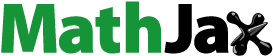
Abstract
Reducing climate-related risks and uncertainties is crucial for the long-term survival of rain-fed smallholder rice farmers in northern Ghana. The study explored the role of psychosocial factors in the adoption and use of sustainable intensification practices to mitigate climate variability among smallholder farmers using the modified Unified Theory of Acceptance and Use of Technologies and Structural Equation Modelling. Employing a mixed methods data were obtained from 240 smallholder rice farmers in the Savelugu municipality in the northern region. The results reveal that there is a strong association between behavioural intention and the number of SIPs adopted by a farmer, supporting the theory of reasoned action based on the assumption that behavioural intention predicts use behaviour contrary to suggestions that the influence of behavioural intention on use behaviour may not be particularly strong or predictable; Facilitating conditions had a direct and positive effect on the number of SIPs adopted by farmers and thus, use behaviour; Performance expectancy and farmers’ attitudes negatively affected behavioural intention while the effect of Social Influence and facilitating conditions on behavioural intension were positive. The results therefore, established the importance of performance expectancy, social influence, facilitating conditions and attitude as predictors of farmers’ intention to adopt SIPs. The study contributes new insights to the adoption literature by presenting empirical data on how frequently underappreciated non-economic factors affect farmers’ adoption decisions in a setting characterised by informal interactions and data quality restrictions.
Introduction
Notwithstanding the potential of socio-economic studies to support the adoption and diffusion of agricultural technology, bottlenecks in the conceptual and empirical framework of current adoption studies reduce their relevance and scope (Brown et al., Citation2017; Doss, Citation2006; Glover et al., Citation2016, Citation2019). Conventional adoption studies have been criticised for their narrow definition of adoption, which essentially excludes the sustained use of technology (Brown et al., Citation2017). The notion that technology adoption only represents the uptake of new technology has often resulted in applying incomplete theories of change to analyse the adoption of agricultural technologies (Loos et al., Citation2014; Struik & Kuyper, Citation2017). It is common to describe technology dissemination interventions in terms of the number of ‘adopters’ of a particular technology which falls short of the actual integration of technologies into the household’s livelihood activities and, eventually, how technologies fit into a given farming system. Furthermore, empirical studies on technology adoption tend to focus narrowly on adoption decisions based on profit maximisation (Borges et al., Citation2015; Dessart et al., Citation2019). The result is that technology adoption is considered a natural progression and that the adoption of new technology is driven mainly by economic motives (Glover et al., Citation2016, Citation2019). However, it must be recognised that farmers’ adoption decisions are mediated by complex social, cognitive, institutional and cultural factors based on heterogeneous and complex incentives, irrespective of the economic benefits (Chouinard et al., Citation2008; Jones-Garcia & Krishna, Citation2021). Garnett and Godfray (Citation2012) note the need to consider the socio-institutional context of technology adoption for a better understanding of inclusive agrarian reform. Behavioural sciences have long suggested that a person’s behaviour is influenced by non-economic and non-intrinsic factors such as attitudes, norms and stewardship motives. However, Mills et al. (Citation2017) indicate that the role of intrinsic factors in influencing farmers’ conservation behaviour has been largely neglected by researchers who focus mainly on the economic factors and their effects on the decision to adopt a technology. The impact of empirical studies on the diffusion of sustainable agricultural intensification practices (SIP) can be enhanced if the social, cultural and economic dimensions are considered. According to Yabi and Omolehin (Citation2009), sustaining the gains from agricultural projects in the long term is related to farmers’ decision to participate and adopt modern technologies, which ultimately affect production systems. By extension, a project’s long-term impact depends on how project beneficiaries perceive the project as being satisfactory or not. Thus, determining project impact purely on technology uptake without considering factors impacting their sustained use in the long term may be necessary but insufficient in determining project impact. This notion is supported by the fact that farmers regularly apply 5 of the 17 sustainable intensification practices introduced in the study area through several interventions over several years. Accordingly, technology adoption is "the long-term integration of technology or component of technology into the family livelihood activities, measured in terms of well-defined and quantitative indicators" (Lotte et al., Citation2015). This suggests combining various criteria, such as biophysical, input, economic, institutional, and cultural contexts, to quantify farmers’ acceptance and usage of agricultural technology.
Rosário et al. (Citation2022) recommend using psychosocial models and their extensions to explain the adoption of sustainable agricultural innovations (Rosário et al., Citation2022). The advent of structural equational models (SEM) has brought the necessary methodological rigour to the study of the influence of psychosocial factors on farmers’ adoption decisions, which has frequently been investigated from behavioural theory perspectives. According to Majidi and Raymond (Citation2022), psychosocial constructs are the result of an individual’s psychological, emotional, and social environment combined. These psychosocial constructs are premised on the idea that human behaviour is influenced by an individual’s desire to engage in a specific behaviour, centring on attitude, behavioural intention, effort expectancy, performance expectancy and social influence. Zeweld et al. (Citation2018) investigated the effect of socio-psychological factors such as social capital, information, attitudes, efficacy, and aversion-affect smallholder farmers’ decisions to adopt sustainable land management practices. Yamano et al. (Citation2013) used psychosocial models to analyse how psychological characteristics such as attitude, subjective norm and perceived behavioural control affected the uptake of agricultural technology.
The study analyses farmers’ adoption decisions from a psychosocial perspective to better understand how psychosocial factors affect the adoption and continued use of sustainable intensification practices (SIPs) among smallholder rainfed rice farmers in northern Ghana. Adoption literature suggests that research on the adoption of agricultural technology, particularly in Sub-Saharan Africa, pays insufficient attention to non-economic and non-intrinsic factors (Borges et al., Citation2019; Zeweld et al., Citation2018). Policies based on economic soully on incentives have failed to increase the adoption of sustainable agricultural practices at farm level (Foguesatto et al., Citation2019). The study, therefore, aims to provide further insights into the adoption literature by presenting empirical data on how frequently underappreciated non-economic factors affect farmers’ adoption decisions in a setting characterised by informal interactions and data quality restrictions.
The study area
The study was conducted in the Savelugu municipality in the Northern Region of Ghana. The municipality has a population of 116,300, with an average household size of 5.8 and a population density of 68.9 persons per sq. km. The area experiences a single rainy season with an average annual rainfall of 600 mm. Like other areas in northern Ghana, the municipality experiences high temperatures averaging 34 °C and ranges from 16 to 42°C. The municipality is mainly rural, with agriculture as the primary source of livelihood. Common crops grown are yam, rice, groundnuts, and cassava. The existence of extensive valleys in the area has made it an important rice-growing hub.
Sampling
Three lowland rice valleys were purposively selected for the study, namely; Nankpanzoo, Yipalsi and Diare. The targeted respondents were smallholder farmers with farm sizes of up to 2.5 ha. Eighty respondents were selected in each community through simple random sampling. Hence, a total of 240 respondents were involved in the study. It is common for rice farmers to own more than one rice plot, in which case the questions focused on the largest rice plot.
