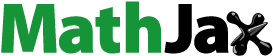
Abstract
In this paper, the fractional order analysis of the behaviour of the four-dimensional chronic wasting disease (CWD) communities model has been presented. The complexity and dynamical behaviour of the CWD model have been calculated using two fractional derivatives. CWD, a neurological disease that affects deer, has resulted in many deaths and infections among deer populations around the world. To better understand and tackle this eco-epidemiological issue, we used two numerical schemes utilizing the Caputo fractional operator and the Atangana-Baleanu (AB) fractional operator. We have investigated the stability of the eco-epidemiological CWD model. The fixed point theory is a volumetric role play in analyzing the existence and uniqueness of the solution. We use bifurcation diagrams, time series diagrams, and phase diagrams to analyze fractional-order eco-epedimological systems with derivative orders and parameters varying. We examine the approximate result of the eco-epidemiological CWD model with the Atangana-Baleanu (AB) operator and the Caputo operator, and we also compare both solutions. We do a brief analysis of the simulated results, which reveals that the suggested methodologies are novel, dependable, and remarkably easy to implement. In addition to determining the direction, stability, bifurcating, and numerical solutions, graphic depiction and graph bifurcation provide better information about the proposed model.
1. Introduction
Fractional calculus has become increasingly important in various interdisciplinary fields such as Mathematics, Physics, Biology, and Engineering. Fractional calculus is associated with Mathematics, which is as old as calculus, and it deals with arbitrary orders of differentiation and integration (Mainardi, Citation2012). In recent times, a lot of biological models have been analyzed using fractional derivatives (Caputo, Citation1969; Kumar, Alshahrani, Yakout, Abdel-Aty, & Kumar, Citation2021). The Caputo fractional operator is the most commonly used operator for simulating real-world problems. The Riemann-Liouville (RL) operator is also closely related to the Caputo fractional operator, but the main difference between them is the singularity quality of the kernels. Using these operators may not always lead to better results when studying the dynamics of various models. Therefore, researchers suggest using fractional operators with non-singular kernels to better understand the dynamics of models. Atangana and Baleanu developed a non-singular kernel derivative operator using the Mittag-Leffer function, which has a non-local and non-singular kernel, making it particularly useful for individuals engaged in numerical modelling of real-world problems. The Atangana-Baleanu (AB) derivative has gained significant importance and popularity in recent decades in various fields, particularly biological models (Alkahtani & Atangana, Citation2016; Kumar, Alshahrani, et al., Citation2021). In fractional calculus, many derivatives are there but the Riemann Liouville (RL), Caputo, and Atangana-Baleanu (AB) operators play major roles in the analysis of biological models but still need more particular attention (Losada & Nieto, Citation2015; Uçar, Uçar, Özdemir, & Hammouch, Citation2019). Fractional order derivatives can be used to illustrate a variety of natural occurrences and facts that have non-local, intricate dynamical behaviour. When examining the system, such operators make it easier to solve the system. As is well known, biological models have memory effects and hereditary characteristics, and fractional calculus is a better way to describe them. Fractional-order systems, as opposed to integer-order systems, results that are more accurate when describing the complicated behaviour of epidemic diseases. In this paper, the authors introduce a new method for modelling the dynamics of influenza A disease and the Caputo-Fabrizio fractional derivative operator into the model, a more accurate representation of the disease’s behaviour (Evirgen, Esmehan, Sümeyra, & Özdemir, Citation2023). In this paper, Veeresha et al. have given information about Zika virus disease spread through the bite of an infected mosquito from the Aedes species. It can become a severe epidemic if not controlled during its early stages. The nonlinear partial differential model is analyzed and solved using the q-homotopy analysis transform method. The Atangana-Baleanu (AB) definition of fractional derivative is utilized in the process (Veeresha, Akinyemi, Oluwasegun, Şenol, & Oduro, Citation2022).
Chronic wasting disease (CWD) is a highly infectious and dangerous disease that affects deer (Williams & Young, Citation1980). The disease was first identified in captive mule deer at a wildlife research lab in northern Colorado, USA, and southeastern Wyoming in 1967 (Sigurdson, Citation2008). In 1996, CWD was discovered among free-ranging deer and elk in northeastern Colorado and southeastern Wyoming. Apart from North America, this disease has only been found in South Korea, where it is believed to have originated from Canada (Miller et al., Citation2000). From 1996 to 1999, the prevalence of this disease was estimated to be approximately 2% in white-tailed deer, less than 1% in elk, and 5% in mule deer, based on disease surveillance of harvested animals in this area (Miller et al., Citation2000). Captive deer and elk are highly susceptible to this disease and it can spread quickly among them. However, we still do not fully understand the source and transmission mechanisms of the prions that cause CWD.
In the past, Chronic Wasting Disease (CWD) used to be considered a rare and foreign disease. However, in recent years, this disease has become increasingly concerning as it has emerged as a transmissible branch of prion diseases (Barlow, Citation1996; Lafferty & Holt, Citation2003). The most effective ways to eliminate diseases are through vaccination and prevention. Unfortunately, there is still no vaccination strategy available for lethal illnesses such as CWD. This disease is quite peculiar as it affects both farmed and wild animals. Animals that are infected with CWD can directly and indirectly affect other animals. When these infected animals leave behind excrement and carcasses, they can be harmful to susceptible animals. To truly understand the disease and its incidence in wildlife, we need to conduct long-term investigations. The narration of the symptoms of a certain disease is derived from the scanning of infected animals (Thompson Hobbs, Citation2006). This disease can be transmitted naturally through bodily fluids such as sputum, urine, and faeces. Infected deer blood in platelets and B cells carries the infectiousness but plasma is not infected. Recent research suggests that CWD prions may also be present in the skeletal muscle of infected animals. Symptoms of this disease in animals include excessive salivation, physiological changes, increased thirst, loss of coordination, changes in behaviour, difficulty swallowing, and more. CWD prions can remain in the environment for at least two years, making the disease highly infectious for the cervine family, including bucks, moose, and elk (Hsieh & Hsiao, Citation2008; Mukherjee, Citation2003; Citation2003; Venturino, Citation2002). Unfortunately, there is currently no perfect medication or treatment for this disease. The primary approach to managing the disease is to hunt infected animals and remove them from their families. In this article, we aim to analyze the eco-epidemic model with indirect transmission and explore ways to control CWD transmission through predation. A reliable eco-epidemiological model can provide vital information about diseases, and further research in this field can be conducted with the assistance of such models.
