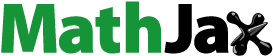
Abstract
Horticulture is an important sub-sector of the Kenyan economy that contributes to food and nutrition security as well as a source of income to small-scale farmers. However, the sub-sector is largely affected by climate variability. Although various efforts have been initiated towards climate-smart horticulture (CSH) practices to reduce the challenges, most farmers have not adopted the practices and the reasons for this are not comprehensively documented. This study sought to address this knowledge gap by analyzing the determinants of the intensity of adoption of CSH practices in Taita-Taveta County in Kenya. The study used primary data from 403 randomly selected farmers who grow green grams and tomatoes. Negative binomial regression model was applied to analyze the determinants of the number of CSH practices adopted. Results show that the main CSH practices adopted were crop rotation, well-adapted seed varieties, live barriers to prevent soil erosion and organic manure. The extent of adoption of CSH practices varied greatly in the three locations due to their geographical and agro-ecological differences. Mobile phone use on CSH, access to agricultural extension services, awareness of CSH and trust on information relayed through the mobile phones increased the intensity of adoption of the identified CSH practices by 24%, 17%, 54% and 12%, respectively. However, climate change awareness and increase in farm size under crop reduced the intensity of adoption of CSH practices by 29% and 4%, respectively. The intensity of adoption of CSH practices was positively affected by mobile phone use, education level, gender, trust and CSH awareness. However, farm size and climate change awareness were found to negatively affect the intensity of adoption of CSH practices. These findings point to the need for location-specific CSH practices and credible climate-smart agriculture (CSA) information. Institutional support services such as agricultural extension, technical skills and mobile phone services should be packaged innovatively to meet the specific requirements of horticulture farmers in different crop enterprises and agro-ecological conditions rather than a one-size fits all approach.
REVIEWING EDITOR:
1. Introduction
Global climate change exhibited through occurrences such as extreme changes in temperature, unpredictable droughts and floods, and variability in rainfall patterns exert serious challenges on horticultural crop production including planting and harvesting schedules and quality of farm output (FAO, Citation2022; Shah et al., Citation2024). The country climate and development reports (CCDR) published by the Conference of the Parties to the United Nations Framework Convention on Climate Change (COP) (specifically COP28) from 42 countries, show that the world is alert on averting the negative impacts of climate change through policy frameworks that will lead to action (World Bank, Citation2023). There have also been concerted efforts by the Food and Agriculture Organization of the United Nations (FAO) to support farmers to develop and implement country-specific climate smart agriculture strategies and technologies around the world in order to deal with the consequences of climate variability (FAO, Citation2021, Citation2022).
According to the world economic forum, Africa derives about 35% of its gross domestic product (GDP) from agriculture. This increases the vulnerability of African states to climate change problems. For example, it is projected that climate shocks will reduce agricultural productivity by up to 17% by the year 2050 across many countries in Sub-Saharan Africa (SSA) since cropping systems are 95% rain-fed (McKinsey Global Institute, Citation2020; World Bank, Citation2023). Further, a thirteen-year assessment of the welfare cost of drought in the SSA region showed that weather variability increased total poverty gap to between 4 million to 2.4 billion US dollars (World Bank, Citation2024). This implies that there is an urgent need to rapidly employ climate-smart agriculture (CSA) strategies that seek to increase productivity, enhance resilience and reduce greenhouse gas emissions.
Horticulture is the second largest foreign exchange earner to Kenya’s economy after tea. It contributes 26% of the agricultural GDP; much of this is from flower farming where 95% of output is exported. About 96% of the fruits and vegetables output is consumed locally; 90% of this coming from small-scale farms (Kenya National Bureau of Statistics (KNBS), Citation2023), which underscores the critical role that the sector plays in the economy. However, the sector faces a myriad of challenges that include inconsistent climatic conditions, which influence pests and disease infestation, water availability, production schedules and output quality (Azam et al., Citation2017; Andati et al., Citation2022; Lanari et al., Citation2016).
In Taita-Taveta, crop farmers have continued to experience yield losses including 75% for maize, 69% for beans and 48% for green grams occasioned by inconsistent climate variability, such as rising temperatures and erratic rainfall patterns (Motaroki et al., Citation2021; Nyambariga et al., Citation2023). For instance, prolonged droughts and rising temperatures caused streams (which are main sources of irrigation water) in Taita hills and some lower parts of the county to dry severely affecting the source of livelihood for crop farmers. This situation is predicted to worsen in future and contribute considerable negative impacts on the food security situation and poverty levels of the farmers in the area (MoALF, Citation2016; Nyambariga et al., Citation2023). In the year 2022, the volume of vegetables exported declined by 20% due to low production. This was occasioned by climate change impacts including prolonged droughts, low water levels and crop failure in the key horticulture producing parts of the country (KNBS, Citation2023).
There have been concerted efforts by the government of Kenya (through Kenya climate smart agriculture project (KCSAP)) to alleviate the climate change problems faced by farmers. However, the approach is broad-based and does not support all the crop value chains. Therefore, specific interventions such as climate-smart horticulture (CSH) are needed to urgently prevent the negative climate change impacts from worsening. The CSH conceptFootnote1 entails adapting and building resilience of horticulture to climate variability, improving horticulture productivity and food security while being cognizant of the environmental sustainability concerns (Sahu, Citation2016).
The CSH practices can be grouped into four main categories that entail innovative ways of: managing farms, crops, reducing farm risk and conserving the soil (Thornton et al., Citation2018; Wekesa et al., Citation2018). Innovative crop management methods include integrated pest management, crop irrigation, use of improved seed varieties that are well adapted to local climate, crop rotation, matching planting dates to climatic conditions and efficient use of inorganic fertilizers (Shah & Wu, Citation2019).
General field management practices include use of terraces, agroforestry and use of live barriers – which are strips of crops (such as grass) planted along a contour to prevent soil erosion (Caulfield et al., Citation2020). Soil conservation practices entail the use of organic fertilizers, cover crops, composting, mulching and conservation agriculture (Baumhardt & Blanco-Canqui, Citation2018).
On the other hand, farm risk reduction practices include crop diversification, use of farm water ponds, use of information technologies to guide farm activities and crop insurance (Malhi et al., Citation2021; Sorvali et al., Citation2021).
