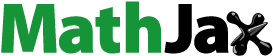
Abstract
This study aims at modelling long-term weather parameters (1955 to 2020), recorded in Bahrain, and at investigating which parameters have strong correlation coefficients with such data to account for mitigation and achieve sustainability. Eight forms of fitting methods were utilized to calculate the correlation coefficient between each parameter versus each given year. These parameters (Average and Anomalies) are the average temperature, maximum temperature, minimum temperature, humidity, wind speed, dust and precipitation. The forms of correlations used are as follows: Linear regression, Quadratic regression, Cubic regression, Power regression, ab-Exponential regression, Logarithmic regression, Hyperbolic regression and Exponential regression. Among all forms of regressions, the Exponential (cubic) regression is found to have the highest correlation coefficient for such data, both Average and Anomalies data; the highest is for humidity versus year (r = 0.900, strong relation), and the least is for precipitation (r = 0.1647, poor relation) for Average data. As for the Anomalies data, the highest is for humidity versus year (r = 0.9019) and the least is for precipitation versus (r = 0.1647, no relation). The novelty of this paper lies in concluding that the exponential (cubic) regression is the most accurate correlation (among 8 correlation coefficients) to predict all the recorded long-term (65 years) weather parameters in Bahrain eight correlation. This regression fit is the most useful one in projecting the weather trend by 2050 in Bahrain to account for the future built environment to become more resilient, sustainable and tolerant with the environment due to the anticipated damage resulting from climate change. More investigation is to be made for other data set in the Gulf Cooperation Countries, and worldwide, to explore whether this Exponential (cubic) regression will still have the highest correlation coefficient among the others.
1. Introduction
Long-term weather parameters (climate change) and the Built Environment nexus were classified into categories. These categories include building structure, which is affected by several factors (floods, landslides, storms and snow loads), building constructions (influenced by fastening systems and water supply), building materials (impacted by frost resistance, ultraviolet resistance and insulation properties) and the indoor climate (affected by humidity and temperature) (Andrić, Koc, & Al-Ghamdi, Citation2019).
Currently, with devastating weather disasters witnessed worldwide; in Asia (flooding of Pakistan and China), Europe (drought of rivers, wildfires, flooding, massive ice stones), North America and South America (drought of rivers, wildfires, flooding), scientists are obliged to conduct more advanced research in climatology, modelling, forecasting the future using Big Data analytics (Sebestyén, Czvetkó, & Abonyi, Citation2021). Now, there is a strong tie and Nexus between Weather (Climate), Prediction & Projection and Built Environment. The latter is defined as human made environment that provides proper setting of houses, buildings, zoning, streets, open spaces transportation options and more. According to the Environmental Protection Agency (EPA) (Citation2022) the built environment touches all aspects of our lives, including the buildings and city habitats, the distribution systems that provide us with water and electricity, roads, bridges, and transportation systems used. It is the man-made or modified structures that facilitate the living, working, and recreational spaces. There is a strong relation between Built Environment and Health. The built environment influences our level of physical activity; for instance, the inaccessibility or nonexistence of sidewalks and bicycle or walking paths contributes to sedentary habits, leading to poor health outcomes such as obesity, cardiovascular disease, diabetes, and some types of cancer (Centre for Disease Control and Prevention (CDC), Citation2022). Additionally, there is a strong relationship between the built environment and weather parameters, and hence climate change has been characterized in order to propose systemic changes to improve the adaptation of cities to climate change (Zięba et al., Citation2020).
Among the useful research in using long-term meteorological data to create awareness and contribute to a growing body of climate change that is necessary for the development of effective mitigation and adaptation strategies is the recent published work of Kazemzadeh, Noori, Jamali, & Abdi (Citation2022). Forty years of air temperature changeover was utilized and the polynomial fitting scheme was used—to both monthly and annual air temperature data—to detect trends and classify them into linear and nonlinear (quadratic and cubic) categories. Applying similar approach of utilizing long term data is the study on a new globally reconstructed sea surface temperature analysis dataset since 1900 developed by the China Meteorological Administration (Chen, Cao, Zhou, Zhang, & Liao, Citation2021).
Furthermore, building environment management systems should allow for minimizing the possible future negative impact of natural hazards caused by the behavior of long-term weather parameters. This can be achieved by looking into the correlation coefficients between these parameters with the passing and forth coming years (Świąder et al., Citation2018). Proper planning and configuring built environment disaster, economic, social, energy, water and comforting risk from monitoring and studying correlation between various weather parameters throughout the years, i.e. modelling, will lead to great reduction and comfort urban life (Mabon, Kondo, Kanekiyo, Hayabuchi, & Yamaguchi, Citation2019; Otto-Zimmermann, Citation2010; Sharifi & Yamagata, Citation2018).
