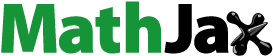
ABSTRACT
The use of data and analytics in professional football organisations has grown steadily over the last decade. Nevertheless, how and whether these advances in sports analytics address the needs of professional football remain unexplored. Practitioners from national federations qualified for the FIFA World Cup Qatar 2022™ and professional football clubs from an international community of practitioners took part in a survey exploring the characteristics of their data analytics infrastructure, their role, and their value for elaborating player monitoring and positional data. Respondents from 29 national federations and 32 professional clubs completed the survey, with response rates of 90.6% and 77.1%, respectively. Summary information highlighted the underemployment of staff with expertise in applied data analytics across organisations. Perceptions regarding analytical capabilities and data governance framework were heterogenous, particularly in the case of national federations. Only a third of national federation respondents (~30%) perceived information on positional data from international sports data analytics providers to be sufficiently clear. The general resourcing limitations, the overall lack of expertise in data analytics methods, and the absence of operational taxonomies for reference performance metrics pose constraints to meaningful knowledge translations from raw data in professional football organisations.
Introduction
Performance science professionals are now fully integrated as part of backroom staff in modern football organisations (Gregson et al. Citation2022) to support the optimisation of strategies relevant to aspects of athlete performance management, talent identification and medical service provision (Bartlett and Drust Citation2021). Like other industries, football is currently undergoing significant transformation which has led to substantial growth of resources, expertise, and data generation (Robertson Citation2020). The role of the sports scientist has become, therefore, even more prominent for translating raw data sources or existing data analysis outcomes into actionable insights to address the practical demands of different stakeholders within the modern sports organisations (Bartlett and Drust Citation2021).
Data analytics has gained increasing importance for sports scientists to develop systematic analysis frameworks for organisations in football (Windt et al. Citation2021), to remain competitive on both a sporting and financial level (Alamar Citation2013; Gregson et al. Citation2022). The notion of sports analytics (Alamar Citation2013) generally refers to ‘the management of structured historical data, the application of predictive analytic models that utilise that data, and the use of information systems to inform decision makers and enable them to help their organisations in gaining a competitive advantage on the field of play’. Alamar (Citation2013) provided a thorough definition identifying data ecosystem and information system as the main components of any contemporary data analytics infrastructure devoted to streamlining decisions of coaching, recruitment, performance support, medical, administration, and operations staff within the core service provision (Gerrard and Alamar Citation2014; Gamble et al. Citation2020; Bartlett and Drust Citation2021). In sports and other fields, the data ecosystem shall denote the process combination of corporate processes ‘to organise, centralise, and streamline how data comes into the team and is processed within the team’s various functions’ (Alamar Citation2013).
In this context, data standardization and centralization represent the foundations of a data ecosystem. Standardization shall denote a pragmatic definition of names, description, and forms for each piece of raw data common throughout the sports organisation (Alamar Citation2013). Centralization refers to the organisation of different quantitative (e.g., game-performance statistics) and qualitative (e.g., medical reports, multimedia information) data sources into an organisation-specific database (Alamar Citation2013). To support these processes, the nature of the organisational structure and staff employment with expertise to deliver applied data solutions is central to any sports analytics infrastructure (Alamar Citation2013). Also, we shall distinguish the functions of data engineers and statisticians/analysts (Alamar Citation2013). A data engineer contributes to the creation of the data infrastructure or ecosystem for a structured management of different data sources, whereas a statistician/analyst delivers business solutions to translate raw data into actionable information (Alamar Citation2013). Despite data management playing a pivotal role for analytics, previous explorations revealed human resource limitations within the modern organisation not only for raw data organisation but also for developing information systems for generating actionable insights (Alamar Citation2013).
With the data management infrastructure being at the one end of the information dissemination spectrum (Delen and Demirkan Citation2013), an information system, on the other, consists of standardized interfaces that can support staff at a football organisation in facilitating the realization of business objectives (Alamar Citation2013). The importance of an information system stems from the need to have flexible and time-efficient access to the reporting of trends, description of patterns, and elaboration of data to leverage actionable insights (Alamar Citation2013; Delen and Demirkan Citation2013). The design of a practical information system requires contextual knowledge, with any set and level of information structured in a logical fashion (Alamar Citation2013). Formalisation of an information system addresses, in practice, problems of knowledge translation within a football organisation (Alamar Citation2013; Bartlett and Drust Citation2021). Accordingly, it was highlighted that the landscape in which sports scientists and performance analysts operate in modern football and other sports organisations demands support from expertise in computer sciences (Robertson Citation2020; Goes et al. Citation2021). For example, integration of domain knowledge from sports sciences and computer sciences is a relatively recent phenomenon in football that aims to leverage fine-grained analyses of player tracking data from match-play (Bornn et al. Citation2018; Jayal et al. Citation2018; Goes et al. Citation2021). A previous investigation surveying professional teams from the NBA, MLS, MLB, and English Premier League revealed that 37.5% did not have a dedicated database programmer, and 20% did not have an analyst on the sports side of the organisation (Alamar Citation2013). With this in mind, technical features and heterogeneity of jargon inherent to player tracking and event data may also pose barriers that can limit meaningful knowledge translation from raw data to coaches and support staff (Mackenzie and Cushion Citation2013). However, despite the ongoing metamorphosis of data analytics in the football industry (Jayal et al. Citation2018) and other sports (Alamar Citation2013; Ward et al. Citation2019), no investigation has provided a contemporary overview of the current state of data ecosystems and information systems in modern football organisations.