Previous studies have indicated that farmers who adopted all the four main CSH practices had higher household food consumption scores (HFCS) and crop revenues than non-adopters (Issahaku & Abdulai, Citation2019; Wekesa et al., Citation2018). It has also been shown that adoption of CSA practices is affected by a myriad of factors. For example, Diro et al. (Citation2022), Issahaku and Abdulai (Citation2019) and Kurgat et al. (Citation2020) revealed that gender, household resources, education of the household head, access to weather information and agricultural extension and ownership of communication devices are major determinants of CSA adoption.
Further, while Musafiri et al. (Citation2022) found that women-headed households adopted more CSA practices than their men counterparts in Western Kenya, Negera et al. (Citation2022) obtained contrary findings in Ethiopia (where men-headed household farmers adopted more CSA practices than women-headed household farmers). This means that there is need for more studies to provide clarity on the household gender variable and its effects on climate change management efforts. Mashi et al. (Citation2022) also noted that awareness and subsequent adoption of CSA technologies and practices in Nigeria depended on farmer and farm environmental factors such as education, economic assets and climate change experience. More specifically, Cavanagh et al. (Citation2017) found an average adoption rate of 53.6% for CSA land management practices; with the ‘very poor’ and ‘poor’ groups exhibiting substantially lower adoption rates (42% and 49%, respectively) relative to the ‘less poor’ wealth groups (73%) in Western Kenya. Facilitating access to credit facilities by poor farmers could help improve the adoption of CSA technologies (Waaswa et al., Citation2024).
While these studies provide rich insights on the determinants of CSA adoption, there is limited empirical knowledge on the factors that influence the intensity of adoption of CSH practices (Howland et al., Citation2018). Taita Taveta is among the counties that have been implementing the KCSAP project since the year 2017 but no study has been conducted on the determinants of adoption of CSH practices in the area. This is the knowledge gap that the present study fills in the specific case of smallholder green gram and tomato farmers in Taita-Taveta, Kenya, which are key sources of income for households, but unpredictable climatic conditions remain a significant barrier (MoALF, Citation2016; Motaroki et al., Citation2021; Taita Taveta County Government, Citation2023).
This study contributes to the existing body of knowledge on climate-smart agriculture by analyzing the determinants of the intensity of adoption of climate-smart horticulture practices in Taita-Taveta County, Kenya and help to shape policy and agricultural development interventions in the area.
2. Methodology
2.1. Study site
Taita-Taveta County is one of the six counties in the Coastal region of Kenya and covers a land area of 17,084.1 Km2 but nearly two-thirds of the land area is occupied by Tsavo West national park leaving only one-third for farming and other activities. The area has eight different agro-ecological zones that range from lowland zone (altitude of below 610 m above the sea level) to lower highland zone (altitude of above 1680 m) (Jaetzold et al., Citation2010; Taita Taveta County Government, Citation2023). It also has different types of soil varying from humic cambisols, rhodic farralsols and calcic cambisols in Wundanyi, Mwatate and Taveta, respectively (Omwakwe et al., Citation2022). The county has an average temperature of 23°C and receives an annual mean rainfall of 650 mm. Most parts are generally dry except for the Taita hills area, which has relatively high rainfall and a total arable land of 2,055 km2. The variations in temperatures and rainfall pattern in the area affect the distribution of diseases and water availability for horticulture farmers, which largely affect their productivity. The farmers practice different farming systems (such as commercialized irrigation, rain-fed and mixed farming systems) owing to the difference in agro-ecological setting and apply different CSA technologies including minimum or zero tillage, crop rotation and planting drought tolerant crop varieties (Autio et al., Citation2021; Motaroki et al., Citation2021). The county is the highest producer of horticultural crops in the Coastal region and contributes about 6% to the national domestic value of marketed horticultural output but still has high number of people who are multi-dimensionally poor relative to national levels (HCD, Citation2020; Taita Taveta County Government, Citation2023). The main horticultural crops grown are tomatoes, bananas, cabbages, onions, French beans and green grams ().
2.2. Sampling and data collection
A multistage sampling technique was applied to select the study sites and respondents. In the first step, Mwatate, Taveta and Wundanyi sub-counties were purposively selected due to high population of crop farmers compared to livestock farmers. Also, the three sub-counties provide different agro-ecological zones and climate setting which are relevant to the context of this study. Before data collection, ethical clearance was obtained from the University of Nairobi and National Commission for Science, Technology and Innovation (license number: NACOSTI/P/21/9137). Data was collected in February/March 2021 through a focus group discussion (FGD) checklist questionnaire and household survey structured questionnaire.
The FGD had eleven (11) participants comprising tomato and green gram farmers, agricultural extension officers, farm input dealers, local administrator and credit providers. The discussion focused on the meaning of climate change and CSH practices adopted in the area.
This helped to gain broader insights on the study area and validate the information collected in the subsequent household survey. The use of questionnaires in the household survey was preferred because it provided a structured sequence in data collection, where the respondents were supposed to provide direct answers to the research questions. The questionnaire was pretested and validated before the survey. The sample size was calculated using the formula of Cochran (Citation1977) because the exact population of tomatoes and green grams farmers in Taita-Taveta County was not known. The formula is shown in EquationEquation (1)(1)
(1) .
(1)
(1)
where
is the sample size, Z is the Z-critical value at a particular confidence level, p is the maximum level of variance, q is (1-p) and e is the desired margin of error. This study used 95.1% confidence level and 0.049 desired margin of error. The p was assumed to take a value of 0.5, since the disparity among CSH farmers was not known and the fact that the study covered different agro-ecological zones. Therefore, the sample size was calculated as shown in EquationEquation (2)
(2)
(2) ;
(2)
(2)
This sample size was proportionately distributed among the three sub-counties according to the 2019 Kenya population and housing census (KNBS, Citation2019) as shown below;
(3)
(3)
(4)
(4)
(5)
(5)
An extra 20 farmers (equivalent to 5% of sample size) were added to cater for incomplete questionnaires and potential non-response. Subsequently, four trained enumerators randomly picked respondents from the three sub-counties using random transect walks in the selected villages for interviews. Prior to the interviews, the enumerators sought the consent of respondents, assured them of data privacy and interviewed only those who were 18 years of age and above.