Methods of data collection
Focused Group Discussions (FGD) were held once each in Diare, Yipalse, and Nakpanzoo in 2019 to acquire preliminary data on farmers’ perceptions of climate variability and related shocks and mitigation, adaptation and coping techniques utilised by farmers. A second round of FGDs was held in 2020 to examine the kinds of shocks farmers experienced further, farmers’ experience of sustainable intensification practices, strategies for mitigating the effects of climate variability, and to confirm some information obtained from the quantitative data. Each FGD comprised 15 to 20 smallholder rice farmers in the study communities. Information was obtained with the help of a checklist. During the discussion, field assistants recorded information using notebooks and audio recorders. Data obtained were later transcribed, summarised and analysed by the team after the field visit. Key Informant Interviews (KII) were held with major stakeholders with the help of a checklist. Key informants included staff of the meteorological service, the Department of Agriculture, experienced farmers and researchers from the University for Development Studies to obtain background information on previous and ongoing interventions, including the WAGRINOVA project. Quantitative data were obtained using questionnaires administered to the household heads.
Conceptual framework
Models of technology adoption are often employed in studies aimed at predicting and explaining how people will behave when adopting and using new technologies. The theoretical basis of these models is that people’s intention to engage in a specific behaviour determines their actual behaviour, which, in this study, is the intention to adopt SIPs. This study adopts a modified version of the Unified Theory of Acceptance and Use of Technology (UTAUT) to explain farmers’ decisions to adopt SIPs (Dwivedi et al., Citation2019). The UTAUT model integrates various models of technology acceptance and adoption (Williams et al., Citation2015). In its initial form, the UTAUT model suggests that four primary constructs, namely; ‘effort expectancy’, ‘performance expectancy’, ‘social influence’ and ‘facilitating conditions’ determine ‘behavioural intention’ to use new technology and that these constructs are moderated by different variables (Venkatesh et al., Citation2003). In this regard, the UTAUT model offers a robust framework to explain the factors determining the acceptance and use of new technology. Its distinguishing quality is that it is a more comprehensive and integrative model that synthesises several ideas and models while attempting to address their flaws. Additionally, its reliability, stability and viability in technology adoption surveys have been validated in various settings. Compared to previous behavioural models, the UTAUT model, according to Brown and Venkatesh (Citation2005), explains a significant portion of behavioural intention to use technology and effective technology use. Taiwo and Downe (Citation2013) state that the UTAUT model is more reliable than other technological acceptance models in assessing and forecasting adoption. Various studies have employed the UTAUT model to analyse agricultural technology adoption. Using the UTAUT model, Eweoya et al. (Citation2021) established that performance expectancy, effort expectancy, social influence and habit significantly affected behavioural intention to accept and adopt e-agriculture in Nigeria. Studies by Ali et al. (Citation2016) and Okumus et al. (Citation2018) show that the uptake and actual usage of technology is influenced by the intention to use technology to the extent that it captures the motivational aspects that influence an individual’s behaviour and willingness to adopt the technology. Other studies have shown that performance expectancy predicts the intention to adopt a technology (Duck-Boung et al., Citation2020; Okumus et al., Citation2018). Facilitating conditions have been confirmed to contribute significantly to technology adoption (Eweoya et al., Citation2021; Mulugu et al., Citation2020; Okumus et al., Citation2018; Schukat & Heise, Citation2021). Several Authors (Abushanab & Pearson, Citation2007; Ali et al., Citation2016; Mulugu et al., Citation2020; Okumus et al., Citation2018); Eweoya et al., Citation2021) have demonstrated the effect of social factors on technology adoption among farmers. Eweoya et al. (Citation2021) and Engotoit et al. (Citation2016) note that effort expectancy significantly affects technology adoption among farmers. Farmers’ attitude is essential in understanding their adoption decisions (O’Shea et al., Citation2018).
Constructs, definitions and their measurement
The five constructs identified in the model as influencing behavioural intention are: Performance Expectancy (PE), representing ‘the extent to which farmers believe that SIPs will help them improve climate change resilience; Effort Expectancy (EE), representing ‘the ease associated with the use of SIPs; Social Influence (SI) representing ‘the extent to which a farmer perceives that people in their community believe they should adopt SIPs; Facilitating Conditions (FC) representing ‘the measure of a farmer’s belief that organisational and technical infrastructure exists to support the implementation of SIPs’; Attitude (ATT) representing ‘Farmers’ positive or negative feelings about implementing SIPs’ and Behavioural Intention (BI) representing a ‘measure of the strength of a farmer’s intention to adopt SIPs’. The latent variables and how they are measured are indicated in .
Table 1. Constructs, definitions and their measurement.
Performance expectancy (PE)
Onaolapo and Oyewole (Citation2018) define Performance Expectancy (PE) as the degree to which a person believes they will use something to make their life or work more productive. PE is the extent to which people believe technology usage will enable them to perform their jobs efficiently (Chao, Citation2019; Thomas et al., Citation2013). Several studies have demonstrated the significance of PE as a predictor of the acceptance and use of new technology (Alwahaishi & Snásel, Citation2013; Chao, Citation2019; Onaolapo & Oyewole, Citation2018; Thomas et al., Citation2013).
Effort expectancy (EE)
Chao (Citation2019) defines Effort Expectancy (EE) as a person’s expectation of the ease of using technology. According to Bervell and Umar (Citation2017) and Chao (Citation2019), EE is crucial to the acceptance and use of technology. Studies by Lee and Song (Citation2013), Shafinah et al. (Citation2013), and Lwoga and Lwoga (Citation2017) all support this.
Behavioural intention (BI)
Behavioural Intention (BI) is the motivation that influences the intention to act or the degree to which a person is prepared to act (Jaradat & Al-Mashaqba, Citation2014). Several previous studies have highlighted the mediating role of BI in the actual use of technology. Eweoya et al. (Citation2021) and Omar et al. (Citation2022) established that performance expectancy, effort expectancy, and social influenced Behavioural Intention. The process influencing farmers’ adoption behaviour is mediated by behavioural intention, according to Ren and Zhong (Citation2022). Ren and Zhong (Citation2022) have recognised the mediating role of BI in the actual adoption and use of agricultural technology.
Social influence (SI)
Social influence (SI), as defined by Thomas et al. (Citation2013), is the outcome of people being persuaded by other people, usually people in their network, such as friends, family members, and famous people in society, who believe that technology is beneficial. The effect of social influence on users’ intention to accept and use technology has been acknowledged in several studies (de Sena Abrahão et al., Citation2016; Eweoya et al., Citation2021; Liu & Luo, Citation2018; Omar et al., Citation2022).
Facilitating conditions (FC)
Facilitation Conditions ‘reflects an individual’s perception about his or her control over behaviour’ (Venkatesh et al., Citation2008). Generally, FC refers to an individual’s perception of the availability of technological and organisational resources that can remove barriers to using a system (Venkatesh et al., Citation2003). Consequently, FCs are deemed external factors related to the context in which adoption will occur (Taylor & Todd, Citation1995; Çelik, Citation2008; Teo, Citation2009). FCs significantly predict actual use behaviour (Mtebe & Raisamo, Citation2014; Omar et al., Citation2022; Schukat & Heise, Citation2021).
