V., Khan, M. K., Alvarado, R., Usman, M., Zakari, A., Rehman, A., Murshed, M., & Tillaguango, B. (2021). Variations in technical efficiency of farmers with distinct land size across agro-climatic zones: Evidence from India. Journal of Cleaner Production, 315, 128109. https://doi.org/10.1016/j.jclepro.2021.128109
- De la Fuente-Mella, H., Fuentes, J. L. R., & Leiva, V. (2020). Econometric modeling of productivity and technical efficiency in the Chilean manufacturing industry. Computers & Industrial Engineering, 139, 105793. https://doi.org/10.1016/j.cie.2019.04.006
- Degefa, K., Jaleta, M., & Legesse, B. (2017). Economic efficiency of smallholder farmers in maize production in Bako Tibe district, Ethiopia. Developing Country Studies, 7(2), 80–86.
- Dossa, K., Diouf, D., Wang, L., Wei, X., Zhang, Y., Niang, M., Fonceka, D., Yu, J., Mmadi, M. A., Yehouessi, L. W., Liao, B., Zhang, X., & Cisse, N. (2017). The emerging oilseed crop Sesamum indicum enters the “Omics” era. Frontiers in Plant Science, 8, 1154. https://doi.org/10.3389/fpls.2017.01154
- Ebers, A., Nguyen, T. T., & Grote, U. (2017). Production efficiency of rice farms in Thailand and Cambodia: a comparative analysis of Ubon Ratchathani and Stung Treng provinces. Paddy and Water Environment, 15(1), 79–92. https://doi.org/10.1007/s10333-016-0530-6
- Ebukiba, E., Anthony, L., & Adamu, S. (2020). Economics and technical efficiency of maize production among small scale farmers in Abuja, Nigeria: Stochastic frontier model approach. European Journal of Agriculture and Food Sciences, 2(6), 1–9. https://doi.org/10.24018/ejfood.2020.2.6.145
- Elias, E. H., Flynn, R., Idowu, O. J., Reyes, J., Sanogo, S., Schutte, B. J., Smith, R., Steele, C., & Sutherland, C. (2019). Crop vulnerability to weather and climate risk: Analysis of interacting systems and adaptation efficacy for sustainable crop production. Sustainability, 11(23), 6619. https://doi.org/10.3390/su11236619
- Fried, G., Chauvel, B., Reynaud, P., & Sache, I. (2017). Decreases in crop production by non-native weeds, pests, and pathogens. In Impact of biological invasions on ecosystem services (pp. 83–101). Springer.
- Gebremedhn, M. B., Tessema, W., Gebre, G. G., Mawcha, K. T., & Assefa, M. K. (2019). Value chain analysis of sesame (Sesamum indicum L.) in Humera district, Tigray, Ethiopia. Cogent Food & Agriculture, 5(1), 1705741. https://doi.org/10.1080/23311932.2019.1705741
- Gebremichael, D. E. (2017). Sesame (Sesamum indicum L.) breeding in Ethiopia. International Journal of Novel Research in Life Sciences, 4, 1–11.
- Gela, A., Haji, J., Ketema, M., & Abate, H. (2019). Technical, allocative and economic efficiencies of small-scale sesame farmers: The case of West Gondar Zone, Ethiopia. Review of Agricultural and Applied Economics, 22(2), 10–17. https://doi.org/10.15414/raae.2019.22.02.10-17(1340-2019-3711)
- Gemeyida, K. H., Yesho, E. G., & Belaneh, A. W. (2021). Production efficiency of sesame producer farm households: The case of Bench Maji Zone, Southwest Ethiopia. Acta Scientiarum Polonorum Administratio Locorum, 20(3), 189–201. https://doi.org/10.31648/aspal.6248
- Girmay, A. B. (2018). Sesame production, challenges and opportunities in Ethiopia. Agricultural Research & Technology: Open Access Journal, 15(5), 555972.
