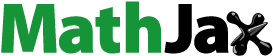
Abstract
Acceptance sampling plans are commonly utilized for planning, process control, and implementation of industrial data sets. In addition to this, they also provide a solution for process quality control. They could be used for executing a decision for acceptance or rejection of a lot. A good acceptance sampling plan can be formulated depending on the requirements of the project. Usually, the plan should follow a defined lifetime distribution. Hence, the appropriate plan would not only determine the plan parameters but would also be cost and time efficient. In this article, an attribute multiple dependent repetitive group sampling (MDRGS) plan is developed assuming that the lifetime of the product follows the Marshall-Olkin logistic-exponential (MOLE) distribution. The parameters of plan are examined by considering a two-point approach. The suggested plan is contrasted with the single sampling and repeated group sampling plans. Finally, the implementation of the proposed plan is discussed using industrial as well as simulated data sets. It is concluded that the proposed plan reduces the sampling inspection cost and time as compared to existing acceptance sampling plans.
1. Introduction
For the sake of its reputation and the satisfaction of its customers, every manufacturing firm strives to keep its goods at a high standard of quality. Acceptance sampling plans are very important for deciding whether to condemn the manufactured product or purchase a lot. It is necessary to ensure the quality of manufactured items but scrutinizing all the lots may not be possible due to shortage of time, the material of the product, inspection errors, and cost. In acceptance sampling, the technique used to choose the sample for examination determines how a submitted batch is sentenced. A typical restriction in life tests is to restrict the amount of time spent on the test since the product’s lifespan is recognized as one of the attribute quality features that reflect the chance of failures happening over time. It is evident that manufacturers would not wait for every item to fail during a test if the life expectancy of the item is large. To solve this problem, the test is stopped at a certain period, the number of failures is noted, and the lot is either approved or rejected depending on the number of defective products. A life test of time-truncated is the name given to this form of experiment. During supervision, there are chances to make a Type-I error (rejecting a good lot) or Type-II error (accepting a bad lot), which are also termed the producer’s risk ( and consumer’s risk (
) respectively. The terms LQL (Limiting Quality Level) and AQL (Acceptable Quality Level) are utilized to describe their parallel quality levels. It is important to remember that the acceptance or rejection of a lot does not always indicate its overall quality, instead it may contain excellent and poor items from the larger lot. The optimal decision is made by a well-designed sample strategy that eliminates both dangers.
Due to the simplicity of implementation, single sampling plan (SSP) is well-known classic testing plan for both attribute and variable sampling scenarios. The SSP is a plan that provides guidelines for rejecting or accepting a batch on the basis of the results of a single haphazard sample. When decision-making relies on the repetition of samples, it is called repetitive group sampling (RGS) plan. The RGS is an improved form of a sequential sampling plan.
Multiple sample plans are also an area of interest. Most of the work has also been done using different lifetime distributions. A new sampling plan under student's-t generalized Birnbaum-Saunders distribution was developed by (Balakrishnan, Leiva, & López, Citation2007). After that the same distribution assuming median lifetime is used by (Lio, Tsai, & Wu, Citation2009; Citation2010).
The Lomax distribution (Rao, Citation2011), following that, a weighted Poisson distribution sampling strategy for several postponed state attributes was proposed by (Subramani & Haridoss, Citation2012). The concept of percentile lifetime was used by (Rao & Naidu, Citation2014). They established an acceptance plan assuming percentile lifetime. Then, using a Pareto distribution of the second sort, (Mughal, Zain, & Aziz, Citation2015) aimed to create a generalized chain sampling strategy. By extending the multiple dependent state sampling approaches for the lifetime of weibull, (Balamurali, Jeyadurga, & Usha, Citation2017) and (Yen, Chang, Aslam, & Jun, Citation2018) proposed a variable multiple dependent repeated acceptance sampling technique that relies on capacity indices. A brand-new MDRGS scheme for gemma and weibull distributions was presented by (Balamurali, Jeyadurga, & Usha, Citation2018) while (Singh, Singh, & Kaur, Citation2019) constructed the RGS plan and on the basis of percentiles for Type-II generalized distribution of log-logistic, a new acceptance plan was developed by (Rao, Rosaiah, & Prasad, Citation2019).