Attitude (ATT)
According to Kaplan (Citation1972), attitude is the propensity to react favourably or unfavourably to an experience. An individual’s assessment of their level of liking or disliking to engage in a given conduct is referred to as their behavioural attitude (Ren & Zhong, Citation2022). Ren and Zhong (Citation2022) have demonstrated that attitude is a significant driver of farmers’ intention to adopt the technology. Zeweld et al. (Citation2017) reports that attitudes influence farmers’ intention to adopt the technology.
As illustrated in , the intention to use technology is influenced directly by performance expectancy, effort expectancy, social influence and facilitating conditions, while use behaviour is influenced directly by the intention to use and facilitating conditions. On the other hand, performance expectancy and effort expectancy influence behavioural intention indirectly and are mediated by their influence on attitude (Dwivedi et al., Citation2019).
Figure 1. Modified UTAUT model.
Source: Dwivedi et al. (Citation2019).

Despite its robustness, it is essential to acknowledge the shortcomings of the UTAUT model, including conceptualising the connection between intention (behavioural intention) and usage (use behaviour). Consequently, "attitude" was modelled as an additional construct. According to Dwivedi et al. (Citation2019), "attitude" has a significant moderating effect on both behavioural intention and use behaviour, partially mediating the effects of exogenous variables on behavioural intention and directly impacting use behaviour. The UTAUT model’s reliance on a limited and often ambiguous understanding of the user is another flaw in the system (El Bilali et al., Citation2021). Therefore, the actual number of technologies that farmers have previously adopted and are still using was used to measure use behaviour to dispel any ambiguity.
Hypotheses
According to Momani (Citation2020), while the actual use of technology depends implicitly on the intention to use, its acceptance and continued use depend on the satisfaction derived from the usage. Consequently, based on this underlying reasoning of the UTAUT model and following Thomas et al. (Citation2013), the study is informed by the following hypotheses:
Hypothesis I
the strength of a farmer’s belief that adopting SIPs will improve climate change resilience will influence their intention to adopt SIPs.
Hypothesis I
I: the strength of farmers’ belief that because other farmers are practising SIPs, they should also adopt SIPs will affect their intention to adopt SIPs.
Hypothesis I
II: the strength of farmers’ belief that the institutional and technical conditionals support the adoption and use of SIPs will affect their intention to adopt SIPs.
Hypothesis I
V: the strength of a farmer’s feelings about the adoption of SIPs will affect their intention to adopt SIPs
Hypothesis
V: the strength of a farmer’s intention to adopt SIPs will affect the number of SIPs adopted by farmers
Hypothesis
VI: the strength of a farmer’s belief that institutional and technical conditions exist to support the adoption of SIPs will directly affect the adoption of SIPs.
Theoretical model
Structural Equation Models (SEM) have found a wide application in social science as they have been instrumental in modelling latent constructs. Generally, SEM is a multivariate method used to estimate a system of simultaneous equations. The method employs regression, factor analysis, path analysis and latent growth curve modelling to determine causality among exogenous and latent variables through a system of simultaneous equations. It provides a helpful way of modelling multiple and complex relationships because it is rigorous and more flexible than linear models due to the following advantages: it allows the modelling of complex dependencies simultaneously; it allows the modelling of causalities between and among unobserved latent variables; it enables the modelling of measurement error terms for both the predictors and outcomes variables; and it allows the decomposition of effects if the direct and indirect effects of the variables are of interest (Stein et al., Citation2012).
SEM comprises two separate models: a measurement model, which estimates the relationship between observed and latent variables, and a structural model, which estimates the relationship among latent variables. Following Bollen (Citation1989), the measurement model may be represented theoretically by the following simultaneous equations:
(1)
(1)
(2)
(2)
(3)
(3)
Where the x’s and y’s are observed indicators for latent variables, the and
are latent variables, the
are factor loadings or regression coefficients, and the
and
are error terms. This is represented in general matrix notation as follows:
(4)
(4)
(5)
(5)
On the other hand, the structural equation model may be represented as below:
(6)
(6)
(7)
(7)
Where and
are factor loadings, the ξ’s are latent variables, and the
’s are error terms. The structural equation may be presented, generally, as follows:
(8)
(8)
Where is an m x n vector of latent endogenous variables,
is an m x n vector of exogenous variables,
is an m x 1 vector of intercept terms, B is an m x 1 vector of coefficients representing the effects of
on each other,
is a vector of coefficients representing the effects of the exogenous variables (
) on
and
is an m x 1 vector of error terms. All the error terms in both models are assumed to be uncorrelated ().
Figure 2. The SEM model.
Source: Stein et al. (Citation2012).

Theoretically, the decision to adopt SIPs may be modelled as follows:
(1)
(1)
This is presented in .
Where INT is the intention to adopt SIPs by farmer ; PE, EE, SI, FC and ATT represent endogenous (latent) variables as defined in ; γ,β,δ, ϒ and ξ represent factor loadings or regression coefficients between constructs (latent variables); ζ1…n, Π1,…,n, θ1,…,n, γ1,….,n and ѱ1,…,n factor loadings between exogenous and latent variables: and Ɛ, µ, Ø, ġ, Ő, and Ş represent error terms.
Issues of model identification, model specification and sample size constitute potential drawbacks to the use of structural equation modelling (Marsh, Citation2007; Schumacker & Lomax, Citation2004). First, at least 200 respondents must be included in the sample for the model to converge (Hu & Bentler, Citation1999). Second, the use of the SEM model relies on the development of testable theories from previous hypotheses. Thus, the relationships between the variables for both the structural and measurement models must be well defined and specified based on sound theoretical foundations to achieve this. Thirdly, a SEM model must be identified to determine whether its parameters are consistent with empirical evidence (Violato & Hecker, Citation2007). A SEM model may be classified as under-identified, just-identified or over-identified. Preferably, the SEM model should be identified. An over-identified model results in positive degrees of freedom and allows for model rejection (Shanmugam & Marsh, Citation2016). These potential limitations were considered to ensure the accuracy and integrity of the findings. The structural model is based on the UTAUT model and was over-identified, while the sample contained 240 respondents.
Results and discussions
Descriptive statistics
Farmers’ perceptions of the various constructs were examined through a 5-point Likert scale namely; ‘strongly agree’, ‘agree’, ‘Undecided’, ‘disagree’ and ‘strongly disagree’ ().
Table 2. Farmer perception of SIP adoption.
Most farmers agreed strongly that practising SIPs helps them improve climate change resilience by enabling them to mitigate the effects of climate variability and increase rice yield. The rest agree that practising SIPs is generally helpful in lowland rice production and will allow them to increase incomes and improve soil fertility. While most farmers agreed that the SIPs are easy to learn, they were inconclusive about their ease of use. Social influence is a strong determinant of the adoption and use of SIPs. Most farmers agreed that they adopted SIPs because people within their network and other community members thought it was right to adopt them. Other factors related to social influence include the fact that extension officers supported the adoption of SIPs. Moreover, early adoption by other smallholder and commercial farmers encourages farmers to adopt SIPs. Regarding the degree to which farmers believe that organisational and technical infrastructure exists to facilitate the implementation of SIPs, while farmers agree strongly that government supports the implementation of SIPs, most farmers disagree equally strongly that they have access to support when they encounter problems while implementing SIPs. Farmers agree that they have acquired the necessary knowledge to implement SIPs. However, they are inconclusive regarding the fact that they have the resources necessary to implement them. Farmers’ attitude towards SIP adoption and use is generally positive. This is supported by the fact that most farmers strongly agree that adopting and using SIPs is a good idea, and they will use SIPs because it enables them to increase their yield. The rest believe that practising SIPs will be interesting. Regarding the strength of a farmer’s intention to adopt SIPs, most farmers agree that they plan to use additional SIPs in the future. However, they were inconclusive regarding whether they will use SIPs immediately during the following seasons.