Some numerical schemes have been developed as mathematical tools for solving biological models and fractional-order differential equations (Kumar, Kumar, & Jleli, Citation2020). Recently, a new fractional operator was created to address issues with the power-law kernel and the existing fractional operator. Literature is abundant on various numerical methods, such as the predictor-corrector method (Evirgen, Esmehan, et al., 2023; Citation2023; Joshi & Yavuz, Citation2023), Newton interpolation formula (Rahman, Arfan, & Baleanu, Citation2023), Adam-Bashforth method, Toufik-Atangana technique (Joshi, Yavuz, Townley, & Jha, Citation2023) and Runge-Kutta technique (Fatima, Yavuz, Ur Rahman, & Al-Duais, Citation2023). The Toufik-Atangana approach is highly regarded as an effective numerical technique for solving non-linear systems (Khan & Atangana, Citation2020; Toufik & Atangana, Citation2017). To overcome the shortcomings of the popular Adams-Bashforth approach, the Toufik-Atangana numerical system is introduced. The novel numerical approach has the combination of the two-step Lagrange polynomial and the fundamental theorem of fractional calculus. This approach converges to the solution rapidly and with great accuracy. We used this numerical technique for investigating the eco-epidemiological system with the help of the Atangana-Baleanu (AB) fractional operator (Toufik & Atangana, Citation2017). In this paper, we have used this scheme with different fractional operators in this biological model.
The work is organized as follows: Section 2 examines fractional calculus definitions and basic concepts. In section 3, we examine Caputo and Atangana-Baleanu’s (AB) non-integer eco-epidemiological model. In section 4, we examine equilibrium points and stability. In section 5, we address the uniqueness and existence of the proposed model solution. In section 6, we present the numerical technique for the Toufik-Atangana (TA) with the Caputo operator. TA numerical scheme using the ABC operator is presented in section 7. Graphical analysis and numerical results are presented in section 8. The study’s conclusions are discussed in section 9.
2. Definitions and basic concepts
In this portion, we have present some essential elucidation, theorems, and symbols of the fractional calculus theory that are applied in the task.
Definition 2.1
(Uçar et al., Citation2019) Suppose that
and
so the Caputo fractional differential operator is defined as follows
(1)
(1)
denotes a gamma function.
Definition 2.2.
The Caputo integral is defined as follows
(2)
(2)
Definition 2.3
(Uçar et al., Citation2019). Suppose that
[0,1]. The Atangana-Baleanu (AB) operator in Riemann-Liouville type is defined as follows
(3)
(3)
It is usually the case that B(0) = B(1) = 1, and is a normalization function.
Definition 2.4
(Uçar et al., Citation2019) Suppose that
and
[0,1]. Then the Atangana-Baleanu (AB) operator in Caputo type is defined as follows
(4)
(4)
Definition 2.5
(Atangana & Koca, Citation2016) Suppose that be the base point and
is the order of operator in the Atangana-Baleanu (AB) integral operator is defined as follows
(5)
(5)
Theorem 2.1
(Chen, Petras, & Xue, Citation2009; Irudayaraj et al., Citation2020; Santosh Kumar, Citation2014) Matignon’s stability theorem and its condition is
(6)
(6) where 0 <
< 1 and
(i.e
) represents the eigenvalues of matrix
3. Caputo and Atangana-Baleanu fractional model of eco-epidemiological
Some years ago, CWD was called zombie deer disease. This deadly- infectious and neurological infection influences the deer family. Based on experiments, it has been seen that CWD can be transmitted to vulnerable animals through the residues of excreta that are left behind in the environment by infected animals and their carcasses (Packer, Holt, Hudson, Lafferty, & Dobson, Citation2003). This mode of transmission is considered older than the traditional models of direct contact between animals (Miller & Wild, Citation2004). It can be challenging to observe these diseases thoroughly since a prolonged epizootic may induce low, frequently undiagnosed infection morbidity or mortality. Long-term investigations will be beneficial to comprehending the incidence and geographical dynamics of chronic wildlife disease. It’s significant to understand the patterns and dynamics of chronic disease in wildlife, and one way to do that is through modelling the complexity of the situation. By doing so, we can gain a better understanding of how these diseases spread over time and space, which can help us develop effective strategies for managing and preventing them. Although CWD prions can remain contagious in the environment for years, relatively little is known about the possible consequences of indirect CWD dynamic transfer. The proposed model obeyed a hunt-predator method and split the hunt population into two parts, the first part infected animals and the second part non-infected animals. The susceptible-infected-recovered (SIR) model in infection transfer among humans is the foundation of the eco-epidemiological model (Allen, Brauer, Van den Driessche, & Wu, Citation2008). This model is expressed in terms of nonlinear equations. Hunter’s functional reaction is supposed to be the Holling type II. Based on the aforementioned, the integer-order CWD model (Maji, Mukherjee, & Kesh, Citation2018) is as follows:
(7)
(7)
with initial conditions S(0) > 0, I(0) > 0, E(0) > 0, P(0)
0.
