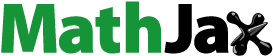
Abstract
Internal moisture generation (IMG), or moisture generated by building occupants via activities such as respiration, cooking, bathing, and cleaning, is a critical input required for design, analysis, and simulation of building enclosure and HVAC systems. Based on previously published values, ASHRAE Standard 160-2021 provides guidance for estimating IMG rates for moisture control design analysis, which is based on occupied home datasets collected in the 1980s and 1990s. Residential energy use simulation software also utilizes estimates for IMG for energy rating, energy analysis, and code compliance calculations, as specified in ANSI/RESNET/ICC Standard 301. Data quantifying IMG rates in newer homes are useful in determining the continued relevance of current design and simulation guidance, and whether the guidance represents conditions found in new housing stock. ASHRAE Research Project 1844, conducted by the Florida Solar Energy Center (FSEC), a research institute of the University of Central Florida, estimated IMG rates in newer occupied homes built since 2013 in the southeastern United States using a moisture balance model approach. The project obtained occupied home data in cooperation with a U.S. Department of Energy Building America research project to characterize indoor air quality (IAQ) in newer U.S. homes, along with presence, functionality, and occupant use of control measures. Full-scale laboratory homes operating with known IMG rates were used to validate a moisture balance model and quantify the accuracy of estimates obtained when the model is applied to data from occupied homes with unknown IMG rates.
Introduction
Internal moisture generation (IMG), or moisture generated by building occupants via activities such as respiration, cooking, bathing, and cleaning, is a critical input required for design, analysis, and simulation of building enclosure and HVAC systems. Other factors influencing the overall moisture load in a home include natural and mechanical ventilation, mechanical humidification/dehumidification, diffusion through building assemblies, and sorption in and out of building materials and furnishings. Based on previously published values, ASHRAE Standard 160-2021 (ASHRAE Citation2021) provides guidance for estimating IMG for moisture control design analysis, which is based on occupied home datasets collected in the 1980s and 1990s. Residential energy use simulation software also utilizes estimates for IMG for energy rating, energy analysis, and code compliance calculations, as specified in ANSI/RESNET/ICC Standard 301 (ANSI Citation2019). Data quantifying IMG rates in newer homes are useful in determining the continued relevance of current design and simulation guidance, and whether the guidance represents conditions found in new housing stock.
ASHRAE Research Project 1844, conducted by the Florida Solar Energy Center (FSEC), a research institute of the University of Central Florida, estimated IMG rates in newer occupied homes built since 2013 in the southeastern United States. Full-scale laboratory homes operating with known IMG rates were used to validate a moisture balance model and to quantify the accuracy of estimates obtained when the model is applied to data from occupied homes with unknown IMG rates. Some of this validation work is described in Martin et al. (Citation2022), and the present article represents an extended version of that previously published conference paper.
ASHRAE Research Project 1844 obtained occupied home data in cooperation with a U.S. Department of Energy Building America research project to characterize indoor air quality (IAQ) in newer U.S. homes, along with presence, functionality, and occupant use of control measures. The Building America IAQ (BAIAQ) study engaged research teams to collect data regionally: Pacific Northwest National Laboratory in Oregon and Colorado, representing marine and cold dry climates (Antonopoulos et al. Citation2023), University of Illinois Urbana–Champaign in Illinois representing a cold climate, and FSEC in Florida, Georgia, and South Carolina representing warm humid climates (Martin et al. Citation2024). Access to study data is forthcoming via a publicly accessible database. All teams used the same base data collection protocol, but the FSEC team added some additional measurements specifically for the purpose of estimating IMG rates, including measurement of air conditioning/dehumidification condensate, and tracer gas testing to better quantify infiltration rates and duct leakage. Therefore, only data from homes in the southeastern United States in which the expanded protocol could be carried out have been used to estimate IMG rates. The BAIAQ project had planned to estimate IMG rates in a limited number of homes, and ASHRAE funding expanded analysis to a greater number of homes.
Background
To quantify IMG in occupied homes, different measurement approaches can be used. One approach is to measure the rate of moisture generated by individual occupant activities. Due to inherent difficulty with this direct form of measurement, another indirect approach that can be used involving measurement of other dominant, non-occupant-related moisture addition and removal mechanisms, and then applying a moisture balance model to calculate an estimated total occupant-related IMG rate. The U.S. Forest Products Laboratory (FPL) prepared a summary of moisture balance models used to predict indoor relative humidity, including a literature review of available data on IMG rates (Glass and TenWolde Citation2009). One finding is that available data relating IMG rates to numbers of occupants vary significantly ().
Fig. 1. IMG rates from literature (Glass and TenWolde Citation2009). IEA values (dashed lines and + symbols) represent measurement and summation of individual internal moisture sources. Other symbols represent whole house estimates calculated with moisture balance models.

One reason the IMG rates vary is the inherent variability in occupant lifestyles (Pallin et al. Citation2017) and interactions with building technologies. Another reason is that relative contribution of individual moisture sources to the total internally generated moisture load may vary over time. For example, the FPL report states that it was previously assumed that clothes washing is a significant source of internal moisture, but it could now be assumed that clothes washing and cleaning can likely be ignored as contributors to internal moisture load in modern homes. The authors of the FPL report call for the need for additional measurements to determine whether current estimates used for IMG rates in design procedures should be adjusted.
Baseline moisture balance model
A baseline moisture balance model was developed for a zone without consideration of diffusion or moisture absorbing surfaces:
(1)
(1)
This equation expresses the rate of change in mass of moisture in the zone ( as a function of moisture added or removed due to air exchange with outdoors (
moisture added due to IMG (
and moisture removed via air conditioning or dehumidification condensate production (
An analytical solution provides an equation for IMG as a function of the other major moisture flows:
(2)
(2)
Unlike IMG, these other moisture flows can be measured with relative ease and accuracy in lab home and occupied home settings. Measurement techniques utilized for the lab homes follow, with similar techniques employed in the occupied homes discussed later in this article. Methods utilized to estimate other moisture flows that are difficult to measure directly, including material sorption and diffusion through building materials, are also discussed.
Lab home experimentation to validate the moisture balance model
For model validation, data were collected in two simulated occupancy lab homes located on the FSEC campus in Cocoa, FL. The first is the Flexible Residential Test Facility (FRTF), a 143-m2 (1536-ft2) single-story, site-built residential structure shown in . The slab-on-grade building was built in 2010 and designed to mimic a typical Florida existing home. It has painted, uninsulated concrete block walls with no exterior cladding, single-pane windows, and a vented attic with shingle roof covering and R-19 kraft-faced fiberglass batt ceiling insulation. The space conditioning system is a SEER 16 two-stage heat pump with air handler located in the garage and flexible ductwork located in the attic. While designed as a three-bedroom, two-bathroom home, the interior floor plan was never built out, resulting in a single well-mixed indoor space. Interior wall modules were constructed with the same type and amount of material as interior walls and placed inside the space for heat and moisture capacitance. Half of the slab floor is left exposed to the space, and half is covered with carpeting and pad.