- González-Quintero, R., van Wijk, M. T., Ruden, A., Gómez, M., Pantevez, H., Castro-Llanos, F., Notenbaert, A., & Arango, J. (2022). Yield gap analysis to identify attainable milk and meat productivities and the potential for greenhouse gas emissions mitigation in cattle systems of Colombia. Agricultural Systems, 195, 103303. https://doi.org/10.1016/j.agsy.2021.103303
- Huang, C. J., & Liu, J.-T. (1994). Estimation of a non-neutral stochastic frontier production function. Journal of Productivity Analysis, 5(2), 171–180. https://doi.org/10.1007/BF01073853
- Hussaini, A., Usman, M., Falgore, J., Sani, S., Zakari, Y., & Muktar, H. (2020). A search for suitable growing environment for sesame production in Nigeria. Asian Journal of Agricultural Extension, Economics & Sociology, 8(8), 18–33. https://doi.org/10.9734/ajaees/2020/v38i830383
- Ismail, R., Noor, Z. M., & Abidin, S. Z. (2014). Determinant of technical efficiency of small and medium enterprises in Malaysian manufacturing firms. International Business Management, 11(2), 299–307.
- Just, R. E., & Pope, R. D. (1978). Stochastic specification of production functions and economic implications. Journal of Econometrics, 7(1), 67–86. https://doi.org/10.1016/0304-4076(78)90006-4
- Khan, M. A., Begum, R., Nielsen, R., & Hoff, A. (2021). Production risk, technical efficiency, and input use nexus: Lessons from Bangladesh aquaculture. Journal of the World Aquaculture Society, 52(1), 57–72. https://doi.org/10.1111/jwas.12767
- Kumbhakar, S. C. (2002). Specification and estimation of production risk, risk preferences and technical efficiency. American Journal of Agricultural Economics, 84(1), 8–22. https://doi.org/10.1111/1467-8276.00239
- Kumbhakar, S. C., & Lovell, C. K. (2003). Stochastic frontier analysis. Cambridge university press.
- Lawal, A., Adedeji, S., Bwala, M., Umaru, A., & Momoh, J. (2017). Technical efficiency of sesame production in Niger State. Nigerian Association of Agricultural Economists.
- Le, V., Vu, X.-B B., & Nghiem, S. (2018). Technical efficiency of small and medium manufacturing firms in Vietnam: A stochastic meta-frontier analysis. Economic Analysis and Policy, 59, 84–91. https://doi.org/10.1016/j.eap.2018.03.001
- Lema, T. Z., Tessema, S. A., & Abebe, F. A. (2017). Analysis of the technical efficiency of rice production in Fogera district of Ethiopia: A stochastic frontier approach. Ethiopian Journal of Economics, 26(2), 88–108.
- Löffler, G., & Raupach, P. (2018). Pitfalls in the use of systemic risk measures. Journal of Financial and Quantitative Analysis, 53(1), 269–298. https://doi.org/10.1017/S0022109017001041
- Mehri, M., Eshraghi, F., & Keramatzadeh, A. (2020). An analysis of the determinants of wheat production risk in Gorgan County. Journal of Agricultural Science and Technology, 22(5), 1153–1164.
- Mezgebo, G. K., Mekonen, D. G., & Gebrezgiabher, K. T. (2021). Do smallholder farmers ensure resource use efficiency in developing countries? Technical efficiency of sesame production in Western Tigrai, Ethiopia. Heliyon, 7(6), e07315. https://doi.org/10.1016/j.heliyon.2021.e07315
- Mekonnen, E. (2019). Assessing the core challenges on the process of exporting quality sesame seeds in Ethiopia. St. Mary’s University.