In this context, a recent survey examined issues of data management processes in professional clubs and national federations from a general perspective (Gregson et al. Citation2022). Importantly, insights from this study highlighted a general lack of expertise in deploying information technology solutions, given the general reliance on off-the-shelf solutions across the football organisations which took part in the survey (Gregson et al. Citation2022). Considering this and the growing appreciation of embedding data analytics solutions within sports organisations (Alamar Citation2013; Delen and Demirkan Citation2013; Ward et al. Citation2019; Robertson Citation2020; Windt et al. Citation2021; Bauer et al. Citation2023), further exploring such aspects and any potential limitations in current processes requires a more in-depth examination of operational frameworks and practices concerning data analytics service provision in professional football organisations. To address recent insights regarding key operational processes delivered in professional football organisations (Gregson et al. Citation2022), we sought to gather information concerning whether current data analytics ecosystems address the needs of support staff.
Materials and methods
Survey design and distribution
On the occasion of an international conference, practitioners working in male football were invited to take part in a cross-sectional survey exploring issues relating to the development of data analytics infrastructures and information systems in professional football organisations. In keeping with examples from the clinical realm (Cook et al. Citation2019), the targeted conference represented an occasion to facilitate the recruitment of representatives that could provide perspectives relevant to an in-depth examination of operating frameworks and practices for data analytics service provision in place at top-tier professional football clubs and national federations at the time of the study. Target delegates represented the 32 national federations qualified for the FIFA World Cup Qatar 2022TM (FIFA), and top-tier professional football organisations part of the Aspire in the World Fellows (Ford et al. Citation2020). The Aspire in the World Fellows is an international community of practice involving professional football organisations from around the world (Ford et al. Citation2020). The survey was developed by a panel of 6 co-investigators involving academics and practitioners with five or more years of experience working in professional football. Existing work in the field of sports analytics (Alamar Citation2013) and sports sciences (Ward et al. Citation2019; Goes et al. Citation2021) informed the survey design with questions covering specific areas on: i) personal and demographics information (4 items), ii) description of data ecosystems, or the actual platform where the information resides (Delen and Demirkan Citation2013) in modern football organisations (3 items), iii) the general importance of using analytics interfaces (Delen and Demirkan Citation2013) that can support staff at modern football organisations in the elaboration of information that can leverage actionable insights (10 items), iv) the value of modern analytics processing of positional data to support decision-making of coaches (6 items). Considering academic experience in similar survey investigation planning (Ford et al. Citation2020; Gregson et al. Citation2022; Lundqvist et al. Citation2022) and years of experience in professional football, the final survey version in the English language (Supplementary File 1) was reviewed and piloted for clarity and consistency among the present study co-investigators and members of the present study collaborating institutions. The survey was available in Arabic, French, German, Portuguese, and Spanish languages. Translations were conducted by native speakers via a verbatim adaptation approach faithful to the original English version with the consistency of each alternative version illustrated point-by-point in Supplementary Files 2–6. The piloting process resulted in minor amendments of text relevant to a given question, clarifications of response options made available to target respondents, and useful to assess whether translations required necessary improvements for clarity where applicable in the survey. Questions involved multiple choice, simple multiple choice (yes/no), checkbox, numerical, or rating formats. The survey was created using the online software SurveyMonkeyⓇ (Momentive Inc., USA), and disseminated to organisation representatives via an email containing a weblink with information on survey purpose, followed by instructions for survey completion. Delegates received an electronic copy of the questionnaire a week prior to the conference to gather all the information necessary to complete the survey from the relevant colleagues at their organisations if unknown. Only delegates from national federations qualified for the FIFA World Cup Qatar 2022TM who consented to participate in the investigation completed section iv) given this target sample characteristics involving respondents with, mainly, coaching and performance analysis backgrounds relevant to explore further aspects beyond general operational frameworks and practices. Text-based fields for role titles were verified against relevant information regarding delegates attendance to the target conference for survey data collection. The survey weblink was opened and shared on 3rd October 2022 and closed on 4th October 2022. We administered the survey separately for the FIFA and Aspire in the World Fellows samples on a purely conceptual basis, considering the underlying study purpose and within-respondent pool differences in organisation-type composition between professional clubs and national federations. The reason for a clear distinction in the survey administration between samples also rests on the objective to describe the state-of-the-industry with reference to a particular event in time similar to the design of previous studies addressing different research questions (McCall et al. Citation2022). This study was approved by the Aspire Zone Foundation Institutional Review Board, Doha, State of Qatar (protocol number: E202209040).