Overview of sustainable intensification practices in the study area
Farmer knowledge and awareness of SIPs and their role in mitigating the effects of climate variability on crop productivity were assessed (). Farmers demonstrated an impressive understanding of SIPs and their role in mitigating the impact of climate variability. The study area has a long history of support from the government and NGOs regarding the promotion of SIPs. Thus, farmers are familiar with SIPs. However, their use has not been encouraging owing to several constraints. Analysis of constraints to adopting these technologies suggests that the cost of adoption, labour availability and access to inputs and mechanisation are significant impediments to SIP adoption in the study area. Seventeen SIPs were identified in our study based on farmers’ recollections and observed current farm practices. Three out of the 17 SIPs identified in the study area accounted for more than 90% of all SIPs currently used by rice farmers, and their usage is consistent among all farmers. These include; mechanised ploughing (98%), fertiliser application (95%) and weedicide application (93%). The extent of use of the rest is inconsistent and accounts for less than 50% of SIPs employed by farmers. Among these, early planting, pesticide use, bunding, improved varieties, mechanised harvesting, and dibbling accounted for between 40% and 20%. Row planting, conservation tillage, use of improved seed, farm residue retention, late planting, crop rotation, IPM and intercropping accounted for less than 20% of technologies employed by rice farmers.
Table 3. Available SIPs, farmers’ understanding of the role in mitigating effects of climate variability and associated constraints.
Model fit
Based on recommendations by Hu and Bentler (Citation1999), the model fit is determined by a combination of the root mean squared error of approximation (RMSEA) and the Standardised root mean square residual (SRMR) statistics. Both the RMSEA (0.028) and SRMR (0.054) indicate a good model fit ().
Table 4. Model fit statistics.
Test of reliability of the variables
The overall reliability of the measurement scale of the constructs is high, with an overall Cronbach’s Alpha of 0.94. However, the item-test correlation indicates that some measurement items correlated poorly with others and were excluded from the estimation. These include FC4 (0.0168), ATT2 (0.2156), ATT4 (-0.0643), BI1 (0.3408) and BI2 (0.0616) ().
Table 5. Test of reliability.
Although two measurement scale variables for the construct attitude (ATT1 and ATT3) were reliable, they were excluded from the estimation due to model identification issues. On the other hand, the inter-item covariance is 0.3, which is reasonably low. Essentially, the inter-item correlation examines the extent to which one item on a set of scores is related to scores of all other items on a given scale and, therefore, is an indication of the extent to which items on a scale are assessing the same phenomenon (Cohen & Swerdlik, Citation2005). Generally, inter-item correlations of 0.20 to 0.40 are deemed acceptable (Piedmont, Citation2014).
Outputs of the structural equation modelling
The measurement model
The results from the measurement model reveal that all the exogenous variables contributed significantly, at the 1% significance level, to the underlying latent variables and were positive (). This is not surprising as the Alpha Cronbach values indicate a highly reliable measurement scale.
Table 6. Output from SEM (direct effects).
The structural model
The outcome of the structural equation model () demonstrates that behavioural intention significantly and positively influenced SIP adoption at the 1% level. This result is consistent with findings by Jin et al. (Citation2022) regarding Tanzanian farmers’ intentions to use enhanced maize varieties. The number of SIPs adopted increases by 72.6% for every one percent rise in behavioural intention. Facilitation conditions directly and positively influenced the number of SIPs used at the 5% significant level. This is supported by findings by Schukat and Heise (Citation2021), who discovered a similar relationship between facilitating conditions and the actual use of smart items by German Farmers. The number of SIPs adopted increases by 25.8% for every 1% improvement in facilitation conditions. Facilitation conditions also positively impacted behavioural intention at a 5% significant level similar to findings by Jin et al. (Citation2022) and Schukat and Heise (Citation2021). A percentage improvement in facilitating conditions influenced farmers’ intention to adopt SIPs by 44.2%. Performance expectancy influenced behavioural intention strongly and negatively at the 1% significant level. Thus, farmers perceive the current SIPs as largely ineffective in helping them mitigate the effects of climate variability. A percentage increase in performance expectancy is associated with a 66.0% decrease in farmers’ intention to adopt SIPs. In contrast to the findings of this study, Omar et al. (Citation2022) and Eweoya et al. (Citation2021) found a positive relationship between performance expectancy and behavioural intention to adopt agricultural technology. Similar to the findings by Eweoya et al. (Citation2021), the effect of social influence on behavioural intention is positive and at the 1% significant level. A percentage increase in social influence is associated with a 7.4% increase in farmers’ intention to adopt SIPs. Effort expectancy is related negatively to behavioural intention and is marginally significant at the 10% significant level. This suggests that farmers perceive the current SIPs as requiring much effort. A percentage increase in effort expectancy decreases farmers’ intention to adopt SIPs by 27.1%. However, Eweoya et al. (Citation2021) found a positive relationship between effort expectancy and behavioural intention. Contrary to findings by Ren and Zhong (Citation2022), attitude had a negative but significant effect on behavioural intention at the 1% significant level. Thus, a percentage increase in farmers’ negative attitude towards the adoption of SIPs results in an 83.9% decrease in farmers’ intention to adopt SIPs.
Hypothesis testing
The test of hypothesis sought to ascertain whether there is a structural relationship among the latent variables rather than the direction of the relationship. This is because the direction of the relationship is determined by the strength of a farmer’s belief in whether to adopt SIPs which also depends on farmers’ positive or negative experiences. The results establish the importance of performance expectancy (PE), social influence (SI), facilitating conditions (FC) and attitude (ATT) as predictors of farmers’ intention to adopt SIPs (). However, the nature of the influence differed. While performance expectancy and farmers’ attitudes negatively affected behavioural intention, social influence and facilitating conditions positively affected behavioural intention. Behavioural intention (H6) and facilitating conditions (H7) are significant predictors of the number of SIPs adopted by farmers, with path coefficients of 0.73 and 0.26, respectively, and thus support our hypothesis that both influence the number of SIPs adopted by farmers and therefore, use behaviour. The results support all the hypothesised relationships apart from the effects of effort expectancy (H2). Among the factors influencing behavioural intention, the numeric value of the path coefficients of H1 (0.66) and H5 (0.84) are high, indicating a strong association between performance expectancy and farmers’ attitudes and behavioural intention, respectively. The numeric value of the path coefficient of H4 (0.44) was reasonably high, while that for H3 (0.07) was the lowest, indicating a reasonable influence on behavioural intention.
Table 7. Path analysis for hypothesis testing.