In recent years, acceptance sampling plans have been an area of interest when a product follows some lifetime distribution. The researchers not only extended the theory of acceptance sampling but also tried to propose such plans which are cost and time efficient as compared to the existing ones. As an example, (Singh, Sood, Singh, & Buttar, Citation2020) provided two attribute acceptance sampling methods that guaranteed the good’s quality in terms of the mean lifespan when the lifetime followed the Pareto distribution, while (Rasay, Pourgharibshahi, & Fallahnezhad, Citation2018) provided sequential sampling plan for weibull distribution. According to (Babu, Rao, & Rosaiah, Citation2021), the product’s lifespan should follow the exponentiated frechet distribution with known parameters. A double-acceptance sampling approach was developed on the basis of this presumption. After that (Al-Omari, Almanjahie, & Dar, Citation2021) developed a sampling plan on the basis of two-parameter quasi-shanker distribution that guarantees mean life and utilizes production data to illustrate how to execute the new plan. Based on the mean and median ratios under the birnbaum-saunders distribution, the RGS and multiple delayed state RGS plans for product lifespan were designed by (Kannan, Jeyadurga, & Balamurali, Citation2022).
The multiple dependent state repeated sampling plan for characteristics on the basis of Type-II generalized half-logistic distribution was developed by (Rao, Jilani, & Rao, Citation2021), and it was contrasted with other sampling plans of the same type already in existence. By satisfying both consumer's as well as producer's risks for the designated quality standards on the basis of percentiles led to the determination of a repeating plan for probabilities of exponential log-logistic distribution on the basis of shortened life tests developed by (Kalyani, Rao, Rosaiah, & Sivakumar, Citation2021). Kannan et al. (Kannan et al., Citation2022) expanded the attribute MDSRGS plan and using a time-truncated life test (Rao, Rosaiah, & Naidu, Citation2023), devised the MDSR (Multiple Dependent State Repetitive) sampling schemes for exponentiated half-logistic distributed percentile life assurance. Additionally, (Kannan et al., Citation2022) created the MDSRGS plan to ensure the birnbaum-saunders distribution of the goods’ mean and median lifetimes. The major findings of their study were that their proposed MDSRGS plan was more efficient than the existing ones. After that (Periyasamypandian & Balamurali, Citation2023) focused MDS sampling strategy to make sure products’ average lifetime that combines with weibull distribution. They also claimed it to be useful to inspect electrical appliances. The bayesian repetitive group sampling approach, developed by (Kaviyarasu & Sivakumar, Citation2022), was shown to be the most effective strategy for ensuring the quality of pharmaceutical items and associated materials. An attribute acceptance sampling plan with minimum sample sizes under Marshall-Olkin extended exponential distribution was presented by (Vijayaraghavan & Pavithra, Citation2022). Recently, most of the work has been done in single, double, group and chain sampling plans (Ahmed & Yousof, Citation2022; Liaqat, Saeed, Saleem, Aslam, & Sherwani, Citation2023; Obulezi, Igbokwe, & Anabike, Citation2023; Yigiter, Hamurkaroglu, & Danacıoglu, Citation2023).
Numerous writers in the literature mentioned above have created MDSRGS plans for different distributions under the risk assumptions involving the customer and producer with various ratios. However, neither research passes the time-truncated test using the MOLE distribution. Therefore, when the levels of the quantiles are used as the product’s lifespan under the MOLE distribution, this research encourages the development of a new MDRGS plan. The present analysis assumes that the percentile ratio and distribution’s known shape parameters would be used to attain the excellence level. The percentile lifetime is a valuable parameter in acceptance sampling plans because it helps to define and assess product reliability, sets quality standards, manages risk, ensures consistency in quality control, and aligns with customer expectations. Since MOLE distribution is asymmetric therefore the mean life may not adequately represent the central tendency of the distribution, hence the percentile lifetime is used.
The Marshal-Olkin logistic exponential distribution was introduced by (Mansoor, Tahir, Cordeiro, Provost, & Alzaatreh, Citation2019) as it is a well-known skewed lifetime distribution. The MOLE distribution demonstrates the monotone failure rate feature. The underlying distribution’s failure rate function may have the form of an inverted bathtub-shaped hazard function and unimodal in various applications of engineering, survival analysis, hydrology, and economics. It makes sense to use a probability distribution for a lifetime variable in practice when the failure rate function exhibits a variety of forms. Additionally, MOLE distribution has many failure rates that go down with time. It is important to acknowledge that no distribution can perfectly capture all aspects of a real-world process. The limitations associated with the Marshall-Olkin logistic exponential distribution include its reliance on certain assumptions.