Indeed, to have a sustainable building, one needs reliable information on the long-term performance and, more specifically, the expected service-life of building materials, components, assemblies and most importantly anticipated effects of climate change on the built environment (Lacasse, Citation2019).
Al-Humaiqani and Al-Ghamdi (Citation2022) listed important indicators that play a crucial role in marking a resilient quality to build environment planning, analysis, and design where among them is weather modelling.
The variation of long-term meteorological parameters, past and future projections, is anticipated to have a significant impact on the built environment, especially, whereby the world faces climate change as one of the biggest environmental challenges ever (Dervis, Citation2007; European Environment Agency (EEA), Citation2021; Carpenter, Walker, Anderies, & Abel, Citation2001; Chen, Moufouma-Okia, Masson-Delmotte, Zhai, & Pirani, Citation2018; Solomon, Plattner, Knutti, & Friedlingstein, Citation2009). In 2015, the estimated climate change cost to private businesses was about USD 43 trillion during the 21st century (UNFCCC, Citation2017). This explains why researchers do modelling and correlations to their weather data (Nabipour et al., Citation2020; Sharafati & Pezeshki, Citation2020; Zhao et al., Citation2021).
The officially accurate recorded data in Bahrain from 1955 to 2020 were used as an example to calculate the various correlation coefficients resulted from each form of statistical fitting between each meteorological parameter and the year. These weather parameters (Average and Anomalies) are the average temperature, maximum temperature, minimum temperature, humidity, wind speed, dust and precipitation. The forms of correlations used are eight: Linear regression, Quadratic regression, Cubic regression, Power regression, ab-Exponential regression, Logarithmic regression, Hyperbolic regression and Exponential regression.
The purpose of the paper is to explore whether there is a specific regression coefficient, among the known eight regression coefficients, which is more accurate than the others to represent all the long-term (65 years) recorded weather data parameters. It also aims to alert policy makers, architects, engineers, and environmentalists to project the temperature, humidity, precipitation, etc., in 2050 to allow for actions, mitigations and adaptation to climate change and be resilient towards it.
This study is one of the measures that policy makers and developers should conduct in order to forecast the required built environment to be of resilient quality. For this reason, we tested eight forms of fitting methods to calculate the long-term meteorological parameters’ correlation coefficient for future built environments. The outcome of this study can be tested when doing similar investigation on other weather data set in the Gulf Cooperation Council Countries (GCCC) and worldwide to explore which form of the aforementioned eight-correlation regression will have the highest correlation coefficient and be used for forecasting.
2. Methodology
Recorded data from 1955 to 2020 were made by Bahrain Meteorological Directorate, Ministry of Transport and Communication, Kingdom of Bahrain. The station is well calibrated and regularly updated. It has data since 1905 but the most reliable data are those from 1955. The station is located at Bahrain International Airport, Muharraq town, which was the first Capital of Bahrain.
The data is measured continuously and distributed at 10 min intervals, then at hour interval and per day to per month to per year. The station records various meteorological parameters; however, for this research we have selected the most important parameters for built environment. Although solar radiation data is important, it was only recorded accurately and systemically in Bahrain since 2016. However, the recorded temperature (minimum, average and maximum) can be considered to represent the solar radiation; it’s a result of absorbing and emitting solar energy. This is a valid point since we are not studying the reason for changing the recorded values of meteorological parameters, but rather we are exploring which correlation coefficient—among eight—is more accurate to represent the long-term meteorological data in Bahrain. Besides, Bahrain has solar radiation data for the past 7 years only while the other data were since 1955. These weather parameters (Average and Anomalies) are average temperature, maximum temperature, minimum temperature, humidity, wind speed, dust and precipitation. These data may represent the entire island because the area of the main island is about 604 km2 (Length about 51 km and width 18 km) with latitude 26.03°N and longitude 50.55°E.
The Kingdom of Bahrain consists of 30 small islands, five of which are relatively large islands. Muharraq island is considered to be one of those large islands. The length of the coastline is 130 km and the highest elevation is in south middle of Bahrain—Aldokhan Mountain (134 m)—. This means there is no much variation in the weather data; it does not exceed 5%.
Figure 1. Bahrain Map showing the location of the official weather station in Muharraq City, where Bahrain International Airport is located.