Statistical analysis
The FIFA and Aspire in the World Fellows samples were examined separately. Responses from the Aspire in the World Fellows were distinguished for professional clubs and national federations. Results were presented as descriptive statistics (Amrhein et al. Citation2019), and by organisation type where applicable. Frequency analysis was conducted for participant characteristics, multiple choice, checkboxes, ranking, Likert-type, and rating-scale questions, with the results presented as percentage of respondents and frequency count. We calculated the response rate as the number of organisations whose delegate consented to participate divided by the total number of eligible organisations we invited to take part in the survey. We described results using qualitative terms assigned to determine the magnitude of the observed frequencies as follows: All = 100% of respondents; Most = ≥75%; Majority = 55 to 75%; Approximately half = ~50%; Approximately a third = ~30%; Minority = <30% (Starling and Lambert Citation2018). Responses involving a numerical answer in single questions (i.e., count data) were presented as median and interquartile range (IQR). All statistical analyses were performed using R (version 3.6.3, R Foundation for Statistical Computing).
Results
Respondents
The participants who completed the questionnaire from the FIFA and Aspire in the World Fellows samples were employed in a range of different roles, involving department directors, heads of department and practitioners with mainly sports sciences, strength & conditioning, medical, and technical backgrounds, respectively.
Of the 32 organisations from the FIFA sample, delegates from the 29 national federations completed the questionnaire. The survey involved 9 Performance Analysts, 4 Football coaches, 3 Heads of Performance, 3 Strength & Conditioning Coaches, 2 Heads of Data Analytics, 2 Heads of Research and Innovation, 2 Technical Directors, and 4 staff members with other roles in their organisations, with a response rate of 90.6%. Respondents had a median experience in professional football of 15 years (IQR, 12 to 20 years), and they had worked at their current federations for 4 years (IQR, 2 to 7 years).
Of the 48 organisations from the Aspire in the World Fellows, 32 clubs and 5 national federations not qualified for the FIFA World Cup Qatar 2022TM agreed to take part in the survey, with a response rate of 77.1%. Overall, the survey involved 9 Heads of Performance, 6 Sports Scientists, 6 Strength & Conditioning Coaches, 5 Technical/Academy Directors, 2 Academy Coordinators, 2 Performance Coordinators, 2 Medical Directors, 2 Medical Doctors, and 3 staff members with coaching roles in their organisations. Respondents from the 32 professional clubs had a median experience in professional football of 14 years (IQR, 9 to 17 years), and they had worked at their current federations for 4 years (IQR, 2 to 8 years). Descriptive information relevant to respondents from the 5 national federations from the Aspire in the World Fellows pool were consistent with what observed in the FIFA sample regarding years of experience in professional football and employment at their organisations.
Data ecosystem
Among the FIFA sample, respondents indicated a median number of 3 (IQR, 2 to 6) match analysts and 1 (IQR, 1 to 2) sports scientist as staff members in the organisation. Conversely, statisticians and data engineers were low in number as staff members within this pool of organisations (). Perspectives on their respective organisations analytical capabilities were heterogenous, with approximately a third of respondents perceiving it as poor (~24%), acceptable (~31%), or good (~28%). Approximately half of respondents (~52%) from this pool agreed with the statement the ‘data management has limited standardization, with data analysts from different business units managing and elaborating data independently to a limited number of data sources’ regarding the data governance framework in place at their organisations. Approximately a third of respondents (~31%) agreed with the statement the ‘data management is standardized with well-defined procedures where different data sources are integrated within a shared platform and elaborated by dedicated IT architects and data analysts’, whereas only a minority of respondents (~17%) indicated ‘data management lacks standardization, with data analysts from different business units managing and elaborating data independently’.
Figure 1. Box-and-whisker plot summarising the number staff in different roles within national federations (a) and professional clubs (b).

The median number of match analysts and sports scientists as staff members at the professional clubs (n = 32) from the Aspire in the World Fellows sample were 5 (IQR, 3 to 7) and 3 (IQR, 1 to 5), respectively. Outcomes concerning the presence of statisticians and data engineers across organisations were heterogeneous, with figures representative of employment of this staff being generally low (). The majority of respondents perceived their respective organisations analytical capabilities as good (57%), and almost the majority of respondents (~53%) agreed with the statement the ‘data management is standardized with well-defined procedures where different data sources are integrated within a shared platform and elaborated by dedicated IT architects and data analysts’ with reference to their clubs’ data governance framework. Approximately half of respondents (~40%) agreed with the statement the ‘data management is standardized with well-defined procedures where different data sources are integrated within a shared platform and elaborated by dedicated IT architects and data analysts’, whereas only a minority of respondents (~7%) indicated ‘data management lacks standardization, with data analysts from different business units managing and elaborating data independently’. Responses relevant to the national federations from Aspire in the World Fellows (n = 5) were in line with insights from the FIFA sample, thereby revealing heterogeneity in analytical capabilities among these organisations and limited standardization in management of data sources.