The second is the Manufactured Housing Laboratory (MH Lab), a 149-m2 (1600-ft2) single-story factory-built home shown in . The three-bedroom, two-bathroom home was manufactured in 2001 and was constructed according to the U.S. Environmental Protection Agency Energy Star Program for manufactured homes. The MH Lab has R-13 wood frame walls with vinyl siding, single-pane windows, a vented attic with R-30 blown cellulose insulation, a sealed crawlspace with R-19 fiberglass batt floor insulation, and a SEER 13 heat pump with indoor-located air handler and duct work. Unlike the FRTF, this home is fully furnished with tables, chairs, couches, and beds, and has carpeting in all rooms except the kitchen and bathrooms, which have tile floor covering.
The internal sensible and latent heat gain schedules were set to approximate the Building America Benchmark hourly schedules (Fang et al. Citation2011). In the FRTF, heat lamps generated sensible internal load, and a calibrated ultrasonic humidifier was utilized to generate internal latent load. In the MH Lab, sensible internal load was generated by a combination of oven operation and heat lamps, and latent internal load was generated in the kitchen area and in the master bathroom by a calibrated system that evaporated water with heat sources. During the course of data collection, the latent gain schedule was periodically adjusted to intentionally simulate varying IMG rates expected in occupied homes. Neither lab has any fan forced air circulation other than that resulting from the operation of the central forced air space conditioning system.
A summary of lab instrumentation and measurements is shown in . Air conditioning condensate from each lab is monitored using tipping buckets that have been calibrated in situ. Measurements of indoor temperature (T) and relative humidity (RH) are used to calculate the humidity ratio (ω).
Table 1. Summary of lab home measurements and instrumentation.
In the FRTF, indoor T and RH were monitored in one central location by a sensor hanging in the middle of the air mass with the thermostat. shows the location of the internal moisture source, and indoor T/RH measurement for the FRTF, overlaid on the designed (but not built-out) interior floor plan.
Fig. 4. FRTF floor plan showing location of internal moisture source and indoor T/RH measurement (interior walls not present in lab).

In the MH lab, indoor T and RH are measured with similar sensors in multiple locations: in the living room near the thermostat, in the utility room, and in each of the three bedrooms. The volume of each space is calculated, and a single humidity ratio representing the whole house is determined using a volume-weighted average. The volume assigned to the central living area measurement includes all spaces not directly monitored. shows the locations of the MH Lab internal moisture sources and indoor T/RH measurements overlaid on the interior floor plan.
Fig. 5. MH Lab floor plan showing location of internal moisture sources and indoor T/RH measurements.

Outdoor T and RH are measured at a weather station in close proximity to the labs and utilized along with a calculated total air exchange rate to determine the contribution of moisture from air exchange with outdoors. To determine the natural infiltration portion of this rate, a blower door test was conducted to determine the flow coefficient and flow exponent for each lab. The FRTF was designed with provisions to adjust envelope leakage, and for these experiments was configured to represent a tighter building with an air changes per hour at 50 pascals value (ACH50) of 2.5. The MH Lab is a leakier building, with ACH50 of 5.0. As discussed later in this article, envelope leakage of occupied homes falls within this range. The flow coefficient and exponent obtained from the blower door results are used along with monitored indoor/outdoor temperatures and wind speed to calculate the wind and stack-driven components of natural infiltration using the ASHRAE enhanced model procedure found in Appendix C of ASHRAE Standard 62.2-2022 (ASHRAE Citation2022). A tracer gas decay test was conducted in each lab and a nonlinear solving method was used to calibrate the natural infiltration model coefficients so that the calculated natural infiltration rate aligned with the one-time measurement of infiltration conducted with a tracer gas decay test. More details on tracer gas testing are given in the occupied home data analysis section of this article.
In the FRTF, the calculated 15-min natural infiltration rate was adjusted to include additional duct leakage induced infiltration using tracer gas measurements conducted with and without the central space conditioning system operating. Additional duct leakage induced infiltration was quantified using this method for each stage of the two-stage 16 SEER heat pump. As the MH Lab has an interior duct system, no duct leakage induced infiltration was included.
Whole house mechanical ventilation (WHMV) was added to each building periodically during the course of experimentation using an unbalanced, continuous point source of supply system. Outside air was introduced directly into the center of the FRTF, and into the utility room of the MH Lab, near the air handler central return. Data collection with and without WHMV was desired as occupied home data collection occurred in homes with and without WHMV operating at ASHRAE 62.2-2010 levels. The WHMV rate was close to ASHRAE 62.2-2010 in each lab building (66 cfm or 31 L/s for FRTF, 57 cfm or 27 L/s for MH Lab). In calculating the total air exchange rate, interactive effects among natural and unbalanced mechanical ventilation were considered using the method described in Appendix C of ASHRAE 62.2-2022.
Lab data was collected from July 2019 to August 2020. shows daily average outdoor dry-bulb and dew-point temperatures in Cocoa, FL. Consistently high summer dry-bulb and dew-point temperatures became variable upon the onset of shoulder season at the beginning of November, with variability lasting until the following summer.
Fig. 6. Outdoor dry bulb and dew point temperatures in Cocoa, FL, during laboratory data collection.

shows daily FRTF indoor conditions during the data collection period. Consistent mechanical cooling kept indoor temperature constant for much of the year. Some cooling load remains throughout each month of the year, as evidenced by the appreciable condensate collection. During the shoulder season beginning in November, air conditioning becomes less consistent, with occasional heating/floating operation resulting from short-term cold fronts. As a result, indoor RH becomes more variable. At the beginning of March, the WHMV system was activated, represented by the orange shaded area, resulting in a significant increase in the total air exchange rate, and a significant increase in condensate production. The correlation between infiltration rate and condensate production is evident, as the WHMV system was toggled off in June, and back on again in August.
shows actual daily IMG in blue, along with the daily value predicted by the baseline moisture balance model in orange. While data are collected in 15-min intervals, a daily time step was used for the analysis because the tipping bucket method of condensate quantification is not always accurate at smaller time steps. This is because condensate produced by the air conditioner does not always immediately drain from the system where it can be measured in real time. It is assumed that summing the condensate on a daily basis is representative of the condensate produced that day. Black circles indicate periods of unquantified window condensation affecting the accuracy of prediction.
Fig. 8. Comparison of actual daily IMG and daily IMG predicted by the baseline moisture balance model.

