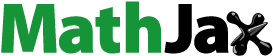
Abstract
An optimal control problem for the variable-order fractional-integer mathematical model of vaccination Covid 19 is presented in this research, where the order of the derivatives varies during the course of the time interval, becoming fractional or classical when it is more favourable. The variable-order derivatives are defined here using both the variable-order integral of Riemann–Liouville and the variable-order Caputo derivative. The existence, uniqueness, boundedness and positivity of the solutions are given. In order to test the rate for the detection of symptomatic infected people, two control variables are introduced. The optimality conditions are derived. The fractional-integer operator is approximated using Grünwald–Letnikov. Examples and comparative studies are presented to demonstrate the simplicity of the approximation approaches and the applicability of the utilized methods.
1. Introduction
Coronavirus has been designated by the world health organization (WHO) as a variant of serious concern. According to scientists, COVID-19 is more contagious compared to severe acute respiratory syndrome (SARS), because there are more routes of transmission and a large number of susceptible people. COVID-19 broke out worldwide at a rapid pace since the end of 2019, which had a tremendous impact on the lives of people around the world where it has claimed the lives of millions. Fighting the COVID-19 epidemic is difficult and expensive, and to control and prevent its spread of it, many governments have implemented very strict Epidemic prevention and control strategies, such as social distancing, self-isolation, medical quarantine, contact tracing, travel restrictions and city shutdown (WHO Int, Citation2020).
Fractional order mathematical models give more information about diseases (Ain, Anjum, & He, Citation2021; Ain et al., Citation2022; Ali, Alshammari, Islam, Khan, & Ullah, Citation2021; Anjum, He, & He, Citation2021; Arenas, Gonzàlez-Parra, & Chen-Charpentierc, Citation2016; Singh, Citation2020; Singh, Ganbari, Kumar, & Baleanu, Citation2021), the constant proportional Caputo fractional derivate (CPC) is proposed by Baleanu, Fernandez, and Akgül (Citation2020) and Sweilam, Al-Mekhlafi, and Baleanu (Citation2021). In epidemic applications constructed in terms of the variable order derivatives see the work by Samko, Kilbas, and Marichev (Citation1993), Samko and Ross (Citation1993), Sun, Chen, Wei, and Chen (Citation2011), Sweilam, Al‐Mekhlafi, and Shatta (Citation2020), Sweilam, Al-Mekhlafi, Mohammed, and Baleanu (Citation2020) and Sweilam and Al-Mekhlafi (Citation2016). Recently, Rosa and Torres (Citation2021) proposed a system of differential equations, the derivatives order varies along the interval of time, also, it is classical or fractional. This idea has many advantages. One such system, a variable order fractional system, is referred to herein as the FractInt system, which has been found to be useful in controlling the disease. Where the derivate order of the fractional-integer (FractInt) system varies accordingly
(1)
(1)
We paid special attention in this article to study the variable-order FracInt mathematical model of vaccination Covid-19 and their optimal control (Olivares and Staffetti, Citation2021). The variable-order derivatives are defined here using both the variable-order integral of Riemann–Liouville and the variable-order Caputo derivative. Moreover, the solution properties such as the existence, uniqueness, boundedness and positivity will be studied. We add two-control variables for the testing rate for detecting symptomatic infected individuals. The optimality conditions are derived. The FracInt operator is approximated using Caputo-proportional-constant Grünwald–Letnikov finite difference method (CPC-GLFDM).
To our knowledge, no numerical investigations of variable-order FracInt for COVID-19 vaccination mathematical model utilizing CPC-GLFDM have been conducted.
This article is organized as follows: Some notations and definitions of variable order fractional derivatives are introduced in Section 2. In Section 3, the model with a hybrid variable-order FracInt with control variables is presented, moreover, the positivity, boundedness, existence, uniqueness of the solutions and the stability are discussed. The numerical method CPC-GLFDM are constructed, moreover, the stability analysis for the proposed method is studied in Section 4. In Section 5, numerical simulations are presented. The conclusions are ultimately outlined in Section 6.