Information system
In the FIFA sample (), only a third to approximately half of respondents indicated ‘the data analysis strategy is consistent across all business units’ (41%) and the ‘information needed to guide decision-making are readily available’ (48%). Approximately more than half of respondents agreed and strongly agreed with the statement ‘information generated from data guide decision-making’ in their technical (65%), recruiting (52%), performance (69%), and medical (62%) departments (). On the other hand, only between a third and approximately half of respondents agreed and strongly agreed with the statements that ‘information generated from data is communicated clearly’ to coaching staff (42%) and individual players (31%). PDF-reports (66%), insights from videos (66%), and presentations from data scientist/match analyst (59%) represented the most common approaches used to shared information amongst coaching staff, with individual players accessing information indirectly through discussions with the coaching staff (62%) or via custom-made applications (55%).
Figure 2. Percentage of responses relevant to the characteristics of the data analytics infrastructure and processes in place at national federations participating to the survey.

Approximately a third of respondents from the Aspire in the World Fellows sample () of professional clubs agreed and strongly agreed that ‘the data analysis strategy is consistent across all business units’ (36%), and the majority indicated ‘the information needed to guide decision-making are readily available’ (58%). The majority and approximately half of respondents agreed and strongly agreed with the statements that ‘information generated from data is communicated clearly via business intelligence solutions’ to coaching staff (~58%) and individual players (48%). Most of respondents from this pool agreed and strongly agreed with the statement ‘information generated from data guide decision-making’ in their technical (~61%), recruiting (~77%), performance (~94%), and medical (~77%) departments (). PDF-reports (70%), presentations from data scientist/match analyst (57%), and dashboards (50%) represented the most common approaches used to shared information amongst coaching staff, with individual players accessing information mainly indirectly through discussions with the coaching staff (67%).
From positional data processing to coaching insights
Information gathered from the FIFA sample respondents was summarized and illustrated in . Perspectives on the clarity and consistency of football metrics from international sports data analytics were heterogenous and inconsistent (), with approximately a third of respondents indicating strong disagreement and disagreement (~27%) versus agreement (30%). On the other hand, there was general agreement amongst respondents concerning the usefulness of general metrics derived from match-analysis data-production () rating these as very useful and extremely useful (55% to 87%). Main data sources used in performance analysis environments were tracking data (76%), video footage (72%), and event data (62%), with detailed data collection conducted for the analysis of own matches (62%), opponents analysis (62%), and trend analysis of major competitions (55%). Respondents generally feel confident and very confident about combining different data sources to draw combined insights (~69%), with ‘event-specific phases’ and ‘customised phases of play at the analyst discretion’ deemed the methods of choice for aiding the interpretation and contextualisation of performance data (~60%).
Discussion
Sports analytics is a relatively new field that is now integral for athlete development and management purposes. The increasing use of data to inform decision-making across various business areas to gain competitive advantage on both sport and financial levels required advancing the integration of data analytics solutions within modern football organisations over the last decade. The increased use of data reflects, in practical terms, some advances in applied sports sciences service provision that also resulted in broadening the number of backroom staff and sources of information available in the pursuit of a more structured approach to player management. Our investigation provided a contemporary overview from professional football clubs and national federations worldwide. Notwithstanding the general appreciation of data analytics as an element pivotal to leveraging raw data for actionable coaching insights, the heterogeneity of our findings highlighted the inconsistency of current data analytics architectures generally due resourcing limitations across professional football organisations.
In the search for competitive advantage, an important aspect for modern football organisations is to conduct formal benchmark appraisals given the current state-of-the-art in the industry (Alamar Citation2013; Robertson Citation2020). In this context, the definition of structured data ecosystems is the cornerstone for a pragmatic organisation and processing of sports data (Alamar Citation2013). Investments in human resources for analytics, therefore, constitute an important indicator regarding how important sports analytics are for the organisation by embedding staff dedicated to data governance and maintenance processes into the organisational framework (Alamar Citation2013). Insights on data ecosystem definition and governance from the Sports Analytics Use Survey suggested more than a third of respondents indicated not having a dedicated data engineer, whereas the majority reported the presence of one or two statistician/analyst on the sports side of the organisation (Alamar Citation2013). The findings from our study are consistent with previous reports regarding the under-representation of data engineers and statisticians across the football clubs and federations we examined in our investigation (). Specifically, our study revealed staff involved in other areas of service provision (e.g., sports scientist; S&C coach) outnumber staff dedicated to data ecosystem development and applied statistical analysis ().