The MOLE is particularly well suited for modeling an item’s lifetime and is often used for the hypothetical component of utilizing life information since it allows for a variety of failure rate function shapes, which is the case comparable to gamma and weibull distributions. Consequently, MOLE may be thought of as a viable probability model to utilize in real-world scenarios and may be used as an alternative to non-normal distributions as it is a member of the lifespan continuous distributions (i.e. gamma, weibull, and other exponentiated family of distributions). According to our understanding, the mentioned distribution is not already covered by the MDRGS plan. Hence considering its importance in reliability studies, it justifies the strength of the work.
In the present study, we aim to design an improved acceptance sampling plan. The newly introduced plan is the generalization of many acceptance plans. As per the most recent studies (Ahmed & Yousof, Citation2022; Liaqat et al., Citation2023; Obulezi et al., Citation2023; Yigiter et al., Citation2023), no sampling plan has been developed to test a repeating group sample strategy for various dependent states where the item quality is distributed according to the MOLE. The main goal of this study is to create the data necessary to guarantee a product’s percentile life with the least amount of money and effort. Also, when compared to the RGSP and SSP, the suggested plan provides the minimum sample size and ASN.
The rest of the work is structured as follows: Section 2 offers a concise overview of the MOLE distribution. Section 3 provides the proposed plan’s design. Section 4 gives discussion of the results. Section 5 explains the comparative study. The application of the strategy is covered in Section 6, and Section 7 concludes the analysis.
2. The Marshall-Olkin logistic exponential distribution
Researchers in several domains have lately utilized the MOLE distribution, a lifetime distribution created by (Mansoor et al., Citation2019), for diverse conditions. The MOLE model may be utilized to examine various forms of lifespan data since the hazard function of the distribution can assume censored as well as uncensored data. The model is flexible and versatile for fitting a broad spectrum of lifetime data, hence it is considered as time truncated model for developing an efficient acceptance sampling plan. The PDF and its hazard functions are presented as:
(1)
(1)
and the hazard function is as
(2)
(2)
The MOLE distribution is an extended version of three models (i.e. Exponential, Logistic and Marshal Olkin distributions) so it is flexible distribution and could be transformed in a variety of distributions by making various transformations (Mansoor et al., Citation2019). The distribution has two shape parameters i.e., and one scale parameter
The cumulative density function (
is given by
(3)
(3)
Driving percentile from
Taking logarithm and rewriting the above equation, we get
(4)
(4)
Using the above expression of percentile function assuming that the shape parameters are known, the scale parameter of the distribution can be obtained as
(5)
(5)
In the above equation, hence
The MOLE is a skewed lifetime distribution, therefore; the mean is not a well-suited measure of central tendency to use in any statistical technique. According to (Lio et al., Citation2009; Citation2010) when the quality of interest has specific low percentile, the acceptance sampling plan using the mean ratio as lifetime may cause a false acceptance decision of a lot when it is a defective one. Hence for this research, the percentile ratio is used as the product lifetime.
3. Designing of the MDSRGS strategy based on time truncated life test
There are four operating parameters of the MDSRGS plan. These parameters are and
here
indicates the size of the sample,
presents the deformed items’ highest legitimate quantity for unrestricted acceptance
denotes the deformed items’ highest auxiliary quantity for limited acceptance
and
signifies many consecutive (preceding) lots required to conclude.
The flow diagram in represents the overall hierarchy of the study which shows that the input data follow MOLE distribution. To proceed with the proposed plan, some design parameters such as consumer’s as well as producer’s risks, termination ratio, and percentile ratio are assumed to be fixed to determine different combinations of plan parameters. The plan parameters for which ASN is lowest, are chosen and respective sample sizes are used for inspection purposes. Finally, a decision is made for possible rejection or acceptance of the entire submitted lot.
3.1. Operating procedure
The MDRGS operating procedure provided by (Aslam, Azam, & Jun, Citation2016), consisting of the following three steps:
Step 1: Take a random sample
item(s) from a submitted lot. Put it on the test for a predetermined time
Accept the hypothesis that
if the number of faulty items
is less than or equal to
Step 2: Reject the lot right away if the number of deformities is more than
in favor of
Step 3: If
the choice between acceptance as well as rejection of the submitted lot depending on specific conditions that accept the lot if
precursory lots were accepted in the condition of
Otherwise, repeat Step-1 until the submitted batch is deemed to have been deducted.