In addition, the enumerators were guided by village guides from the local area for acceptability and to assist with translation to local languages in cases where farmers didn’t understand either Swahili or English languages. A total of 415 respondents were interviewed during the field survey but 12 questionnaires were found to be inadequately filled hence they were not used in the analysis. There was low response rate than expected from Wundanyi and Mwatate sub-counties, where some farmers refused to be interviewed. The difference was compensated by interviewing more farmers in Taveta sub-county. Therefore, this study used 403 questionnaires for analysis (59 from Wundanyi, 122 from Mwatate and 222 from Taveta); 115 respondents were tomato farmers, 259 green gram farmers while 29 farmers produced both green grams and tomatoes.
2.3. Conceptual framework
Climate change problems such as erratic rainfall pattern and high temperatures leading to floods and prolonged droughts, often result in huge losses of agricultural output (FAO, Citation2018). In order to reduce this damage, farmers in the horticultural sector need to adopt climate-smart practices such as innovative timing of planting and harvesting periods, using seed varieties adapted to the local environment, crop rotation and diversification, insurance, integrated pest management, conservation agriculture and using real-time weather information from meteorologists to plan farm activities that build resilience and improve farm productivity (Amadu et al., Citation2020; Moranga, Citation2016; Wekesa et al., Citation2018).
However, improved adoption of these practices depends on several factors such as education, gender, CSH awareness, farmer’s financial and physical asset endowment, farm size, use of mobile phone to access CSH information, access to credit and extension services, distance from farm to commercial bank, trust on CSH information conveyed via mobile phone and group membership (Kassa & Abdi, Citation2022; Ogisi & Begho, Citation2023). All these factors including adoption intensities are influenced by the policy environment and market access at macro-level. The interaction of these factors and how they influence the intensity of adoption of CSH practices are presented in .
2.4. Data analysis
Considering that the dependent variable (number of CSH practices adopted) was a non-negative count variable, either a Poisson model or negative binomial regression model (NBRM) could be used in the analysis (Greene, Citation2003; Wooldridge, Citation2013). The negative binomial regression is a discrete distribution of the number of successes in a sequence of independent and identically distributed Bernoulli trials before a specified number of failures are observed (Yang & Berdine, Citation2015).
The NBRM is used for modelling count variables that follow the negative binomial distribution. The NBRM was preferred in this study because it generalizes the Poisson model by introducing unobserved effect into the conditional mean and relaxes the assumption of equality between the conditional mean and variance. This means that the NBRM does better for over-dispersed data than the Poisson model (Greene, Citation2003) (). Therefore, this study applied the NBRM expressed as:
(6)
(6)
where
is distribution parameter,
(countable variable that takes non-negative integer),
is a vector of independent variables and
is the stochastic term. This model was run using Stata 13 software by expressing EquationEquation (6)
(6)
(6) as follows:
(7)
(7)
Table 1. Variables used in the analysis.
Taking logs on EquationEquation (7)(7)
(7) ;
(8)
(8)
3. Results and discussion
3.1. Characteristics of farmers adopting climate-smart horticulture practices
Majority of farmers were men (60% pooled average) and practiced tomato farming (84%) while more women farmers (53%) produced green grams (). The average household size consisted of 5 members, which is 20% higher than the national figure (KNBS, Citation2019). Further, results show that most farmers had completed eight years in school. This means that the farmers had completed the primary level of education according to the current education system in Kenya. It was noted that the farmers had more than 9 years of experience in tomato and green gram production. This means that they were aware of various challenges and opportunities exhibited by the crop. The average land size under green grams and tomatoes was slightly more than one acre (1.39 acres pooled average). This was less than 50% of their average farm size (Nyambariga et al., Citation2022).
Table 2. Characteristics of different types of climate-smart horticulture farmers.
Over half of farmers used their mobile phones on CSH and trusted the information relayed through it. The findings show that less than half of farmers were in farmer groups. Similarly, less than 15% of farmers in both crops accessed credit and 92% were aware of climate change. The farmers aware of CSH practices were 69%. Most farmers were far from a commercial bank (19 km on average). This reduces the opportunities for farmers to access credit unless they use mobile banking services.
Although women generally dominate labour supply in farming, the high number of men interviewed in this study could be an artefact of the random sampling approach and the fact that male-household heads in many African cultures rarely permit female persons to participate in research interviews. The relatively higher number of male respondents for tomato than green grams is attributed to the fact that tomato is a high value crop and has higher returns while green grams have low returns. It also implies that men farmers control most of the market-oriented crops (IITA, Citation2016). This finding is consistent with Rodgers and Akram-Lodhi (Citation2018) and Leavens and Anderson (Citation2011) who found that cash crops, which were high-value crops were mainly grown by men.
The average household size consisted of 5 members, which is 20% higher than the national figure (KNBS, Citation2019). The household size affects the adoption of CSA practices (Kassa & Abdi, Citation2022; Tadesse & Ahmed, Citation2023). For example, Agbenyo et al. (Citation2022) showed that cocoa farmers who adopted irrigation had significantly smaller household sizes compared to those who did not adopt but the results were vice versa on adoption of organic fertilizers. This implies that a large household size favors adoption of labor-intensive CSA practices.
Most farmers in Taita-Taveta County had completed eight years of formal schooling. This means that the farmers had completed the primary level of education according to the current education system in Kenya. It is also an indication that most farmers were able to read and write, which is in line with the national literacy levels depicted by an increase in the number of primary school students joining secondary schools (KNBS, Citation2023). Education shapes the perception of farmers and increases their likelihood of adopting CSA practices (Kafando et al., Citation2022; Kalu & Mbanasor, Citation2020). It also enables farmers to interpret climate-smart information (such as weather predictions) and match it with the right type and variety of crop.
The farmers in the area had more than 9 years of experience in tomato and green gram production, which implies that they were aware of various challenges and opportunities exhibited by the crops. For example, they were aware of varieties that were easily destroyed by storage pests and therefore sold them immediately after harvesting to avoid post-harvest losses. Further, some tomato farmers were able to distinguish the effectiveness of different agro-chemicals on various pests.
