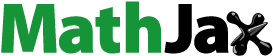
Abstract
Evaluation of maize varieties under multiple environments, including drought and low nitrogen (N) stressed sites is an important breeding approach, to identify well adapted and stable maize varieties. This study was undertaken to identify new quality protein maize (QPM) hybrids that have good agronomic performance and assess the presence of genotype by environment (G × E) interaction and grain yield stability of QPM hybrids under different environment conditions. Forty-five hybrids, including two QPM, two non-QPM and one local check were evaluated across 34 environments under stress and non-stress conditions in Ethiopia, Zimbabwe, Zambia, Mozambique, and Malawi during 2018 to 2020. Additive Main Effects and Multiplicative Interaction (AMMI) and Genotype main effects plus G × E interaction (GGE) bi-plots were used for stability analysis. Environment, genotype and G × E interaction effects were significant for grain yield and other traits in all management conditions. The top yielding hybrids were 44 (QS7646) 12 (CZH15099Q) under optimum; 14 (CZH15142Q), 44 (QS7646) and 23 (CZH17192Q) under random stress; 9 (CZH142237Q) and 10 (CZH142238Q) under managed drought; and 14 (CZH15142Q) and 34 (CZH17203Q) under low N conditions. Among these, 10 (CZH142238Q) and 14 (CZH15142Q) were the most stable hybrids and can be recommended for release in sub-Saharan Africa to improve food and nutritional security of smallholder farmers who depend on maize. Kwekwe (KWE), Bindura (BIN), Chokwe (CHO) and Bako (BK2) were identified as the most discriminating and representative for optimum, random stress, managed drought and low N environments, respectively and help to identify superior hybrids.
REVIEWING EDITOR:
1. Introduction
Malnutrition is a persistent problem in Africa, especially in rural areas where maize is used as the main source of carbohydrates and protein (Tandzi et al., Citation2017; Vivek et al., Citation2008). Biofortified crops like quality protein maize (QPM) have significant nutritional benefits in combating malnutrition if it is produced and consumed in sufficient quantities (Krivanek et al., Citation2007; Tandzi et al., Citation2017). It can provide a more balanced protein for human and other monogastric animals. The quality and biological value of its protein are much higher than that of conventional maize. For example, the biological value of common maize protein is equal to about 40% of the biological value of milk protein, whereas the biological value of QPM protein is about 90% of that of milk protein (Nuss & Tanumihardjo, Citation2011). Thus, the nutritional benefits of QPM approach those of milk protein, a common standard of nutritional excellence.
Nowadays, some QPM varieties have comparable productivity potential compared with normal maize (Abakemal et al., Citation2016; Mebratu et al., Citation2019; Setimela et al., Citation2017; Wegary et al., Citation2014). It is also difficult to distinguish them from normal maize using morphological characteristics such as ear height, silk and flower characteristics and kernel texture. However, normal maize generally yields more, though in some situations the difference with QPM genotypes can be marginal (Tandzi et al., Citation2017). Determination of lysine and tryptophan content of the grain is necessary to confirm whether the genotype is QPM or not (Teklewold et al., Citation2015).
Maize production and productivity in sub-Saharan Africa (SSA) are affected by biotic and abiotic factors such as drought, low soil fertility, high pressure of weeds, insect pests, diseases, low input availability (especially quality seed and fertilizer), high cost of agricultural inputs and limited use of inputs (Meseka et al., Citation2013; Shiferaw et al., Citation2011). Among these factors, drought and low N levels in the soil are major challenges in smallholder farmers’ fields (Ertiro et al., Citation2017; Mebratu et al., Citation2019; Wegary et al., Citation2014). Combined effects of these stress factors can be devastating (Badu-Apraku et al., Citation2016). Drought affects maize at all stages of development, but the flowering and grain-filling periods are the most sensitive with yield losses ranging from 10–50% (Fisher et al., Citation2015). Nitrogen deficiency is also common in farmers’ fields in the tropics where fertilizer application is far below the optimal rate, or not used at all (Meseka et al., Citation2013). Under such conditions, it is important to develop maize varieties that are tolerant to abiotic stress conditions which is a more reliable and affordable option for poor farmers in marginal areas (Beyene et al., Citation2015; Ertiro et al., Citation2017).
Evaluation of genotypes in multi-environments can help to identify well adapted and stable genotypes before commercial release (Abakemal et al., Citation2016; Makumbi et al., Citation2015; Rezende et al., Citation2020). Hybrids that are superior in one environment during a season/year may not be superior in other environments in the same season or year, due to the existence of G × E interaction (Makumbi et al., Citation2015; Sserumaga et al., Citation2016, Citation2018). This makes it difficult to allocate a variety that is successfully adapted to a certain location or across locations (Mebratu et al., Citation2019; Wolde et al., Citation2018). As a result, measuring G × E interaction is important in optimizing breeding strategies for selecting cultivars that are well adapted to specific environments (Abakemal et al., Citation2016; Matongera et al., Citation2023). So far, several studies have been conducted to measure G × E interaction and stability of both conventional (non-QPM) and QPM germplasms under stress and non-stress conditions. However, compared to the conventional maize germplasm, limited information has been generated on G × E interaction and stability of QPM germplasms under managed drought and low N conditions. In addition, some studies also indicated that the impact of drought and low N on agronomic and nutritional traits of QPM germplasms is high (Ngaboyison et al., Citation2012; Wegary et al., Citation2011). Thus, information on G × E interaction and stability of QPM genotypes across different management conditions would provide a scientific basis to develop future breeding strategies that target the development of QPM germplasm for release and marketing across different countries in SSA (Mebratu et al., Citation2019). Several statistical methods have been developed and deployed to measure G × E interaction and stability. Additive Main Effects and Multiplicative Interaction (AMMI) (Zobel et al., Citation1988) and genotype plus G × E interaction (GGE) biplot (Yan & Kang, Citation2003; Yan & Tinker, Citation2006) analysis are the most commonly and widely used statistical models. AMMI model separates the additive effects for genotype and environment from the multiplicative effects for G × E interaction by applying principal component analysis (PCA) (Pacheco et al., Citation2015). GGE biplot is a linear-bilinear model that removes the environmental main effect and considers the genotype plus the G × E interaction. This model is powerful in depicting “which won where” pattern and easily identify stable and ideal hybrids as well as environments with representativeness and discriminating ability of the environments (Matongera et al., Citation2023).