2. Basic definitions
Definition 2.1.
Riemann–Liouville’s left and right (L-R) sides integral (Podlubny,Citation1999):
(2)
(2)
(3)
(3)
where
is a continuous function and
Definition 2.2.
The right-side and the left-side Caputo’s derivatives of order for a function
are defined, respectively by (Podlubny,Citation1999)
(4)
(4)
(5)
(5)
where
Moreover, if (Almeida & Torres, Citation2011):
Proposition 2.1
(Podlubny, Citation1999). Let L-R sides Caputo’s and Riemann–Liouville’s fractional derivatives of given respectively
then
(6)
(6)
(7)
(7)
for
We can prove that
if
where s = a or b, as in Definition 2.2.
Definition 2.3.
The variable order hybrid operator CPC (Baleanu et al., Citation2020):
(8)
(8)
where,
, Q is a constant.
On the other side, we can define (Baleanu et al., Citation2020):
(9)
(9)
where,
is the CPC inverse operator.
3. Mathematical model of FractInt variable order
FractInt variable order COVID-19 model (Olivares and Staffetti, Citation2021) is developed here. Also, two control variables and
are added;
denotes the vaccination rate, ranging from 0 to
where
is an upper bound to be provided by the designers of the optimal control model. Therefore, the vaccination policy is represented in the model by the term
where the parameter
represents the vaccine efficacy level.
denotes the test rate for the detecting of asymptomatic infected people, which ranges from
to
where
and
represent a lower and an upper bound, respectively, be provided by the designers of the optimal control model. A new parameter
is presented in order to be consistent with the physical model problem. Moreover, we avoid dimensional mismatches by modifying the fractional model with an auxiliary parameter
As a result, the left side possesses the dimension
(Ullah & Baleanu, Citation2021).
(10)
(10)
subject to the following positive values:
(11)
(11)
for more details on the variables meaning of the proposed model see .
Table 1. All variables definitions of Eq. (Equation10(10)
(10) ).
By adding all equations of Equation(10)(10)
(10) , boundedness of the proposed model solution can be verified as follows:
(12)
(12)
where,
is the total population summation and A is a constant. The solution of EquationEq. (12)
(12)
(12) is given as follows:
(13)
(13)
At solutions of EquationEq. (10)
(10)
(10) are bounded.
Lemma 3.1.
All solutions of EquationEq. (10)(10)
(10) are nonnegative under conditions Equation(11)
(11)
(11) for
(Lin Citation2007).
Proof.
Using EquationEq. (11)(11)
(11) , we have:
(14)
(14)
□
3.1. Existence and uniqueness
System Equation(10)(10)
(10) can be written as follows
(15)
(15)
where, the state variables are
and
is defined as follow
with an initial condition
Furthermore, Lipschitz requirement holds (Bonyah, Sagoe, Kumar, & Deniz, Citation2021):
(16)
(16)
Theorem 3.2.
If the following condition holds,
(17)
(17)
the hybrid model Equation(10)
(10)
(10) has a unique solution
Proof.
Using EquationEqs. (8)(8)
(8) and Equation(15)
(15)
(15) , we have
(18)
(18)
Let and
achieved that:
(19)
(19)
We have:
The supremum norm on Q is represented by Thus
Then, with
with
achieved that:
Also, relation Equation(19)(19)
(19) can be written as
(20)
(20)
Then
(21)
(21)
where
So EquationEq. (10)(10)
(10) has a unique solution. □
4. The control problem
Let, the admissible control set
The objective functional:
(22)
(22)
and
are the weight constants. The control problem is find
such that
(23)
(23)
is minimum. It is subject to the constraints
(24)
(24)
Where
In the following using (Agrawal, Citation2008; Sweilam, Al-Mekhlafi, Alshomrani, & Baleanu, Citation2020; Sweilam, Al-Mekhlafi, Mohammed, et al., Citation2020; Sweilam, Al-Mekhlafi, Albalawi, & Tenreiro Machado, Citation2021) we extend numerically optimality conditions
We define the Hamiltonian:
(25)
(25)
The necessary conditions of optimal control as in (Agrawal, Citation2008; Sweilam, Al-Mekhlafi, Alshomrani et al., Citation2020; Sweilam, Al-Mekhlafi, Mohammed et al., Citation2020; Sweilam et al., Citation2021) are
(26)
(26)
where,
(27)
(27)
(28)
(28)
(29)
(29)
Now, there exists
that minimizes
over
with
Furthermore, there exists adjoint variables
satisfy the following
Adjoint equations:
i.e., this is transversality conditions.