As seen in , the IMG rate was intentionally varied to simulate a range of conditions expected in occupied homes. The COVID-19 pandemic that began in March of 2020 necessitated that researchers work remotely; therefore, the internal loads were shut down at that time since the labs could not regularly undergo safety inspections. However, data collection continued. The lab was predominantly operated without WHMV, resulting in relatively low daily average air exchange rates. The WHMV system was operated for periods represented by the orange shaded areas seen in .
The overall root mean square error (RMSE) of the prediction over the entire data collection period is 2.6 kg/d (5.7 lb/d). The prediction is consistently biased high during the first summer, indicating an underestimation of moisture sources, or overestimation of moisture sinks. Assuming unexpected measurement error can be ruled out, factors causing the bias may include error in the estimate for natural ventilation rate, and uncertainty regarding the location that the outdoor air infiltrates from. Periodic, short-term tracer gas testing confirms reasonableness for estimates of natural ventilation rate, but the parameter is not directly monitored but rather calculated from monitored temperature and wind data. While the analysis assumes all of the air came from outdoors, with conditions indicated by onsite weather station data, a portion may originate from a different location, such as the attic, which at times may contain more moisture than outdoors. The FRTF has a relatively tight ceiling plane, however, with no full height interior walls to break up the continuous gypsum air barrier. Moisture diffusion from outdoors or the attic may play a role, with possible vapor drive through the slab, through the uninsulated concrete block walls, or through the ceiling insulated with kraft-faced fiberglass insulation.
The overall prediction improves at the beginning of November 2019 with the onset of the swing season; however the prediction gets significantly more variable. At this time of year, with less consistent mechanical cooling, indoor RH becomes highly variable and the need to account for moisture sorption may result in prediction error. While the FRTF is unfurnished, the gypsum drywall, carpet, and exposed slab floor likely play a role in moisture buffering during RH swings. A few events of window condensation on the interior of the single-pane windows, indicated by the black circles in , result in relatively large errors due to unquantified moisture sinks during condensation and unquantified moisture sources during subsequent reevaporation. A reasonable prediction is obtained during the relatively few consistent periods of zero condensate collection, indicating that error in the condensate measurement may be a factor.
Average indoor RH is reduced upon the return to consistent mechanical cooling beginning in March 2020, but remains variable due to the activation of the WHMV system. Error in estimating total air exchange rate is expected to be significantly less during WHMV periods since, unlike during natural ventilation periods, the dominant fan-forced component of the total air exchange is measured and monitored directly. The WHMV results in a marked increase in condensate production. While still variable, the average prediction for IMG rate is reasonable during this period, and not biased high like during the initial naturally ventilated summer period, indicating the possibility that the condensate measurement is likely accurate. WHMV was disabled around the middle of June 2020 and reenabled at the end of the data collection period in August 2020, and similar physical and predicted trends can be observed as in similar previous periods.
Moisture balance enhancements to improve the accuracy of prediction
As stated in the FPL report, moisture balance models mostly differ in terms of how they address the sorption of moisture into and out of building materials and furnishings, a potentially key component to an accurate moisture balance. While it may be justified to neglect sorption if the intent is to model average conditions over long periods (months), when estimating conditions over shorter terms (days, weeks), the effect of sorption can be considerable if indoor RH (and/or vapor pressure) variations take place. Similar to IMG rate, the effect of sorption is also difficult to measure directly. Boardman and Glass (Citation2015) developed a model that included both short- and long-term effects for a study investigating the reduction of stack-driven moisture infiltration by active soil depressurization. Woods, Winkler, and Christensen (Citation2014) experimentally determined model inputs on a whole house basis for the effective moisture penetration depth (EMPD) model, which assumes sorption in shallow and deep material layers and is utilized in the U.S. Department of Energy’s EnergyPlus building energy simulation program.
During periods with constant indoor RH, one may assume that moisture exchange between the home’s air volume and any moisture buffering material in the home is negligible. However, during periods of variable indoor RH, prediction of IMG may be improved via the addition of a moisture buffering term to EquationEquation 1(1)
(1) :
(3)
(3)
Rearranging and solving for IMG rate with the buffering term yields
(4)
(4)
The moisture buffering term requires knowledge of the whole house buffering material effective surface area, mass transfer coefficient, and humidity ratio at the buffering material surface. The humidity ratio at the shallow and deep layers of the buffering material were determined using the two-layer effective moisture penetration depth (EMPD) model (Woods and Winkler Citation2018). The FRTF was one of a few buildings with varying levels of interior furnishings used to experimentally determine whole-house-based inputs to this model including the area of buffering material (Woods, Winkler, and Christensen Citation2014). A value of 162 m2 (1744 ft2) for the surface area of buffering material produced a best fit of data for the FRTF, representing a minimally furnished home, while a value of 504 m2 (5425 ft2) produced a best fit in other houses, representing average furnished homes. Those values have been used for the buffered model enhancement for the FRTF and MH Lab, respectively. Experiments performed by Woods, Winkler, and Christensen (Citation2014) also determined values necessary to estimate the mass transfer coefficient, including depth of shallow and deep layers, slope of the sorption curve, and material vapor permeability The set of equations used in the EMPD moisture buffering model is summarized in .
Table 2. EMPD model equations for whole house moisture buffering estimation.
The surface temperature of the buffering material is also a required input; however, the aforementioned research to determine model inputs obtained the surface temperature from within an EnergyPlus simulation. In the case of our research, we intended to apply the moisture balance model to data from a large number of occupied homes, in which the buffering material temperature is unknown, and accurate EnergyPlus simulations for each home are not possible. As a result, the buffering material temperature was estimated from the average of indoor and outside air temperature by empirically correcting the difference between this average value and the temperature of the buffering material, as shown in EquationEquation 5(5)
(5) :
(5)
(5)
Six temperature correction values (ΔTs) were determined for a range of indoor and outdoor air temperature differences by minimizing the residual between actual and predicted IMG rate from the base model. Values are shown in Appendix A. EnergyPlus simulations were used to inform the range of values expected for surface temperature of various surfaces contributing to the internal moisture buffering in the FRTF.
While the ability of a moisture buffering focused model enhancement to improve the prediction can be explained in physical terms, the unknown surface temperature and resulting empirical determination based on an optimized fit of the model to the data suggest that the resulting correction could also account for any of the previously discussed sources of error, as well as any difference in the rate by which the model responds to moisture sources/sinks versus various lags that likely exist in the physical building. Therefore, a second model enhancement, shown by EquationEquation 6(6)
(6) , was investigated using a linear model approach to correcting errors in significant measured and estimated quantities including indoor and outdoor humidity ratio, condensate, and ventilation rate. Coefficients of EquationEquation 7
(7)
(7) were determined through regression of the moisture generation residual between the actual and predicted values determined by the base model, and are shown in Appendix A.