In the realm of applied sports sciences, research previously emphasised the importance of integrating staff with expertise in applied biostatistics to support, for example, the control and surveillance of sports-injury data (Casals and Finch Citation2017). Our findings on the employment of support staff with expertise in football performance analysis and sports sciences are in line with recent insights (Gregson et al. Citation2022). Conversely, the inconsistencies in the number of staff dedicated to data organisation and elaboration from our study () highlighted potential limitations in the elaboration of raw data. This finding appears consistent with and reflected by the heterogeneity of responses on perceptions regarding the extent of analytical capabilities and data governance framework, particularly in the case of national federations. The fact that data management processes reached more advanced stages within football clubs than national federations seems a plausible and an interesting finding from our study. A logical explanation of this finding could be related to the nature of service provision demands among professional clubs operating on a day-to-day basis as opposed to national federations over a calendar year (Buchheit and Dupont Citation2018). Likewise, the nature of each organisation’s business model as well as own financial resources represent additional elements determining the scale and composition of data analytics staff employment (Gregson et al. Citation2022). From a general standpoint, the differences between professional football clubs and national federations in data analytics solutions are inherently contextual. Data analytics may generate a competitive advantage for professional clubs in obtaining, for example, optimal estimates on player transfer markets and appropriate salaries. These elements are, nevertheless, not of real practical relevance to the context of a national federation setting, hence why federations may invest less into a data infrastructure than clubs. The fact that national federations do not have consistent access to individual players could also be considered a contextual barrier limiting any potential advancement in data management innovation processes. Collectively, the heterogeneity in the development of data infrastructures revealed inconsistencies in data management processes that have the potential to constrain knowledge translations from data.
Investments in data-management technology provide foundations to build a data analytics system (Alamar Citation2013; Robertson Citation2020). Importantly, the set-up of an organised data infrastructure is central also to streamlining the communication of information and dissemination of knowledge from one area of the organisation to another (Alamar Citation2013). Information systems, or the standardised interfaces integrating sets of metrics relevant to the decision-making process, bridge the gap between data storage to organisational actions both at the club- and player-level (Alamar Citation2013; Delen and Demirkan Citation2013). Results from our study highlighted a general understanding and appreciation among respondents regarding the importance of developing information systems to guide decisions across national federations and professional clubs irrespective of the business unit (). Nevertheless, the communication of data insights is established on tools inconsistent with recent technological advances and innovations in the sports industry (Ratten Citation2016; Ward et al. Citation2019; Robertson Citation2020). Specifically, PDF-reports (70%) and presentations from support staff (
60%) represented the main tools used to communicate information and were common to both samples in our investigation. This line of evidence substantiated further recent insights suggesting issues of communication among different members of a sporting organisation is partly due to limitations in the development of decision support services integrating elements of data analytics (Gregson et al. Citation2022). Consistent with recent findings (Gregson et al. Citation2022), the limited number of support staff with expertise in data management and insights elaboration also highlighted the need to devote attention to potential issues of illiteracy across organisations with the use of general and more advanced data governance and analytics methods. Such aspects relevant to organisational expertise and any potential human resources limitations can hinder innovation management capabilities (Ratten Citation2016) and preclude maximising any return on data investments (Alamar Citation2013; Robertson Citation2020). The limitations on information system development that emerged from our study, however, were coherent with the general underemployment of sports data-dedicated staff () that is pivotal to the design and realisation of data infrastructures and elaboration processes (Alamar Citation2013; Ward et al. Citation2019; Robertson Citation2020; Windt et al. Citation2021). In practical terms, our findings suggested that information systems at national federations and professional clubs lack a comprehensive blueprint that can serve the needs of the decision-makers within and between organisational units.
The contemporary advances in technology and computer sciences also provided opportunities to enhance the analysis of positional data relevant to sports performance analysis (Jayal et al. Citation2018) and the study of tactical performance (Goes et al. Citation2021). Sports data providers in football have introduced a number of different metrics to support performance analysis (e.g., StatsBomb Citation2024; StatsPerform Citation2024), with their development increasing exponentially and in parallel with progresses in tracking solutions (Ellens et al. Citation2022) in recent years. For example, among these, expected goals (xG) refers to the probability of a shot resulting in a goal (Anzer and Bauer Citation2021). The proliferation of new performance metrics and analytical approaches (StatsBomb Citation2024; StatsPerform Citation2024) can be attributable to general attempts to address the diverse nuances of questions and potential problems in modern high-performance sport (Jayal et al. Citation2018; Robertson Citation2020; Windt et al. Citation2021). This phenomenon, nonetheless, contributed to posing additional challenges for performance analysts, mandating flexibility to improve, diversify, and adapt the operational skillset (Windt et al. Citation2021). Despite this and the consistency of perspectives on the usefulness of specific performance metrics for the post-match analysis, only a third of survey respondents perceived football metrics from international sports data analytics providers as sufficiently clear (). These findings can be explained from distinct perspectives. First, the heterogeneity and diversity of data sources, metrics definitions and calculations hinder their generalisation and utilisation for applied and research purposes. Second, and accordingly, our results reflected issues of literacy with data analytics methods and education among more technical and support staff within the modern sports organisation also common to other sports and industries (Ward et al. Citation2019; Robertson Citation2020; Windt et al. Citation2021). Taken together, insights from our study highlighted long-standing problems associated with the absence of scientific consensus on taxonomies for reference performance metrics (Mackenzie and Cushion Citation2013; Carling et al. Citation2014). Conceptual clarity on operational taxonomies across different commercial data providers and problems of data literacy both deserve attention and further investigation in the football industry and future research in sports analytics.