In this scenario, S represents the density of the susceptible animals, while I denotes the infected ones. E represents the mass of infectious material in the environment, and P is the density of the predator. In this context, the various parameters are defined as follows:
r represents the maximum per-capita growth rate,
d represents the death rate of the predator,
g represents the environmental carrying capacity of the prey,
ϱ represents the mass-specific rate of loss of infectious material from the environment,
ν represents the death rate of infected animals through CWD,
ϵ represents the per-capita rate of excretion of infectious material by infected animals,
a represents the predation coefficient,
m represents a half-saturation constant,
b represents the conversion coefficient of the predator,
β represents the indirect transmission coefficient for the disease.
When animals that are vulnerable to infection come in contact with infectious material in their surroundings, they have contracted the disease. These infected animals can then transmit the infection to others through contact with the contaminated environment, thus facilitating the spread of the disease. This model can be applied to the spread of various other diseases such as tuberculosis in livestock (Brennan, Kemp, & Christley, Citation2008), viral hepatitis A (Ajelli, Iannelli, Manfredi, & Ciofi Degli Atti, Citation2008), Vibrio cholerae (Das & Mukherjee, Citation2012), avian influenza (Breban, Drake, Stallknecht, & Rohani, Citation2009), and so on.
The fractional Caputo derivative is applied in system Equation(7)(7)
(7) . Thus, the existing model is replaced by the following model:
(8)
(8)
with initial conditions S(0) > 0, I(0) > 0, E(0) > 0, P(0)
0.
To gain a fractional derivative model, we operate the Atangana-Baleanu operator in system Equation(7)(7)
(7) . We found the new model is an expression of the Atangana-Baleanu (AB) operator:
(9)
(9)
with initial conditions S(0) > 0, I(0) > 0, E(0) > 0, P(0)
0.
4. Equilibrium points and stability analysis
The fractional-order eco-epidemiological model is employed here to examine its stability. At first, our work is to find out the equilibrium points of the system Equation(9)(9)
(9) .
(10)
(10)
We have found four equilibrium points:
Table
Let (S, I, E, P) be any point, then the Jacobian matrix for system Equation(9)(9)
(9) is described as
(11)
(11)
In this portion, we have investigated the behaviour of the equilibrium points of the fractional epidemiological system Equation(9)(9)
(9) with the help of the Matignon condition and the Jacobian matrix.
Theorem 4.1.
The trivial equilibrium point is unstable.
Proof 4.1.
The Jacobian matrix Equation(11)(11)
(11) evaluated at the equilibrium point
The eigenvalues of are
= r,
=
=
and
=—d. With the help of Matignon’s condition, we observed that
= π >
, i = 2, 3, 4 for all 0 <
< 1. But
does not satisfy the Matignon condition. Therefore equilibrium point E0 is unstable.
Theorem 4.2.
The equilibrium point of system Equation(9)
(9)
(9) is
If ν ϱ >
then the equilibrium point E1 is locally asymptotically stable (LAS).
If ν ϱ <
then the equilibrium point E1 is unstable.
Proof 4.2.
The Jacobian matrix Equation(11)(11)
(11) evaluated at the equilibrium point
The characteristic equation of is following as
Therefore, eigenvalues are =—r,
=
= −d and
=
. All the eigenvalues have negative real parts, then
= π >
for all 0 <
< 1. So, we can say that Matiginon’s condition hold, if
>
. Then the equilibrium point E1 is locally asymptotically stable (LAS). We assume that if
<
then the Matiginon’s condition not holds. Hence, the equilibrium point E1 is unstable in this condition.
Theorem 4.3.
The equilibrium point of system Equation(9)
(9)
(9) is
(i) If
> g >
and d <
then the equilibrium point E2 is locally asymptotically stable.
Proof 4.3.
The Jacobian matrix Equation(11)(11)
(11) around the equilibrium point E2 is given as
So, the characteristic equation of is following as
and
is discriminant of the polynomial
When
, and
are greater than zero and
-
> 0 and
in this case the equilibrium point E2 is LAS.
When
and
in this case the equilibrium point E2 is LAS.
First, we assume that , and
are greater than zero and
-
> 0. We see clearly one of the root of the characteristic equation is negative (i.e
=
). With the use of given conditions and our assumption, we found that each of the eigenvalues has negative real parts. Consequently, the equilibrium point
is satisfied Matignon’s condition (i.e
>
) and the present point is locally asymptotically stable.
Theorem 4.4.
The equilibrium point is locally asymptotically stable (LAS) or unstable.
Proof 4.4.
Now, the equilibrium point E3 putting in the Jacobian matrix Equation(11)(11)
(11) , we have
Therefore, has the characteristic equation
We analyze the behavior of equilibrium point E3 and is discriminant of the polynomial
(i) Let and
are Routh-Hurwitz determinants
when
equilibrium point E3 to be LAS
and this is sufficient conditions for this point.
(ii) When and
, in this case the equilibrium point E3 is unstable.
(iii) When and
, in this case point E3 is LAS. Again, when
in this case E3 is unstable.
(iv) When and
in this case the point E3 is LAS
(v) E3 equilibrium point is LAS, necessary condition for this point is if
5. Essential uniqueness and existent for the solution for the model
Within this segment, we investigate the essential uniqueness and existent of the solution for the Atangana-Baleanu fractional model with system Equation(9)(9)
(9) . Applying the AB-fractional integral operator into system Equation(9)
(9)
(9) , we obtain
(12)
(12)
We define the following kernels:
(13)
(13)
Theorem 5.1.
If only the undermentioned dissimilitude holds:
Then the kernel N1 convinces the Lipschitz condition and contraction mapping.
Proof 5.1.
We assume that S and S1 are any two functions, so we get
Let , ourself do suppose that S, I, E, and P are positive and bounded functions, i.e.
and
where θ1, θ2, θ3 and θ4 are some positive constants.
(14)
(14)
Hence, kernel N1 is satisfies the Lipschitz condition and since is also a contraction.