Discussion
Farmers adopt and use technologies that best serve their interests at a time. The strong association between behavioural intention and the number of SIPs adopted by a farmer supports the theory of reasoned action based on the assumption that behavioural intention predicts use behaviour (Ajzen & Fishbein, Citation1980; Momani, Citation2020). The results from this study are contrary to suggestions that the influence of behavioural intention on use behaviour may not be particularly strong or predictable (Pardamean & Susanto, Citation2012; Weerakkody et al., Citation2013). The positive effect of facilitating conditions on the number of SIPs adopted by farmers reflects the importance of the government’s subsidy on agricultural inputs and other forms of support provided by NGOs and government projects in the study area over the years. Performance expectancy, social influence, facilitating conditions and attitudes influenced farmers’ intention to adopt SIPs. Performance expectancy has been shown to affect behavioural intention significantly (Barnes et al., Citation2019; Fountas et al., Citation2015; Michels et al., Citation2019). In this study, the effect of performance expectancy and farmers’ attitudes negatively affected behavioural intention, reflecting farmers’ negative experiences with SIPs. Comparatively, a positive attitude towards technology positively influences behavioural intention towards agricultural technology (Dwivedi et al., Citation2019; Ren & Zhong, Citation2022). It is important to note that while the actual use of SIPs implicitly depends on a farmer’s intention to use them, the continuous use of SIPs is premised on their acceptance and farmers’ satisfaction with SIPs (Momani, Citation2020). The negative influence of performance expectancy and attitude on behavioural intention must be placed in context. Whetten (Citation2009) defines contextual effects as the set of factors surrounding the focal phenomenon, in this case, the adoption and use of SIPs, that exerts a direct or indirect influence. In this study, farmers in the study area are aware of SIPs as they have been introduced to several SIPs over the past 40 years. However, they regularly employ an average of five out of 17 SIPs. Farmers’ attitude towards SIPs has been poor as they do not believe that the results are commensurate with the effort of implementing them. For example, farmers believe that row planting and dibbling are labour-intensive and costly and, therefore, are unwilling to practice them. Moreover, farmers prefer broadcasting rice seeds to planting them because of uncertainty about the onset of rains and lack of tractors as they want to maximise their time among several other plots. Thus, farmers’ positive or negative experience will influence their behavioural intention for adopting SIPs positively or negatively. The positive effect of social influence on behavioural intention is consistent with the findings of other studies. Eweoya et al. (Citation2021) established that social influence affects behavioural intention to accept and adopt e-agriculture in Nigeria. Digvijah et al. (Citation2020) found that adopting modern varieties among households is strongly influenced by social networks. Fox et al. (Citation2021) found that social influence affects the acceptance and use of mobile technology by farm enterprises. The positive effect of facilitating conditions on the intention to adopt and use technology is consistent with the favourable input subsidy and other support received by farmers in the study area. Technology adoption is influenced by the environment and context in which it takes place (El Bilali et al., Citation2021). Thus, facilitation conditions shaped by contextual factors are important in explaining technology adoption and sustained usage (Schukat & Heise, Citation2021). Favourable facilitating conditions give farmers the assurance they require to navigate the risks and uncertainties associated with adopting and using technology.
Conclusion and recommendations
The increasingly devastating incidence of climate variability has made it critical for rain-fed smallholder and resource-poor rice farmers in northern Ghana to mitigate the risks and uncertainties associated with rice production. Adopting the optimum mix of technologies is necessary to mitigate the varied and often unpredictable nature of climate variability. This calls for the need to examine the factors that inform farmers’ decisions to adopt and use technology by farmers beyond economic factors. While most studies on adoption decisions by farmers focus mainly on economic and social factors, this study examines adoption decisions from the perspectives of psychosocial factors. The study, anchored on the modified Unified Theory of Acceptance and Use of Technologies, explored the role of psychosocial factors in adopting sustainable intensification strategies to mitigate climate variability among smallholder farmers in the Savelugu district in the northern region. The results established the importance of performance expectancy, social influence, facilitating conditions and attitude as predictors of farmers’ intention to adopt SIPs and the role of facilitating conditions on the actual use of technology. The a strong association between behavioural intention and the number of SIPs adopted by a farmer, supports the theory of reasoned action based on the assumption that behavioural intention predicts use behaviour contrary to suggestions that the influence of behavioural intention on use behaviour may not be particularly strong or predictable. The results also reveal the importance of contextualising theories as context affects the factors surrounding the focal phenomenon that directly or indirectly influences it. In this study, farmers’ previous experience with existing technology was critical in shaping their expectations about the adoption of SIPs. The current favourable conditions ensured by the ongoing input subsidy and sustained support from NGOs and government project over the years has exposed farmers to several SIPs enhancing their expectations and contextual relevance of SIPs, positively impacting the number of SIPs adopted. However, their unmet expectations regarding the efficiency and benefits of SIP adoption negatively affected their adoption and use decisions. The study also reveals that social influence is important in farmers’ SIP adoption and use decisions. Moreover, farmers prefer cultivating multiple plots of the same crops to mitigate climate variability rather than adopting additional SIPs. Due to high labour requirements, these are often considered labour-intensive, economically unrewarding, and sometimes counterproductive. Although farmers agree that SIPs are beneficial, several constraints were identified as hampering the successful promotion and sustained use of SIPs in the study area. The major constraints to adopting SIPs in the study area are labour shortage, access to inputs and mechanisation and lack of capital. The following recommendations are made:
The dynamic and complexity of farmers’ adoption decisions require critically re-examining current methodological approaches. Therefore, the results from this study suggest the need to look beyond socio-economic variables in explaining farmers’ technology adoption decisions.
The extent of decontextualisation and oversimplification of the adoption process is linked intrinsically to the chosen analytical approach. Therefore, adequate contextualisation of technology adoption and use offers valuable lessons and could suggest an alternative narrative of adoption for the applied research
Beyond the adoption of individual technologies, adoption studies should consider the actual and sustained usage of technology as critical to determining the impact of technology adoption.
Disclosure statement
No potential conflict of interest was reported by the author(s).
Additional information
Funding
Notes on contributors
Yakubu Balma Issaka
Mr. Yakubu Balma Issaka is a Senior Lecturer and Director of the Business Innovation and Incubation Centre of the University for Development Studies, Tamale, Ghana. He has previously served as Deputy Director of the UDS Business Directorate and the Head of the Department of Agribusiness Management and Finance, Faculty of Agriculture, Food and Consumer Sciences of the University for Development Studies. I have been key in conceptualising and establishing the Business Innovation and Incubation Centre of the University for Development Studies (UDS). He has extensive experience designing, implementing, monitoring, evaluating, and reporting development programmes, and he has collaborated with several local and international NGOs and the UN. His research interests include rural development, agricultural marketing, natural resource management and entrepreneurship. H has published articles in referred journals, books, chapters in books and conference proceedings.
Samuel A. Donkoh
Professor Samuel A. Donkoh Professor Samuel. Donkoh is a professor of Agricultural Economics. He is currently the Dean of the School of Economics, University for Development Studies (UDS), Tamale, Northern Ghana. He holds BA (Hons) Econs, Dip Ed and MPhil in Economics from the University of Cape Coast, Ghana; and PhD in Agricultural Economics from the University of Reading, UK. He is also a Fellow of the Institute of Chartered Economists of Ghana (ICEG). His research work falls in the domain of Agricultural Economics and Applied Microeconomics. Specific research interests are in Agricultural technology adoption, efficiency and welfare of agricultural households. Professor Samuel A. Donkoh has a strong passion in helping to build a resilient food and cash security system among small-holder farmers in Ghana and across the globe.