CIMMYT in collaboration with the National Agricultural Research Systems (NARS), have been screening a number of QPM and non-QPM genotypes under drought and low N environmental conditions for a number of years (Ertiro et al., Citation2017; Mebratu et al., Citation2019; Sserumaga et al., Citation2016, Citation2018; Wegary et al., Citation2013, Citation2014). As a result, several stress tolerant maize varieties have been released and disseminated for commercial production in partnership with various public and private organizations in SSA (Abate et al., Citation2017; Fisher et al., Citation2015; Teklewold et al., Citation2015). Compared to the conventional maize varieties, however, a very limited number of drought and low N tolerant QPM varieties have been released and commercialized. Therefore, the objectives of this study were to (1) identify new QPM hybrids that have good grain yield and agronomic performance under optimum, random stress, managed drought and low N conditions and (2) explore G × E interaction and grain yield stability of experimental QPM hybrids across different management conditions to promote release for commercialize in eastern and southern Africa (ESA).
2. Materials and methods
2.1. Genetic materials and testing sites
Forty newly developed QPM hybrids were obtained from CIMMYT-Zimbabwe and partner seed companies for this study. These hybrids were selected based on grain yield, disease resistance and other agronomic trait from early-stage trials for further regional trial evaluation under different environmental conditions. Five commercial checks (two QPM, two normal maize and one locally developed QPM or normal check), were included in the trials (). The aim of including the best local check at each location within CIMMYT trials was to compare the performance of the CIMMYT hybrids with local check. In combined analysis, the local check was excluded from the analysis because different local checks were used at different locations. The experimental materials were evaluated across 34 environments in 14 optimum, 9 random stress, 3 managed drought and 8 low N environments in five countries: Ethiopia, Zimbabwe, Zambia, Mozambique, and Malawi during 2018 to 2020. The descriptions of these test sites are given in .
Table 1. Genotype name, seed source and protein quality traits of 45 hybrids used in this study.
Table 2. Description of the study sites, mean grain yield and CV of the trials evaluated during 2018–2020.
2.2. Experimental design and trial management conditions
The trials were conducted using a 5 × 9 alpha lattice design with two replications. At all sites, each genotype was planted in two rows per plot of 5 m row length with spacing of 0.75 m between rows and 0.25 m between plants except at Bako in 2018 where both optimum and low N sites were planted at 4 m row length and 0.3 m spacing between plants and one row per plot. Due to shortage of land, Ambo (optimum site) in 2020 was planted at 5 m row length and 0.25 m spacing between plants and one row per plot. Two seeds were planted per hole and then thinned to one plant at three weeks after emergence, to obtain a final plant population density 53,333 plants per hectare. Fertilizer was applied based on recommendations for each location. For low N trials, all recommended rates of phosphorous, potassium and sulfur fertilizer were applied at planting, without further top dressing of urea. For optimum, random stress and managed drought trials, phosphorus, potassium and sulfur fertilizer sources were applied at planting, followed by a top dressing of urea fertilizer three weeks after planting. In Ethiopia, fertilizer was applied at a rate of 92 kg N and 69 kg diammonium phosphate (P2O5) ha−1. Nitrogen was applied twice, at knee height and anthesis stage. All other agronomic practices such as weeding and pest management were done as needed. Optimum, random stress and low N trials were grown under rainfed conditions. However, random stress trials experienced random drought conditions where experimental sites normally received less than 600 mm rainfall per year (Setimela et al., Citation2017). Low N trials were conducted on N depleted sites. Nitrogen depletion was done by growing maize on it for more than 5 year and removing all crop stover from the field after harvest (Bänziger et al., Citation2006). Managed drought stress trials were also conducted during the dry/winter season with water applied entirely by irrigation. The trials were exposed to moisture deficiency by withholding irrigation supply 2 weeks before flowering up to 3 weeks post flowering so that drought stress would coincide with flowering and grain filling, which is the most vulnerable stages (Magorokosho et al., Citation2004). The aims of the managed drought and low N experiments were to had an average yield reduction of 25–40% compared to yields under optimal agronomic management conditions (Setimela et al., Citation2017). These environmental conditions simulate stresses that occurs in smallholder farmer’s fields where the grain yields are between 1 and 2 t ha−1 (Magorokosho et al., Citation2010; Setimela et al., Citation2017). At harvesting, the first and the last plant of each row of low N and managed drought stress trials were discarded to minimize border effects.
2.3. Data collection
Grain yield and other agronomic data were collected from all sites for all management conditions. Grain yield was recorded in kilograms at harvesting by weighing the total ears per plot. This was adjusted to 12.5% moisture content to estimate grain yield expressed in ton per ha. The number of days to anthesis was recorded from planting date to when 50% of the plants in a plot started pollen shedding. The number of days to silking were recorded from planting date to when 50% of the plants in the plot produced 2–3 cm long silks. Anthesis-silking interval (ASI) was calculated as the difference between the number of days to silking and days to anthesis. The mean plant and ear height was measured in centimetre from five random plants in a plot, plant height was measured from the ground level to the first tassel branch, and ear height was measured from the ground to the top useful ear insertion base of the node. Ear position was calculated as a ratio of the ear height to plant height. At harvesting, the total number of harvested plants and ears were counted in all plots at each site. Number of ears per plant was calculated as the ratio of total ears in a plot to total number of plants harvested in a plot.
2.4. Data analysis
Individual and combined analysis of variance (ANOVA) was done for the collected data using PROC MIXED procedure of SAS® software (SAS, Citation2002). The hybrids were considered as a fixed factor while environments, replications within environments and the incomplete blocks within replications were considered random factors. Levene’s test was used to test the homogeneity of variances before analyzing the combined data (Gastwirth et al., Citation2009). In the combined environment analysis of ANOVA, genotype effects were tested for significance using the corresponding interaction with the environment as the error term, whereas the genotype × environment interaction was tested against the pooled error. Mean comparison among hybrid means was done by the least significant difference (LSD) test at 5% level of significance (Gomez & Gomez, Citation1984). After confirming the presence of significant G × E interaction in combined analysis for grain yield, further partitioning of the G × E interaction and stability analyses was conducted using AMMI and GGE biplot models. Data were combined across optimum, random stress, managed drought, low N stress and across all environments.
The combined ANOVA linear mixed model was followed:
Where, Yijk is the observed mean of the ith genotype (Gi) in the environment (Ej), in the kth block (Bk), µ is the overall mean, Gi is the effect of ith genotype, Ej is the effect of jth environment, Rk is replication effect of the kth replication in the jth environment, Bo [Rk(Ej)] is the random effect of incomplete block Bo within replicate kth and environment jth, GEij is the interaction effect of the ith genotype as well as jth environment and Ɛijko is the error term.
Broad sense heritability (H2) for a given trait across environments was estimated using the variance components (Alvarado et al., Citation2020). H2 = where σ2g is genotype variance, σ2ge is genotype by environment interaction variance, and σ2e is error variance for r replicates and E environments of the genotypes at each testing site under optimum, random stress, manged drought and low N conditions.