In this, similar way for the kernels N2, N3 and N4 can be obtained using , and
respectively, as follow:
(15)
(15)
With help of Equationequations (14)(14)
(14) and Equation(15)
(15)
(15) , we apply the above mentioned kernels, then system Equation(12)
(12)
(12) becomes
(16)
(16)
We now introduce the following recursive formulas:
(17)
(17) where the initial condition are
The differences between the consecutive terms for the recursive formulas can be written as
(18)
(18)
(19)
(19)
(20)
(20)
(21)
(21)
Note that:
(22)
(22)
We solve the Equationequation (18)(18)
(18) , and the norm is applied on both sides of the Equationequation (18)
(18)
(18) , then we obtain
(23)
(23)
Applying the triangle inequality on Equationequation (23)(23)
(23) , we obtain
(24)
(24)
So the kernel N1 propitiate the Lipschitz condition with Lipschitz constant , we find
(25)
(25) thus, we obtain
(26)
(26)
In a similar manner, we get
(27)
(27)
Theorem 5.2.
If there exists a time , then following inequalities hold:
(28)
(28) then proposed model of solution exist.
Proof 5.2.
We know that and P(t) are bounded and the kernels satisfy Lipschitz condition.Using Equationequations (26)
(26)
(26) and Equation(27)
(27)
(27) , and applying the recursive method, we find
(29)
(29)
We will prove the above functions are solutions of system Equation(9)(9)
(9) . Let assume that
(30)
(30)
Then, we have
(31)
(31)
Repeating this process recursively, it becomes
(32)
(32)
At the point t0, we get
(33)
(33)
As e tends to infinity,then limit in the Equationequation (33)(33)
(33) , we get
Similarly, we get
As a result, the existence of proposed model is proven.
Theorem 5.3.
The system Equation(9)(9)
(9) has a unique solution if satisfies the following conditions:
(34)
(34)
Proof 5.3.
Let assume that and
be different solutions of the proposed model. We have
(35)
(35) taking norm on both sides,
(36)
(36) the kernel satisfies Lipschitz condition, so
then
So
we have
(37)
(37)
Like this way, we obtain
(38)
(38)
Therefore, the proof is completed for a unique solution of the fractional eco-epidemiological system.
6. Toufik-Atangana (TA) numerical scheme for eco-epidemiological model with Caputo operator
In this paper, authors have achieved various numerical simulations and results through the Toufik-Atangana scheme for host-parasitoid model (Kumar, Alshahrani, et al., Citation2021). We operate the Caputo fractional derivative in system Equation(7)(7)
(7) . We obtain new model in terms of Caputo sense:
(39)
(39)
First, assume we have the non-integer differential equation below:
(40)
(40)
Integrate Equationequation (40)(40)
(40) using fractional integrals
(41)
(41)
when
in Equationequation (41)
(41)
(41) , then we get
(42)
(42)
We are estimated the function with the help of the two-step Lagrange polynomial interpolation over the closed interval
and we also use the polynomial interpolation concept. Thus
(43)
(43)
With help of Equationequations (42)(42)
(42) and Equation(43)
(43)
(43) , we get
(44)
(44)
Toward simplifying the Equationequation (44)(44)
(44) , we defined the undermentioned equations
(45)
(45)
(46)
(46)
The approximate solution of Equationequation (44)(44)
(44) is obtain with help of Equationequations (45)
(45)
(45) and Equation(46)
(46)
(46) , then we get
(47)
(47)
the numerical solution for proposed model are given as
(48)
(48)
(49)
(49)
(50)
(50)
(51)
(51)
where
7. Toufik-Atangana (TA) numerical scheme for eco-epidemiological model with ABC-operator
Fractional derivative with the biological model is very complicated work to find solutions because of their nonlinearity (Atangana & Baleanu, Citation2016). So many numerical methods are used for the solution of the fractional biological model. We present the complete details of this method how to developed the method with the help of the Lagrange interpolation polynomial of two-step (Kumar, Kumar, & Jleli, Citation2020; Toufik & Atangana, Citation2017). We apply this method and find the approximate solution of the eco-epidemiological model. First, we assume the non-integer differential equation as follows:
(52)
(52)
Apply the Atangana-Baleanu integral operator on Equationequation (52)(52)
(52) , then we have:
(53)
(53)
when
=
we have
(54)
(54)
We are estimated the function with the help of the Lagrange polynomial over the closed interval
and we also use the polynomial interpolation concept (Alzahrani, El-Dessoky, & Baleanu, Citation2021; Toufik & Atangana, Citation2017). Thus,
(55)
(55)
Now, we simplify Equationequation (54)(54)
(54) with the help of Equationequation (55)
(55)
(55) , we get
(56)
(56)
We simplifying the Equationequation (56)(56)
(56) , we get
(57)
(57)
We apply the above scheme (Alzahrani et al., Citation2021) on the system Equation(9)(9)
(9) , then we find
(58)
(58)
8. Numerical results and graphical analysis
Here, we present a new numerical technique with Caputo and ABC non-integer operators to simulate the non-linear fractional eco-epidemiological model. Throughout this simulation, we display graphic results for different fractional orders and infection rates. Monitoring and controlling the infection rate is crucial for determining the system’s behavior and operation. I followed the instructions and accomplished the task of the numerical scheme detailed in section 7. Simulations have been conducted using version R2016a (MATLAB 9.0) of MATLAB (The MathWorks Inc, Citation2016) to demonstrate the efficiency of the proposed approach. We used MATLAB-9 to analyse numerical results for different fractional orders and time durations. Through the simulations, we can observe how changes in the parameters and initial conditions impact the model’s predictions. It gave me a better understanding of the model’s dynamics and allowed me to conduct a more comprehensive analysis. The graphical results from the fractional order analysis were more informative and generalized than other relative works. Model parameters used for the simulation are as follows
S(0) =1/3, I(0) =1, E(0) =1 and P(0) =1/3 are our initial values of proposed system.