Gordana Kranjac-Berisavljevic
Biographical Sketch: Gordana Kranjac-Berisavljevic (PhD) is Professor of Agricultural Engineering in the Department of Agricultural Mechanisation and Irrigation Technology (AMI), where she is working since its establishment. She has served as the Head of Department, Vice-Dean of the Faculty of Agriculture, Dean of Students and Director of International Relationships and Advancement (DIRA) and as Deputy Director of WACWISA Center of Excellence in Irrigation, Drainage and Sustainable Agriculture, funded by the World Bank in 2019. She is serving University for Development Studies, Tamale, Ghana in these various capacities since 1995. She is currently teaching about seven (7) different undergraduate and postgraduate courses across three different Schools and Faculties (FOAFCS, Natural Resources and Engineering) and supervises numerous MPhil and PhD thesis. Her research work covers different areas related to wastewater use in agriculture, feasibility and other technical studies for irrigation projects, weather information provision to small-scale farmers using mobile devices, integration of local and technical knowledge, etc. Prof Gordana has been collaborating with many local and international partners, including World Bank, GEF, GiZ, UNU, EU, USAID, DANIDA, NORHED, JICA and universities and research institutions across the African continent, as well as world-wide. She has published articles in referred journals, books, chapters in books, etc. https://www.researchgate.net/profile/Gordana-Kranjac-Berisavljevic. https://universityfordevelopmentstudies.academia.edu/GordanaKranjacBerisavljevic
References
- Abushanab, E., & Pearson, J. M. (2007). Internet banking in Jordan: The unified theory of acceptance and use of technology (UTAUT) perspective. Journal of Systems and Information Technology, 9(1), 1–17. https://doi.org/10.1108/13287260710817700
- Ajzen, I., & Fishbein, M. (1980). Understanding attitudes and predicting social behaviour: Attitudes. In Intentions and perceived behavioral control. Prentice Hall, Englewood Cliffs, N.J.
- Ali, F., Nair, P. K., & Hussain, K. (2016). An assessment of students’ acceptance and usage of computer supported collaborative classrooms in hospitality and tourism schools. Journal of Hospitality, Leisure, Sport and Tourism Education, 18, 51–60. https://doi.org/10.1016/j.jhlste.2016.03.002
- Alwahaishi, S., & Snásel, V. (2013). Consumers’ acceptance and use of information and communications technology: A UTAUT and flow based theoretical model. Journal of Technology Management & Innovation, 8(2), 9–10. https://doi.org/10.4067/S0718-27242013000200005
- Barnes, A. P., Soto, I., Eory, V., Beck, B., Balafoutis, A., Sánchez, B., Vangeyte, J., Fountas, S., van der Wal, T., & Gómez-Barbero, M. (2019). Exploring the adoption of precision agricultural technologies: A cross regional study of EU farmers. Land Use Policy, 80, 163–174. https://doi.org/10.1016/j.landusepol.2018.10.004
- Bervell, B., & Umar, I. N. (2017). Validation of the UTAUT model: Re-considering non-linear relationships of exogenous variables in higher education technology acceptance research. EURASIA Journal of Mathematics Science and Technology Education, 13(10), 6471–6490.
- Bollen, K. A. (1989). A new incremental fit index for general structural equation models. Sociological Methods & Research, 17(3), 303–316. https://doi.org/10.1177/0049124189017003004
- Borges, J. A. R., Foletto, L., & Xavier, V. T. (2015). An interdisciplinary framework to study farmers decisions on adoption of innovation: Insights from expected utility theory and theory of planned behavior. African Journal of Agricultural Research, 10(29), 2814–2825.
- Borges, J. A. R., Lansink, A., & Emvalomatis, G. (2019). Adoption of innovation in agriculture: A critical review of economic and psychological models. International Journal of Innovation and Sustainable Development, 13(1), 36–56. https://doi.org/10.1504/IJISD.2019.096705
- Brown, B., Nuberg, I., & Llewellyn, R. (2017). Negative evaluation of conservation agriculture: Perspectives from African smallholder farmers. International Journal of Agricultural Sustainability, 15(4), 467–481. https://doi.org/10.1080/14735903.2017.1336051
- Brown, S.A., & Venkatesh, V. (2005) Model of adoption of technology in the household: A baseline model test and extension incorporating household life cycle. Management Information System Quarterly, 29 (3), 399–426. https://doi.org/10.2307/25148690
- Çelik, H. (2008). What determines Turkish customers’ acceptance of internet banking? International Journal of Bank Marketing, 26(5), 353–370. https://doi.org/10.1108/02652320810894406
- Chao, C. M. (2019). Factors determining the behavioral intention to use mobile learning: An application and extension of the UTAUT model. Frontiers in Psychology, 10, 1652. https://doi.org/10.3389/fpsyg.2019.01652
- Chouinard, H., Paterson, T., Wandschneider, P., & Ohler, A. (2008). Will farmers trade profits for stewardship? Heterogeneous motivations for farm practice selection. Land Economics, 84(1), 66–82. https://doi.org/10.3368/le.84.1.66
- Cohen, R. J., & Swerdlik, M. E. (2005). Psychological testing and assessment: An introduction to tests and measurement (6th ed.). McGraw-Hill.