Optimality conditions:
(32)
(32)
Moreover:
(33)
(33)
(34)
(34)
EquationEquations (30)(30)
(30) can be obtained from EquationEq. (26)
(26)
(26) , where
(35)
(35)
is the Hamiltonian.
We can obtain EquationEqs. (33)
(33)
(33) and Equation(34)
(34)
(34) from EquationEq. (32)
(32)
(32) .
We rewrite EquationEq. (10)(10)
(10) using EquationEqs. (33)
(33)
(33) and Equation(34)
(34)
(34)
(36)
(36)
5. Numerical method for solving FracInt optimality systems
5.1. CPC-GLFDM
Let
(37)
(37)
Using EquationEq. (8)(8)
(8) we have:
(38)
(38)
Now by using GL-approximation, we have:
(39)
(39)
(40)
(40)
is a natural number.
Also, let us assume that (Scherer, Kalla, Tang, & Huang, Citation2011):
EquationEquation (40)(40)
(40) is used to solve EquationEq. (30)
(30)
(30) . Moreover, using the same technique with backward in time, EquationEq. (36)
(36)
(36) with transitivity condition can be discretized.
Remark 1.
If and
in Equation(40)
(40)
(40) , this is the disceritization of Caputo opretor using finite differance method (C-GLFDM).
5.2. CPC-GLFDM stability analysis
Consider the following test problem:
(41)
(41)
Thanks to EquationEq. (38)(38)
(38) then, EquationEq. (41)
(41)
(41) can be discretized as follows:
(42)
(42)
put
Then from boundness theorem (Arenas et al., Citation2016) we have:
(43)
(43)
then we have:
and
Now by discretize EquationEq. (38)(38)
(38) :
(44)
(44)
(45)
(45)
where,
The proposed method is convergent because it is consistent and stable (Yuste & Quintana-Murillo, Citation2012).
6. Numerical experiments
This section examines the simulations of optimality systems EquationEqs. (30)(30)
(30) and Equation(36)
(36)
(36) . The parameter values in are used with the following initial conditions:
and transversality conditions
CPC-GLNFDM Equation(40)
(40)
(40) are presented to study EquationEqs. (30)
(30)
(30) with Equation(31)
(31)
(31) . EquationEquation (36)
(36)
(36) will be discretized using the same technique and backward in time, then we solve algebraic system of equations. The numerical results of the optimality systems Equation(30)
(30)
(30) and Equation(36)
(36)
(36) are shown graphically at different values of
if
and
if
in . shows the behavior of the solution of the proposed model before and after the optimal control when
if
if
We found that, in the controlled case, the detected infected people with life-threatening symptoms is lower. Also, the number of deceased people are less in the controlled case. This has enabled us to reduce the number of confirmed infections with life-threatening symptoms and the number of deaths. Our results in show that controlling Covid-19 infection through optimal control is effective and that the FracInt model is the best one. shows the behavior of the control variables at variable-order FracInt
when
if
if
and classical order when
and
Also, we noted the variable-order FracInt model is the best one. and show how the behavior of the solutions in controlled case varies with the values of
where
if
if
and show the objective functional values in FracInt case and the classical case. Clear that values of objective functional 22 in case of the variable-order FracInt are better than the classical one.
Table 2. System (Equation10(10)
(10) ) parameters.
Table 3. Comparison between the J values and
Table 4. Comparison between the values of J,
using different methods.