In epidemiological modeling, infection rates provide a crucial insight into the dynamic of the system. Thus, we have taken the different infection rates and order of the derivative to observe different behaviors of the PP model. Based on classical models and β = 2, the system exhibits stable behavior in and . and depict the three-dimensional and two-dimensional stable phase portrait representation of Caputo eco-epidemiological model Equation(8)
(8)
(8) when the order of the derivative is
and β = 2. Furthermore, in and , if we change the value of the fractional order
of the epidemiological model, it is quickly stable compared to the previous classical model. Also, and depict the three-dimensional and two-dimensional stable phase portrait representation of Atangana-Baleanu (AB) eco-epidemiological model Equation(9)
(9)
(9) when the order of the derivative is
and β = 2. When the orders of fractional derivatives are different,
illustrate the dynamic nature and complexity of the eco-epidemiological Equation(8)
(8)
(8) based on the Caputo Toufic-Atangana (TA) Scheme and we observe that if we decrease the order of the derivative, the model quickly goes to the stable stage. During the simulation, we noticed that the susceptible animal population appeared to be fluctuating at different rates for different values of
and eventually reached a stable equilibrium state. The infected population, on the other hand, showed a rapid increase and then a decrease, followed by a gradual decline for varying fractional orders. The amount of infectious material in the environment also followed a similar pattern, rising quickly and then gradually falling over time, with different paths for different fractional orders. Lastly, the predator density showed a rapid decrease and then a gradual decline, eventually stabilizing towards the end of the simulation period. show the comparative results relating to the eco-epidemiological model of Caputo and Atangana-Baleanu (AB) with
and
We have examined the graphs and observed that when
the Caputo and AB derivatives models yield the same results with identical parameter values and initial conditions. However, for fractional orders, the proposed fractional models display different paths. We have also noticed significant deviations in the efficacy of both the Caputo and AB models when using the same parameter values, which is caused by the memory properties of the kernels involved in describing the fractional operators. depict the phase diagram for eco-epidemiological model Equation(8)
(8)
(8) with
and various
respectively by Caputo Toufik-Atangana (TA) scheme. In the proposed model, it is essential to note that β is a sensitive parameter. Even a slight change in this parameter has a drastic impact on the behaviour of the system. Further, we have analysed the variation of parameter values with the state variable of the proposed model. represent the bifurcation diagram of the considered eco-epidemiological model for analysed the complex behaviour and stability analysis. Based on the findings, it has become apparent that the Caputo and AB approach is the most significant and reliable method for explaining physical processes, compared to the regular fractional and classical-order cases.
Figure 1. Three-dimensional numerical results for Caputo eco-epidemiological model Equation(8)(8)
(8) with β = 2 and
![Figure 1. Three-dimensional numerical results for Caputo eco-epidemiological model Equation(8)(8) C0DtφS=S[r(1−S+Ig)−βE],C0DtφI=βSE−νI−aIPm+I,C0DtφE=ϵI−ϱE,C0DtφP=P(−d+bIm+I),(8) with β = 2 and φ=1.](/cms/asset/7c998357-e3f3-4cac-865f-722542ecd925/tabs_a_2270229_f0001_c.jpg)
Figure 2. Two-dimensional numerical results for Caputo eco-epidemiological model Equation(8)(8)
(8) with β = 2 and
![Figure 2. Two-dimensional numerical results for Caputo eco-epidemiological model Equation(8)(8) C0DtφS=S[r(1−S+Ig)−βE],C0DtφI=βSE−νI−aIPm+I,C0DtφE=ϵI−ϱE,C0DtφP=P(−d+bIm+I),(8) with β = 2 and φ=1.](/cms/asset/46bcfd83-4b16-4412-b167-92d04faf4e89/tabs_a_2270229_f0002_c.jpg)
Figure 3. Three-dimensional numerical results for Atangana-Baleanu (AB) eco-epidemiological model Equation(9)(9)
(9) with β = 2 and
![Figure 3. Three-dimensional numerical results for Atangana-Baleanu (AB) eco-epidemiological model Equation(9)(9) ABC0DtφS=S[r(1−S+Ig)−βE],ABC0DtφI=βSE−νI−aIPm+I,ABC0DtφE=ϵI−ϱE,ABC0DtφP=P(−d+bIm+I),(9) with β = 2 and φ=0.99.](/cms/asset/82d584cd-0ea2-4973-829c-93dc6b175b7e/tabs_a_2270229_f0003_c.jpg)
Figure 4. Two-dimensional numerical results for Atangana-Baleanu (AB) eco-epidemiological model Equation(9)(9)
(9) with β = 2 and
![Figure 4. Two-dimensional numerical results for Atangana-Baleanu (AB) eco-epidemiological model Equation(9)(9) ABC0DtφS=S[r(1−S+Ig)−βE],ABC0DtφI=βSE−νI−aIPm+I,ABC0DtφE=ϵI−ϱE,ABC0DtφP=P(−d+bIm+I),(9) with β = 2 and φ=0.99.](/cms/asset/e3156b5b-149a-4237-aa4e-debaa6053a73/tabs_a_2270229_f0004_c.jpg)
Figure 5. Three-dimensional numerical results for eco-epidemiological model Equation(8)(8)
(8) with
and various fractional orders by Caputo Toufik-Atangana (TA) scheme.
![Figure 5. Three-dimensional numerical results for eco-epidemiological model Equation(8)(8) C0DtφS=S[r(1−S+Ig)−βE],C0DtφI=βSE−νI−aIPm+I,C0DtφE=ϵI−ϱE,C0DtφP=P(−d+bIm+I),(8) with β=2.5 and various fractional orders by Caputo Toufik-Atangana (TA) scheme.](/cms/asset/90f41792-2b43-4b8a-bfbb-8064d51bf018/tabs_a_2270229_f0005_c.jpg)
Figure 6. Two-dimensional numerical results for eco-epidemiological model Equation(8)(8)
(8) with
and various fractional orders by Caputo Toufik-Atangana (TA) scheme.