O’Donoghue (Citation2007) emphasised that end-users must possess an understanding of the metrics that sports performance analysts consider benchmarks for insights dissemination in their analysis process. Limited subject matter knowledge can pose unnecessary barriers hindering the flow of any structured decision-making process (Windt et al. Citation2021). Our investigation highlighted further the general lack of clarity regarding operational taxonomies of match performance-related events that remained unaddressed in performance analysis research (Mackenzie and Cushion Citation2013). Likewise, the fact the majority of respondents deemed ‘customised phases of play at the analyst discretion’ as a method of choice for aiding the interpretation and contextualisation of performance data is another interesting and important finding of our study. These insights, together with perspectives on the usefulness of outcome metrics from the match data production, may serve as a blueprint to inform future research efforts that aim to enhance and standardise performance analysis for service provision purposes. Accordingly, the failure to define a standardised set of performance-related taxonomies and performance analysis processes render data insights prone to subjective re-elaborations (Mackenzie and Cushion Citation2013). As a counterexample among other recent illustrations (Bauer et al. Citation2023), the development of the FIFA Football Language provided a conceptual framework serving as an educational tool established on a unified language (FIFA Citation2022). The FIFA Football Language encompasses different yet defined metrics with the aim to support the alignment of performance datasets for better understanding and breaking down of match-play (FIFA Citation2022). Pursuing a balance between a data-informed approach and technical interpretation by bringing together experienced data-literate professionals and football practitioners, the FIFA Football Language is a potential blueprint that can address, to some extent, current gaps in performance analysis research and warrants further investigation from technical, tactical, and physical perspectives. With the real-world example of the FIFA Football Language and previous considerations in mind (Carling et al. Citation2014), our findings emphasised further previous concerns (O’Donoghue Citation2007; Mackenzie and Cushion Citation2013) and the need to finalise scientific consensus on a framework of established taxonomies relevant to the different stakeholders involved in the data provision-to-utilisation spectrum.
Limitations
Although we provided a contemporary overview of the current state of data ecosystems and information systems in modern football organisations worldwide, our study is not without limitations. First, the heterogeneity of educational and professional backgrounds among our survey respondents, consisting mainly of technical and fitness coaches working in male football, represents an element of our study deserving consideration. Nonetheless, any degree of diversification in the respondents’ background did not preclude us from gathering the information meaningful to an objective description of infrastructures, processes, and insights generation, given data and analytics integration currently in place at modern football organisations. On the other hand, despite surveying delegates from top-tier professional clubs and national federations, the general heterogeneity of the members’ associations might have contributed to exaggerating aspects that pose limitations to data analytics service provision, which can be logically and, perhaps, directly attributed to resourcing limitations across these organisations.
Second, although we informed our study design and methods following seminal work in this area (Alamar Citation2013), the lack of clarity on the terminology used to identify different professional roles involved in analysing and elaborating different sources of football-related data constituted a meaningful challenge for our survey development. In general, researchers and practitioners in sports medicine and exercise sciences tend to interchangeably adopt the terms data scientist, statistician, and data analyst. Windt et al. (Citation2021) denoted the data scientist as a professional figure with responsibilities in providing decision-support systems encapsulating the integration of data management and analysis skillsets applied to developing and maintaining analytical solutions athlete monitoring, scouting/recruitment, match performance and epidemiological analysis purposes. Casals and Finch (Citation2017) defined a sports data analyst as a specialist blending expertise in statistics, sports sciences, and computer sciences. Conversely, the role of the sports biostatistician integrates knowledge of statistics, epidemiology, public health, and sports science (Casals and Finch Citation2017). With this diversity of nomenclatures in mind, in our survey, we used the term statistician as the expert at the ultimate end of the data provision-to-utilization continuum accountable for translating data into information (Alamar Citation2013).
Third, and importantly, the absence of information for our survey content validity represents another limitation that should be acknowledged. Likewise, the strategies we adopted to maximise survey items interpretation and understanding as intended require attention. Specifically, the general survey design involving mainly closed items and the fact we shared a copy of the survey in advance to conference delegates ultimately aimed to maximise response rates (Boynton Citation2004). In addition to this, the verbatim adaptation approach used to conduct survey translation in other languages might have necessitated considerations of other relevant approaches to ensure consistency with the original instrument despite its fundamental simplicity. The fact we also distinguished the pool of survey respondents, with the FIFA sample only addressing questions of survey section iv) given the larger backgrounds in football coaching and performance analysis, ought to be considered as another potential limitation despite aiming to explore the current state-of-the-industry based on organisations taking part to a particular event than others following similar examples from previous studies addressing different research questions (McCall et al. Citation2022). We also highlight that caution is necessary to generalise our findings which are unlikely to apply to other football contexts with fewer financial resources or limited infrastructures since our study described the current state-of-the-industry regarding data analytics service provision based on the perspectives of different stakeholders working within men’s top-tier football clubs and national federations.