- de Sena Abrahão, R. D. S., Moriguchi, S. N., & Andrade, D. F. (2016). Intention of adoption of mobile payment: An analysis in the light of the unified theory of acceptance and use of technology (UTAUT). RAI Revista de Administração e Inovação, 13(3), 221–230. https://doi.org/10.1016/j.rai.2016.06.003
- Dessart, F. J., Barreiro-Hurlé, J., & van Bavel, R. (2019). Behavioural factors affecting the adoption of sustainable farming practices: A policy-oriented review. European Review of Agricultural Economics, 46(3), 417–471. https://doi.org/10.1093/erae/jbz019
- Digvijah, S. N., Birthal, P. S., Kumar, A., & Tripathi, G. (2020). Farmers’ social networks and adoption of modern crop varieties in India. IFPRI Discussion Paper 1918. International Food Policy Research Institute (IFPRI). https://doi.org/10.2499/p15738coll2.133684
- Doss, C. R. (2006). Analysing technology adoption using microstudies: Limitations, challenges and opportunities for improvement. Agricultural Economics, 34(3), 207–219. https://doi.org/10.1111/j.1574-0864.2006.00119.x
- Duck-Boung, K., Kwang-Jin, C., Yang-Kyu, L., & Min-Uk, J. (2020). A study on the effects of changes in smart farm introduction conditions on willingness to accept agriculture - application of extended UTAUT model. Korean Journal of Organic Agriculture, 28(2), 119–138. Doi: 10.11625/KJOA.2020.28.2.119
- Dwivedi, Y. K., Rana, N. P., Jeyaraj, A., Clement, M., & Williams, M. D. (2019). Re-examining the unified theory of acceptance and use of technology (UTAUT): Towards a revised theoretical model. Information Systems Frontiers, 21(3), 719–734. https://doi.org/10.1007/s10796-017-9774-y
- El Bilali, H. L., Hassen, T. B., Bottalico, F., Berjan, S., & Capone, R. (2021). Acceptance and adoption of technologies in agriculture. AGROFOR, 6(1), 135. https://doi.org/10.7251/AGRENG2101135E
- Engotoit, E., Kituyi, G. M., & Moya, M. B. (2016). Influence of performance expectancy on commercial farmers’ intention to use mobile-based communication technologies for agricultural market information dissemination in Uganda. Journal of Systems and Information Technology, 18(4), 346–363. https://doi.org/10.1108/JSIT-06-2016-0037
- Eweoya, I., Senanu, R., Okuboyejo, S. R., Odetunmibi, O. A., & Odusote, B. O. (2021). An empirical investigation of acceptance, adoption and the use of E-agriculture in Nigeria. Heliyon, 7(7), e07588. https://doi.org/10.1016/j.heliyon.2021.e07588
- Foguesatto, C. R., Borges, J. A. R., & Machado, J. A. D. (2019). Farmers’ typologies regarding environmental values and climate change: Evidence from southern Brazil. Journal of Cleaner Production, 232(2019), 400–407. https://doi.org/10.1016/j.jclepro.2019.05.275
- Fountas, S., Carli, G., Sørensen, C. G., Tsiropoulos, Z., Cavalaris, C., Vatsanidou, A., Liakos, B., Canavari, M., Wiebensohn, J., & Tisserye, B. (2015). Farm management information systems: Current situation and future perspectives. Computers and Electronics in Agriculture, 115, 40–50. https://doi.org/10.1016/j.compag.2015.05.011
- Fox, G., Mooney, J., Rosati, P., & Lynn, T. (2021). AgriTech innovators: A study of initial adoption and continued use of a mobile digital platform by family-operated farming enterprises. Agriculture, 11(12), 1283. https://doi.org/10.3390/agriculture11121283
- Garnett, T., & Godfray, C. (2012). Sustainable intensification in agriculture. Navigating a course through competing food system priorities. Food Climate Research Network and the Oxford Martin Programme on the Future of Food, University of Oxford, 51.
- Glover, D., Sumberg, J., & Andersson, J. A. (2016). The adoption problem; Or why we still understand so little about technological change in African agriculture. Outlook on Agriculture, 45(1), 3–6. https://doi.org/10.5367/oa.2016.0235
- Glover, D., Sumberg, J., Ton, G., Andersson, J., & Badstue, L. (2019). Rethinking technological change in smallholder agriculture. Outlook on Agriculture, 48(3), 169–180. https://doi.org/10.1177/0030727019864978
- Hu, L. T., & Bentler, P. M. (1999). Cutoff criteria for fit indexes in covariance structure analysis: conventional criteria versus new alternatives. Structural Equation Modeling: A Multidisciplinary Journal, 6(1), 1–55. https://doi.org/10.1080/10705519909540118
- Jaradat, M. I. R. M., & Al-Mashaqba, A. M. (2014). Understanding the adoption and usage of mobile payment services by using TAM3. International Journal of Business Information Systems, 16(3), 271–296. https://doi.org/10.1504/IJBIS.2014.063768
- Jin, Y., Lin, Q., & Mao, S. (2022). Tanzanian farmers’ intention to adopt improved maize technology: Analyzing influencing factors using SEM and fsQCA methods. Agriculture, 12(12), 1991. https://doi.org/10.3390/agriculture12121991
- Jones-Garcia, E., & Krishna, V. V. (2021). Farmer adoption of sustainable intensification technologies in the maise systems of the Global South: A review. Agronomy for Sustainable. Development, 41(1), 8. https://doi.org/10.1007/s13593-020-00658-9
- Kaplan, K. J. (1972). On the ambivalence-indifference problem in attitude theory and measurement: A suggested modification of the semantic differential technique. Psychological Bulletin, 77(5), 361–372. https://doi.org/10.1037/h0032590
- Lee, J. H., & Song, C. H. (2013). Effect of trust and perceived risk on user acceptance of a new technology service. Social Behavior and Personality: An International Journal, 41(4), 587–597. https://doi.org/10.2224/sbp.2013.41.4.587
- Liu, H. B., & Luo, X. J. (2018). Understanding farmers’ perceptions and behaviors towards farmland quality change in Northeast China: A structural equation modeling approach. Sustainability, 10(9), 3345. https://doi.org/10.3390/su10093345
- Loos, J., Abson, D. J., Chappell, M. J., Hanspach, J., Mikulcak, F., Tichit, M., & Fischer, J. (2014). Putting meaning back into “sustainable intensification”. Frontiers in Ecology and the Environment, 12(6), 356–361. https://doi.org/10.1890/130157
- Lotte, W., Katrien, D., & Ken, G. E. (2015). Adoptability of sustainable intensification technologies in dryland smallholder farming systems of West Africa. Research Report No. 64. Patancheru 502 324. International Crops Research Institute for the SemiArid Tropics, 84 pp.
- Lwoga, E. T., & Lwoga, N. B. (2017). User acceptance of mobile payment: The effects of user- centric security, system characteristics and gender. The Electronic Journal of Information Systems in Developing Countries, 81(1), 1–24. https://doi.org/10.1002/j.1681-4835.2017.tb00595.x
- Majidi, S., & Raymond, J. K. (2022). Psychosocial responses to telehealth for diabetes care. In David C. Klonoff, David Kerr, Elissa R. Weitzman (Eds.), Diabetes digital health and telehealth (pp. 159–169). Elsevier.
- Marsh, H. W. (2007). Application of confirmatory factor analysis and structural equation modeling in sport and exercise psychology. In G. Tenenbaum & R. C. Eklund (Eds.), Handbook of sport psychology (pp. 774–798). John Wiley & Sons, Inc. https://doi.org/10.1002/9781118270011.ch35
- Michels, M., Bonke, V., & Mußhoff, O. (2019). Understanding the adoption of crop protection smartphone apps: An application of the unified theory of acceptance and use of technology. (No. 1905). Diskussionsbeitrag.
- Mills, J., Gaskell, P., Ingram, J., Dwyer, J., Reed, M., & Short, C. (2017). Engaging farmers in environmental management through a better understanding of behaviour. Agriculture and Human Values, 34(2), 283–299. https://doi.org/10.1007/s10460-016-9705-4
- Momani, A. M. (2020). The unified theory of acceptance and use of technology: A new approach in technology acceptance. International Journal of Sociotechnology and Knowledge Development, 12(3), 79–98. https://doi.org/10.4018/IJSKD.2020070105
- Mtebe, J. S., & Raisamo, R. (2014). Investigating students’ behavioural intention to adopt and use mobile learning in higher education in East Africa. International Journal of Education and Development Using Information and Communication Technology (IJEDICT), 10(3), 4–20.
- Mulugu, L., Birungi, F., Kyazze, P., Kibwika, E., Kikulwe, A., Omondi, B., & Ajambo, S. (2020). Unravelling technology-acceptancefactors influencing farmer use of banana tissue culture planting materials in Central Uganda. African Journal of Science, Technology, Innovation and Development, 12(4), 453–465. https://doi.org/10.1080/20421338.2019.1634900
- O’Shea, R., O’Donoghue, C., Ryan, M., & Breen, J. (2018, August 30–31). Understanding farmers: From adoption to attitudes [Paper presentation]. 166th EAAE Seminar Sustainability in the Agri-Food Sector, Galway Galway, Ireland. National University of Ireland.