According to (Balamurali et al., Citation2018), it is vital to observe that the suggested MDRGS plan could be decreased to several sampling plans that are given below:
When
the MDRGS plan diminishes to the plan of RGS.
When
the MDRGS plan changes to SSP.
When
the MDRGS plan diminishes to SSP with the acceptance number
3.2. Performance measures
The effectiveness of every plan may be evaluated using its performance metrics, such as the OC (Operating Characteristic) function and ASN (Average Sample Number), which are both regarded as crucial performance tools. Normally, the binomial distribution is considered when the size of a lot is high enough so ASN and OC functions of the suggested plan on the basis of binomial distribution are derived below:
The lot’s acceptance probability following a single sample is described as
(6)
(6)
The rejection probability of the lot on the basis of the single sample could be expressed as
(7)
(7)
When sampling is done repeatedly, the probability would be
(8)
(8)
where
and
are two associated probabilities. Moreover, the first part of
can be stated as:
(9)
(9)
and the next part is as
(10)
(10)
Also, the first part of could be rewritten as:
(11)
(11)
Hence, the OC function as well as the ASN of the suggested MDRGS plan, when the product follows MOLE distribution, are given as
(12)
(12)
and
(13)
(13)
The further details regarding operating procedure provided in Equationequations 6-13 can be seen in (Aslam et al., Citation2016).
3.3. Design methodology
Although several sampling strategies have been devised for lot disposal, an inspection strategy that decreases sample size is often favored. Since for the small sample size, these designs are regarded as inexpensive sampling plans because they lower the inspection cost. Therefore, the primary aim of the MDSRGS strategy is to reduce the size of sample. Hence, the following steps are taken to develop a proposed plan.
The failure probability for MOLE distribution is developed with CDF in terms of specified lifetime, which is Hence, we get the expression below:
(14)
(14)
where
is percentile ratio,
and
indicates the item’s failure probability before the time
expressed as
(15)
(15)
where
and
indicate the product’s specified and unknown percentile lifetimes. The goal is to determine the parameters of the suggested plan by fulfilling the producer’s risk
at different percentile ratios
with AQL
and consumer's risk
at
with LQL
Consequently, the two inequalities mentioned below as
(16)
(16)
(17)
(17)
The ASNs at two associated probabilities are expressed as
(18)
(18)
and
(19)
(19)
Hence the plan parameters can be computed by fulfilling the following constraints
(20)
(20)
(21)
(21)
(22)
(22)
(23)
(23)
(24)
(24)
3.4. Algorithm
Step 1: Provide the values for the shape parameters
producer's, and consumer's risks
percentile lifetime
and termination ratio
as stated, under the MOLE distribution. Suppose the highest value for ASN and name it as
Step 2: Set plan parameters values as
Step 3: Applying the values of known shape parameters, plan parameters as well as percentile ratio at
The AQL values are found and similarly probability of failure (LQL) at
is obtained.
Step 4 Attain the acceptance probabilities
as well as
of the lot at LQL and AQL using Eqs. Equation(16)
(16)
(16) and Equation(17)
(17)
(17) . Compute the ASNs i.e.
and
using
Equation(18)
(18)
(18) and Equation(19)
(19)
(19) .
Step 5: check the inequalities (i.e.
&
to be fulfilled to obtain proposed plan’s optimal parameters.
Step 6: Analogize the value of
If
is less than
then set
and continue the process with various combinations of plan parameters until the smallest
is observed.
Step 7: Whenever
exists, pick the required combination of optimal parameters as (
). If it does not exist, steps 4 to 6 are repeated till a set of ideal parameters is attained.
3.5. Computation methodology
The suggested plan’s optimal parameters are derived with the above method and a code is developed in R-language for examining the values of optimal parameters in MOLE distribution. To find the parameters, the following ranges are used as
. Moreover, the design parameters are obtained at the pre-specified degree of consumer’s risk as
and the producer’s risk as
Termination ratios at two levels are set as
and
These levels are also used by (Lio et al., Citation2009). The different combinations of MOLE distribution’s shape parameters are used as (
), (
) as well as (
) to obtain optimal parameters. It may be possible that EquationEqs. 21-24 are fulfilled for higher than one combination of plan parameters; hence the choice of combinations of the plan parameters depends upon the constraint at which the ASN is reduced. The optimum parameters are displayed in for different shape parameters of the MOLE distribution, experiment time (
) and percentiles ratio (
).