The relatively small farm sizes allocated to both green grams and tomatoes could be explained by the cost of inputs such as fertilizer, agro-chemicals and water, which represent a considerable expense to many peasant farmers. Over half of farmers used their mobile phones on CSH and trusted the information relayed through it. This means that mobile phones are becoming part of important factors of production among farming households (Aminou et al., Citation2018). Factors such as improved access to mobile phone network, electricity (solar and hydro-electricity) and reduced cost of acquiring mobile phones by the farmers are attributed to their increased use in farming (CGTT, Citation2018; Mwikamba et al., Citation2022).
The results also show that less than half of farmers were members of farmer groups. These findings are similar to that of Ahmed et al. (Citation2023) who found that almost half of the farmers in Ethiopia were in farmer groups (both formal and informal). This implies that these farmers are connected through social networks that play an important role in peer learning about production technologies and can thus improve adoption of CSH practices. Similarly, less than 15% of farmers accessed credit. The low access to credit is attributed to lack of collateral and collective action for credit (Twine et al., Citation2019) since most loans offered through government departments such as the youth enterprise development fund, women enterprise fund and national government affirmative action fund were mainly issued to groups. This was a limitation to farmers in the area as most of them were operating in family farms, leased land while others were squatters.
Further, the results show that over 90% of farmers were aware of climate change. However, less than 70% were aware of CSH practices. This implies that their capacity to deal with emerging climate change related problems was limited due to lack of relevant knowledge and skills. Also, the results show that, on average, farmers were away from the nearest commercial bank (19 km on average). This reduces the opportunities for farmers to access credit unless they use mobile banking services.
The deliberate focus on the commercial banks (including the Agricultural Finance Corporation, Equity bank, Kenya commercial bank and Qwetu Sacco – a deposit taking savings and credit cooperative) was because farmers trusted these institutions more than micro-finance institutions operating in the area.
3.2. Adoption of climate-smart horticulture practices by crop and geographical location
shows the adoption of CSH practices by crops planted. The results demonstrate that the CSH practice adopted is closely related to the type of crop produced. Crop rotation was the most adopted practice by farmers in the area. This is because crop rotation is perceived as the cheapest method to control weeds, pests and diseases and restore soil fertility (Jalli et al., Citation2021; Weisberger et al., Citation2019).
On the contrary, a very low number (<10%) of farmers adopted crop insurance. This is in line with previous studies that showed low crop insurance adoption rates due to inadequate knowledge about insurance, high cost of premiums and the fact that most small-scale farmers are poor (Ankrah et al., Citation2021). Tomato farmers adopted more CSH practices compared to green gram farmers. This is because tomatoes are more sensitive to soil, nutrients and climatic conditions compared to green grams, which are more resilient to climatic changes. Moreover, considering that tomato is a high value crop, farmers are willing to invest more in it with an expectation of higher returns.
shows that the percentage of farmers who adopted CSH practices greatly varied according to the location. For instance, well-adapted seed varieties were mainly used by farmers in Wundanyi and least in Taveta. Most CSH practices identified were highly adopted by farmers in Wundanyi (highlands) (70%) compared to Mwatate (61%) and Taveta (40%). The CSH practices include crop rotation, use of cover crops, terracing, use of live barriers, use of organic fertilizer or compost manure, contour cultivation and mulching.
Table 3. Differences in adoption of CSH practices in the study locations.
The percentage of farmers who adopted CSH practices greatly varied according to the location (). For instance, well-adapted seed varieties were mainly used by farmers in Wundanyi and least in Taveta. This is attributed to location-specific factors where Wundanyi has experienced more dramatic climate variations (including permanent streams drying up) compared to Mwatate and Taveta (CGTT, Citation2018). Therefore, farmers had to choose their seeds wisely since they are dependent on rain. This finding conforms to previous studies, which noted that agro-ecological, crop variety characteristics and rainfall affected the choice of crop variety to be produced (Dasmani et al., Citation2020; Kaliba et al., Citation2000).
Most CSH practices identified were highly adopted by farmers in Wundanyi (highlands) compared to Mwatate and Taveta (). The high adoption of live barriers, terracing, cover cropping and contour cultivation is attributed to the topography of Wundanyi sub-county – which is characterized by steep slopes. This led farmers to use these methods to prevent soil erosion. This means that adoption of CSH practices are site specific (Thornton et al., Citation2018).
High adoption of organic manure is due to the farming systems – such as mixed farming (livestock and crop farming) existing in the area. This makes manure readily available for farming. Likewise, the small sizes of farm land available in the Taita hills (mostly Wundanyi) and the belief that certain farming methods control crop pests make farmers to practice crop rotation (Acheampong et al., Citation2021).
3.3. Overall climate smart horticultural practices adopted by farmers in Taita-Taveta County
Pooled results in show that 71% of farmers in Taita-Taveta County adopted crop rotation while only 2% adopted crop insurance. Terracing and use of well-adapted seed varieties were adopted by over half of farmers. These results conform to those of Oostendorp et al. (Citation2021) who found that most farmers (over 66%) in Western Kenya adopted improved seed varieties. On the other hand, more than half of farmers did not adopt other practices such as integrated pest management, contour cultivation, mulching, minimum tillage, water harvesting, agroforestry and efficient use of inorganic fertilizer.
Andati et al. (Citation2022) showed that adoption of climate-smart agriculture practices by farmers in Kenya was largely determined by their entrepreneurial orientation reflected in their pro-activeness, innovativeness and risk taking behavior. The high adoption of crop rotation is attributed to the farmers’ perception that it is the cheapest climate-smart approach compared to other methods such as crop insurance. This could also be attributed to the farmer’s innovativeness (Andati et al., Citation2022). Further, the limited knowledge on insurance products among small-scale farmers and high premiums charged hinder them from adopting these products (Ankrah et al., Citation2021). These findings are similar to those of Kurgat et al. (Citation2020) who found that farmers in Tanzania adopted various practices based on their location, specific needs and affordability.