(6)
(6)
(7)
(7)
shows how both the moisture-buffering-based model enhancement, shown in yellow, and the linear model correction-based enhancement, shown in light blue, improve the prediction of the base model shown in green. Both model enhancements result in similar prediction improvement with the RMSE for the buffering and linear correction enhancements of 1.8 and 1.7 kg/d (4.0 and 3.7 lb/d), respectively, compared to the 2.6 kg/d (5.7 lb/d) obtained with the base model.
Fig. 9. Improved predictions of the moisture buffering based enhancement (yellow) and the linear model based correction (light blue), over the baseline moisture balance model (green).

Additional model enhancements were considered to potentially account for, in physical terms, the overprediction of IMG by the baseline moisture balance model during the first summer and second summer. During these periods, indoor RH is relatively constant, indicating that moisture buffering flux is likely negligible. During these periods, outdoor moisture content is high, and an underestimation of natural ventilation rate could result in the overprediction of IMG. Models that applied a consistent correction factor to natural ventilation rates throughout the entire data collection period were developed, resulting in the desired summertime prediction improvement with negligible impact prediction accuracy during times of the year with lower outdoor moisture content. However, there was no definitive justification for applying a correction factor to the calculated natural ventilation rates, which were spot checked with tracer gas testing.
To arrive at the desired correction, consideration of moisture diffusion was added to the baseline model, considering diffusion through both the wall and the ceiling, shown in EquationEquation 8(8)
(8) . While the effect of moisture buffering may be negligible during the summer months, it may be significant during times of the year with variable indoor RH, and therefore was also added to the diffusion enhanced model. The equation formulation was also changed from humidity ratio to vapor density.
(8)
(8)
The difference between indoor and outdoor conditions are utilized to estimate moisture diffusion flux through both the walls and the ceiling. Attic conditions were not monitored in the occupied homes, and daily average attic moisture conditions are closely approximated by daily average outdoor moisture conditions in vented attics. An analytical solution solving for IMG is included in Appendix B, and yields
(9)
(9)
To determine vapor transfer coefficients for the diffusion and buffering terms, the EnergyPlus combined heat and moisture transfer (HAMT) algorithm was used to inform us on the range of vapor transfer coefficient values anticipated. The EnergyPlus source code was modified to add a vapor transfer coefficients report variable for the HAMT model. The vapor transfer coefficients in the steady-state vapor diffusion model through the assemblies were determined from layer-by-layer combination of vapor diffusion coefficients of the material layers of the assembly construction for each surface type and the air films on both sides the surfaces. The diffusion coefficients through each of the construction layers were determined from vapor permeability assumed for each of the materials of construction. Constant vapor diffusion coefficients were assumed for the air films on both sides of the surfaces.
One of the challenges of working with buffering enhanced moisture balance models has been determining buffering material moisture content. In the previous formulation, the EMPD model was utilized, which required knowledge of the buffering material surface temperature, which had to be estimated. In this new formulation, moisture content of the buffering material was investigated by estimating the vapor density at buffering material surface using a linear model.
Rearranging the preceding equation enables the calculation of surface vapor density using the laboratory’s known IMG rate. In order to utilize the moisture balance with data from occupied homes with unknown IMG rates, the calculated vapor density was fitted to a linear model as a function of variables monitored/measured in both the lab homes and occupied homes. Detailed and simple linear model equations for predicting vapor density at the buffering material surface were formulated and investigated, and are discussed more in Appendix B. Ultimately, a simple model was utilized that expressed surface vapor density as a function of indoor and outdoor moisture:
(10)
(10)
shows how the moisture balance model with the added diffusion enhancement, shown in black, results in an improved RMSE of 2.1 kg/d (4.6 lb/d) compared to the baseline model, shown in orange. Adding the newer surface vapor density moisture buffering formulation, shown in red, improves the RMSE of the prediction to 1.7 kg/d (3.7 lb/d), which is similar to the previous linear correction enhancement.
Fig. 10. The prediction of the diffusion-enhanced baseline model (black) in the FRTF is further improved over the baseline model (orange) with the addition of the new surface vapor density moisture buffering formulation (red).

On average the relative errors obtained are reasonable considering long-term data collection. However in consideration of application of a final model to occupied home data, for this particular research only six days of data are available for each home (further discussion of the occupied home data collection protocol is included in the next section). Over such a short term, model prediction may be poor, depending on a number of factors, including season, whether the home is naturally or mechanically ventilated, and so on. Therefore, RMSE was evaluated using six-day averages of daily IMG to see whether it improved over results obtained with daily data. Results of the six-day averaging are shown in . The overall RMSE for the model with diffusion and buffering improves slightly to 1.3 kg/d (2.9 lb/d). However, the six-day averaging significantly smooths out the magnitude of short-term variability of prediction accuracy under certain conditions. This indicates that when applied to short-term data from occupied homes, IMG predicted for an individual day could have a relatively large error (more than ±5 kg/d, 11 lb/d), but the six-day average IMG is likely to be able to be predicted with reasonable accuracy. RMSE was also evaluated by running the models with six-day averages of all independent input variables, and results were found to be almost identical to those obtained by averaging the daily calculated IMG rate.
Application of the moisture balance and enhancements to data from the MH Lab
A diffusion-enhanced moisture balance was applied to data from the MH Lab collected between September 2019 and July 2020 to determine how the accuracy of the predictions may change when the approach is applied to a different building: in this case a lower mass, less air tight, more heavily furnished home with multiple spaces. For the MH Lab, diffusion through the walls was considered negligible due to the vinyl exterior cladding and vapor-retarding wall sheathing and insulation. However, a diffusion term for the crawlspace floor was added. As with most manufactured homes, the MH Lab does have a vapor barrier “belly board” under the floor; however, its integrity has deteriorated over time. Monitored conditions in the crawlspace and inside the belly of the home were utilized as inputs to the diffusion terms in the model. shows the results for the base model in orange with an RMSE over the period of 8.4 kg/d (18.5 lb/d). It was later discovered that air conditioning condensate had been pooling inside the crawlspace, with diffusion through the floor, resulting in a large source of error during summer months. The model that considers both diffusion and buffering (red) reduces the RMSE to 3.0 kg/d (6.6 lb/d).
Fig. 12. The prediction of the diffusion-enhanced baseline model (black) in the MH Lab is further improved over the baseline model (orange) with the addition of the new surface vapor density moisture-buffering formulation (red).

The models result in a more variable prediction than in the FRTF, presumably due to additional error in calculating natural infiltration rates in this leakier building. shows that the six-day averaging increases the accuracy of prediction with an RMSE of 1.9 kg/d (4.2 lb/d).