In order to produce regression equations for each meteorological parameter (y) versus year (x), we used several regression fits. These are available online, and they provide target function table data in the form of points [x, f(x)] to build several regression models listed below (PLANETCALC Online, Citation2022):
Linear regression: y = ax + b
Quadratic regression: y = ax2+bx + c
Cubic regression: y = ax3+bx2+cx + d
Power regression: y = a xb
ab-Exponential regression: y = a⋅bx
Logarithmic regression: y = a+b ln(x)
Hyperbolic regression: y = a−b/x
Exponential regression: y = e (a + bx)
The correlation between each meteorological parameter (y) and year (x) for each type of regression fit can be compared using the following:
Correlation coefficient (r): It is called also Pearson’s correlation. It is used to measure how strong a relationship is between two variables and is commonly used in linear regression to find how strong a relationship is between data. The formulas return a value between −1 and 1, where 1 indicates a strong positive relationship, −1 indicates a strong negative relationship and zero indicates no relationship at all.
Coefficient of determination (R2): It is a number between 0 and 1 that measures how well a statistical model predicts an outcome. R2 can be interrupted as the proportion of variation in the dependent variable that is predicted by the statistical model.
Average relative error (ARE): It is used as a measure of precision; it is the ratio of the absolute error of a measurement to the measurement being taken. This type of error is relative to the average size of the item being measured. RE is expressed as a percentage and has no units.
Such statistical data allow decision makers to prioritize a particular meteorological parameter that most likely will affect the future built environment.
lists all regression fits for the average long-term weather data in Bahrain from 1955 to 2022 while lists all regression fits for the anomalies average long-term weather data from 1955 to 2022. The Regression fit that has the highest correlation coefficient for each weather set parameter (in Bold blue color) was marked in bold black color.
Table 1. Regression fits for the average long-term weather data in the Kingdom of Bahrain from 1955 to 2020.
Table 2. Regression fits for the anomalies average long-term weather data in the Kingdom Bahrain from 1955 to 2020.
3. Results
summarizes the correlation coefficients (r) for the Average Data of the long-term Meteorological parameters; average temperature T, maximum temperature Tmax, minimum temperature Tmin, average humidity H, average wind speed V, dust D and precipitation Pr . It clearly shows that the highest correlation coefficient (R) is when we use the cubic regression type equation. These results are derived from the following regression fits for the Average data (, sections 1.1 to 7.8).
Table 3. Summary of the correlation coefficients (r) for the different quantities fitted to the different forms for Average Data.
summarizes the correlation coefficients (r) for the Anomalies Data of the long-term meteoritical parameters; average temperature T, maximum temperature Tmax, minimum temperature Tmin, average humidity H, average wind speed V, dust D and precipitation Pr. Herein, Anomalies are the deviation of a measurable unit (e.g. temperature or precipitation) over a period in a given location from the long-term average, often the 65-year mean, for that location. Similarly, it clearly shows that the highest correlation coefficient (R) is evident when the cubic regression type equation is used. These results are derived from the following regressions fit for the Anomalies data (, sections 8.1 to 14.8).
Table 4. Summary of the correlation coefficients (r) for the different quantities fitted to the different forms for Anomalies Data.
The data reveal that the Exponential (cubic) regression has the highest correlation coefficient for both the Average and Anomalies data (fitting) for temperature, maximum temperature, minimum temperature, humidity, wind speed, dust and precipitation against the year, using eight forms of correlations. The highest correlation is for humidity versus year (r = 0.900, strong relation) and the least is for precipitation (r = 0.1647, no relation) for Average data fitting. These are presented in sections , sections 1.3, 2.3, 3.3, 4.3, 5.3, 6.3, 7.3 and 8.3. This exponential (cube) regression fit has also the highest Coefficient of determination (R2)—compared to others for the Average data, with highest R2 of 0.8100 for humidity. Similar findings for Anomalies data fitting are reported (, sections 8.3, 9.3, 10.3, 11.3, 12.3, 13.3 and 14.3), where Exponential (cubic) regression has the highest correlation coefficient; the highest is for humidity versus year (r = 0.9019) and the least is for precipitation (r = 0.1647). This Exponential (cube) fit has the highest Coefficient of determination (R2)—compared to others, with the highest correlation for humidity (R2 = 0.8135) and least for precipitation (R2 = 0.0271).
Therefore, the best fitting regression equation to predict or foresee a particular meteorological parameter is the exponential cube regression fit, for both Average data and Anomalies data. These can be used to plan for future built environment. This is crucial due to concentration of greenhouse gases (GHG), led by carbon dioxide (CO2), in our atmosphere, along with all negative man-made destruction to the environment which has resulted in climate change and subsequently to the evolution or creation of extremities.
4. Discussion
shows the annual variation of the recorded average long-term temperature throughout the years from 1955 to 2022. The figure shows that the temperature is expected to increase exponentially in Bahrain. It might reach an average of 36.0 °C by 2050. Considering the temperature, the built environment should undergo substantial changes. It seems that, taking into account the house walls’ thickness and the use of shade forecasting on the houses, is not enough. It is notable, too, that some serious consideration should also be given to the exposure of the roofs or facades to sun light in order to harvest solar electricity to avoid CO2 emission. It is indeed a big challenge to architects, developers and environmentalists.
Figure 2. The annual variation of the recorded average long-term temperature throughout the years from 1955 up to 2022. The curve is represented by the Following equation: .