The AMMI analysis was performed by using the raw data of a lattice design with GEA-R (Genotype by Environment Analysis with R) version 4.1 software (Pacheco et al., Citation2015). AMMI partitions the sum of squares into interaction principal component (IPC) axes.
The basic model is:
Where yij is the yield of the ith genotype (i = 1,…,I) in the jth environment (j = 1,…,J); µ is the grand mean; gi and ej are the genotype and environment deviations from the grand mean, respectively; τn is the eigenvalue of the PC analysis axis n; γin and δjn are the genotype and environment principal components scores for axis n; N is the number of principal components retained in the model and "ij is the error term.
GGE biplot analysis was used for visualising patterns and interactions without environmental effects (Matongera et al., Citation2023). The GGE biplot graph was generated using singular value decomposition (SVD) of the first two principal component axis derived from environment-centered data (axis1 and axis2) using the Site Regression (SREG) model (Mekonnen et al., Citation2022; Yan & Kang, Citation2003). The first principal component (PC1) represented the variation due to genotype while the second principal component (PC1) represents the variation in the yield due to the G × E interaction (Yan, Citation2011).
According to Purchase et al., (Citation2000), AMMI stability values (ASV) for grain yield stability analysis were calculated.
ASV = ASV = AMMI stability value; SS = sum of squares; IPCA1 and IPCA2= the first and the second interaction principal component axes, respectively
3. Results
3.1. Analysis of variance and hybrid performance
Combined analysis for each management condition showed significant difference among genotypes (G), environments (E), and G × E interaction for grain yield and almost all recorded agronomic traits (). High grain yield heritability values were observed for the combined environments (0.81), followed by random stress (0.69), optimum management (0.64), and low N stress conditions (0.61) while the lowest heritability was recorded under managed drought stress (0.08) (). All other traits showed high heritability values in combined environments analysis. Mean grain yield ranged from 7.3 t ha−1 to 8.9 t ha−1 with a mean of 8.3 t ha−1 across optimum environments, 3.2 to 5.3 t ha−1, with an overall mean of 4.3 t ha−1 under random drought stress, 1.8 t ha−1 to 3.6 t ha−1 with a mean of 2.7 t ha−1 under managed drought conditions, and 1.9 to 3.2 t ha−1 with an average of 2.4 t ha−1 under low N stress conditions (). Some QPM hybrids recorded better grain yield and agronomic performance than other QPM and normal maize checks under optimum, drought and low N stress conditions. The mean of top ten QPM hybrids under optimum, random stress, managed drought and low N stress conditions showed 15.8 and 6.4%, 32.0 and 13.3%, 20.1 and 27.6%, and 11.4 and 13.2% yield advantage compared to the mean of other QPM and normal maize checks, respectively. The first highest yielding QPM hybrid under each management condition also showed 13.7%, 30.3%, 29.3% and 15.4% grain yield advantage over the best QPM check under optimum, random drought stress, managed drought and low N stress conditions, respectively. Entries, 44 and 12 under optimum, 14, 44 and 23 under random drought, 9 and 10 under managed drought, and 14 and 34 were the first two top yielding hybrids.
Table 3. Mean squares for grain yield and agronomic traits for 40 QPM hybrids and four commercial checks evaluated under different environmental conditions in 2018–2020.
Table 4. Grain yield and some agronomic traits of top ten QPM hybrids and commercial checks evaluated under different environmental conditions in ESA during 2018 to 2020.
In combined environments, grain yield ranged from 4.6 t ha−1 for commercial QPM check (ZS261) to 6.0 t ha−1 for entry 14 (CZH15142Q) with an overall mean of 5.4 t ha1 (). The mean grain yield of the top ten QPM hybrids was 5.7 t ha−1 and showed 17.0% and 8.0% yield advantage over the mean of other QPM and normal maize checks, respectively. Among the top ten hybrids, the top yielding hybrid, entry 14, showed a 15.7% yield advantage over the best QPM check and comparable yield performance with the normal maize check. Moreover, this entry showed a 38.6 and 27.1% lower ASI and 12.3 and 22.9% higher number of ears per plant, compared to the best QPM and normal maize checks, respectively, and recorded comparable anthesis and ear position with the mean of the best QPM and normal maize commercial checks. All top yielding hybrids showed mid ear placement, which can prevent lodging.
Compared to optimum environments, mean of grain yield was reduced by 48% under random drought stress, 68% under managed drought and 71% under low N conditions (). Days to anthesis and silking were also decreased by 7 and 6% under random stress, and 11 and 8% under managed drought stress conditions, and increased by 13% and 16% under low N conditions, respectively. ASI was increased by 80%, 218% and 203% under random stress, managed drought and low N compared to optimum conditions, respectively. Plant and ear height were reduced by 20% and 25% under random stress, 30% and 29% under managed drought and 23% and 27% under low N environments, respectively. Ear position was also reduced by 7% under random stress and 2% under managed drought and 6% under low N conditions. Number of ears per plant was reduced by 11% under random drought stress, 24% under managed drought and 20% under low N environments.
Table 5. Percentage of grain yield and agronomic performance reduction under random stress, managed drought and low N stress conditions compared to the performance under optimum management condition.
3.2. AMMI analysis
Mean squares from the AMMI analysis showed highly significant difference among genotypes (G), environments (E) and G × E interaction for grain yield under optimum, random stress, managed drought, and low N conditions (). The proportion of total variations explained by G, E and G × E were 10, 36 and 54% under optimum, 8, 70 and 22% under low N conditions, 11, 54 and 35% under random stress and 28, 20 and 52% under managed stress, respectively. The G × E interaction source of variation was further partitioned into interaction principal components (IPCA). Under optimum conditions, G × E interaction was partitioned into eight significant IPCAs, which explained 86.1% of the total G × E interaction variation. Under random stress, G × E interaction was partitioned into four significant IPCAs. These IPCAs explained a total of 76.9% of the G × E interaction variation. Under managed drought, G × E was partitioned into two highly significant IPCAs, which explained 100% of the variation. Under low N conditions, G × E interaction was partitioned into six significant IPCAs, which explained 97.1% of the variation. The first two IPCAs in each management type explained 38.5%, 60.3%, 51.9% and 100% of variation under optimum, low N, random stress and managed drought, respectively.
Table 6. AMMI analysis of variance for grain yield of 40 QPM hybrids and four commercial checks evaluated under optimum and stressed environments in ESA during 2018 to 2020.