Table 1. MDSRGS plan with shape parameters and
Table 2. MDSRGS plan with shape parameters and
Table 3. MDSRGS plan with shape parameters and
Table 4. MDSRGS plan with shape parameters
and
Table 5. MDSRGS plan with shape parameters
and
Table 6. MDSRGS plan with shape parameters
and
Table 7. MDSRGS plan with shape parameters
and
Table 8. MDSRGS plan with shape parameters
and
Table 9. MDSRGS plan with shape parameters
and
4. Results and interpretation
4.1. Minimum sample number
It is observed from that under the fixed values of consumer’s risk as the percentile ratio
rises from 2 to 10, the ASN declines. The same trend in ASN is also found for other values of shape parameters. Additionally, the increase in the experiment termination ratio
shows an inverse relation with ASN (). It is important to notice that, in certain situations, even though the ideal parameters are the same, the ASNs vary.
4.2. Probability of acceptance
The acceptance probabilities for various shape parameters and percentile lifetime levels of a product are shown in as q = 0.3, 0.5 and 0.7 under the MOLE distribution. It can be observed from the tables that the likelihood of acceptance at AQL increases for every value of when the producer’s risk decreases, but the chance of acceptance at LQL increases for the majority of situations even though the percentile ratio increases when the consumer’s risk increases. Results indicated that raising the degree of product quality might reduce the risk to the producer.
Table 10. OC value of proposed plan with shape parameters
at
Table 11. OC value of proposed plan with shape parameter
at
Table 12. OC value of proposed plan with shape parameter
at
5. Comparative study
This section compares the suggested MDRGS plan’s ASN effectiveness to that of the current plans. For the comparison purpose, are constructed to describe the values of ASN of the suggested plan in comparison with SS and RGS plans for specific values of shape parameters and
Keeping consistent with the literature i.e., see in (Aslam, Khan, & Khan, Citation2015), three percentile lifetime levels of a product are considered as
The specified values of both risks, percentile ratio and ratio to terminate a test are considered to construct the tables.
Table 13. ASNs of proposed versus SS and RGS plans with and
Table 14. ASNs of proposed versus SS and RGS plans with and
Table 15. ASNs of proposed versus SS and RGS plans with and
Overall, it is revealed that the suggested plan minimizes the ASN as compared to other prevailing plans i.e., RGSP and SSP. Hence proposed plan is more cost and time efficient and also recommendable for skewed processes. Our findings are also supported by literature such as (Mansoor et al., Citation2019) that justifies the importance of MOLE distribution for the positively skewed data.
A similar conclusion is shown in (panels a-f) in which the graphical representation of ASN versus percentile ratios at two levels of termination ratio is made for three plans such as the new proposed MDSRGS, repetitive group sampling, and single sampling plans respectively. From these line graphs, we can see that the MDSRGS plan requires the lowest sample size as its average sample number is the smallest amongst all which also verifies the plan as an efficient one. Furthermore, at termination ratio
the curve flatters which shows that the MDSRGS plan needs the same sample size for all levels of percentile ratio
6. Industrial application of the proposed plan
For the application purpose, three examples are considered. In the First instance, the suggested plan is executed on failure times of the brake pads data set while in the second example, breakdown times of the drug data set are considered. The last example is based on the simulated data set generated from MOLE distribution.
6.1. Example-1: failure times of brake pads data
The adoption of acceptance sampling plans is seen as being very important in industries because these plans offer quality assurance for incoming items in less time and cost. To raise the level of product quality, every industrialist uses an acceptance sampling plan.
This section will explore the implementation of a given sampling plan for the disposal of a quantity of automobile front disc brake pads. In cases when the parameters of lifetime distribution are uncertain, the recommended plan can be implemented. The historical performance of the production process is used to manage this scenario. We use the information from (Lawless, Citation2003) for this investigation. The information shows the lifespans (in 1000 km) of front disc brake pads on 40 randomly chosen automobiles of the same type that were kept under close dealer network monitoring. There are 9 censored observations in this dataset. Let’s say the researcher wishes to determine the actual 70th (q = 0.7) percentile lifetime for the distance (km) it takes for car brake pads to wear out.