shows the annual variation of the recorded maximum long-term temperature throughout the years from 1955 to 2020. The figure shows that the temperature is expected to increase exponentially in Bahrain. It might reach an average of 33.7 °C by 2050. This seems to be an unusual result as it should be higher than the expected average temperature in 2050 (36.0 °C).
Figure 3. The annual variation of the recorded average long-term maximum temperature throughout the years from 1955 up to 2022. The curve is represented by the following equation: .

shows the annual variation of the recorded minimum long-term minimum temperature throughout the years from 1955 to 2022. The figure shows that the temperature is expected to increase exponentially in Bahrain. It might reach an average of 35.0 °C by 2050. This result is consistent with the expected average temperature in 2050 (36.0 °C), where both are subjected to nearly a “worrying” exponential increase. The result means that no significant winter is expected in the future. Accordingly, the built environment should be thought off very well.
Figure 4. The annual variation of the recorded average long-term minimum temperature throughout the years from 1955 up to 2022. The curve is represented by the following equation: .

shows the annual variation of the long-term average temperature anomalies of the years from 1955 up to 2022. The curve (exponential cube regression fit) shows that the temperature in 2050 will be larger by 9.5 °C than the long-term average temperature (which was normalized to 0 °C). This is a substantial increase for a span of 30 years.
Figure 5. The annual variation of the long-term average temperature anomalies of the years from 1955 up to 2022. The curve is represented by the following equation: .

The annual variation of the long-term maximum temperature anomalies of the years from 1955 up to 2022 () shows that by 2050, the rise of the maximum temperature will be 9.5 °C compared to long-term maximum average (65 years) which is alerting especially because the correlation coefficient r is relatively high (0.7879). However, the situation seems to be worse when looking at the annual variation of the long-term minimum temperature anomalies of the years from 1955 to 2022 (). It shows that in about 30 years the increase in the long-term average minimum temperature will be 12 °C, given the fact that the correlation coefficient r is relatively high (0.8424).
Figure 6. The annual variation of the long-term average maximum temperature anomalies of the years from 1955 up to 2022. The curve is represented by the following equation: .

Figure 7. The annual variation of the long-term average minimum temperature anomalies of the years from 1955 up to 2022. The curve is represented by the following equation: .

The most surprising result is the variation of annual mean relative humidity from 1955 to 2020, which shows that humidity is expected to decrease from 66% (the long-term average) to 46% by 2050 (). This is an advantage for people living on islands as the cooling system may perform better, particularly for air conditioners working in water due to the evaporation mechanism.
Figure 8. The annual variation of the long-term average relative humidity of the years from 1955 up to 2022. The curve is represented by the following equation: .

The highest correlation coefficient, among all the examined meteorological parameters, belongs to humidity (r = 0.9000). The same observation is applied to the annual variation of relative humidity anomalies (). The relative humidity will be less than the long-term average humidity by 22 unit percentage. This is the highest correlation coefficient calculated in this study (r = 0.9019).
Figure 9. The annual variation of the long-term average anomalies relative humidity of the years from 1955 up to 2022. The curve is represented by the following equation: .

illustrates the long-term average annual wind speed variation (from 1955 to 2022). The results indicate that the wind speed has a tendency to reduce annually reaching an average wind speed of 8.2 knots (4.1 m/s) by 2050. This means that the potential of using wind energy for city and homes electrification is less optimistic unless micro turbines are used where they have a low cut-in speed value (1 m/s). It is interesting to note that the correlation coefficient is not high (r = 0.6754). For the annual variation of the wind speed anomalies, the result shows that the wind speed drops by 1.4 knots (0.7 m/s) by 2050 compared to the long-term average ().
Figure 10. The annual variation of the long-term average wind of the years from 1955 up to 2022. The curve is represented by the following equation: .