![Figure 6. Two-dimensional numerical results for eco-epidemiological model Equation(8)(8) C0DtφS=S[r(1−S+Ig)−βE],C0DtφI=βSE−νI−aIPm+I,C0DtφE=ϵI−ϱE,C0DtφP=P(−d+bIm+I),(8) with β=2.5 and various fractional orders by Caputo Toufik-Atangana (TA) scheme.](/cms/asset/73766716-91d6-4290-ad9a-43d74725fe7a/tabs_a_2270229_f0006_c.jpg)
Figure 7. Time series numerical results for eco-epidemiological model Equation(8)(8)
(8) with
and various fractional orders by Caputo Toufik-Atangana (TA) scheme.
![Figure 7. Time series numerical results for eco-epidemiological model Equation(8)(8) C0DtφS=S[r(1−S+Ig)−βE],C0DtφI=βSE−νI−aIPm+I,C0DtφE=ϵI−ϱE,C0DtφP=P(−d+bIm+I),(8) with β=2.5 and various fractional orders by Caputo Toufik-Atangana (TA) scheme.](/cms/asset/3bdea1c8-8559-4d54-af38-2ecb39b1df8d/tabs_a_2270229_f0007_c.jpg)
Figure 8. Comparison of Three-dimensional numerical results for Caputo and Atangana-Baleanu (AB) eco-epidemiological model with and

Figure 9. Comparison of two-dimensional numerical results for Caputo and Atangana-Baleanu (AB) eco-epidemiological model with and

Figure 10. Comparison of time series numerical results for Caputo and Atangana-Baleanu (AB) eco-epidemiological model with and

Figure 11. Three-dimensional phase diagram for eco-epidemiological model Equation(8)(8)
(8) with
and various
respectively by Caputo Toufik-Atangana (TA) scheme.
![Figure 11. Three-dimensional phase diagram for eco-epidemiological model Equation(8)(8) C0DtφS=S[r(1−S+Ig)−βE],C0DtφI=βSE−νI−aIPm+I,C0DtφE=ϵI−ϱE,C0DtφP=P(−d+bIm+I),(8) with φ=1 and various β=2,2.07,2.5 respectively by Caputo Toufik-Atangana (TA) scheme.](/cms/asset/88753118-1d19-435e-b826-b3f602babc46/tabs_a_2270229_f0011_c.jpg)
Figure 12. Two-dimensional phase diagram for eco-epidemiological model Equation(8)(8)
(8) with
and various
respectively by Caputo Toufik-Atangana (TA) scheme.
![Figure 12. Two-dimensional phase diagram for eco-epidemiological model Equation(8)(8) C0DtφS=S[r(1−S+Ig)−βE],C0DtφI=βSE−νI−aIPm+I,C0DtφE=ϵI−ϱE,C0DtφP=P(−d+bIm+I),(8) with φ=1 and various β=2,2.07,2.5 respectively by Caputo Toufik-Atangana (TA) scheme.](/cms/asset/77e4cccb-9a05-4517-98c8-7d6cf0a46f9f/tabs_a_2270229_f0012_c.jpg)
Figure 13. Time series diagram for eco-epidemiological model Equation(8)(8)
(8) with
and various
respectively by Caputo Toufik-Atangana (TA) scheme.
![Figure 13. Time series diagram for eco-epidemiological model Equation(8)(8) C0DtφS=S[r(1−S+Ig)−βE],C0DtφI=βSE−νI−aIPm+I,C0DtφE=ϵI−ϱE,C0DtφP=P(−d+bIm+I),(8) with φ=1 and various β=2,2.07,2.5 respectively by Caputo Toufik-Atangana (TA) scheme.](/cms/asset/056ed0d6-919e-4cb5-8011-132690a6484d/tabs_a_2270229_f0013_c.jpg)
Figure 14. Bifurcation plots of the proposed eco-epedimological model Equation(7)(7)
(7) : (a) β versus S (b) ε versus S.
![Figure 14. Bifurcation plots of the proposed eco-epedimological model Equation(7)(7) dSdt=S[r(1−S+Ig)−βE],dIdt=βSE−νI−aIPm+I,dEdt=ϵI−ϱE,dPdt=P(−d+bIm+I),(7) : (a) β versus S (b) ε versus S.](/cms/asset/dadbf1e0-aa7d-460c-a1b8-379462f65d54/tabs_a_2270229_f0014_c.jpg)
Figure 15. Bifurcation plots of the proposed eco-epedimological model Equation(7)(7)
(7) : (a) r versus S (b) g versus S.
![Figure 15. Bifurcation plots of the proposed eco-epedimological model Equation(7)(7) dSdt=S[r(1−S+Ig)−βE],dIdt=βSE−νI−aIPm+I,dEdt=ϵI−ϱE,dPdt=P(−d+bIm+I),(7) : (a) r versus S (b) g versus S.](/cms/asset/731c1a48-fb7b-47d0-8912-860284190b68/tabs_a_2270229_f0015_c.jpg)
Figure 16. Bifurcation plots of the proposed eco-epedimological model Equation(7)(7)
(7) : (a) ν versus S (b) a versus S.