Conclusion
Sports science service provision processes evolved in professional football organisations over the last decade. This evolution resulted in a concomitant increase in the number of information sources requiring the development of data analytics infrastructures devoted to data-to-insights translations to support decision-making of backroom staff on player management strategies. The present survey outcomes also indicated football organisations made improvements in making information readily available and relatively clearly communicated to coaches. Nevertheless, our findings also suggested that, despite the general appreciation of data analytics as an element pivotal to supporting player and team management decisions, current data analytics ecosystems are inconsistent in addressing the contemporary demands of support staff at modern football organisations. The limited number of support staff with expertise in data governance and applied biostatistics as well as the lack of an established framework with clear operational taxonomies for reference performance metrics pose constraints to insights generation from raw data. At present, these elements represent challenges for being at the cutting edge of sports analytics service provision and require attention in future lines of inquiry to leverage capabilities of service-oriented data analytics in top-tier football. Our insights are, therefore, anticipated to inform organisational decisions for improving data analytics service provision in football contexts and future research in performance analysis.
Author contributions
Lorenzo Lolli: Conceptualization, Methodology, Software, Investigation, Formal analysis, Writing – original draft, Writing – review & editing, Project administration. Pascal Bauer: Methodology, Investigation, Writing – original draft, Writing – review & editing. Callum Irving: Methodology, Investigation, Writing – original draft, Writing – review & editing. Daniele Bonanno: Methodology, Investigation, Writing – original draft, Writing – review & editing. Oliver Höner: Methodology, Investigation, Writing – original draft, Writing – review & editing. Warren Gregson: Conceptualization, Methodology, Investigation, Writing – original draft, Writing – review & editing. Valter Di Salvo: Conceptualization, Methodology, Writing – original draft, Writing – review & editing.
Ethics approval statement and informed consent
This study was approved by the Aspire Zone Foundation Institutional Review Board, Doha, State of Qatar (protocol number: E202209040).
Supplemental Material
Download Zip (121 KB)Acknowledgements
The authors wish to thank the Aspire in the World Fellows and staff from national federations qualified for the FIFA World Cup Qatar 2022TM without whom this study would not have been possible. The authors would like to express their gratitude to Mr. Nidhal Zarrouk for the translation of original survey draft in the Arabic language and to colleagues at FIFA for addressing translations in the other languages. The authors are grateful to the 2 anonymous reviewers whose suggestions were invaluable to improving the manuscript. Open Access funding provided by the Qatar National Library.
Disclosure statement
No potential conflict of interest was reported by the author(s).
Data availability statement
The survey copies are available as supplementary material.
Supplementary material
Supplemental data for this article can be accessed online at https://doi.org/10.1080/24733938.2024.2341837
Additional information
Funding
References
- Alamar BC. 2013. Sports analytics: a guide for coaches, managers, and other decision makers. New York Chichester, West Sussex: Columbia University Press.
- Amrhein V, Trafimow D, Greenland S. 2019. Inferential statistics as descriptive statistics: there is no replication crisis if we don’t expect replication. Am Stat. 73(sup1):262–270. doi: 10.1080/00031305.2018.1543137.
- Anzer G, Bauer P. 2021. A goal scoring probability model for shots based on synchronized positional and event data in football (soccer). Front Sports Act Living. 3:624475. doi: 10.3389/fspor.2021.624475.
- Bartlett JD, Drust B. 2021. A framework for effective knowledge translation and performance delivery of sport scientists in professional sport. European Journal of Sport Sciences. 21(11):1579–1587. doi: 10.1080/17461391.2020.1842511.
- Bauer P, Anzer G, Shaw L. 2023. Putting team formations in association football into context. J Sports Anal Preprint. 9(1):1–21. doi: 10.3233/JSA-220620.
- Bornn L, Cervone D, Fernandez J. 2018. Soccer analytics: unravelling the complexity of “the beautiful game”. Significance. 15(3):26–29. doi: 10.1111/j.1740-9713.2018.01146.x.
- Boynton PM. 2004. Administering, analysing, and reporting your questionnaire. BMJ. 328(7452):1372–1375. doi: 10.1136/bmj.328.7452.1372.
- Buchheit M, Dupont G. 2018. Elite clubs and national teams: sharing the same party? Sci Med Footb. 2(2):83–85. doi: 10.1080/24733938.2018.1470156.
- Carling C, Wright C, Nelson LJ, Bradley PS. 2014. Comment on ‘Performance analysis in football: a critical review and implications for future research’. J Sports Sci. 32(1):2–7. doi: 10.1080/02640414.2013.807352.
- Casals M, Finch CF. 2017. Sports Biostatistician: a critical member of all sports science and medicine teams for injury prevention. Inj Prev. 23(6):423–427. doi: 10.1136/injuryprev-2016-042211.