Application of the moisture balance to other buildings with unknown parameters
While RMSE for the moisture balance model with enhancements is reasonable when coefficients can be determined with an empirical fit of the models to known IMG rates, the ultimate intent of this research is to validate a model that can be applied to data from occupied homes with unknown IMG rates. Therefore, the resulting models with coefficients empirically determined for one lab were applied to the data from the other lab. shows the result of applying the MH Lab buffering model coefficients to data from the FRTF. Resulting RMSE increases slightly to 1.9 kg/d (4.2 lb/d), up from a value of 1.3 kg/d (2.9 lb/d).
Application of the moisture balance model to occupied home data
Home recruitment
FSEC developed a recruitment strategy and targeted a diverse mix of homes that reflect the general characteristics of newer housing in the warm humid climate region. FSEC’s energy-rated homes database served as the primary source of records to identify homes that met study requirements for recruiting purposes. The database contains detailed information on homes that have undergone an energy audit for which FSEC has performed quality control and archived the results. A similar database maintained by RESNET was used as a secondary source of records. The databases include the address of the home, year of construction, and detailed information on physical characteristics of the home and mechanical systems. The databases do not contain any homeowner contact information or other personally identifiable information. As it is more common for homes that have been built better than code to obtain an energy audit, the databases contain significantly more above-code homes than minimum-code homes.
The main outreach method for recruitment was postcards that were sent to homes identified from the preexisting databases as meeting the general criteria of being built since 2013 in the states of Florida, Georgia, and South Carolina. The postcards directed interested persons to either contact FSEC by phone or e-mail or visit a project website to obtain more information. Homeowners were offered compensation in the form of home improvement store gift cards for their participation. A report summarizing data collected from each home was also provided.
Home characterization
In total, 51 homes were recruited and participated in the BAIAQ study. Forty homes were located in Florida, six in South Carolina, and five in Georgia. This article includes estimates for IMG in 30 of those homes, 23 of which were located in Florida, five in South Carolina, and two in Georgia. shows the geographic distribution of the homes. Recruitment and data collection began in September 2018 and was started/stopped a few different times as a result of the COVID-19 pandemic. Data collection ended in March 2022.
provides a summary of the conditioned area, year of construction, and full-time occupancy for the recruited homes. All homes were single-family detached, and all foundations were slab on grade, with the exception of one slab/stem wall home in SC. Twenty-two homes were single-story and eight homes were two-story. Additional data for each home are shown in Appendix C.
Table 3. Summary of home and occupancy characteristics.
Whole house mechanical ventilation system (WHMV) characterization
Homes with WHMV systems for which source records indicated they met the minimum requirements of ASHRAE 62.2-2010 were targeted for recruitment. This version of the standard was chosen as the benchmark for this study as it is the version required by code and above-code programs for homes in the southeastern United States region, and it is difficult to find a significant number of homes with systems that meet minimum requirements of more recent versions of the standard. As described in Martin et al. (Citation2024), many of the WHMV systems were found to be nonoperational. Problems encountered included failed dampers, disconnected wiring, and maintenance issues. For the systems that were found to be operational, the vast majority were not operating upon arrival, or were operating well below design. In certain homes, the study team obtained permission from the occupants to operate the WHMV system during all or part of the data collection period.
The most common approach found for providing WHMV was via an exhaust fan (EXH). All exhaust WHMV fans encountered were installed in bathrooms, and were also designed for spot exhaust purposes. While most exhaust fans had simple on/off switches, a small number of systems had controls located in a switch that enabled the fan to operate intermittently based on an adjustable time setting and to achieve a minimum hourly run time. Such controls often also enabled the fans to be operated based on indoor humidity and/or occupancy, either independently from or in addition to the time setting. The second most common WHMV system type encountered was central fan integrated supply (CFIS) systems that were configured to use the home’s central forced air heating and cooling system to temper and distribute outdoor air that is pulled in through a ducted connection from outside to the return side of the forced air system. This supply-based WHMV system is typically set up to pull outdoor air through the system using the negative return side pressure generated when the forced air system fan operates. Passive CFIS systems that are “uncontrolled” rely exclusively on the heating/cooling runtime of the forced air system and thus are not ASHRAE 62.2 compliant. Some manufacturers package control and damper systems that invoke additional air handler fan runtime to meet flow targets on an hourly basis. These “controlled” CFIS systems may be programmed via a thermostat or a separate controller that connects to the thermostat.
A limited number of balanced ventilation systems were encountered that combine supply and exhaust fans that are set to operate in unison, balancing pressure between indoors and outdoors. All such systems encountered were energy recovery ventilators (ERV) that enable both heat and moisture to be transferred between incoming and outgoing air streams to retain some of the energy used to condition the indoor air. A limited number of ventilating dehumidifiers (DEHU) were encountered that delivered outside air into the return duct system of the central heating and cooling system. The systems deliver outside air via their own fixed-capacity fans, and operate independently of the central heating and cooling system, with programming that enables them to operate intermittently and deliver a target hourly ventilation rate. Most systems were not configured with capability to dehumidify the main living space and did not have a return duct from the space. The dehumidifier compressor operated based on a customizable humidity set point, but only removed moisture from the outdoor air stream when the humidity in the main living space exceeded the set point during ventilation operation.
Occupied home data collection methodology
The occupied home data collection protocol used for the BAIAQ study, and leveraged for ASHRAE RP-1844, was modeled after an earlier study called the Healthy, Efficient, New Gas Homes study (Chan, 2020) that was conducted by Lawrence Berkeley National Laboratory and collected data in California representing mixed dry climates. The description of the field measurement protocol that follows is not representative of the entire BAIAQ protocol, and only focuses on measurements/activities related to estimating IMG rates.
Each study home was visited multiple times by a two-person field team. On the first visit, the field team:
Explained the study intent and requirements and obtained written consent from participants.
Completed house and heating, cooling, and ventilation system characterization.
Installed devices to measure environmental conditions indoors and outdoors, and installed devices to monitor heating/cooling system operation and use/operation of home features and equipment that affect air exchange with the outdoors, including WMHV systems; kitchen, bath, and laundry exhaust for spot ventilation; and clothes dryers, and exterior doors.
Participants were asked to partake in normal household activities with the exception that windows and doors should not be used for natural ventilation during the data collection period. Most homes were monitored for one week—some with an operating WHMV system, some without. During the final field team visit, all data collection devices were removed, and tests were conducted to quantify airflow of air moving equipment and to characterize building envelope and duct leakage.
Temperature and relative humidity were measured outdoors, and in various locations inside the home. describes measurement equipment, and sampling locations in each home. Wind speed was obtained from the National Weather Service monitoring site geographically closest to each home.
Table 4. Instrumentation for temperature and relative humidity measurements.