3.4. Factors influencing the intensity of adoption of climate-smart horticulture practices
presents the results from the NBRM on the determinants of the intensity of adoption of CSH practices. In this study, a CSH farmer was described as a farmer who adopted at least one of the CSH practices. Using a mobile phone on CSH increased the number of CSH practices adopted by 24%. Most farmers in the area received information, through short messaging service (SMS), from the international crop research institute for semi-arid tropics (ICRISAT). The organization advised them on the time and type of crop to plant. Other farmers received weather information from the Kenya meteorological department. Improving CSH awareness increased the number of CSH practices adopted by 54%. The farmers received climate-smart horticulture information from various sources including KCSAP officers, ICRISAT agricultural extension agents, other farmers and online sources – through the smartphones. However, being aware of climate change decreased the number of CSH practices adopted by 29%.
Table 4. Negative binomial regression results on determinants of the number of CSH practices adopted in Taita-Taveta County.
The study found that increasing education level and farming experience by a year increases the number of CSH practices adopted by 4% and 1%, respectively. Access to agricultural extension services and trust on the information received increased the number of CSH practices adopted by 17% and 12%, respectively. On the other hand, increasing farm size under green grams and tomatoes by one acre decreases the number of CSH practices adopted by 4%.
Using a mobile phone on CSH increased the number of CSH practices adopted by 24% (). This may be attributed to reduced costs in searching for climate-smart information and easy connectivity with other farmers through the mobile phone. This finding is consistent with the observation of Mittal and Hariharan (Citation2018) who showed that farmers who used mobile phones in farming had adopted at least one improved technology to deal with climate change consequences.
Improving CSH awareness increased the number of CSH practices adopted by 54%. This is because farmers who were aware of CSH had knowledge on which practices were best suited to their farms. Also, awareness determines the nature and quality of climate change adaptation methods (Abbasi & Nawaz, Citation2020). The results conform to Rohila et al. (Citation2018) who found that CSA practices awareness improved adoption and productivity in Haryana state, India. However, being aware of climate change decreased the number of CSH practices adopted by 29%. The finding conforms to Nyang et al. (Citation2021) who found that most farmers in Kisii County, Kenya, perceived climate change but adopted indigenous strategies, that did not require technical skills. This implies that merely being aware of climate change does not necessarily mean that a farmer will adopt the CSH practices. This is because awareness without addressing the factors limiting adoption cannot overcome the serious issues caused by climate change (Tufa et al., Citation2023).
The study found that increasing education level and farming experience by a year increases the number of CSH practices adopted. This implies that adoption of CSH practices depends on the skills accumulated through the education system and farmers’ own experience in farming. This finding conforms to Akrofi-Atitianti et al. (Citation2018) and Li et al. (Citation2019) who revealed that farmers’ education level and experience increased the likelihood of practicing CSA. These findings reinforce the observation among rice producers in Nepal by Upendram et al. (Citation2023) that lack of information and technical knowledge are among the key reasons for non-adoption of climate change adaptation practices by smallholder farmers.
Access to agricultural extension services and trust on the information received increased the number of CSH practices adopted. This means that extension services coupled with farmers’ perception and belief on the information received would have a great effect in shaping agricultural practices and technology adoption. Similar findings were obtained by Li et al. (Citation2019) who noted that institutional factors, including trust, shaped the adoption of agricultural innovations. Upendram et al. (Citation2023) also noted that access to institutional resources significantly enhance the likelihood of adoption of more climate change mitigation practices.
On the other hand, increasing farm size under green grams and tomatoes by one acre decreases the number of CSH practices adopted. This finding contradicts that of Wekesa et al. (Citation2018) who noted that increasing farm size increased the adoption of CSA practices. However, Ogisi and Begho (Citation2023) observed that though the effect of farm size on adoption of climate-smart agriculture practices (CSAP) showed mixed results, the direction of the effect largely depended on the type of CSAP being assessed and location of the farmer. The negative effect of farm size in this study could be attributed to inadequate resources required to meet the CSH costs, which increases with farm size(s). The difference in context (including type of crop and agroecological zones) of the farmers could be attributed to the negative effect of farm size on CSH practice adoption. This is because farmers in the lower zones of Mwatate and Taveta had large tracts of land but adopted less CSH practices due to capital resource constraints.
4. Conclusion
The adoption of one or more of CSH practices was largely determined by; awareness of climate-smart horticulture (what it means in theory and practice), use of mobile phones to access and facilitate sharing of CSH-related information and products, farmer’s education level, access to agricultural extension services and trust on the information received through the mobile phones. There is need for farmer organizations and governments to collaborate in building the capacity of farmers on various crop specific CSH and risk reduction practices. Insurance firms that cover crops need to create awareness at the local level to ensure farmers understand their products and terms of service. Further, agricultural development partners such as the Food and Agriculture Organization of the United Nations (FAO) and the World Vision need to deliberately support programs that provide specific CSH knowledge and skills to farmers on soil, water, crop and agricultural market management.
Farmers’ sensitization on appropriate mobile phone platforms utilization, such as the online social groups/networks (including WhatsApp, twitter and Facebook, and short messaging services (SMS)) should be encouraged to improve access and sharing of useful CSH information and skills. This will bridge the gap in access to agricultural extension services that is already constrained. Farmers should also be encouraged to utilize their mobile phones to access digital credit to facilitate access to well adapted seed varieties, organic manure and development of farm water ponds, which require considerable funding. The county government and development partners, through their agricultural extension agents, should put more effort into addressing location and crop specific CSH practices for farmers to improve their productivity. The use of mobile phone platforms such as WhatsApp, SMS and encouraging farmers to use smartphones (to access various CSH sites -including YouTube and other mobile applications) would be useful in enhancing farmers awareness of CSH practices.
The findings of this study imply that there is a great potential for farmers to improve horticulture production and food security in the region despite the changing climatic conditions. Considering that the study was limited to Taita-Taveta County with specific interest on green grams and tomato crops, there is need for context-specific climate-smart interventions to enable farmers to realize the full benefits of the CSH policies. Moreover, future research efforts should focus on comparative analysis of the effect of use or non-use of mobile phones in CSH on farmers’ welfare indicators such as income, nutrition and health.
Disclosure statement
The authors report that there are no competing interests to declare.
Additional information
Funding
Notes
1 CSH concept entails adapting and building resilience of horticulture to climate variability, improving productivity and food security while being cognizant of the environmental sustainability concerns. This concept slightly differs with CSA (as defined by Lipper et al., Citation2014); since the latter (CSA) is an approach/concept that considers agriculture (including all crops and livestock) in totality – hence broad-based. However, the two concepts follow the same principles of adaptability and building resilience to climate change and improving productivity.