7. Conclusions
In the present article, we paid special attention to study the hybrid variable-order FracInt mathematical model of vaccination Covid-19 and their optimal control. The variable-order derivative is defined using both the variable-order integral of Riemann–Liouville and the variable-order Caputo derivative. It is more general than Caputo fractional operator. The stability analysis of the proposed model was discussed, as well as the existence of equilibrium points. In this model, boundedness, positivity and stability are proved. is introduced to make the model compatible with the physical problem. The optimality conditions are numerically derived. CPC-GLNFDM is developed to study the optimality system. Generally, the numerical results show that the proposed FracInt system is effective in controlling the disease. Generally, the numerical findings demonstrate that the FOC system performs better only during a portion of the time period. In order to combat infection, we therefore suggest a system in which the derivative order changes over the course of the time interval, becoming fractional or integer as appropriate. This FracInt-named variable-order fractional model appears to be the most successful at controlling the illness. In the future, the present study can be extended to FracInt delay optimal control.
Disclosure statement
The authors have declared no conflict of interest.
Correction Statement
This article has been corrected with minor changes. These changes do not impact the academic content of the article.
References
- Agrawal, O. P. (2008). A formulation and numerical scheme for fractional optimal control problems. Journal of Vibration and Control, 14(9–10), 1291–1299. doi:10.1177/1077546307087451
- Ain, Q. T., Anjum, N., & He, C.-H. (2021). An analysis of time-fractional heat transfer problem using two-scale approach. GEM—International Journal on Geomathematics, 12(1), 18. doi:10.1007/s13137-021-00187-x
- Ain, Q. T., Anjum, N., Din, A., Zeb, A., Djilali, S., & Khan, Z. A. (2022). On the analysis of Caputo fractional order dynamics of Middle East Lungs Coronavirus (MERS-CoV) model. Alexandria Engineering Journal, 61(7), 5123–5131. doi:10.1016/j.aej.2021.10.016
- Ali, A., Alshammari, F. S., Islam, S., Khan, M. A., & Ullah, S. (2021). Modeling and analysis of the dynamics of novel coronavirus (COVID-19) with Caputo fractional derivative. Results in Physics, 20, 103669. doi:10.1016/j.rinp.2020.103669
- Almeida, R., & Torres, D. F. M. (2011). Necessary and sufficient conditions for the fractional calculus of variations with Caputo derivatives. Communications in Nonlinear Science and Numerical Simulation, 16(3), 1490–1500. doi:10.1016/j.cnsns.2010.07.016
- Anjum, N., He, C.-H., & He, J.-H. (2021). Two-scale fractal theory for the population dynamics. Fractals, 29(07), 2150182. doi:10.1142/S0218348X21501826
- Arenas, A. J., Gonzàlez-Parra, G., & Chen-Charpentierc, B. M. (2016). Construction of nonstandard finite difference schemes for the SI and SIR epidemic models of fractional order. Mathematics and Computers in Simulation, 121, 48–63. doi:10.1016/j.matcom.2015.09.001
- Baleanu, D., Fernandez, A., & Akgül, A. (2020). On a fractional operator combining proportional and classical differintegrals. Mathematics, 8(3), 360. doi:10.3390/math8030,
- Bonyah, E., Sagoe, A. K., Kumar, D., & Deniz, S. (2021). Fractional optimal control dynamics of Coronavirus model with Mittag-Leffler law. Ecological Complexity, 45, 100880. doi:10.1016/j.ecocom.2020.100880
- Eikenberry, S. E., Mancuso, M., Iboi, E., Phan, T., Eikenberry, K., Kuang, Y., … Gumel, A. (2020). To mask or not to mask: Modeling the potential for face mask use by the general public to curtail the COVID-19 pandemic. Infectious Disease Modelling, 5, 293–308. doi:10.1016/j.idm.2020.04.001
- Lin, W. Global existence theory and chaos control of fractional differential equations. J. Math. Anal. Appl. 2007, 332, 709–726.
- Olivares, A. and Staffetti, E., Optimal Control Applied to Vaccination and Testing Policies for COVID-19, Mathematics, 2021, 9, 3100. https://doi.org/10.3390/math9233100
- Podlubny, I. (1999). Fractional differential equations. New York: Academic Press.