Figure 11. The annual variation of the long-term average wind anomalies of the years from 1955 up to 2022. The curve is represented by the following equation: .

illustrates the long-term average annual variation from 1955 to 2022, taking into consideration the annual total number of days with dust, dust storms or sandstorms (visibility 1000 meters or less). It seems that there is a tendency that storm or sand storm events will increase to 15 by 2050 (). This means that the performance of PV panels and, to a larger degree, the solar concentrators parabolic (CSP) will be hugely affected as they are very sensitive to sand and dust. If this is the trend, then future renewable energy option for the built environment should exclude CSP, otherwise, the consumption of water will be high which will offset the advantage of solar benefit. For the annual variation of dust, dust storm or sandstorm anomalies, the result shows that 9 more dust storms or sandstorms will occur by 2050 compared to the long-term average (). Fortunately, the correlation coefficient between dust events (both average and anomalies) are low, i.e. r = 0.1733 and 0.1733, respectively.
Figure 12. The annual variation of the long-term average annual total number of days with dust, dust storm or sandstorm (visibility 1000 meters or less) from 1955 up to 2022. The curve is represented by the following equation: .

Figure 13. The annual variation of the long-term average annual total number of days with dust, dust storm or sandstorm anomalies (visibility 1000 meters or less) from 1955 up to 2022. The curve is represented by the following equation: .

shows the results for the long-term average annual precipitation variation from 1955 to 2022. The results indicate that there is a great tendency to reduce annually reaching deficit value (−270 mm) by 2050 (), while for the anomalies, the annual precipitation is less than the long-term average (71 mm) by 340 mm (huge deficit) by 2050 compared to the long-term average (). Due to the very low correlation coefficient between precipitation versus year—which is 0.1647 for both average and anomalies data, no consideration is given for this analysis.
Figure 14. The annual variation of the long-term average annual precipitation from 1955 up to 2022. The curve is represented by the following equation: .

Figure 15. The annual variation of the long-term average annual precipitation anomalies from 1955 up to 2022. The curve is represented by the following equation: .

In order to mitigate the impacts of random, short-term fluctuations on the weather over a specified time frame, the moving average was calculated and illustrated for the annual mean temperature average () and annual mean temperature anomalies average (). This moving average offer an analysis of average temperature data points by creating a series of averages of different subsets of the full data set, herein, every five years. We had selected the temperature (both average and anomalies) because of its significance in built environment and both have relatively high correlation coefficient (r = 0.73922495, R2 = 0.54645353 and r = 0.73922495, R2 = 0.54645353, respectively) as this weather parameter is significant in the building design, either to minimize heat input (in high solar radiation countries), or maximize heat input (in low radiation countries) (Build, Citation2021; El Bakkush, Bondinuba, Bondinuba, & Harris, Citation2015). and ascertained that temperature increase is expected to be substantial and a lot of mitigation should be made to offset such expected increase.
Figure 16. The annual variation of the recorded average long-term temperature, along with using moving average per 5 years subset, throughout the years from 1955 up to 2022.

Figure 17. The annual variation of the recorded mean long-term temperature anomalies, along with using moving average per 5 years subset, throughout the years from 1955 up to 2022.