- Mhlanga, O. (2019). Factors impacting airline efficiency in Southern Africa: A data envelopment analysis. GeoJournal, 84(3), 759–770. https://doi.org/10.1007/s10708-018-9889-9
- Mishra, A. K., Kumar, A., Joshi, P. K., & D’souza, A. (2018). Production risks, risk preference and contract farming: Impact on food security in India. Applied Economic Perspectives and Policy, 40(3), 353–378. https://doi.org/10.1093/aepp/ppy017
- Mwalupaso, G. E., Wang, S., Rahman, S., Alavo, E. J.-P., & Tian, X. (2019). Agricultural informatization and technical efficiency in maize production in Zambia. Sustainability, 11(8), 2451. https://doi.org/10.3390/su11082451
- Myint, D., Gilani, S. A., Kawase, M., & Watanabe, K. N. (2020). Sustainable sesame (Sesamum indicum L.) production through improved technology: An overview of production, challenges, and opportunities in Myanmar. Sustainability, 12(9), 3515. https://doi.org/10.3390/su12093515
- Ng’ombe, J. N. (2017). Technical efficiency of smallholder maize production in Zambia: A stochastic meta-frontier approach. Agrekon, 56(4), 347–365. https://doi.org/10.1080/03031853.2017.1409127
- Nhemachena, C., Nhamo, L., Matchaya, G., Nhemachena, C. R., Muchara, B., Karuaihe, S. T., & Mpandeli, S. (2020). Climate change impacts on water and agriculture sectors in Southern Africa: Threats and opportunities for sustainable development. Water, 12(10), 2673. https://doi.org/10.3390/w12102673
- Obianefo, C. A., Ng’ombe, J. N., Mzyece, A., Masasi, B., Obiekwe, N. J., & Anumudu, O. O. (2021). Technical efficiency and technological gaps of rice production in Anambra State, Nigeria. Agriculture, 11(12), 1240. https://doi.org/10.3390/agriculture11121240
- Ogunniyi, A., Oluseyi, O. K., Adeyemi, O., Kabir, S. K., & Philips, F. (2017). Scaling up agricultural innovation for inclusive livelihood and productivity outcomes in sub-Saharan Africa: The case of Nigeria. African Development Review, 29(S2), 121–134. https://doi.org/10.1111/1467-8268.12267
- Osamwonyi, I. O., & Imafidon, K. (2016). The technical efficiency of manufacturing companies on the Nigerian Stock Exchange. Journal of Applied Finance and Banking, 6(1), 127.
- Osei-Akoto, I., Adamba, C., & Osei, R. (2013). The effect of health shocks on agricultural productivity: Evidence from Ghana. International Journal of Agricultural Policy and Research, 1(3), 067–079.
- Paltasingh, K. R., & Goyari, P. (2018). Impact of farmer education on farm productivity under varying technologies: case of paddy growers in India. Agricultural and Food Economics, 6(1), 1–19. https://doi.org/10.1186/s40100-018-0101-9
- Rahman, A., Bhattarai, S., Akbar, D., Thomson, M., Trotter, T., Timilsina, S., & Australia, C. (2020). Market analysis of sesame seed. https://crcna.com.au/resources/publications/market-analysis-sesame-seed
- Rambe, P., & Khaola, P. (2022). The impact of innovation on agribusiness competitiveness: The mediating role of technology transfer and productivity. European Journal of Innovation Management, 25(3), 741–773. https://doi.org/10.1108/EJIM-05-2020-0180
- Saleh, N. I. (2020). Impact of automation on sesame seed production in Nigeria. National College of Ireland.