References
- Abbasi, Z., & Nawaz, A. (2020). Impact of climate change awareness on climate change adaptions and climate change adaptation issues. Pakistan Journal of Agricultural Research, 36(3), 1–15. https://doi.org/10.17582/journal.pjar/2020/33.3.619.636
- Acheampong, E., Sayer, J., Macgregor, C., & Sloan, S. (2021). Factors influencing the adoption of agricultural practices in Ghana’s forest-fringe communities. Land, 10(3), 266. https://doi.org/10.3390/land10030266
- Agbenyo, W., Jiang, Y., Jia, X., Wang, J., Ntim-Amo, G., Dunya, R., Siaw, A., Asare, I., & Twumasi, M. (2022). Does the adoption of climate-smart agricultural practices impact farmers’ income? Evidence from Ghana. International Journal of Environmental Research and Public Health, 19(7), 3804. https://doi.org/10.3390/ijerph19073804
- Ahmed, B., Haji, J., Ketema, M., & Jemal, K. (2023). Impacts and adaptation extents of climate smart agricultural practices among smallholder farmers of Ethiopia: Implication to food and nutrition security. Cogent Economics & Finance, 11(1), 1–21. https://doi.org/10.1080/23322039.2023.2210911
- Akrofi-Atitianti, F., Speranza, C., Bockel, L., & Asare, R. (2018). Assessing climate smart agriculture and its determinants of practice in Ghana: A case of the cocoa production system. Land, 7(1), 30. https://doi.org/10.3390/land7010030
- Amadu, F. O., Mcnamara, P. E., & Miller, D. C. (2020). Understanding the adoption of climate-smart agriculture: A farm-level typology with empirical evidence from southern Malawi. World Development, 126, 104692. https://doi.org/10.1016/j.worlddev.2019.104692
- Aminou, F., Houensou, D., & Hekponhoue, S. (2018). Effect of mobile phone ownership on agricultural productivity in Benin: The case of maize farmers. Journal of Economics and Development Studies, 6(4), 77–88. https://doi.org/10.15640/jeds.v6n4a7
- Andati, P., Majiwa, E., Ngigi, M., Mbeche, R., & Ateka, J. (2022). Determinants of adoption of climate smart agricultural technologies among potato farmers in Kenya: Does entrepreneurial orientation play a role? Sustainable Technology and Entrepreneurship, 1(2), 100017. https://doi.org/10.1016/j.stae.2022.100017
- Ankrah, D., Kwapong, N., Eghan, D., Adarkwah, F., & Boateng-Gyambiby, D. (2021). Agricultural insurance access and acceptability: Examining the case of smallholder farmers in Ghana. Agriculture & Food Security, 10(1), 1–14. https://doi.org/10.1186/s40066-021-00292-y
- Autio, A., Johansson, T., Motaroki, L., Minoia, P., & Pellikka, P. (2021). Constraints for adopting climate-smart agricultural practices among smallholder farmers in Southeast Kenya. Agricultural Systems, 194(103284), 103284. https://doi.org/10.1016/j.agsy.2021.103284
- Azam, M., Qadri, R., Jahangir, M., Khan, M., & Ali, L. (2017). Impact of climate change vulnerabilities on horticultural production. Horticulture International Journal, 1(2), 45–47.
- Baumhardt, R. L., & Blanco-Canqui, H. (2018). Soil: Conservation practices. Encyclopedia of Agriculture and Food Systems, 5(2014), 153–165.
- Caulfield, M., Groot, J. C. J., Fonte, S. J., Sherwood, S., Oyarzun, P., Borja, R. M., Dumble, S., & Tittonell, P. (2020). Live barriers and associated organic amendments mitigate land degradation and improve crop productivity in hillside agricultural systems of the Ecuadorian Andes. Land Degradation & Development, 31(13), 1650–1661. https://doi.org/10.1002/ldr.3558
- Cavanagh, C., Chemarum, A., Vedeld, P., & Petursson, J. (2017). Old wine, new bottles? Investigating the differential adoption of ‘climate smart’ agricultural practices in western Kenya. Journal of Rural Studies, 56, 114–123. https://doi.org/10.1016/j.jrurstud.2017.09.010
- Cochran, W. G. (1977). Sampling techniques (3rd ed.). John Wiley & Sons.
- County Government of Taita Taveta. (2018). County integrated development plan 2018–2022.
- Dasmani, I., Darfor, K., & Abdul-Wakeel, A. (2020). Farmers’ choice of adaptation strategies towards weather variability: Empirical evidence from the three agro-ecological zones in Ghana. Cogent Social Sciences, 6(1), 1–17. https://doi.org/10.1080/23311886.2020.1751531
- Diro, S., Tesfaye, A., & Erko, B. (2022). Determinants of adoption of climate – Smart agricultural technologies and practices in the coffee‑based farming system of Ethiopia. Agriculture & Food Security, 11(1), 1–14. https://doi.org/10.1186/s40066-022-00385-2
- FAO. (2018). FAO’s work on climate. United Nations Climate Change Conference held in Katowice, Poland, between 2nd to 15th December 2018.
- FAO. (2021). Climate-smart agriculture case studies 2021: Projects around the world. Rome. https://doi.org/10.4060/cb5359en
- FAO. (2022). Crops and climate change impact briefs. Climate-smart agriculture for more sustainable, resilient, and equitable food systems. Rome. https://doi.org/10.4060/cb8030en
- Greene, W. H. (2003). Econometric analysis (5th ed.). Pearson Education.
- Horticultural Crops Directorate (HCD). (2020). Horticulture validated report 2019-2020. Kenya.
- Howland, F., Andrieu, N., & Bonilla-Findji, O. (2018). Understanding socioeconomic aspects influencing CSA adoption. Working Paper No. 247 (pp. 1–47).
- International Institute of Tropical Agriculture (IITA). (2016). Qualitative gender evaluation of agricultural intensification practices in northern Ghana.