It is worth mentioning that the occurrence of construction disasters in Poland, during a period of 12 years, were due to random extreme weather events (73%) such as strong wind or strong wind with heavy precipitation causing floods and landslides (Zięba et al., Citation2020). Therefore, studying correlation between time scale (years) and different weather parameters is significant and could save lives, economy, safety and security.
Finally, the outcome of this study can be tested by doing similar investigation on other weather data set in the Gulf Cooperation Countries (GCCC) and worldwide to explore whether still the Exponential (cubic) regression will have the highest correlation coefficient or other may other regression from the other seven regression coefficients tested in this study. This is important because most of these data set of weather parameter is used for estimating the potential of renewable energy (Alnaser, Citation1993; Alnaser, Awadalla, & Almoataz, Citation1992; Alnaser & Aldudiafa, Citation1990; Alnaser & Awadalla, Citation1990; Danny, Li, & Lam, Citation2012) as well as in built environment & sustainable cities (Ceccato, Ramirez, Manyangadze, Gwakisa, & Thomson, Citation2018; Hui & Tsang, Citation2005) and sustainability & Sustainable Development Goals (SDGs) (Griggs et al., Citation2021).
As mentioned earlier, the purpose of this paper is not to monitor the weather parameters and then make projection; if this were the case, then one can use artificial intelligence techniques (Duhoon & Bhardwaj, Citation2021; Hung, Babel, Weesakul, & Tripathi, Citation2009; Litta, Idicula, & Mohanty, Citation2013; Sfetsos & Coonick, Citation2000). However, the purpose of the paper is to explore whether there is a specific regression coefficient that is more accurate than the other eight regressions coefficient (the exponential cubic regression) to represent all the long-term recorded weather data parameters in Bahrain, or if there may be different correlation coefficients are suitable for each different long-term recorded weather data parameter. The novel finding, herein, is that the exponential cubic correlation is found to be more accurate to represent all the long-term meteorological recorded data (65 years) in Bahrain. In future research paper, we will attempt to use Artificial intelligent technique for projecting the weather parameters using Sequential Minimal Optimization (SMO), Radial Basis Function (RBF) and Multilayer perceptron (MLP).
5. Conclusion
The exponential (cubic) regression is the most accurate correlation (among 8 correlation coefficients) to predict all the recorded long-term (65 years) weather parameters in Bahrain; there is no multi correlations between different parameters.
Weather parameters can cause hazards for communities and may cause huge disturbances in achieving suitability. It is important to anticipate the variation of weather parameters (extremes) in order to achieve mitigation and establish resilience strategies and technologies to minimize such hazards, i.e. convert to sustainable cities and make buildings more resilient to changes in weather such as energy, water, food and transport. Therefore, this study focused on modelling the weather parameters and exploring which weather parameter has a strong correlation coefficient throughout the years.
The analysis in this study showed that the two weather parameters that have high correlation coefficient in modelling their annual changes (past and future) are temperature and humidity, while the other parameters give relatively lower value of correlation coefficient (r). The weather parameter that gives the lowest r is precipitation and dust storms or sand storms.
The investigation and modeling of weather parameters throughout the years in this study will help to reduce the risk and mitigate the effect of construction disasters if they occur.
This study is a milestone in modelling the variation of meteorological parameters to improve the built environment resilience qualities and probably help in setting mitigation and resilience strategies based on the projection of future weather parameters. However, further research to develop plans and policies for effective and timely responses to climate change effects is also required. The limitation of this study lies on using one country weather data set (Bahrain) and not testing for other GCC countries, including cities like Dharan City in KSA, Doha City, Qatar, Kuwait City, Kuwait or other worldwide countries. This limitation is mainly due to the inability to obtain officially recorded data. This limitation, however, will not demote the significance of the outcome and concept of this paper.