Based on IPCA1 scores, hybrids such as 6, 10, 14, 20 and 24 under optimum, 3, 8, 9, 23 and 44 under random stress, 7, 19, 35 and 40 under managed drought and 3, 11 and 14 under low N had relatively close to zero IPCA1 scores and high mean grain yield. This suggested that these hybrids were relatively stable and high yielding in the respective environmental conditions (). On the other hand, hybrids 3, 12,23, 44, 11, 15, 17, 37 and 41 under optimum, 10, 14, 18, 34 and 38 under random stress, 8, 10, 15, 23, 3 (check), 9, 14 and 22 under managed drought, 7, 10, 27 and 34 under low N had high positive and negative IPCA1 values. This indicated that these hybrids were therefore unstable and adapted to specific environments under the respective environment conditions. Hybrids such as 1 (check) and 31 under optimum and 23, 30, 32, 33 and 36 under low N had low mean grain yield and close to zero IPCA1 values, indicating consistent, but poor yield performance across optimum and low N environments, respectively.
Table 7. Mean grain yield and IPCA scores and ASV values of 40 QPM hybrids and four commercial checks evaluated under stress and non-stress environments.
AMMI stability values showed variation in grain yield stability among the 44 hybrids under different management conditions (). Hybrids with ASV values closer to zero are considered more stable than hybrids with higher positive or negative ASV values (Purchase et al., Citation2000). Across optimum conditions, hybrids 1 (ZS261) check, 6, 20, 24, 35, 14 and 28 had the lowest ASV values and are considered as more stable. Among these hybrids, 6, 14, 20 and 24 showed above overall trial mean grain yield while hybrids 1 and 35 showed the lowest mean yield. Across random stress trials, hybrids 37, 12, 8 and 23 had relatively lower ASV values and high mean grain yield and these hybrids were considered more stable and widely adaptable across random stress environments. On the other hand, hybrids 3 (check), 9 and 44 were top yielding, but with the high ASV values. These hybrids were considered unstable but adapted to specific environments under random stress conditions.
Hybrids 16, 26, 40, 33, 7, 6 and 24 showed the lowest ASV values compared to the remaining hybrids, indicating these hybrids were relatively more stable under managed drought environments. Hybrid 40 was also both stable and a widely adaptable hybrid under managed drought environments. Hybrids 9 and 10 exhibited the highest ASV values, but were top yielding which indicates that these hybrids were unstable, but could adapt to specific environments under managed drought environments. Across low N environments, hybrids 30, 42, 39, 32 and 37 showed relatively low ASV values and mean yield below the average mean of the trial whereas hybrids 3, 14, 11 and 34 had relatively high ASV values and showed high mean grain yield. This indicates that these hybrids were adapted to specific testing environments under low N stress conditions.
3.3. GGE biplot analysis
3.3.1. Which won where pattern
GGE biplot analysis of grain yield for 40 QPM hybrids and four checks are presented in . The first two principal axis explained 39.74% (PC1 = 20.98% and PC2 = 18.76%) under optimum, 49.41% (PC1 = 30.85 and 18.56%) under random stress, 74.86% (PC1 = 41.95 and PC2 = 32.91%) under managed drought and 59.15% (PC1 = 39.11 and PC2 = 20.15%) under low N of the total G × E interaction variations. The which-won-where polygon view of GGE biplots in showed which genotypes performed well at which site. The line that starts from the origin of the biplot and passes perpendicular to the side of the polygon was divided into different sectors. Under optimum conditions, ten sectors; under random stress, seven sectors; under managed drought, six sectors and under low N conditions six sectors were formed. Hybrids that formed the vertex of the polygon were the highest yielding in the corresponding environments within each sector. On the other hand, hybrids located far away from all the test environments were considered as poor yielding at each testing site. The vertex hybrids 15, 41 and 12 were the highest yielding at AM2, BK1 and CHI sites, respectively, whereas hybrids 1, 4, 5 and 34 were low yielding under optimum conditions ().
Figure 1. “Which won where” polygon view of the GGE biplot for 40 QPM hybrids and four standard checks evaluated across (a) optimum management, (b) random stress, (c) managed drought and (d) low nitrogen stress. Environment codes are explained in .

Hybrids 14 and 19 were on the corners of the polygon and the highest yielding at BIN and MER, and UMB testing sites, respectively under random stress conditions (). Similarly, hybrids 9 and 10 were top yielding at CHI, and MAK and CHO respectively, while hybrids 31, 27, 25 and 15 were on the lower yielding vertex of the polygon under managed drought conditions (). Under low N conditions, hybrids 3, 7 and 34 were top yielding at the corresponding sites of HAR, AM1 and BK2, respectively, whereas hybrids 18 and 36 were on the low yielding vertex of the polygon (). Hybrids like 23, 7, 18, 43, 35 and 25 under optimum; 12, 31 and 24 under random stress; 33, 37, 29 and 11 under managed drought; 5, 40 and 35 under low N stress were relatively closer to the origin of the biplot and considered as more stable than hybrids far from the origin, in either positive or negative direction.
3.3.2. Mean vs. stability under different management conditions
Average environment coordination (AEC) view of the GGE biplot was drawn to see the stability and adaptability of hybrids evaluated under optimum, random stress, managed drought, and low N conditions (). The axis of the AEC ordinate is a line that passes through the origin of biplot and is perpendicular to the AEC abscissa to separate genotypes that had below average grain yield from those showing higher grain yields. The hybrids were ranked along the average environment axis with the arrow pointing to a higher value based on mean performance across all testing environments. The top yielding hybrids according to their projections onto the AEC, in descending order were 10, 12, 3, 15, 38 and 6 under optimum (); 14, 34, 38, 10, 39, 23, 9 and 3 under random stress (); 9, 10, 19, 40, 8 and 35 under managed drought (); and 34, 14, 3, 7, 27 and 1 under low N (). Among these top yielding hybrids, 3, 12 and 15 under optimum; 14 and 9 under random drought stress; 9, 8 and 10 under managed drought and 3 and 7 under low N conditions had large projections onto the AEC abscissa. Hence, these hybrids were unstable and might be adapted to specific environments. Hybrids like 10, 14, 44, 6, 9, 38 and 20 under optimum; 38, 23, 10, 3 (check) and 39 under random stress; 19, 40, 35, 13 and 12 under managed drought; 34, 14, 1 (check), 23 and 35 under low N environments had above average grain yield, located on the AEC abscissa with low projections. Hence, these hybrids were considered as stable and might have wide adaptability across corresponding environments. Among checks, hybrid 3 (PAN53) was the highest yielding normal maize hybrid in this study but it was not stable under all management conditions except under random stress. In contrast, hybrid 1 (ZS261) and 4 (SC533) were the lowest yielding and most stable QPM and normal maize checks, respectively under most conditions.