- Okumus, B., Ali, F., Bilgihan, A., & Ozturk, A. B. (2018). Psychological factors influencing customers’ acceptance of smartphone diet apps when ordering food at restaurants. International Journal of Hospitality Management, 72, 67–77. https://doi.org/10.1016/j.ijhm.2018.01.001
- Omar, Q., Yap, C. E., Ho, P. L., & Keling, W. (2022). Predictors of behavioral intention to adopt e-AgriFinance app among the farmers in Sarawak, Malaysia. British Food Journal, 124(1), 239–254. https://www.emerald.com/insight/0007-070X.htm https://doi.org/10.1108/BFJ-04-2021-0449
- Onaolapo, S., & Oyewole, O. (2018). Performance expectancy, effort expectancy, and facilitating conditions as factors influencing smart phones use for mobile learning by postgraduate students of the University of Ibadan, Nigeria. Interdisciplinary Journal of e-Skills and Lifelong Learning, 14, 95–115. https://doi.org/10.28945/4085
- Pardamean, B., & Susanto, M. (2012). Assessing user acceptance toward blog technology using the UTAUT model. International Journal of Mathematics and Computers in Simulation, 1(6), 203–216.
- Piedmont, R. L. (2014). Inter-item correlations. In A. C. Michalos (Ed.), Encyclopedia of quality of life and well-being research (Vol. 1, pp. 3303–3304). Springer, Dordrecht. https://doi.org/10.1007/978-94-007-0753-5_1493
- Ren, Z., & Zhong, K. (2022). Driving mechanism of subjective cognition on farmers’ adoption behavior of straw returning technology: Evidence from rice and wheat producing provinces in China. Frontiers in Psychology, 13, 922889. https://doi.org/10.3389/fpsyg.2022.922889
- Rosário, J., Madureira, L., Marques, C., & Silva, R. (2022). Understanding farmers’ adoption of sustainable agriculture innovations: A systematic literature review. Agronomy, 12(11), 2879. https://doi.org/10.3390/agronomy12112879
- Schukat, S., & Heise, H. (2021). Towards an understanding of the behavioral intentions and actual use of smart products among German farmers. Sustainability, 13(12), 6666. https://doi.org/10.3390/su13126666
- Schumacker, R. E., & Lomax, R. G. (2004). A beginner’s guide to structural equation modeling (2nd ed.). Lawrence Erlbaum Associates Publishers.
- Shafinah, K., Sahari, N., Sulaiman, R., Yusoff, M. S. M., & Ikram, M. M. (2013). Determinants of user behavioral intention (BI) on mobile services: A preliminary view. Procedia Technology, 11, 127–133. https://doi.org/10.1016/j.protcy.2013.12.171
- Shanmugam, V., & Marsh, J. E. (2016). Application of structural equation modeling to the social sciences: A brief guide for researchers. Mesure et Évaluation en Éducation, 37(3), 99–123. https://doi.org/10.7202/1036329ar
- Stein, C. M., Morris, N. J., & Nock, N. L. (2012). Structural equation modeling. Methods in Molecular Biology (Clifton, N.J.), 850, 495–512. https://doi.org/10.1007/978-1-61779-555-8_27
- Struik, P. C., & Kuyper, T. W. (2017). Sustainable intensification in agriculture: the richer shade of green. A review. Agronony for Sustainable Development, 37(39), 1–15. https://doi.org/10.1007/s13593-017-0445-7
- Taiwo, A. A., & Downe, L. G. (2013). The theory of user acceptance and use of technology (UTAUT): A meta-analytic review of empirical findings. Journal of Theoretical and Applied Information Technology, 49(1), 48–58.
- Taylor, S., & Todd, P. A. (1995). Understanding information technology usage: A test of competing models. Information Systems Research, 6(2), 144–176. https://doi.org/10.1287/isre.6.2.144
- Teo, T. (2009). The impact of subjective norm and facilitating conditions on pre-service teachers’ attitude toward computer use: A structural equation modeling of an extended technology acceptance model. Journal of Educational Computing Research, 40(1), 89–109. https://doi.org/10.2190/EC.40.1.d
- Thomas, T. D., Singh, L., & Gaffar, K. (2013). The utility of the UTAUT model in explaining mobile learning adoption in higher education in Guyana. International Journal of Education and Development Using Information and Communication Technology, 9(3), 71–85.
- Venkatesh, V., Morris, M.G., Davis, G.B., & Davis, F.D. (2003), User acceptance of information technology: Toward a unified view. MIS Quarterly, 27 (3), 425–478. https://doi.org/10.2307/30036540
- Venkatesh,V., Brown, S.A., Maruping, L.M., & Bala, H.(2008). Predicting different conceptualizations of system use: The competing roles of behavioral intention, facilitating conditions, and behavioral expectation. MIS Quarterly, 32 (3), 483–502. Doi: https://www.jstor.org/stable/25148853 https://doi.org/10.2307/25148853
- Violato, C., & Hecker, K. G. (2007). How to use structural equation modeling in medical education research: A brief guide. Teaching and Learning in Medicine, 19(4), 362–371. https://doi.org/10.1080/10401330701542685
- Weerakkody, V., El-Haddadeh, R., Al-Sobhi, F., Shareef, M. A., & Dwivedi, Y. K. (2013). Examining the influence of intermediaries in facilitating e-government adoption: An empirical investigation. International Journal of Information Management, 33(5), 716–725. https://doi.org/10.1016/j.ijinfomgt.2013.05.001
- Whetten, D. A. (2009). An examination of the interface between context and theory applied to the study of Chinese organisations. Management and Organization Review, 5(1), 29–56. https://doi.org/10.1111/j.1740-8784.2008.00132.x
- Williams, M. D., Rana, N. P., & Dwivedi, Y. K. (2015). The unified theory of acceptance and use of technology (UTAUT): A literature review. Journal of Enterprise Information Management, 28(3), 443–488. https://doi.org/10.1108/JEIM-09-2014-0088
- Yabi, A. J., & Omolehin, A. R. A. (2009). Structural equation modelling of socio-economic determinants of modern technology adoption for sustainable natural resources management in Adja and Nagot Areas of Benin Republic. Journal of Humanities, Social Science and Creative Arts, 4(1), 65–81.
- Yamano, T., Rajendran, S., & Malabayuabas, M. L. (2013, April 8–10). Psychological constructs toward agricultural technology adoption: Evidence from Eastern India [Paper presentation]. 87th Annual Conference, Coventry, UK. Warwick University.
- Zeweld, W., Huylenbroeck, G. V., Tesfay, G., & Speelman, S. (2017). Smallholder farmers’ behavioural intentions towards sustainable agricultural practices. Journal of Environmental Management, 187(2017), 71–81. https://doi.org/10.1016/j.jenvman.2016.11.014
- Zeweld, W., Huylenbroeck, G. V., Tesfay, G., Azadi, H., & Speelman, S. (2018). Impacts of socio-psychological factors on actual adoption of sustainable land management practices in dryland and water stressed areas. Sustainability, 10(9), 2963. https://doi.org/10.3390/su10092963