The data’s non-normality is investigated in the Shapiro-Wilk test ( The PP plot in shows that the data set perfectly fits the MOLE distribution. The CDF line of MOLE aligns perfectly with the diagonal line. This means that the quantiles of data match the quantiles of the MOLE distribution.
We fitted the MOLE model as well as a few alternative models to the actual data set taken into account by (Mansoor et al., Citation2019). The comparison of the MOLE model’s goodness-of-fit with respect to MOW (Marshall- Olkin Weibull), LE (Logistic-Exponential), and MOE (Marshall-Olkin Exponential) on the basis of popular measures i.e. AIC, BIC, and Anderson-Darling () is presented in .
Table 16. Goodness-of-fit evaluations for the lifespan data of the disk brake pads.
It can be found that the MOLE model has the lowest AIC (Akaike Information Criterion), BIC (Bayesian Information Criterion), and Anderson-Darling statistic values, which shows that the MOLE distribution is the best-fitted distribution on the data set. Hence, the tests significantly provide enough evidence that the data set is non-normal and distribution is well fitted, therefore it can be used for illustration purposes of the proposed plan.
For the illustration of the given plan, assuming the shape parameter as and
that are estimated from failure data of past 10 observations using R-studio software, the termination ratio of the test is presumed as
km with specified percentile is
= 56 km which leads to a termination time
km (i.e.,
). Also, suppose the producer’s risk
customer’s risk
and the percentile ratio
from the selected plan parameters are
and
The plan is carried out as follows:
From the present lot, 23 brake pads are chosen randomly and put on a life test for 28 km. The failure times of every brake pad are noted. Assume that the failure times of 23 brake pads are as follows:
38.8, 81.7, 22.7, 40.0, 50.2, 62.4, 51.6, 54.2, 36.7, 56.7, 61.5, 28, 46.9, 52.1, 33.8, 45.5, 22.6, 50.7, 48.8, 31.7, 59.8, 81.3 and 73.1.
The number of brake pad failures is denoted by . From the brake pad failure data, it can be seen that 20 failures are recorded before 28 km, which is greater than
so the current lot of brake pads will be rejected.
Moreover, the classical SS and RGS plans are compared with the MDSRGS plan on the basis of the considered dataset using the same design parameters. indicates the ASNs of the suggested plan are smaller as compared to SSP and RGSP which gives enough evidence to conclude that the proposed plan is an efficient plan for time-truncated product inspection.
Table 17. ASNs of proposed versus SS and RGS plans with and
6.2. Example 2: breakdown times of drug data
Suppose a pharmacist wants to adopt MDSRGS plan to reject or accept a submitted lot of drugs. He wants to develop the true unknown 50th () percentile lifetime for time (in months) to expire the drug. Furthermore, the suggested plan implementation is explained where the lifetime of the drug follows the MOLE distribution with unknown shape parameters which is controlled with the history of the production process. The breakdown times of drugs for various (mg) levels was also examined by (Tsong, Chen, & Chen, Citation2003). For our depiction purpose, we only consider the drug at 10 (mg) and estimated the shape parameters. The non-normality of the expiration time of the drug is confirmed through the Shapiro-Wilk test (
and p-value
). Furthermore, shows that the data set better fits the MOLE distribution. The CDF line of MOLE aligns with the diagonal line. This means that the quantiles of data match the quantiles of the MOLE distribution.
The goodness of fit of data is checked by using the Anderson-Darling () which provided enough evidence that data is best fitted on MOLE distribution. Hence, the maximum likelihood estimates of the shape parameters of the previous 10 breakdown data are found to be
and
under the proposed distribution.
Let the specified percentile time to breakdown the drug is months, the termination ratio
months,
is experiment ending time that is 100 months, the producer’s risk is
the consumer’s risk is
and the percentile ratio is
with
shows the MDSRGS’ plan optimal parameters as
and
with
The implementation follows the steps below:
The first random sample of is drawn from the lot and put on the test for 100 months. Accept the product if the experiment shows no failure. The dataset reproduced is as follows:
99, 100, 101, 102, 100 101, 98
From the data, it is noted that two drugs broke down before 100 months and it is higher than Thus, until the present lot is accepted, the sample is carried out again. This type of plan proves to be useful in post-production stability checking of pharmaceutical items (drugs, syringes, etc.) to make decisions about a lot of products by selecting a smaller sample size.