3.3.3. Genotype ranking
The small circle on the AEC axis, with an arrow pointing to it and drawn based on the genotype-focused biplot, represents the ideal hybrid. In this study, hybrid 10 under optimum (); hybrids 14 and 10 under random stress (); hybrids 19 and 40 under managed drought (); and hybrids 14 and 34 under low N () stress conditions were the most ideal. Contrary to this, hybrids 1 (ZS261) QPM check, 4 (normal maize check) and 5 were the least ideal genotypes under optimum and random drought conditions and hybrids 25 and 36 were the least ideal under managed drought and low N conditions, respectively.
3.3.4. Representativeness and discriminating ability, and association
The AEC axis indicated the most representative and discriminating testing environments among the testing sites (). A test environment with a small angle with the AEC axis is more representative than other environments and a longer vector length of the environment is considered as more discriminative (Yan & Tinker, Citation2006). In this study, BK2 was the most discriminating, but it was the least representative environment under optimum conditions, whereas KWE, BK1 and CHI were the most representative and discriminating environments. In contrast, MSE and CHT were non-representative and non-discriminating environments (). Under random stress, LIC was the most representative and non-discriminating environment. UMB and MER were the most discriminating and non-representative environments (). Under managed drought, CHO was the most representative, while CHI was the most discriminating testing site (). Under low N conditions, RAT was the most representative and the least discriminating environment, whereas HAR was the most discriminating, while AM1 was also a representative and non-discriminating environment ().
Figure 4. Discriminativeness vs. representativeness view of the GGE biplots based on grain yield of 40 new QPM hybrids and four standard checks evaluated across (a) optimum management, (b) random stress, (c) managed drought and (d) low nitrogen. Environment codes are explained in .

The angle between two environments shows the relationship among testing environments and this was visualized by vectors drawn by a dotted line that connects each testing site with the biplot origin (). An angle between two environments of <90° indicates a strong positive correlation between them, whereas an angle of equal to 90° indicates no correlation between them. A degree angle of >90° and <270°, indicates negative correlation between the environments (Yang et al., Citation2009). Under optimum conditions, testing sites such as KWE, RAT, LUS, MPO, BK1, AM1 had strong positive association because they had a less than 90° angle among them, whereas BK2 and HAR had a negative association (>90° angle).
Under random stress, MER and UMB showed negative association, while strong positive association was observed among LIC, BIN, MAG, GWB, GWE testing sites. Across managed drought testing sites, CHO and MAK showed positive association while CHI with MAK showed negative association. No correlation was observed between CHI and CHO sites. Under low N environments, BK2, GWB, AREX and HAR sites had strong positive association, whereas negative association was observed between AM1 with BK2 and AM1 with HAR. In this study Ethiopian sites Bako and Ambo were included for two seasons under both optimum and low N environmental conditions. Both Bako and Ambo sites showed positive association during season one and two under optimum and low N conditions ().
4. Discussion
In this study, significant variability was shown for yield and other important agronomic traits among experimental QPM hybrids across all environments. This indicates the existence of different genetic responses among hybrids for measured traits under non-stressed and stressed environments. Previous research also showed the presence of considerable variation among QPM hybrids under different management conditions for grain yield and other agronomic traits (Abakemal et al., Citation2016; Mebratu et al., Citation2019; Setimela et al., Citation2017; Wegary et al., Citation2014). The highest grain yield was recorded under optimum conditions, followed by random stress, and managed drought, while low N had the lowest grain yield. Even though all stress types had high negative effects on grain yield and other agronomic traits, the effects of low N and managed drought stress were more severe than random stress conditions. Grain yield reduction was also recorded up to 68% under managed drought and 71% under low N environments. This indicated that when N level decreases and drought conditions become more severe, grain yield is reduced (Bänziger et al., Citation2006). Drought and low N stress conditions that occur before or at anthesis, delaying silk emerging time, results in an increase in ASI, causing incomplete or zero fertilization and resulting in absence of kernel development (Beyene et al., Citation2013; Ertiro et al., Citation2017; Magorokosho et al., Citation2004). As a result, it is useful using secondary traits, like ASI and number of ears per plant as an efficient approach for indirect selection to improve maize grain yield under these stress conditions (Bänziger et al., Citation2006; Beyene et al., Citation2013; Ertiro et al., Citation2017; Magorokosho et al., Citation2004).
Among the top ten QPM hybrids, some hybrids showed high or comparable grain yield potential to the best non-QPM commercial checks. And almost all of them also showed higher grain yield than the best QPM checks under different management conditions. Compared to the mean of all checks and best QPM check, the top ten QPM hybrids showed high grain yield advantage across all management conditions. However, these top ten QPM hybrids also showed comparable or slightly higher grain yield compared to the best normal maize check under stressed and non-stressed environments. For more than three decades, CIMMYT, in collaboration with other institutes, have been developing QPM germplasms that are superior to commercial QPM and normal maize checks under stressed and non-stressed environments (Mebratu et al., Citation2019; Setimela et al., Citation2017; Wegary et al., Citation2014), and these findings were confirmed in the yield progress reported in this study. Setimela et al., (Citation2017) reported that QPM genotypes showed varying performance in different management conditions. However, in this research, QPM hybrids such as 9 (CZH142237Q), 10 (CZH142238Q) and 14 (CZH15142Q) performed the best under both drought and low N stress conditions. These findings confirmed the results reported by Beyene et al., (Citation2013) who found that maize varieties developed for drought tolerance also showed good levels of tolerance to low N stress. This suggesting the presence of common adaptive mechanisms for both water and low N stresses.
The results of the AMMI ANOVA indicated genotypic difference in grain yield performance among new QPM hybrids across testing sites under stress and non-stress environment conditions. Significant environmental variation and G × E interactions reflected the diversity of the testing environments and the reaction of the hybrids to this, indicating the existence of ranking change of QPM hybrids across different environments. This could significantly limit the selection of superior QPM hybrids that have good grain yield performance in multi-environments. A suitable breeding strategy should follow to develop genotypes that have good performance in multi-environments or genotypes adapted to a specific environment (Mebratu et al., Citation2019). Similar findings were reported in the previous studies conducted in tropical and sub-tropical maize genotypes evaluated under stress and non-stress environmental conditions (Abakemal et al., Citation2016; Mebratu et al., Citation2019; Matongera et al., Citation2023; Setimela et al., Citation2017).
Some QPM hybrids showed high positive and negative IPCA1 scores and yielded above the mean, indicating the existence of variation among hybrids in response to the different environments. These kinds of hybrids were considered as unstable and adapted to specific environments. On the other hand, some QPM hybrids also produced good mean grain yield and showed better stability than the rest of the hybrids (had close to zero IPCA1 and ASV values) across different management conditions. Similar findings have been reported in the previous research conducted on QPM hybrids (Abakemal et al., Citation2016; Mebratu et al., Citation2019; Setimela et al., Citation2017), on normal maize hybrids (Ertiro et al., Citation2017; Sserumaga et al., Citation2016; Wolde et al., Citation2018) and on PVA genotypes (Mengesha et al., Citation2019).