Acknowledgements
The authors thank Arabian Gulf University (AGU) and University of Bahrain (UoB) for their support to publish this paper. Thanks, are also due to Mr Nader Ahmed Abdulla, Chief of Climate & Seismology Meteorological Directorate, Ministry of Transport and Communication, Kingdom of Bahrain, for providing accurate long-term meteorological data. The authors are also grateful to Dr. Ghada Ahmed, Head of the Department of English Language and Literature at UoB, and Mrs. Luma Al Salah, Language Instructor at AGU for editing this paper.
Disclosure statement
No potential conflict of interest was reported by the authors.
References
- Al-Humaiqani, M. M., & Al-Ghamdi, S. G. (2022). The built environment resilience qualities to climate change impact: Concepts, frameworks, and directions for future research. Sustainable Cities and Society, 80, 103797. doi:10.1016/j.scs.2022.103797
- Alnaser, W. E. (1993). New model to estimate the solar global irradiation using astronomical and meteorological parameters. Renewable Energy. 3(2-3), 175–177. doi:10.1016/0960-1481(93)90016-A
- Alnaser, W. E., & Aldudiafa, H. S. (1990). Calculation of the global, diffused and direct solar radiation in Bahrain. Solar & Wind Technology, 7(2-3), 309–311. doi:10.1016/0741-983X(90)90101-7
- Alnaser, W. E., & Awadalla, N. S. (1990). Empirical model to estimate the global radiation in Bahrain by the knowledge of some astronomical parameters. Solar & Wind Technology, 7(5), 537–539. doi:10.1016/0741-983X(90)90059-B
- Alnaser, W. E., Awadalla, N. S., & Almoataz, L. A. (1992). Use of solar radiation measurement to detect the pollution of Bahrain’s sky caused by the Gulf War. Proceedings of the 1st Bahrain International Conference on Environment, Manama, Bahrain, February 24–26 (Vol. 1, pp. 309–323).
- Andrić, I., Koc, M., & Al-Ghamdi, S. G. (2019). A review of climate change implications for built environment: Impacts, mitigation measures and associated challenges in developed and developing countries. Journal of Cleaner Production, 211, 83–102. doi:10.1016/j.jclepro.2018.11.128
- Build. (2021). What climate factors are important considerations for building projects? Posted on 2nd June 2021. Retrieved from https://www.build-review.com/what-climate-factors-are-important-considerations-for-building-projects/
- Carpenter, S., Walker, B., Anderies, J. M., & Abel, N. (2001). From metaphor to measurement: Resilience of what to what? Ecosystems, 4(8), 765–781. doi:10.1007/s10021-001-0045-9
- Ceccato, P., Ramirez, B., Manyangadze, T., Gwakisa, P., & Thomson, M. C. (2018). Data and tools to integrate climate and environmental information into public health. Infectious diseases of Poverty, 7(1), 126. doi:10.1186/s40249-018-0501-9
- Centre for Disease Control and Prevention (CDC). (2022). Impact of the built environment on health. Retrieved from https://www.cdc.gov/nceh/publications/factsheets/impactofthebuiltenvironmentonhealth.pdf
- Chen, L., Cao, L., Zhou, Z., Zhang, D., & Liao, J. (2021). A new globally reconstructed sea surface temperature analysis dataset since 1900. Journal of Meteorological Research, 35(6), 911–925. doi:10.1007/s13351-021-1098-7
- Chen, Y., Moufouma-Okia, W., Masson-Delmotte, V., Zhai, P., & Pirani, A. (2018). Recent progress and emerging topics on weather and climate extremes since the fifth assessment report of the intergovernmental panel on climate change. Annual Review of Environment and Resources, 43(1), 35–59. doi:10.1146/annurev-environ-102017-030052
- Danny, H. W., Li, L. Y., & Lam, J. C. (2012). Impact of climate change on energy use in the built environment in different climate zones – A review. Energy, 42(1), 103–112. doi:10.1016/j.energy.2012.03.044
- Dervis, K. (2007). Devastating for the world’s poor. UN Chronicle Online Edition, 1–4. Retrieved from https://www.uncclearn.org/wp-content/uploads/library/undp30.pdf
- Duhoon, V., & Bhardwaj, R. (2021). Artificial intelligence technique for weather parameter forecasting. International Conference on Computational Performance Evaluation (ComPE), Shillong, India (pp. 098–102). doi:10.1109/ComPE53109.2021.9751934
- El Bakkush, A. F., Bondinuba, F. K., Bondinuba, F., & Harris, D. J. (2015). The effect of outdoor air temperature on the thermal performance of a residential building. Journal of Multidisciplinary Engineering Science and Technology (JMEST), 2(9), 2563–2570.
- Environmental Protection Agency (EPA). (2022). Basic information about the built environment. Retrieved from https://www.epa.gov/smm/basic-information-about-built-environment#builtenviron
- European Environment Agency (EEA). (2021). Climate change is one of the biggest challenges of our times. Retrieved from https://www.eea.europa.eu/themes/climate/climate-change-is-one-of