For comparison of the MDSRGS plan with SS and RGS plans at
the consumer’s risk is
percentile ratio
and
the is constructed for the best combination of plan parameters.
Table 18. ASNs of proposed versus SS and RGS plans with and
shows that SSP requires a large sample size (i.e. ) than RGS and MDSRGS plans while proposed MDSRGS plan is relatively more cost efficient amongst them by requiring a small sample i.e.
under respective plan parameters to inspect the breakdown lifetime of drugs. Hence, the proposed plan is preferable.
6.3. Example-3: simulated data
Simulation is an imitation of a process or situation; an experimenter wishes to study. It can be used to illustrate the performance of any process. In this research simulated data is applied to assess the performance of the MDSRGS plan assuring the 50th percentile lifetime of the product in MOLE distribution. For implementation purposes, we create a data set that follows MOLE distribution by following assumed conditions. The shape parameters of the given distribution are supposed to be and
The specified percentile life is
that means 100 s and the experiment time is
00
s. Thus, the test termination ratio will be considered as
The consumer’s, as well as the producer’s risks, are considered as
and
with a percentile ratio
The best MDSRGS plan parameters for the aforementioned values are shown in as
and
The implementation of the suggested plan is organized as follows:
Randomly choose 17 sample products from the current batch, and evaluate their longevity for 100 s. Assume that the sample items from the present lot’s failure times are as follows:
We can see from these statistics that seven sample items failed the test before the experiment was supposed to start. The present lot is therefore rejected. Hence, the sampling procedure is repeated for the lot until a choice is reached.
Comparison of suggested plan using simulated dataset is also considered to check the efficiency of the plan against SS and RGS plans under same design parameters. indicated that the ASN of the MDSRGS plan is lower than SS and RGS plans, which indicates that classical plans will require more samples to inspect a product than the proposed plan.
Table 19. ASNs of proposed versus SS and RGS plans with and
7. Conclusion
7.1. Results
In this research, the attribute multiple repetitive group sampling plans for the Marshal-Olkin logistic exponential distribution is presented. The product’s percentile life (i.e., the 30th, 50th, and 70th) is regarded as one of its quality characteristics. The MDSRGS plan’s parameters and ASN have been chosen in such a way that, for a range of chosen parameter’s values, both risks (i.e., producer's and consumer's risks) are minimized with a minimal ASN. The effectiveness of the suggested plan was also examined in terms of the OC function and plan parameters.
The Major research findings are as follows:
As the termination ratios increase from
to
the average sample number decreases.
When the products’ percentile lifetime is 30th (
); the ASN is very large, at 50th (median lifetime
), the ASN becomes stable while at 70th (
), the ASN value becomes small. So, it can be concluded that when the distribution is skewed, the median and 70th percentile are appropriate measures of central tendency.
It can be seen that the high acceptance probability can be achieved at a small producer’s risk. Moreover, as the percentile ratio rises, the acceptance probability
approaches to unity, which suggests that the producer’s risk could be dropped if the product’s quality level is upraised and consumer risk is increased when the percentile ratio rises.
From the results, it can be seen that there is an inverse relation between percentile ratio and ASN.
7.2. Discussion
The suggested plan implementation is described. In terms of ASN, the effectiveness of the suggested plan under the MOLE distribution is contrasted with that of traditional schemes. From the findings, we could conclude that the suggested plan performs better as compared to usual single and repetitive sampling plans in terms of cost as well as time for product inspection. Therefore, one of the potential applications of the proposed plan is that it could be adopted by product inspectors, pharmacists, and hydrologists to determine the quality of data specifically when the quality of a given product follows MOLE distribution at the lowest cost as it minimizes the size of the sample as compared to other plans. In the literature, the same MDSRGS plan was used for non-normal distributions (Balamurali et al., Citation2017). It can be concluded that MDSRGS worked efficiently in the proposed study as well as in previous research. In the case of a variable sampling plan, the suggested plan may be expanded by utilizing process capacity index or loss function research in the future. Furthermore, neutrosophic statistics is the extension of classical statistics and is applied when the data is coming from a complex process or from an uncertain environment. The current study can be extended using neutrosophic statistics as future research (Aslam, Citation2019; Aslam, Bantan, & Khan, Citation2020).