The GGE biplot was provided a polygon view to visualize “which-won-where” pattern () by dividing the biplot into different sectors. When different testing environments occurred in different sectors, it indicated that they have different winner hybrids for those sectors (Mebratu et al., Citation2019; Yan & Tinker, Citation2006), indicating the presence of crossover (G × E interaction). This suggesting the possibility of dividing the environments into different mega-environments (Yan et al., Citation2007) and help to deploy different hybrids in different mega-environments (Abakemal et al., Citation2016; Mebratu et al., Citation2019; Wolde et al., Citation2018). Under optimum conditions, the polygon was divided into ten sectors, but testing sites fell into three of them that were represented by BK2, AM2 and CHI (). Under random stress, the polygon was divided into seven sectors, but the testing sites fell only into two of them that were represented by MER and UMB sites (). Similarly, under managed drought, the polygon was divided into six sectors, but the testing sites fell into two of them that were represented by CHO and CHI (). Under low N conditions, the polygon was also divided into six sectors, but the testing sites fell into three of them that were represented by AM1, BK2, HAR (). On the other hand, genotypes that were placed far away from all the testing environments indicated that they were poor yielding at those testing environments (Makumbi et al., Citation2015; Wolde et al., Citation2018). In this study, hybrids 5 (CZH04032), 4 (SC533), and 34 (CZH17203Q) under optimum, 1 (ZS261), 25 (CZH17194Q) and 35 (CZH17204Q) under random stress, 25 (CZH17194Q), 27 (CZH17196Q) and 31(CZH17200Q) under managed drought and 36 (CZH17205Q) and 18 (CZH17187Q) under low N stress were poor performing hybrids. In general, the results of this study gave initial information on mega-environment delineation of ESA maize testing locations. To validate this information, multi-location and season data are needed.
Based on genotype ranking GGE biplot analysis, genotypes that are closest to the concentric circle are considered as ideal genotypes that have both high mean grain yield and are stable in multi-environments (Yan & Kang, Citation2003; Yan & Tinker, Citation2006; Wolde et al., Citation2018). As indicated in , the most desirable hybrids closest to the concentric circle under each management condition were identified. Entries 10 and 14 were identified as ideal genotypes across non-stressed and stressed environments, indicating that these hybrids had inherently high yielding potential and wide adaptability across different management conditions. Because of the genetic background of the genotypes or nature of the trait (grain yield), the ideal genotype does not always exist in the reality. However, such kind of hybrids can be used as a reference for further studies. On the other hand, entry 1 (ZS261), the QPM check, was far from the concentric circle under optimum and random drought environments compared with experimental QPM hybrids. This tells, this hybrid was inherently low yielding and poorly adapted to some or all the testing sites, which is similar with results reported by Mebratu et al. (Citation2019).
Environments that have small angles with the AEC are more representative, whereas long vectors from the biplot origin is considered as more discriminative environments (Yan & Tinker, Citation2006). Environments that have both long vectors and small angles with the AEC abscissa are more discriminating and representative environments and are considered “ideal testing sites” and are important to identify desirable hybrids (Mebratu et al., Citation2019). In this study, KWE under optimum (), BIN under random drought (), CHO under managed drought () and BK2 under low N stress () were both discriminating and representative environments. These sites were recognized as ideal environments in the respective management conditions. An environment which could discriminate the genotypes and is representative of the target environment is considered as the ideal environment (Yan & Tinker, Citation2006). So these testing environments can be used to select the top yielding hybrids that will perform consistently across environments (Mebratu et al., Citation2019; Tolessa & Gela, Citation2014). Testing environments which had strong negative association indicates the presence of cross-over among genotypes (Yan & Tinker, Citation2006). Even though most of the environments had positive association, negative association was shown between BK2 and HAR environments under optimum conditions, MER and UMB under random drought, CHI and MAK under managed drought and AM1 with BK2 and AM1 with HAR under low N conditions. If two testing sites associated consistently across seasons/years, one could be dropped without significant loss of information about the genotypes (Lule et al., Citation2014; Tukamuhabwa et al., Citation2012). In this study, Bako and Ambo testing sites showed positive association during season one and two both under optimum and low N conditions, but negative correlation was observed between BK2 and AM1 under low N. This kind of inconsistency might have been due to variation in weather conditions like rainfall, temperature, soil conditions, and other unpredictable factors.
5. Conclusions
In this study, promising QPM hybrids under stressed and non-stressed environments were identified. Based on AMMI and GGE biplot analysis, the most desirable hybrids were 6, 10, 14 and 20 under optimum; 3, 14 and 23 under random stress; 19, 35 and 40 under managed drought and 14 under low N conditions. GGE biplot analysis identified siting sites for proper selection of QPM hybrids on the basis of their discriminating ability and representativeness. Accordingly, Kwekwe (KWE), Bindura (BIN), Chokwe (CHO) and Bako (BK2) testing sites were the most suitable environments in discriminating the experimental QPM hybrids and being representative test environments for optimum, random stress and low N stress conditions, respectively. Generally, further evaluation of the selected QPM hybrids across multi-location and season would help to promote into variety verification for commercialization and could contribute to improve food and nutritional security problems in sub-Saharan Africa.
Author contributions
This work was conducted as part of BTE’s PhD thesis in plant breeding at University of the Free State, South Africa in collaboration with CIMMYT. The trials were planned and designed by AT, DW and BTE. The first draft of the manuscript was written by BTE and revised the manuscript by MTL. All authors have read and approved the final manuscript for submission.
Acknowledgments
The authors acknowledge the International Maize and Wheat Improvement Centre (CIMMYT), National Agricultural Research System and private seed company scientists and technicians who participated in conducting the trials and data collection at all testing sites. The National Research Foundation is also acknowledged for supporting the thesis work.
Disclosure statement
No potential conflict of interest was reported by the author(s).
Data availability statement
The data that support the findings of this study are included in the manuscript and further inquiries can be forwarded to the corresponding author.
Correction Statement
This article was originally published with errors, which have now been corrected in the online version. Please see Correction (http://dx.doi.org/10.1080/23311932.2024.2355806).