- Issahaku, G., & Abdulai, A. (2019). Adoption of climate-smart practices and its impact on farm performance and risk exposure among smallholder farmers in Ghana. Australian Journal of Agricultural and Resource Economics, 64(2), 396–420. https://doi.org/10.1111/1467-8489.12357
- Jaetzold, R., Schmidt, H., Hornetz, B., & Shisanya, C. (2010). Farm management handbook of Kenya: Taita-Taveta County (Vol. II). Ministry of Agriculture.
- Jalli, M., Huusela, E., Jalli, H., Kauppi, K., Niemi, M., Himanen, S., & Jauhiainen, L. (2021). Effects of crop rotation on spring wheat yield and pest occurrence in different tillage systems: A multi-year experiment in Finnish growing conditions. Frontiers in Sustainable Food Systems, 5(647335), 1–14. https://doi.org/10.3389/fsufs.2021.647335
- Kafando, B., Pelenguei, E., & Gnedeka, K. (2022). Education and agricultural technology adoption: Evidence from rural India. International Journal of Research in Business Management and Economics, 1(1), 53–74.
- Kaliba, A., Verkuijl, H., & Mwangi, W. (2000). Factors affecting adoption of improved maize seeds and use of inorganic fertilizer for maize production in the intermediate and lowland zones of Tanzania. Journal of Agricultural and Applied Economics, 32(1), 35–47. https://doi.org/10.1017/S1074070800027802
- Kalu, C. A., & Mbanasor, J. A. (2020). Factors influencing the adoption of climate smart agricultural technologies. FARA Research Report, 7(57), 744–753.
- Kassa, B. A., & Abdi, A. T. (2022). Factors influencing the adoption of climate-smart agricultural practice by small-scale farming households in Wondo Genet, Southern Ethiopia. SAGE Open, 12(3), 215824402211216. https://doi.org/10.1177/21582440221121604
- Kenya National Bureau of Statistics (KNBS). (2019). 2019 Kenya population and housing census volume I: Population by county and sub-county (Vol. I). Ministry of Finance and Planning.
- Kenya National Bureau of Statistics (KNBS). (2023). Economic survey 2023. Ministry of Finance and Planning.
- Kurgat, B., Lamanna, C., Kimaro, A., Namoi, N., Manda, L., & Rosenstock, T. (2020). Adoption of climate-smart agriculture technologies in Tanzania. Frontiers in Sustainable Food Systems, 4(55), 1–9. https://doi.org/10.3389/fsufs.2020.00055
- Lanari, N., Liniger, H., & Kiteme, B. P. (2016). Commercial Horticulture in Kenya: Adapting to Water Scarcity. CDE Policy Brief No. 8.
- Leavens, M., & Anderson, C. (2011). Gender and agriculture in Tanzania. Evans School Policy Analysis and Research (EPAR). Policy Brief No. 134 (pp. 1–14).
- Li, H., Huang, D., Ma, Q., Qi, W., & Li, H. (2019). Factors influencing the technology adoption behaviors of litchi farmers in China. Sustainability, 12(1), 271. https://doi.org/10.3390/su12010271
- Lipper, L., Thornton, P., Campbell, B. M., Baedeker, T., Braimoh, A., Bwalya, M., Caron, P., Cattaneo, A., Garrity, D., Henry, K., Hottle, R., Jackson, L., Jarvis, A., Kossam, F., Mann, W., McCarthy, N., Meybeck, A., Neufeldt, H., Remington, T., … Torquebiau, E. F. (2014). Climate-smart agriculture for food security. Nature Climate Change, 4(12), 1068–1072. https://doi.org/10.1038/nclimate2437
- Malhi, G., Kaur, M., & Kaushik, P. (2021). Impact of climate change on agriculture and its mitigation strategies: A review. Sustainability, 13(3), 1318. https://doi.org/10.3390/su13031318
- Mashi, S., Inkani, A., & Oghenejabor, O. (2022). Determinants of awareness levels of climate smart agricultural technologies and practices of urban farmers in Kuje, Abuja, Nigeria. Technology in Society, 70, 102030. https://doi.org/10.1016/j.techsoc.2022.102030
- McKinsey Global Institute. (2020). How will African farmers adjust to changing patterns of precipitation? Climate risk and response. A Case Study of Agriculture in Africa (pp. 1–20).
- Ministry of Agriculture, Livestock and Fisheries (MoALF). (2016). Climate risk profile for Taita Taveta County. Kenya County Climate Risk Profiele Series (pp. 1–23).
- Mittal, S., & Hariharan, V. (2018). Climate risk management mobile-based climate services impact on farmers’ risk management ability in India. Climate Risk Management Journal, 22(2018), 42–51.
- Moranga, L. O. (2016). Analysis of factors influencing tomato farmers’ willingness to adopt innovative timing approaches for management of climate change effects in Taita Taveta County, Kenya [MSc. Thesis]. University of Narobi.
- Motaroki, L., Ouma, G., & Kalele, D. (2021). Conservation agriculture, possible climate change adaptation option in Taita Hills, Kenya. In African Handbook of Climate Change Adaptation (pp. 1331–1351). Cham: Springer.
- Musafiri, C. M., Kiboi, M., Macharia, J., Ng’etich, O. K., Kosgei, D. K., Mulianga, B., Okoti, M., & Ngetich, F. K. (2022). Adoption of climate-smart agricultural practices among smallholder farmers in Western Kenya: Do socioeconomic, institutional, and biophysical factors matter? Heliyon, 8(1), e08677. https://doi.org/10.1016/j.heliyon.2021.e08677
- Mwikamba, J., Otieno, D. J., & Kosura, W. (2022). Factors influencing mobile phone use on climate-smart horticulture in Taita-Taveta County. Kenya. East African Agricultural and Forestry Journal, 86(1–2), 19–24.
- Negera, M., Alemu, T., Hagos, F., & Haileslassie, A. (2022). Determinants of adoption of climate smart agricultural practices among farmers in Bale-Eco region, Ethiopia. Heliyon, 8(7), e09824. https://doi.org/10.1016/j.heliyon.2022.e09824
- Nyambariga, F., Opere, A., Kituyi, E., & Amwata, D. (2022). Historical food security trends in Taita Taveta County: Analysis of past extreme climate events and coping strategies. East African Agricultural and Forestry Journal, 88(4), 252–266.