The run time of equipment that affects air exchange with the outdoors was monitored utilizing instruments listed in . The position (open or closed) of the patio and garage-to-house doors, along with the door to the master bedroom, was also monitored. Condensate produced by air conditioning and dehumidification systems was collected and quantified by modifying the condensate drain line outside of the home to drain into a tipping bucket.
Table 5. Instrumentation for equipment run time, door status, and condensate production.
A one-time measurement to quantify air flow generated by all equipment generating fan forced-air flows into and out of the home was conducted. Devices utilized for these measurements included a powered flow hood, an exhaust fan flowmeter, and a duct blaster. Except in the case of a few WHMV systems integrated with multi- or variable-speed heat pumps, all fan flows delivered a constant amount of airflow during operation, and therefore a single airflow measurement was sufficient for flow characterization.
Determining outdoor air exchange
Daily average total air exchange was calculated by combining calculated hourly natural infiltration and measured/monitored fan forced ventilation flows according to the method found in Appendix C of ASHRAE Standard 62.2-2022 (ASHRAE Citation2022), considering interactive effects among unbalanced flows, and rolling up to daily values. To determine inputs and coefficients for the natural infiltration model, a Delta-Q test (Walker, Dickerhoff, and Sherman Citation2002) was conducted in each home to measure building envelope and duct leakage, and to determine envelope leakage flow coefficient and exponent. In most homes, short-term tracer gas testing with SF6 was also conducted to yield a secondary measure of infiltration resulting from envelope leakage and duct leakage. Some occupants chose to opt out of this testing. In other homes, time constraints or instrument issues prevented the tracer gas test from being conducted. The tracer gas decay test (ASTM Citation2017) was performed over the course of approximately 1½ h. At the start of the test SF6 was injected with the central space conditioning operating to aid in mixing. Maximum concentrations of no more than 1 ppm of SF6 were targeted as a safety precaution. Concentrations were sampled at multiple locations throughout the house, and once concentration was determined to be well mixed, the SF6 concentration decay was recorded approximately every 10 min. After approximately 40 min of measurements with the central space conditioning system operating, another 40 min of measurements were collected with the central space conditioning system “off.” Typically, tracer gas decay tests are conducted over a minimum of one complete air change. However, the length of time spent inside the homes carrying out the entire data collection protocol limited the amount of time available for tracer gas testing. As mentioned, the testing was conducted for two operating conditions, typically with central space conditioning system “on” and “off,” yielding snapshot estimates of natural infiltration, and additional infiltration resulting from duct leakage. In some instances, one or both of the operating conditions may have included an operating WHMV system, depending on how the home operated during the data collection periods.
As with the lab homes, the flow coefficient and exponent obtained from the Delta Q test were used along with monitored indoor/outdoor temperatures and wind speed to calculate the wind and stack-driven components of natural infiltration using the ASHRAE enhanced model procedure found in Appendix C of ASHRAE Standard 62.2-2022 (ASHRAE Citation2022). For homes with tracer gas results, a nonlinear solving method was used to calibrate the natural infiltration model coefficients so that the calculated natural infiltration rate during the test aligned with the measured result obtained during the test. For homes without tracer gas results, the default model coefficients were used. shows how calculated natural infiltration in one home obtained with coefficients derived from tracer gas testing compares to calculated natural infiltration rates with default model coefficients. compares natural infiltration obtained during tracer gas testing with calculated natural infiltration rates for the same time period.
Fig. 16. Comparison of calculated natural infiltration using default model coefficients and coefficients calibrated through the use of tracer gas testing.

Fig. 17. Comparison of natural infiltration obtained with tracer gas testing and obtained via calculation.

In many homes, tracer gas testing results were also used as a secondary measure of duct leakage to outdoors. For homes where delta Q results indicated a supply or return duct leakage as dominant, the infiltration obtained from tracer gas testing while the space conditioning system was “on” was used to represent duct leakage to outdoors. For homes were delta Q results indicated balanced duct leakage on the supply and return side, the difference in infiltration obtained from tracer gas testing while the space conditioning system was “on” and “off” was used to represent duct leakage to outdoors. For homes that did not undergo tracer gas testing, or tracer gas testing involved operation of a WHMV system, delta Q duct leakage values were used. Select homes had ducts located within a conditioned attic, in which case zero duct leakage to outdoors was assumed.
Application of moisture buffering model enhancements to occupied home data
Data collected from each occupied home were processed and used as input into the baseline moisture balance model. The diffusion enhancement was applied to account for diffusion through ceilings under vented attics only. As all homes were slab on grade, no floor diffusion was modeled. All homes were of newer construction with sufficient vapor resistance from exterior claddings and insulation in the wall assembly; therefore, no wall diffusion was modeled. The moisture buffering enhancement was applied to homes with a swing in daily average indoor RH greater than 5% during the data collection period, utilizing coefficients derived from the MH Lab. The area of whole-house buffering material (m2) was estimated to be 1.3 times the house air volume (m3), which was a typical ratio found in test buildings used by Woods and Winkler (Citation2018). In a sensitivity analysis of inputs to the EMPD model, Woods and Winkler (Citation2018) found that of all moisture buffering material, drywall played the largest role in overall moisture buffering. Experimental homes utilized to assess the model’s ability to predict indoor RH had drywall area-to-volume ratios of approximately 1.3.
Results and discussion
Results are shown in and , and include the calculated IMG rate for each day of the six-day testing period and the average rate for the period. Also included are the ASHRAE Standard 160-2021 guidance, based on the number of occupants, and the ANSI/RESNET/ICC Standard 301 values, based on the conditioned floor area and the number of bedrooms. As seen in the results tables, IMG varies from day to day, as expected, with the variation likely resulting from occupancy and occupant behavior. The number of full-time occupants in each home is listed in the tables; however, there are daily variations in occupancy during the data collection period. These occupancy variations can explain some variations in daily IMG rates. For example, in home 402, the homeowners reported having a party on day 2, likely accounting for the higher daily generation rate. Similarly, for home 414, the homeowners reported having a party and overnight guests on days 4 and 5. There are a number of instances of zero daily IMG in the tables. The calculated result for these days are often slightly negative, but have been truncated to zero in the tables, as negative IMG is not possible. These low daily values may be explained by lower than average occupancy/activity, or possibly by condensate measurement error. Accuracy of condensate collection for occupied homes may occasionally be lower than experienced in the lab homes. While the lab home tipping buckets are permanently installed, the tipping buckets for the occupied homes needed to be custom installed and leveled at each home, and the potential exists for them to be accidentally clogged or bumped by homeowners, pets, and so on.
Table 6. Calculated IMG rates (SI units
Table 7. Calculated IMG rates (IP units).