![Figure 16. Bifurcation plots of the proposed eco-epedimological model Equation(7)(7) dSdt=S[r(1−S+Ig)−βE],dIdt=βSE−νI−aIPm+I,dEdt=ϵI−ϱE,dPdt=P(−d+bIm+I),(7) : (a) ν versus S (b) a versus S.](/cms/asset/b59e8550-a881-4a93-809c-d4c09e3079ed/tabs_a_2270229_f0016_c.jpg)
9. Conclusion
In this article, we have analyzed the eco-epidemic model that explains the spread of this disease within an animal community and how the environment influences this model. We have converted the eco-epidemiological CWD integer order model presented into a fractional order model. With the aid of fixed-point theory, we have proven the existence and uniqueness of solutions to the proposed model under certain conditions. We have investigated the stability of all equilibrium points of the non-integer eco-epidemiological CWD model with the help of Matignon’s condition. With the support of the Lagrange interpolation polynomial of two steps, we have used two novel numerical methods for locating the approximate solution. We have examined the behaviour of the proposed model by bifurcation plots for various parameter values. Finally, we have compared the Atangana-Baleanu and Caputo solutions of the eco-epidemiological CWD model, the graphical results give better information about the model and its solutions. Our study’s findings play a crucial role in improving the accuracy of the CWD model and developing effective strategies. In future work, we can employ other non-integer order operators and more effective numerical techniques for this model to learn more about the behaviour of the CWD model. These efforts will undoubtedly advance our understanding of this disease and help us combat it more effectively.
Disclosure statement
The authors declare that they have no conflicts of interests.
Data availability statement
Data will be made available on reasonable request.
References
- Ajelli, M., Iannelli, M., Manfredi, P., & Ciofi Degli Atti, M. L. (2008). Basic mathematical models for the temporal dynamics of hav in medium-endemicity italian areas. Vaccine, 26(13), 1697–1707. doi:10.1016/j.vaccine.2007.12.058
- Alkahtani, B. S. T., & Atangana, A. (2016). Analysis of non-homogeneous heat model with new trend of derivative with fractional order. Chaos, Solitons & Fractals, 89, 566–571. doi:10.1016/j.chaos.2016.03.027
- Allen, L. J., Brauer, F., Van den Driessche, P., & Wu, J. (2008). Mathematical epidemiology, Berlin Heidelberg: Springer-Verlag.
- Alzahrani, E., El-Dessoky, M. M., & Baleanu, D. (2021). Mathematical modeling and analysis of the novel coronavirus using Atangana–Baleanu derivative. Results in Physics, 25, 104240. doi:10.1016/j.rinp.2021.104240
- Atangana, A., & Baleanu, D. (2016). New fractional derivatives with nonlocal and non-singular kernel: theory and application to heat transfer model. arXiv preprint arXiv:1602.03408.
- Atangana, A., & Koca, I. (2016). Chaos in a simple nonlinear system with Atangana–Baleanu derivatives with fractional order. Chaos, Solitons & Fractals, 89, 447–454. doi:10.1016/j.chaos.2016.02.012
- Barlow, N. D. (1996). The ecology of wildlife disease control: Simple models revisited. Journal of Applied Ecology, 33(2), 303–314. doi:10.2307/2404752
- Breban, R., Drake, J. M., Stallknecht, D. E., & Rohani, P. (2009). The role of environmental transmission in recurrent avian influenza epidemics. PLoS Computational Biology, 5(4), e1000346. doi:10.1371/journal.pcbi.1000346
- Brennan, M. L., Kemp, R., & Christley, R. M. (2008). Direct and indirect contacts between cattle farms in north-west england. Preventive Veterinary Medicine, 84(3-4), 242–260. doi:10.1016/j.prevetmed.2007.12.009
- Caputo, M. (1969). Elasticita e dissipazione. Bologna: Zanichelli.
- Chen, Y., Petras, I., & Xue, D. (2009). 2009 American Control, Conference Hyatt Regency Riverfront, St. Louls, MO, USA June 10-12, 2009, Fractional Order Control - A Tutorial.
- Das, P., & Mukherjee, D. (2012). Qualitative analysis of a cholera bacteriophage model. International Scholarly Research Notices, 2012, 1–13. doi:10.5402/2012/621939
- Evirgen, F., Esmehan, U. Ç. A. R., Sümeyra, U. Ç. A. R., & Özdemir, N. (2023). Modelling influenza a disease dynamics under Caputo-–Fabrizio fractional derivative with distinct contact rates. Mathematical Modelling and Numerical Simulation with Applications, 3(1), 58–73. doi:10.53391/mmnsa.1274004
- Evirgen, F., Ucar, E., Özdemir, N., Altun, E., & Abdeljawad, T. (2023). The impact of nonsingular memory on the mathematical model of hepatitis c virus. Fractals, 31(04), 2340065. doi:10.1142/S0218348X23400650
- Fatima, B., Yavuz, M., Ur Rahman, M., & Al-Duais, F. S. (2023). Modeling the epidemic trend of middle eastern respiratory syndrome coronavirus with optimal control. Mathematical Biosciences and Engineering: MBE, 20(7), 11847–11874. doi:10.3934/mbe.2023527
- Hsieh, Y.-H., & Hsiao, C.-K. (2008). Predator–prey model with disease infection in both populations. Mathematical Medicine and Biology: A Journal of the IMA, 25(3), 247–266. doi:10.1093/imammb/dqn017
- Irudayaraj, A. X. R., Wahab, N. I. A., Umamaheswari, M. G., Mohd Radzi, M. A., Sulaiman, N. B., Veerasamy, V., … Ramachandran, R. (2020). A matignon’s theorem based stability analysis of hybrid power system for automatic load frequency control using atom search optimized fopid controller. IEEE Access. 8, 168751–168772. doi:10.1109/ACCESS.2020.3021212