- Nyambariga, F., Opere, A., Kituyi, E., & Amwata, D. (2023). Climate change scenario projections and their implications on food systems in Taita Taveta County, Kenya. PLOS Climate, 2(6), e0000114. https://doi.org/10.1371/journal.pclm.0000114
- Nyang, J., Mohamed, J., Mango, N., Makate, C., & Wangeci, A. (2021). Smallholder farmers’ perception of climate change and adoption of climate smart agriculture practices in Masaba South Sub-county, Kisii, Kenya. Heliyon, 7(4), e06789. https://doi.org/10.1016/j.heliyon.2021.e06789
- Ogisi, O., & Begho, T. (2023). Adoption of climate-smart agricultural practices in sub-Saharan Africa: A review of the progress, barriers, gender differences and recommendations. Farming System, 1(2), 100019. https://doi.org/10.1016/j.farsys.2023.100019
- Omwakwe, J. A., Chemining’wa, G. N., Esilaba, A. O., & Thuraniraa, E. G. (2022). Macro and micro-nutrient status of selected Kenya soils. East African Agricultural and Forestry Journal, 87(2), 88–98.
- Oostendorp, R., Van Asseldonk, M., Gathiaka, J., Mulwa, R., Radeny, M., Recha, J. W., Wattel, C., & Van Wesenbeeck, L. (2021). Adoption of CSA practices in Nyando Basin, Western Kenya. CCAFS Technical Report. CGIAR Research Program on Climate Change, Agriculture and Food Security (CCAFS).
- Rodgers, Y., & Akram-Lodhi, H. (2018). The gender gap in agricultural productivity in Sub-Saharan Africa: Causes, costs and solutions. UN Women Policy Brief, 11, 1–5.
- Rohila, A. K., Malik, J. S., & Malik, J. S. (2018). Awareness, constraints and prospects of climate smart agricultural practices (CSAP). Journal of Agrometeorology, 20(Special Issue), 167–171.
- Sahu, F. M. (2016). Climate smart horticulture: Converting waste to wealth. International Journal of Science, Environment and Technology, 5(3), 1296–1302.
- Shah, I., Manzoor, M., Jinhui, W., Li, X., Hameed, M., Rehaman, A., Li, P., Zhang, Y., Niu, Q., & Chang, L. (2024). Comprehensive review: Effects of climate change and greenhouse gases emission relevance to environmental stress on horticultural crops and management. Journal of Environmental Management, 351, 119978. https://doi.org/10.1016/j.jenvman.2023.119978
- Shah, F., & Wu, W. (2019). Soil and crop management strategies to ensure higher crop productivity within sustainable environments. Sustainability, 11(5), 1485. https://doi.org/10.3390/su11051485
- Sorvali, J., Kaseva, J., & Peltonen-Sainio, P. (2021). Farmer views on climate change—A longitudinal study of threats, opportunities and action. Climatic Change, 164(3–4), 1–19. https://doi.org/10.1007/s10584-021-03020-4
- Tadesse, B., & Ahmed, M. (2023). Impact of adoption of climate smart agricultural practices to minimize production risk in Ethiopia: A systematic review. Journal of Agriculture and Food Research, 13(100655), 100655. https://doi.org/10.1016/j.jafr.2023.100655
- Taita Taveta County Government. (2023). County integrated development plan 2023–2027.
- Thornton, P. K., Whitbread, A., Baedeker, T., Cairns, J., Claessens, L., Baethgen, W., Bunn, C., Friedmann, M., Giller, K. E., Herrero, M., Howden, M., Kilcline, K., Nangia, V., Ramirez-Villegas, J., Kumar, S., West, P. C., & Keating, B. (2018). A framework for priority-setting in climate smart agriculture research. Agricultural Systems, 167, 161–175. https://doi.org/10.1016/j.agsy.2018.09.009
- Tufa, A., Kanyamuka, J., Alene, A., Ngoma, H., Marenya, P., Thierfelder, C., Banda, H., & Chikoye, D. (2023). Analysis of adoption of conservation agriculture practices in southern Africa: Mixed-methods approach. Frontiers in Sustainable Food Systems, 7(1151876), 1–25. https://doi.org/10.3389/fsufs.2023.1151876
- Twine, E., Rao, E., Baltenweck, I., & Omore, A. (2019). Are technology adoption and collective action important in accessing credit? Evidence from milk producers in Tanzania. European Journal of Development Research, 31(3), 388–412. https://doi.org/10.1057/s41287-018-0158-z
- Upendram, S., Regmi, H., Cho, S.-H., Mingie, J., & Clark, C. (2023). Factors affecting adoption intensity of climate change adaptation practices: A case of smallholder rice producers in Chitwan, Nepal. Frontiers in Sustainable Food Systems, 6, 1016404. https://doi.org/10.3389/fsufs.2022.1016404
- Waaswa, A., Oywaya Nkurumwa, A., Mwangi Kibe, A., & Ng’eno Kipkemoi, J. (2024). Adapting agriculture to climate change: Institutional determinants of adoption of climate-smart agriculture among smallholder farmers in Kenya. Cogent Food & Agriculture, 10(1), 1–19. https://doi.org/10.1080/23311932.2023.2294547
- Weisberger, D., Nichols, V., & Liebman, M. (2019). Does diversifying crop rotations suppress weeds? A meta-analysis. PloS One, 14(7), e0219847. https://doi.org/10.1371/journal.pone.0219847
- Wekesa, B. M., Ayuya, O. I., & Lagat, J. K. (2018). Effect of climate‑smart agricultural practices on household food security in smallholder production systems: micro‑level evidence from Kenya. Agriculture & Food Security, 7(1), 1–14. https://doi.org/10.1186/s40066-018-0230-0
- Wooldridge, J. M. (2013). Introductory econometrics: A modern approach (5th ed.). Cengage Learning. https://doi.org/10.4324/9781351140768-8
- World Bank. (2023). The development, climate, and nature crisis: Solutions to end poverty on a livable planet – Insights from World Bank Country Climate and Development Reports covering 42 economies.
- World Bank. (2024). The welfare cost of drought in Sub-Saharan Africa. Policy Research Working Paper, 10683.
- Yang, S., & Berdine, G. (2015). Negative binomial regression. Southwest Respiratory and Critical Care Chronicles, 3(10), 50–53.