- Griggs, D., Stafford-Smith, M., Warrilow, D., Street, R., Vera, C., Scobie, M., & Sokona, Y. (2021). Use of weather and climate information essential for SDG implementation. Nature reviews. Earth & Environment, 2(1), 2–4. doi:10.1038/s43017-020-00126-8
- Hui, S. C. M., & Tsang, M. F. (2005). Climatic data for sustainable building design in Hong Kong. In Proceedings of the Joint Symposium 2005: New Challenges in Building Services, 15 November 2005, Hong Kong SAR, Climatic data for sustainable building design in Hong Kong. Retrieved from https://www.researchgate.net/publication/255575775_Climatic_data_for_sustainable_building_design_in_Hong_Kong
- Hung, N. Q., Babel, M. S., Weesakul, S., & Tripathi, N. K. (2009). An artificial neural network model for rainfall forecasting in Bangkok, Thailand. Hydrology and Earth System Sciences, 13(8), 1413–1425. doi:10.1155/2013/525383
- Kazemzadeh, M., Noori, Z., Jamali, S., & Abdi, A. M. (2022). Forty years of air temperature change over Iran reveals linear and nonlinear warming. Journal of Meteorological Research, 36(3), 462–477. doi:10.1007/s13351-022-1184-5
- Lacasse, M. A. (2019). An overview of durability and climate change of building components. Canadian Journal of Civil Engineering, 46(11), v–viii. doi:10.1139/cjce-2019-0625
- Litta, A. J., Idicula, S. M., & Mohanty, U. C. (2013). Artificial neural network model in prediction of meteorological parameters during premonsoon thunderstorms. International Journal of Atmospheric Sciences, 2013, 1–14. doi:10.1155/2013/525383
- Mabon, L., Kondo, K., Kanekiyo, H., Hayabuchi, Y., & Yamaguchi, A. (2019). Fukuoka: Adapting to climate change through urban green space and the built environment? Cities (London, England), 93, 273–285. doi:10.1016/j.cities.2019.05.007
- Nabipour, N., Mosavi, A., Hajnal, E., Nadai, L., Shamshirband, S., & Chau, K.-W. (2020). Modeling climate change impact on wind power resources using adaptive neuro-fuzzy inference system. Engineering Applications of Computational Fluid Mechanics, 14(1), 491–506. doi:10.1080/19942060.2020.1722241
- Otto-Zimmermann, K. (2010). Resilient cities: Cities and adaptation to climate change. Proceedings of the Global Forum 2010, Bonn, Germany ,Springer. doi:10.1007/978-94-007-4223-9
- PLANETCALC Online. (2022). Calculators function approximation with regression analysis. Retrieved from https://planetcalc.com/5992/
- Sebestyén, V., Czvetkó, T., & Abonyi, J. (2021). The applicability of big data in climate change research: The importance of system of systems thinking. Frontiers in Environmental Science, 9, 619092. doi:10.3389/fenvs.2021.61909
- Sharafati, A., & Pezeshki, E. (2020). A strategy to assess the uncertainty of a climate change impact on extreme hydrological events in the semi-arid Dehbar catchment in Iran. Theoretical and Applied Climatology, 139(1-2), 389–402. doi:10.1007/s00704-019-02979-6
- Sfetsos, A., & Coonick, A. H. (2000). Univariate and multivariate forecasting of hourly solar radiation with artificial intelligence techniques. Solar Energy, 68(2), 169–178. doi:10.1016/S0038-092X(99)00064-X
- Sharifi, A., & Yamagata, Y. (2018). Resilience-oriented urban planning. In Y. Yamagata & A. Sharifi (Eds.), Resilience-oriented urban planning. Lecture notes in energy (Vol. 65). Cham: Springer. doi:10.1007/978-3-319-75798-8_1
- Solomon, S., Plattner, G. K., Knutti, R., & Friedlingstein, P. (2009). Irreversible climate change due to carbon dioxide emissions. Proceedings of the National Academy of Sciences of the United States of America, 106(6), 1704–1709. doi:10.1073/pnas.0812721106
- Świąder, M., Szewrański, S., Kazak, J. K., Van Hoof, J., Lin, D., Wackernagel, M., & Alves, A. (2018). Application of ecological footprint accounting as a part of an integrated assessment of environmental carrying capacity: A case study of the footprint of food of a large city. Resources, 7(3), 52. doi:10.3390/resources7030052
- UNFCCC. (2017). Survey of adaptation actions and needs. United Nations Framework Convention on Climate Change. https://unfccc.int/news/survey-of-adaptation-actions-and-needs
- Zhao, G. H., Xinyue, Cui, X., Sun, J., Li, T., Wang, Q., … Ye, X., B. (2021). Analysis of the distribution pattern of Chinese Ziziphus jujuba under climate change based on optimized biomod2 and MaxEnt models. Ecological Indicators, 132, 108256. doi:10.1016/j.ecolind.2021.108256
- Zięba, Z., Dąbrowska1, J., Marschalko, M., Pinto, J., Mrówczyńska, M., Leśniak, A., … Kazak, J. K. (2020). Built environment challenges due to climate change. IOP Conference Series: Earth and Environmental Science, 609, 1–10. 10.1088/1755-1315/609/1/012061/pdf.