As a result of the lab home validation showing the potential for larger error in the short-term daily IMG prediction, it is most useful to compare the six-day average generation rate to typical values used for design and simulation. shows statistics for six-day average IMG rates for homes with differing occupancy. compares the six-day IMG averages and standard deviations to ASHRAE Standard 160-2021 values, which assume two occupants for the master bedroom, and one additional occupant for each additional bedroom. The ASHRAE Standard 160-2021 values are 70th percentile values, meaning 70% of homes with a corresponding number of occupants (or bedrooms) are expected to have an IMG rate lower than these values. shows that the ASHRAE Standard 160-2021 values generally fall at the upper end of the standard deviation, which is to be expected considering the standard deviation represents 68% of each sample. Referring back to and , seven of the 30 homes show six-day average IMG rates at or above the ASHRAE Standard 160-2021 rate. Only two homes exceed this rate by 20% or more (414 and 424).
Table 8. Six-day IMG statistics for homes of differing occupancy.
and show similar data based on number of bedrooms instead of number of occupants. In this figure, six-day average IMG estimates are found to fall even further below the ASHRAE 160-2021 values. This is because, as seen in and , the occupied homes from this study generally had fewer occupants per bedroom than what standards tend to assume.
Table 9. Six-day IMG rates for homes of differing numbers of bedrooms.
Table 10. Occupancy statistics for homes with differing numbers of bedrooms.
also compares the six-day average IMG estimates based on number of bedrooms to the ANSI/RESNET/ICC Standard 301 rates, used to represent a typical value for energy simulation purposes. Referring back to and , 12 homes show six-day average IMG rates exceeding the ANSI/RESNET/ICC Standard 301 rates, with four homes exceeding this rate by 50% or more. The average values align very closely. However, one should consider that, as previously stated, the homes from this study generally had fewer occupants per bedroom than what standards tend to assume. Higher occupancy would likely result in six-day average IMG estimates that exceed the ANSI/RESNET/ICC Standard 301 rates.
Except for five homes where the results show low weekly average IMG rates, all homes show six-day average values within the range of values summarized by Glass and TenWolde (Citation2009) and seen in . While detailed error analysis is difficult to accomplish given the complexity of measured and calculated quantities, the laboratory validation estimates the error of the six day-average results to be 1.3–1.9 kg/d (2.9–4.2 lb/d).
Conclusions
ASHRAE Research Project 1844 estimated IMG rates in 30 newer occupied homes built since 2013 in the southeastern United States using a moisture balance model approach applied to six days of collected data in each home. Full-scale laboratory homes operating with known IMG rates were used to validate the moisture balance model and quantify the accuracy of estimates obtained when the model is applied to data from occupied homes with unknown IMG rates. In humid climates, air conditioning condensate, air exchange with outdoors, and diffusion can significantly contribute to an overall moisture balance, especially during summer months. During periods of varying indoor RH, moisture buffering of building materials and furnishings can also be a significant factor. It is difficult to make direct measurements of all moisture fluxes needed to accurately predict IMG rates with a moisture balance. For practicality, some moisture fluxes need to be estimated with indirect measurements, and both physics-based models and empirical models can be employed to estimate unknown parameters. Lab validation showed that the final moisture balance model could predict average IMG rates over a six-day period in the range of 1.3–1.9 kg/d (2.9–4.2 lb/d). Prediction error over the shorter term for individual days could be as high as 5 kg/d (11 lb/d).
IMG was found to vary from day to day in the occupied homes, as expected, likely resulting from occupancy and occupant behavior. As a result of the lab home validation showing the potential for larger error in the short-term daily IMG prediction, it is most useful to compare the six-day average generation rate to typical values used for design and simulation. With the exception of five homes whose results show low weekly average IMG rates, all homes show six-day average values within the range of values summarized by Glass and TenWolde (Citation2009) and seen in .
Seven of the homes show six-day average internal moisture generation rates at or above the ASHRAE Standard 160-2021 rate, which is a 70th percentile value, meaning 70% of homes with a corresponding number of occupants are expected have an internal generation rate lower than this value. Only two homes exceed this rate by 20% or more. ASHRAE Standard 160-2021 values generally fall at the upper end of the standard deviations of the occupied home estimates, which is to be expected considering the standard deviation represents 68% of each sample. Twelve homes show six-day average internal moisture generation rates exceeding the ANSI/RESNET/ICC Standard 301 rate, used to represent a typical value for energy simulation purposes, with four homes exceeding this rate by 50% or more. However, the average values align very closely.
Results indicate that current design guidance for estimating IMG rates based on occupancy provided by ASHRAE Standard 160-2021, while initially based on occupied home datasets collected in the 1980s and 1990s, is still relevant when applied to newer homes. Results also show a trend that typical IMG values used by ANSI/RESNET/ICC Standard 301 for energy simulation purposes are also appropriate. However, it should be considered that the homes from this study generally had fewer occupants per bedroom than what standards assume. Higher occupancy would likely result in six-day average IMG estimates that exceed the ANSI/RESNET/ICC Standard 301 rates.
Nomenclature | ||
A | = | surface area of buffering, walls, ceiling, floor surfaces in the whole house, m2 |
g | = | coefficients of linear model internal moisture generation correction term |
= | vapor transfer coefficient, m/s | |
= | product sum of surface area and vapor transfer coefficient, m3/s | |
= | internal moisture generation rate of the whole house, kgH2O/s | |
= | internal moisture generation rate of the whole house with buffering term, kgH2O/s | |
= | internal moisture generation rate correction, kg/s | |
= | moisture removal rate by the air handling unit, kgH2O/s | |
= | ventilation plus infiltration air volume flow rate, m3/s | |
= | volume of air in the conditioned space house, m3 | |
= | time, s | |
= | time step, s | |
= | saturation pressure of water vapor at a given temperature, Pa | |
= | total pressure of the ambient, Pa | |
R | = | gas constant of water vapor, 461.52 J/(kg⋅K) |
T | = | temperature of air or buffering material, K |
= | temperature difference used to estimate buffering material temperature, K | |
= | thickness of the surface layer of the EMPD model, m | |
= | thickness of the deep layer of the EMPD model, m | |
= | water vapor permeability of buffering material, kg/(m⋅s⋅Pa) | |
= | density of buffering material, kg/m3 | |
= | density of air, kg/m3 | |
= | relative humidity (–) | |
= | slope of moisture sorption curve of buffering material, kgH2O/m3 | |
= | vapor density of moist air, kgH2O/m3 | |
= | vapor density of mist air in the conditioned space of the house, kgH2O/m3 | |
= | vapor density of outdoor air, kgH2O/m3 | |
= | vapor density of moist air at the buffering material surface, kgH2O/m3 | |
= | vapor density of moist air in the attic space, kgH2O/m3 | |
= | vapor density of moist air in the crawl space, kgH2O/m3 | |
= | mass transfer coefficient, kg/m2 s | |
= | total (ventilation plus infiltration) air mass flow rate, kg/s | |
= | mass of the air in the whole house, kg | |
= | humidity ratio of air node of the whole house, kgH2O/kgair | |
= | humidity ratio of outdoor air, kgH2O/kgair | |
= | humidity ratio of air at the surface of moisture absorbing material, kgH2O/kgair | |
= | humidity ratio of air at deep layer of buffering material, kgH2O/kgDryAir | |
= | time step |
Disclosure statement
The authors report there are no competing interests to declare.