- Rosa, S., & Torres, D. F. M. (2021). Fractional-order modelling and optimal control of Cholera transmission. Fractal and Fractional, 5(4), 261. doi:10.3390/fractalfract5040261
- Samko, S. G., & Ross, B. (1993). Integration and differentiation to a variable fractional order. Integral Transform and Special Functions, 1(4), 277–300. doi:10.1080/10652469308819027
- Samko, S. G., Kilbas, A. A., & Marichev, O. I. (1993). Fractional integrals and derivatives: Theory and applications. New York: Gordon and Breach Science Publishers.
- Scherer, R., Kalla, S., Tang, Y., & Huang, J. (2011). The Grünwald-Letnikov method for fractional differential equations. Computers & Mathematics with Applications, 62(3), 902–917. doi:10.1016/j.camwa.2011.03.054
- Singh, J. (2020). Analysis of fractional blood alcohol model with composite fractional derivative. Chaos, Solitons and Fractals, 140, 110127. doi:10.1016/j.chaos.2020.110127
- Singh, J., Ganbari, B., Kumar, D., & Baleanu, D. (2021). Analysis of fractional model of guava for biological pest control with memory effect. Journal of Advanced Research, 32, 99–108. doi:10.1016/j.jare.2020.12.004
- Sun, H. G., Chen, W., Wei, H., & Chen, Y. Q. (2011). A comparative study of constant-order and variable-order fractional models in characterizing memory property of systems. The European Physical Journal Special Topics, 193(1), 185–192. doi:10.1140/epjst/e2011-01390-6
- Sweilam, N. H., & Al-Mekhlafi, S. M. (2016). Numerical study for multi-strain tuberculosis (TB) model of variable-order fractional derivatives. Journal of Advanced Research, 7(2), 271–283. doi:10.1016/j.jare.2015.06.004
- Sweilam, N. H., Al-Mekhlafi, S. M., & Baleanu, D. (2021). A hybrid fractional optimal control for a novel Coronavirus (2019-nCov) mathematical model. Journal of Advanced Research, 32, 149–160. doi:10.1016/j.jare.2020.08.006
- Sweilam, N. H., Al-Mekhlafi, S. M., & Shatta, S. A. (2020). On the awareness programs of the epidemic outbreaks fractional model. Journal of Fractional Calculus and Applications, 11(1), 26–40.
- Sweilam, N. H., Al-Mekhlafi, S. M., Albalawi, A. O., & Tenreiro Machado, J. A. (2021). Optimal control of variable-order fractional model for delay cancer treatments. Applied Mathematical Modelling, 89, 1557–1574. DOI: , (2020). doi:10.1016/j.apm.2020.08.012
- Sweilam, N. H., Al-Mekhlafi, S. M., Alshomrani, A. S., & Baleanu, D. (2020). Comparative study for optimal control nonlinear variable-order fractional tumor model. Chaos, Solitons and Fractals, 136, 109810. doi:10.1016/j.chaos.2020.109810
- Sweilam, N. H., Al-Mekhlafi, S. M., Mohammed, Z. N., & Baleanu, D. (2020). Optimal control for variable order fractional HIV/AIDS and malaria mathematical models with multi-time delay. Alexandria Engineering Journal, 59(5), 3149–3162. (2020). doi:10.1016/j.aej.2020.07.021,
- Ullah, M. Z., & Baleanu, D. (2021). A new fractional SICA model and numerical method for the transmission of HIV/AIDS. Mathematical Methods in the Applied Sciences, 44(11), 8648–8659. doi:10.1002/mma.7292
- WHO Int. (2020). World Health Organization: Advice for the public. Retrieved from https://www.who.int/emergencies/diseases/novel-coronavirus-2019/advice-for-public.
- Yuste, S. B., & Quintana-Murillo, J. (2012). A finite difference method with non-uniform time steps for fractional diffusion equations. Computer Physics Communications, 183(12), 2594–2600. doi:10.1016/j.cpc.2012.07.011