- Joshi, H., & Yavuz, M. (2023). Transition dynamics between a novel coinfection model of fractional-order for covid-19 and tuberculosis via a treatment mechanism. European Physical Journal plus, 138(5), 468. doi:10.1140/epjp/s13360-023-04095-x
- Joshi, H., Yavuz, M., Townley, S., & Jha, B. K. (2023). Stability analysis of a non-singular fractional-order covid-19 model with nonlinear incidence and treatment rate. Physica Scripta, 98(4), 045216. doi:10.1088/1402-4896/acbe7a
- Khan, M. A., & Atangana, A. (2020). Modeling the dynamics of novel coronavirus (2019-ncov) with fractional derivative. Alexandria Engineering Journal, 59(4), 2379–2389. doi:10.1016/j.aej.2020.02.033
- Kumar, A., Alshahrani, B., Yakout, H. A., Abdel-Aty, A.-H., & Kumar, S. (2021). Dynamical study on three-species population eco-epidemiological model with fractional order derivatives. Results in Physics, 24, 104074. doi:10.1016/j.rinp.2021.104074
- Kumar, S., Kumar, A., & Jleli, M. (2020). A numerical analysis for fractional model of the spread of pests in tea plants. Numerical Methods for Partial Differential Equations, 38, 540–565. doi:10.1002/num.22663
- Kumar, S., Kumar, A., Abdel-Aty, A.-H., & Alharthi, M. R. (2021). A study on four-species fractional population competition dynamical model. Results in Physics, 24, 104089. doi:10.1016/j.rinp.2021.104089
- Kumar, S., Kumar, A., Samet, B., & Dutta, H. (2021). A study on fractional host–parasitoid population dynamical model to describe insect species. Numerical Methods for Partial Differential Equations, 37(2), 1673–1692. doi:10.1002/num.22603
- Kumar, S., Kumar, A., Samet, B., Gómez-Aguilar, J. F., & Osman, M. S. (2020). A chaos study of tumor and effector cells in fractional tumor-immune model for cancer treatment. Chaos, Solitons & Fractals, 141, 110321. doi:10.1016/j.chaos.2020.110321
- Lafferty, K. D., & Holt, R. D. (2003). How should environmental stress affect the population dynamics of disease. Ecology Letters, 6(7), 654–664. doi:10.1046/j.1461-0248.2003.00480.x
- Losada, J., & Nieto, J. J. (2015). Properties of a new fractional derivative without singular kernel. Progress in Fractional Differentiation and Applications, 1(2), 87–92.
- Mainardi, F. (2012). Fractional calculus: some basic problems in continuum and statistical mechanics. arXiv preprint arXiv:1201.0863.
- Maji, C., Mukherjee, D., & Kesh, D. (2018). Deterministic and stochastic analysis of an eco-epidemiological model. Journal of Biological Physics, 44(1), 17–36. doi:10.1007/s10867-017-9472-5
- Miller, M. W., & Wild, M. A. (2004). Epidemiology of chronic wasting disease in captive white-tailed and mule deer. Journal of Wildlife Diseases, 40(2), 320–327. doi:10.7589/0090-3558-40.2.320
- Miller, M. W., Williams, E. S., McCarty, C. W., Spraker, T. R., Kreeger, T. J., Larsen, C. T., & Thorne, E. T. (2000). Epizootiology of chronic wasting disease in free-ranging cervids in colorado and wyoming. Journal of Wildlife Diseases, 36(4), 676–690. doi:10.7589/0090-3558-36.4.676
- Mukherjee, D. (2003). Persistence in a prey-predator system with disease in the prey. Journal of Biological Systems, 11(01), 101–112. doi:10.1142/S0218339003000634
- Mukherjee, D. (2003). Stability analysis of a stochastic model for prey-predator system with disease in the prey. Nonlinear Analysis: Modelling and Control, 8(2), 83–92. doi:10.15388/NA.2003.8.2.15186
- Packer, C., Holt, R. D., Hudson, P. J., Lafferty, K. D., & Dobson, A. P. (2003). Keeping the herds healthy and alert: Implications of predator control for infectious disease. Ecology Letters, 6(9), 797–802. doi:10.1046/j.1461-0248.2003.00500.x
- Rahman, M. u., Arfan, M., & Baleanu, D. (2023). Piecewise fractional analysis of the migration effect in plant-pathogen-herbivore interactions. Bulletin of Biomathematics, 1(1), 1–23. doi:10.59292/bulletinbiomath.2023001
- Santosh Kumar, C. (2014). Stability and performance analysis of fractional order control systems. Wseas Transactions on Systems and Control, 9(45), 438–444.
- Sigurdson, C. J. (2008). A prion disease of cervids: Chronic wasting disease. Veterinary Research, 39(4), 41. doi:10.1051/vetres:2008018
- The MathWorks Inc. (2016). Matlab version: 9.0 (r2016a).
- Thompson Hobbs, N. (2006). A model analysis of effects of wolf predation on prevalence of chronic wasting disease in elk populations of rocky mountain national park. Report submitted to the National Park Service
- Toufik, M., & Atangana, A. (2017). New numerical approximation of fractional derivative with non-local and non-singular kernel: Application to chaotic models. The European Physical Journal plus, 132(10), 1–16. doi:10.1140/epjp/i2017-11717-0
- Uçar, S., Uçar, E., Özdemir, N., & Hammouch, Z. (2019). Mathematical analysis and numerical simulation for a smoking model with atangana–baleanu derivative. Chaos, Solitons & Fractals, 118, 300–306. doi:10.1016/j.chaos.2018.12.003
- Veeresha, P., Akinyemi, L., Oluwasegun, K., Şenol, M., & Oduro, B. (2022). Numerical surfaces of fractional zika virus model with diffusion effect of mosquito-borne and sexually transmitted disease. Mathematical Methods in the Applied Sciences, 45(5), 2994–3013. doi:10.1002/mma.7973
- Venturino, E. (2002). Epidemics in predator–prey models: Disease in the predators. Mathematical Medicine and Biology, 19(3), 185–205. doi:10.1093/imammb/19.3.185
- Williams, E. S., & Young, S. (1980). Chronic wasting disease of captive mule deer: A spongiform encephalopathy. Journal of Wildlife Diseases, 16(1), 89–98. doi:10.7589/0090-3558-16.1.89