Additional information
Funding
References
- Abakemal, D., Shimelis, H., & Derera, J. (2016). Genotype-by-environment interaction and yield stability of quality protein maize hybrids developed from tropical-highland adapted inbred lines. Euphytica, 209(3), 1–19. https://doi.org/10.1007/s10681-016-1673-7
- Abate, T., Fisher, M., Abdoulaye, T., Kassie, G. T., Lunduka, R., Marenya, P., & Asnake, W. (2017). Characteristics of maize cultivars in Africa: How modern are they and how many do smallholder farmers grow? Agriculture & Food Security, 6(1), 30. https://doi.org/10.1186/s40066-017-0108-6
- Alvarado, G., Rodríguez, F. M., Pacheco, A., Burgueño, J., Crossa, J., Vargas, M., Pérez-Rodríguez, P., & Lopez-Cruz, M. A. (2020). META-R : A software to analyze data from multi-environment plant breeding trials. The Crop Journal, 8(5), 745–756. https://doi.org/10.1016/j.cj.2020.03.010
- Badu-Apraku, B., Fakorede, M. A. B., Talabi, A. O., Oyekunle, M., Akaogu, I. C., Akinwale, R. O., Annor, B., Melaku, G., Fasanmade, Y., & Aderounmu, M. (2016). Gene action and heterotic groups of early white quality protein maize inbreds under multiple stress environments. Crop Science, 56(1), 183–199. https://doi.org/10.2135/cropsci2015.05.0276
- Bänziger, M., Setimela, P. S., Hodson, D., & Vivek, B. (2006). Breeding for improved abiotic stress tolerance in maize adapted to southern Africa. Agricultural Water Management, 80(1–3), 212–224. https://doi.org/10.1016/j.agwat.2005.07.014
- Beyene, Y., Mugo, S., Semagn, K., Asea, G., Trevisan, W., Tarekegne, A., Tefera, T., Gethi, J., Kiula, B., Gakunga, J., Karaya, H., & Chavangi, A. (2013). Genetic distance among doubled haploid maize lines and their testcross performance under drought stress and non-stress conditions. Euphytica, 192(3), 379–392. https://doi.org/10.1007/s10681-013-0867-5
- Beyene, Y., Semagn, K., Mugo, S., Tarekegne, A., Babu, R., Meisel, B., Sehabiague, P., Makumbi, D., Magorokosho, C., Oikeh, S., Gakunga, J., Vargas, M., Olsen, M., Prasanna, B. M., Banziger, M., & Crossa, J. (2015). Genetic gains in grain yield through genomic selection in eight bi-parental maize populations under drought stress. Crop Science, 55(1), 154–163. https://doi.org/10.2135/cropsci2014.07.0460
- Ertiro, B. T., Beyene, Y., Das, B., Mugo, S., Olsen, M., Oikeh, S., Juma, C., Labuschagne, M., & Prasanna, B. M. (2017). Combining ability and testcross performance of drought-tolerant maize inbred lines under stress and non-stress environments in Kenya. Plant Breeding = Zeitschrift Fur Pflanzenzuchtung, 136(2), 197–205. https://doi.org/10.1111/pbr.12464
- Fisher, M., Abate, T., Lunduka, R. W., Asnake, W., Alemayehu, Y., & Madulu, R. B. (2015). Drought tolerant maize for farmer adaptation to drought in sub-Saharan Africa: Determinants of adoption in Eastern and Southern Africa. Climatic Change, 133(2), 283–299. https://doi.org/10.1007/s10584-015-1459-2
- Gastwirth, J. L., Gel, Y. R., & Miao, W. (2009). The impact of Levene’s test of equality of variances on statistical theory and practice. Statistical Science, 24(3), 343–360. https://doi.org/10.1214/09-STS301
- Gomez, K., & Gomez, A. (1984). Statistical procedures for agricultural research (2nd ed.). John Wiley and Sons.
- Krivanek, A. F., De Groote, H., Gunaratna, N. S., Diallo, A. O., & Friesen, D. K. (2007). Breeding and disseminating quality protein maize (QPM) for Africa. African Journal of Biotechnology, 6, 312–324.
- Lule, D., Fetene, M., de Villiers, S., & Tesfaye, K. (2014). Additive main effects and multiplicative interactions (AMMI) and genotype by environment interaction (GGE) biplot analyses aid selection of high yielding and adapted finger millet varieties. Journal of Applied Biosciences, 76(1), 6291–6303. https://doi.org/10.4314/jab.v76i1.1
- Magorokosho, C., Pixley, K. V., & Tongoona, P. (2004). Selection for drought tolerance in two tropical. African Crop Science Journal, 11(3), 151–161. https://doi.org/10.4314/acsj.v11i3.27566
- Magorokosho, C., Vivek, B., MacRobert, J., & Tarekegne, A. (2010). Characterization of maize germplasm grown in Eastern and Southern Africa. Results of the 2009 regional trials coordinated by CIMMYT. CIMMYT.
- Makumbi, D., Diallo, A., Kanampiu, F., Mugo, S., & Karaya, H. (2015). Agronomic performance and genotype × environment interaction of herbicide‐resistant maize varieties in eastern Africa. Crop Science, 55(2), 540–555. https://doi.org/10.2135/cropsci2014.08.0593
- Matongera, N., Ndhlela, T., van Biljon, A., & Labuschagne, M. (2023). Genotype x environment interaction and yield stability of normal and biofortified maize inbred lines in stress and non-stress environments. Cogent Food & Agriculture, 9(1), 2163868. https://doi.org/10.1080/23311932.2022.2163868
- Mebratu, A., Wegary, D., Mohammed, W., Teklewold, A., & Tarekegne, A. (2019). Genotype × environment interaction of quality protein maize hybrids under contrasting management conditions in Eastern and Southern Africa. Crop Science, 59(4), 1576–1589. https://doi.org/10.2135/cropsci2018.12.0722
- Mekonnen, T. W., Mekbib, F., Amsalu, B., Gedil, M., & Labuschagne, M. (2022). Genotype by environment interaction and grain yield stability of drought tolerant cowpea landraces in Ethiopia. Euphytica, 218(5), 57. https://doi.org/10.1007/s10681-022-03011-1
- Mengesha, W., Menkir, A., Meseka, S., Bossey, B., Afolabi, A., Burgueno, J., & Crossa, J. (2019). Factor analysis to investigate genotype and genotype × environment interaction effects on pro-vitamin A content and yield in maize synthetics. Euphytica, 215(11), 1–15. https://doi.org/10.1007/s10681-019-2505-3
- Meseka, S. K., Menkir, A., Ibrahim, A. E. S., & Ajala, S. O. (2013). Genetic analysis of maize inbred lines for tolerance to drought and low nitrogen. Jonares, 1, 29–36.