- Sharaby, N., & Butovchenko, A. (2019 Cultivation technology of sesame seeds and its production in the world and in Egypt [Paper presentation]. Paper Presented at the IOP Conference Series: Earth and Environmental Science. https://doi.org/10.1088/1755-1315/403/1/012093
- Sharma, A., Kumar, V., Shahzad, B., Tanveer, M., Sidhu, G. P. S., Handa, N., Kohli, S. K., Yadav, P., Bali, A. S., Parihar, R. D., Dar, O. I., Singh, K., Jasrotia, S., Bakshi, P., Ramakrishnan, M., Kumar, S., Bhardwaj, R., & Thukral, A. K. (2019). Worldwide pesticide usage and its impacts on ecosystem. SN Applied Sciences, 1(11), 1–16. https://doi.org/10.1007/s42452-019-1485-1
- Simtowe, F., Amondo, E., Marenya, P., Rahut, D., Sonder, K., & Erenstein, O. (2019). Impacts of drought-tolerant maize varieties on productivity, risk, and resource use: Evidence from Uganda. Land Use Policy, 88, 104091. https://doi.org/10.1016/j.landusepol.2019.104091
- Teklu, D. H., Shimelis, H., Tesfaye, A., & Abady, S. (2021). Appraisal of the sesame production opportunities and constraints, and farmer-preferred varieties and traits, in Eastern and Southwestern Ethiopia. Sustainability, 13(20), 11202. https://doi.org/10.3390/su132011202
- Tiedemann, T., & Latacz-Lohmann, U. (2013). Production risk and technical efficiency in organic and conventional agriculture–The case of arable farms in Germany. Journal of Agricultural Economics, 64(1), 73–96. https://doi.org/10.1111/j.1477-9552.2012.00364.x
- Tilasto. (2019). Statistics, data by country, market data and more. Tilasto.
- Tudi, M., Daniel Ruan, H., Wang, L., Lyu, J., Sadler, R., Connell, D., Chu, C., & Phung, D. T. (2021). Agriculture development, pesticide application and its impact on the environment. International Journal of Environmental Research and Public Health, 18(3), 1112. https://doi.org/10.3390/ijerph18031112
- Ubabukoh, C., & Imai, K. S. (2021). Are farmers "efficient but poor"?: The impact of crop choices on agricultural productivity and poverty in Nigeria. Research Institute for Economics and Business Administration, Kobe University.
- Ubabukoh, C. L., & Imai, K. S. (2023). Are farmers ‘efficient but poor’? The impact of crop choices on technical efficiency and poverty in Nigeria. Journal of Agricultural Economics, 74(1), 191–213. https://doi.org/10.1111/1477-9552.12501
- Ullah, H., Santiago-Arenas, R., Ferdous, Z., Attia, A., & Datta, A. (2019). Improving water use efficiency, nitrogen use efficiency, and radiation use efficiency in field crops under drought stress: A review. Advances in Agronomy, 156, 109–157.
- Umenberger, J., Wågberg, J., Manchester, I. R., & Schön, T. B. (2018). Maximum likelihood identification of stable linear dynamical systems. Automatica, 96, 280–292. https://doi.org/10.1016/j.automatica.2018.06.036
- Vanlauwe, B., Coe, R., & Giller, K. E. (2019). Beyond averages: New approaches to understand heterogeneity and risk of technology success or failure in smallholder farming. Experimental Agriculture, 55(S1), 84–106. https://doi.org/10.1017/S0014479716000193
- Wang, J., Yang, Y., Huang, J., & Adhikari, B. (2019). Adaptive irrigation measures in response to extreme weather events: Empirical evidence from the North China plain. Regional Environmental Change, 19(4), 1009–1022. https://doi.org/10.1007/s10113-018-1442-3
- Yeshitila, M., Bunyasiri, I., Sirisupluxana, P., & Suebpongsakorn, A. (2020). The impact of contract farming on technical efficiency in Ethiopia’s smallholder sesame production. Journal of the Austrian Society of Agricultural Economics, 16(4), 57–66.
- Yu, D., Hu, S., Tong, L., Xia, C., & Ran, P. (2022). Dynamics and determinants of the grain yield gap in major grain-producing areas: A case study in Hunan Province, China. Foods, 11(8), 1122. https://doi.org/10.3390/foods11081122
Appendix A
Table A1. Description, measurement, and expected sign of explanatory variables.