- Cook JA, Julious SA, Sones W, Hampson LV, Hewitt C, Berlin JA, Vale LD, Emsley R, Fergusson DA, Walters SJ. 2019. Practical help for specifying the target difference in sample size calculations for RCTs: the DELTA(2) five-stage study, including a workshop. Health Technol Assess. 23(60):1–88. doi: 10.3310/hta23600.
- Delen D, Demirkan H. 2013. Data, information and analytics as services. Decis Support Syst. 55(1):359–363. doi: 10.1016/j.dss.2012.05.044.
- Ellens S, Hodges D, McCullagh S, Malone JJ, Varley MC. 2022. Interchangeability of player movement variables from different athlete tracking systems in professional soccer. Sci Med Footb. 6(1):1–6. doi: 10.1080/24733938.2021.1879393.
- FIFA. 2022. The FIFA football language. https://www.fifatrainingcentre.com/en/game/performance-analysis/football-language-analysis/the-fifa-football-language.php.
- Ford PR, Bordonau JLD, Bonanno D, Tavares J, Groenendijk C, Fink C, Di Salvo V, Gregson W, Varley MC, Weston M. 2020. A survey of talent identification and development processes in the youth academies of professional soccer clubs from around the world. J Sports Sci. 38(11–12):1269–1278. doi: 10.1080/02640414.2020.1752440.
- Gamble P, Chia L, Allen SF. 2020. The illogic of being data-driven: reasserting control and restoring balance in our relationship with data and technology in football. Sci Med Footb. 4(4):338–341. doi: 10.1080/24733938.2020.1854842.
- Gerrard B, Alamar BC. 2014. Sports analytics: A guide for coaches, managers and other decision makers. Sport Manage Rev. 17(2):240–241. doi: 10.1016/j.smr.2013.06.005.
- Goes FR, Meerhoff LA, Bueno MJO, Rodrigues DM, Moura FA, Brink MS, Elferink‐Gemser MT, Knobbe AJ, Cunha SA, Torres RS. 2021. Unlocking the potential of big data to support tactical performance analysis in professional soccer: a systematic review. European Journal of Sport Sciences. 21(4):481–496. doi: 10.1080/17461391.2020.1747552.
- Gregson W, Carling C, Gualtieri A, O’Brien J, Reilly P, Tavares F, Bonanno D, Lopez E, Marques J, Lolli L. 2022. A survey of organizational structure and operational practices of elite youth football academies and national federations from around the world: A performance and medical perspective. Front Sports Act Living. 4:1031721. doi: 10.3389/fspor.2022.1031721.
- Jayal A, McRobert A, Oatley G, O’Donoghue P. 2018. Sports analytics applications in soccer. London: Routledge.
- Lundqvist C, Gregson W, Bonanno D, Lolli L, Di Salvo V. 2022. A worldwide survey of perspectives on demands, resources, and barriers influencing the youth-to-senior transition in academy football players. Int J Sports Sci Coa 19(1):17479541221135626. doi: 10.1177/17479541221135626.
- Mackenzie R, Cushion C. 2013. Performance analysis in football: a critical review and implications for future research. J Sports Sci. 31(6):639–676. doi: 10.1080/02640414.2012.746720.
- McCall A, Davison M, Massey A, Oester C, Weber A, Buckthorpe M, Duffield R. 2022. The exchange of health and performance information when transitioning from club to National football teams: A Delphi survey of National team practitioners. J Sci Med Sport. 25(6):486–491. doi: 10.1016/j.jsams.2022.03.011.
- O’Donoghue P. 2007. Reliability issues in performance analysis. Int J Perform Anal Sport. 7(1):35–48. doi: 10.1080/24748668.2007.11868386.
- Ratten V. 2016. Sport innovation management: towards a research agenda. Innov. 18(3):238–250. doi: 10.1080/14479338.2016.1244471.
- Robertson S. 2020. Man & machine: adaptive tools for the contemporary performance analyst. J Sports Sci. 38(18):2118–2126. doi: 10.1080/02640414.2020.1774143.
- Starling LT, Lambert MI. 2018. Monitoring rugby players for fitness and fatigue: what do coaches want? Int J Sport Physiol. 13(6):777–782. doi: 10.1123/ijspp.2017-0416.
- StatsBomb. 2024. Metrics & explainers. https://statsbomb.com/articles/soccer/metrics-and-explainers/.
- StatsPerform. 2024. Advanced metrics. https://www.statsperform.com/opta-analytics/.
- Ward P, Windt J, Kempton T. 2019. Business intelligence: how sport scientists can support organization decision making in professional sport. Int J Sport Physiol. 14(4):544–546. doi: 10.1123/ijspp.2018-0903.
- Windt J, Taylor D, Little D, Sporer BC. 2021. Making everyone’s job easier. How do data scientists fit as a critical member of integrated support teams? Br J Sports Med. 55(2):73–75. doi: 10.1136/bjsports-2020-102938.