- Ngaboyison, C., Njoroge, K., Kirubi, D., & Githiri, S. M. (2012). Quality protein maize under low N and drought environments : Endosperm modification, protein and tryptophan concentrations in grain. Agricultural Journal, 7(5), 327–338. https://doi.org/10.3923/aj.2012.327.338
- Nuss, E. T., & Tanumihardjo, S. A. (2011). Quality protein maize for Africa: closing the protein inadequacy gap in vulnerable populations. Advances in Nutrition (Bethesda, MD), 2(3), 217–224. https://doi.org/10.3945/an.110.000182
- Pacheco, A., Vargas, M., Alvarado, G., Rodríguez, F., & López, M. (2015). Genotype by environment analysis with R software (GEA-R) (pp. 1–42). International Maize and Wheat Improvement Center.
- Purchase, J. L., Hatting, H., & Van Deventer, C. S. (2000). Genotype × environment interaction of winter wheat (Triticum aestivum L.) in South Africa: II. Stability analysis of yield performance. South African Journal of Plant and Soil, 17(3), 101–107. https://doi.org/10.1080/02571862.2000.10634878
- Rezende, W. S., Beyene, Y., Mugo, S., Ndou, E., Gowda, M., Sserumaga, J. P., Asea, G., Ngolinda, I., Jumbo, M., Oikeh, S. O., Olsen, M., Borém, A., Cruz, C. D., & Prasanna, B. M. (2020). Performance and yield stability of maize hybrids in stress-prone environments in Eastern Africa. The Crop Journal, 8(1), 107–118. https://doi.org/10.1016/j.cj.2019.08.001
- SAS. (2002). Statistical analysis system. Version 9.3 SAS institute Inc.
- Setimela, P. S., Gasura, E., & Tarekegne, A. T. (2017). Evaluation of grain yield and related agronomic traits of quality protein maize hybrids in Southern Africa. Euphytica, 213(12), 289. https://doi.org/10.1007/s10681-017-2082-2
- Shiferaw, B., Prasanna, B. M., Hellin, J., & Bänziger, M. (2011). Crops that feed the world 6. Past successes and future challenges to the role played by maize in global food security. Food Security, 3(3), 307–327. https://doi.org/10.1007/s12571-011-0140-5
- Sserumaga, J. P., Beyene, Y., Pillay, K., Kullaya, A., Oikeh, S. O., Mugo, S., Machida, L., Ngolinda, I., Asea, G., Ringo, J., Otim, M., Abalo, G., & Kiula, B. (2018). Grain-yield stability among tropical maize hybrids derived from doubled-haploid inbred lines under random drought stress and optimum moisture conditions. Crop & Pasture Science, 69(11), 691–712. https://doi.org/10.1071/CP17348
- Sserumaga, J. P., Oikeh, S. O., Mugo, S., Asea, G., Otim, M., Beyene, Y., Abalo, G., & Kikafunda, J. (2016). Genotype by environment interactions and agronomic performance of doubled haploids testcross maize (Zea mays L.) hybrids. Euphytica, 207(2), 353–365. https://doi.org/10.1007/s10681-015-1549-2
- Tandzi, L. N., Mutengwa, C. S., Ngonkeu, E. L. M., Woïn, N., & Gracen, V. (2017). Breeding for Quality Protein Maize (QPM) Varieties. Agronomy, 7(4), 80. https://doi.org/10.3390/agronomy7040080
- Teklewold, A., Wegary, D., Tadesse, A., Tadesse, B., Bantte, K., Friesen, D., & Prasanna, B. M. (2015). Quality protein maize (QPM): A guide to the technology and its promotion in Ethiopia. CIMMYT, Addis Ababa.
- Tolessa, T. T., & Gela, T. S. (2014). Sites regression GGE biplot analysis of haricot bean (Phaseolus vulgaris L.) genotypes in three contrasting environments. World Journal of Agricultural Research, 2(5), 228–236. https://doi.org/10.12691/wjar-2-5-5
- Tukamuhabwa, P., Asiimwe, M., Nabasirye, M., Kabayi, P., & Maphosa, M. (2012). Genotype by environment interaction of advanced generation soybean lines for grain yield in Uganda. African Crop Science Journal, 20(2), 107–116.
- Vivek, B. S., Krivanek, A. F., Palacios-Rojas, N., Twumasi-Afriyie, S., & Diallo, A. O. (2008). Breeding quality protein maize (QPM): Protocols for developing QPM cultivars. CIMMYT.
- Wegary, D., Labuschagne, M. T., & Vivek, B. S. (2011). Protein quality and endosperm modification of quality protein maize (Zea mays L.) under two contrasting soil nitrogen environments. Field Crops Research, 121(3), 408–415. https://doi.org/10.1016/j.fcr.2011.01.010
- Wegary, D., Vivek, B. S., & Labuschagne, M. T. (2014). Combining ability of certain agronomic traits in quality protein maize under stress and nonstress environments in Eastern and Southern Africa. Crop Science, 54(3), 1004–1014. https://doi.org/10.2135/cropsci2013.09.0585
- Wegary, D., Vivek, B., & Labuschagne, M. (2013). Association of parental genetic distance with heterosis and specific combining ability in quality protein maize. Euphytica, 191(2), 205–216. https://doi.org/10.1007/s10681-012-0757-2
- Wolde, L., Keno, T., Tadesse, B., Bogale, G., & Abebe, B. (2018). Mega-environment targeting of maize varieties using Ammi and GGE bi-plot analysis in Ethiopia. Ethiopian Journal of Agricultural Sciences, 28(2), 65–84.
- Yan, W. (2011). GGE biplot vs. AMMI graphs for genotype-by-environment data analysis. Journal of the Indian Society of Agricultural Statistics, 65(2), 181–193.
- Yang, R. C., Crossa, J., Cornelius, P. L., & Burgueño, J. (2009). Biplot analysis of genotype × environment interaction: Proceed with caution. Crop Science, 49(5), 1564–1576. https://doi.org/10.2135/cropsci2008.11.0665
- Yan, W., & Kang, M. S. (2003). GGE biplot analysis: A graphical tool for breeders, geneticists, and agronomists. CRC Press.
- Yan, W., Kang, M. S., Ma, B., Woods, S., & Cornelius, P. L. (2007). GGE biplot vs. AMMI analysis of genotype-by-environment data. Crop Science, 47(2), 643–653. https://doi.org/10.2135/cropsci2006.06.0374
- Yan, W., & Tinker, N. A. (2006). Biplot analysis of multi-environment trial data: Principles and applications. Canadian Journal of Plant Science, 86(3), 623–645. https://doi.org/10.4141/P05-169
- Zobel, R. W., Wright, M. J., & Gauch, H. G.Jr (1988). Statistical analysis of a yield trial. Agronomy Journal, 80(3), 388–393. https://doi.org/10.2134/agronj1988.00021962008000030002x