Additional information
Funding
References
- ANSI. 2019. ANSI/RESNET/ICC 301-2019, standard for the calculation and labelling of the energy performance of dwelling and sleeping units using an Energy Rating Index.
- Antonopoulos, C., S. Rosenberg, H. Zhao, I. Walker, W. Delp, W. Chan, and B. Singer. 2023. Mechanical ventilation and indoor air quality in recently constructed U.S. homes in marine and cold-dry climates. Building and Environment 245 (2023):110480. doi:10.1016/j.buildenv.2023.110480
- ASHRAE. 2021. ASHRAE 160-2021, criteria for moisture-control design analysis in buildings.
- ASHRAE. 2022. ASHRAE 62.2-2022, ventilation and acceptable indoor air quality in residential buildings.
- ASTM. 2017. ASTM E741-11, standard test method for determining air change in a single zone by means of a tracer gas dilution. Philadelphia: American Society for Testing and Materials.
- Boardman, C. R., and S. Glass. 2015. Basement radon entry and stack driven moisture infiltration reduced by active soil depressurization. Building and Environment 85 (2015):220–32. doi:10.1016/j.buildenv.2014.12.008
- Fang, X., D. Christiansen, G. Barker, and E. Hancock. 2011. Field test protocol: Standard internal load generation for unoccupied test homes. NREL/TP-550-51928. Golden, CO: National Renewable Energy Laboratory.
- Glass, S., and A. TenWolde. 2009. Review of moisture balance models for residential indoor humidity. 12th Canadian Conference on Building Science and Technology, Montreal, QC, 2009, 231–46.
- Martin, E., T. Khan, D. Chasar, J. Sonne, and C. Withers, Jr. 2024. Ventilation and indoor air quality in recently constructed U.S. homes: Measured data from select southeastern states. United States. doi:10.2172/2309736
- Martin, E., B. Nigusse, C. Withers, Jr., and T. Khan. 2022. Lab home validation of a moisture balance model to estimate internal moisture generation rates in occupied homes. Conference Paper for 2021 ASHRAE Buildings XV Conference, Tampa, FL, December 2022.
- Pallin, S., P. Boudreaux, S. J. Jo, M. Perez, and A. Albaugh. 2017. Simulations of indoor moisture generation in U.S. homes. In Advances in hygrothermal performance of building envelopes: Materials, systems and simulations, ASTM STP159, ed. P. Mukhopadhyaya, 261–90. West Conshohocken, PA: ASTM International.
- Walker, I. S., D. J. Dickerhoff, and M. H. Sherman. 2002. The Delta Q method of testing the air leakage of ducts. 2002 ACEEE Summer Study on Energy Efficiency in Buildings, Pacific Grove, CA, August 2002.
- Woods, J., and J. Winkler. 2018. Effective moisture penetration depth model for residential buildings: Sensitivity analysis and guidance on model inputs. Energy and Buildings 165 (2018):216–32. doi:10.1016/j.enbuild.2018.01.040
- Woods, J., J. Winkler, and D. Christensen. 2014. Using whole house field tests to empirically derive moisture buffering model inputs. National Renewable Energy Laboratory NREL/TP-5500-62456. August 2014.
Appendix A.
Temperature correction values (
Ts Equation 4) and internal moisture generation correction coefficients (for 
in Equation 5)
Table A1. Vapor density at buffer material surface prediction detail linear model coefficients (SI).
Table A2. Coefficients of internal moisture generation correction linear equation model (SI).
Appendix B.
Analytical solution to estimate IMG with diffusion and application of linear model to estimate contribution of moisture buffering
The whole house moisture balance equations formulated using vapor density with and without moisture buffering term are given by
These two equations are first-order differential equations with constant coefficients and can be represented in a simplified form as follows:
If there is a moisture buffer term the constant coefficients of the equation are given by
If there is no moisture buffering term, then the constant coefficients of the equation are given by
The general analytical solution for this differential equation is as follows:
Substituting for a and b coefficients and solving for internal moisture generation rate yields
The whole house internal moisture generation rate depends on knowledge of the whole house buffering material and exterior envelope diffusion surface areas, moisture transfer coefficients, vapor density at buffering material surface, and the boundary conditions that include outside air, crawl space, and the attic where applicable. In this investigation the attic air condition was replaced with outside air condition, assuming the attic is fully vented.
Setting the moisture buffer term Ah ̅buff to zero the whole house base case (without the buffering term) internal moisture generation rate becomes
One of the challenges of the internal moisture generation prediction model has been estimation of buffering material moisture content. In a previous attempt an EMPD model to estimate moisture dynamics of buffering materials was investigated, which also required knowledge buffering material temperature history. In this alternative approach, which includes diffusion through the envelope, moisture content of the buffering material was investigated by estimating the vapor density at the buffering material surface using a linear model and is described next.
Rearranging the internal moisture generation prediction equation and solving for the vapor density at the buffering material surface yields
The vapor density at the buffering surface can be determined using the preceding formula from a long-term laboratory monitored dataset that includes boundary conditions (conditioned space air, outside air, attic space air, crawl space air), calculated infiltration air flow rate per ASHRAE 62.2, measured ventilation air flow rate, measured internal moisture generation rate, measured condensation, and assumed vapor transport coefficients for the walls, ceilings, and floors assemblies of the FSEC laboratory homes. The estimated vapor density was fitted to a linear model as a function of characteristics of the two FSEC’s laboratory homes. A detailed and simple linear model equation for predicting vapor density at the buffering material surface was formulated and investigated.
Detailed linear model for predicting vapor density at the buffering material surface consists of known parameters and measured variables, including actual internal moisture generation rate, is given by
The constant coefficients of the detailed linear model equation are provided in . A simple linear model for predicting buffering material surface vapor density consisting of whole house ventilation and infiltration air flow rate and indoor and outside air vapor densities as input variables is given by
The coefficients of the simple linear model equation are provided in . The simple model was intended for field home application where detailed measured data may not be available.
Table B1. Vapor density at buffer material surface prediction detail linear model coefficients (SI).
Table B2. Vapor density at buffer material surface prediction simple linear model coefficients (SI).
Appendix C.
Additional home-specific data
Table C1. Additional home-specific data (SI).
Table C2. Additional home-specific data (IP).