Although the proposed plan is a valuable tool when used appropriately to manage quality control and mitigate risks, but it’s essential to select the right plan for your specific circumstances and to use them as part of a broader quality management strategy. Also the presence of sampling risks can be one of the important restrictions of the suggested plan.
Nomenclature | ||
= | Acceptance number for first sample | |
= | Combined acceptance number for first and second samples | |
= | Sample size | |
= | Number of successive preceding lots | |
= | Failure probability at a specific percentile ratio | |
= | Failure probability at specific percentile ratio 1 | |
AQL | = | Acceptance Quality Level |
LQL | = | Limiting Quality Level |
LTPD | = | Lot Tolerance Percent Defective |
= | Average Sample Number at a failure probability | |
= | Average Sample Number at a failure probability | |
= | Consumer’s risk | |
= | Producer’s risk | |
= | Percentile ratio of true to specified percentile life | |
= | True and Specified percentile life | |
= | Termination ratio | |
= | Experiment time | |
= | Distribution’s shape parameter | |
= | Distribution’s shape parameter | |
= | Distribution’s scale parameter | |
MOLE | = | Marshall-Olkin logistic-exponential |
MDSR | = | Multiple dependent state repetitive |
RGSP | = | Repetitive group sampling plan |
MDRGS/MDSRGS | = | Multiple dependent state repetitive group sampling |
DASP | = | Double acceptance sampling plan |
SSP | = | Single sampling plan |
GASP | = | Group acceptance sampling plan |
Data sharing information statement
The data that underpins the study’s conclusions are presented in the application portion of the publication.
Acknowledgement
The authors are deeply thankful to the editor in chief and anonymous referees for their invaluable constructive comments and suggestions, which helped clarify several ideas and improved the quality and presentation of this manuscript.
Disclosure statement
No potential conflict of interest was reported by the author(s).
References
- Ahmed, B., & Yousof, H. (2022). A new group acceptance sampling plans based on percentiles for the weibull frechet model. Statistics, Optimization & Information Computing, 11(2), 409–421. doi:10.19139/soic-2310-5070-1320
- Al-Omari, A. I., Almanjahie, I. M., & Dar, J. G. (2021). Acceptance sampling plans under two-parameter Quasi Shanker distribution assuring mean life with an application to manufacturing data. Science Progress, 104(2), 368504211014350. doi:10.1177/00368504211014350
- Aslam, M. (2019). Control chart for variance using repetitive sampling under neutrosophic statistical interval system. IEEE Access. 7, 25253–25262. doi:10.1109/ACCESS.2019.2899020
- Aslam, M., Azam, M., & Jun, C. H. (2016). Multiple dependent state repetitive group sampling plan for burr XII distribution. Quality Engineering. 28(2), 231–237. doi:10.1080/08982112.2015.1068331
- Aslam, M., Bantan, R. A., & Khan, N. (2020). Design of NEWMA np control chart for monitoring neutrosophic nonconforming items. Soft Computing, 24(21), 16617–16626. doi:10.1007/s00500-020-04964-y
- Aslam, M., Khan, N., & Khan, H. (2015). SkSP-V acceptance sampling plan based on process capability index. Chiang Mai Journal of Science, 42(1), 258–267.
- Babu, S., Rao, S. G., & Rosaiah, K. (2021). Double acceptance sampling plan for exponentiated frechet distribution with known shape parameters. Mathematical Problems in Engineering, 2021, 1–9. doi:10.1155/2021/7308454
- Balakrishnan, N., Leiva, V., & López, J. (2007). Acceptance sampling plans from truncated life tests based on the generalized Birnbaum–Saunders distribution. Communications in Statistics - Simulation and Computation, 36(3), 643–656. doi:10.1080/03610910701207819
- Balamurali, S., Jeyadurga, P., & Usha, M. (2017). Optimal designing of a multiple deferred state sampling plan for weibull distributed lifetime assuring mean life. American Journal of Mathematical and Management Sciences, 36(2), 150–161. doi:10.1080/01966324.2017.1298064
- Balamurali, S., Jeyadurga, P., & Usha, M. (2018). Optimal design of repetitive group sampling plans for Weibull and gamma distributions with applications-and comparison to the Birnbaum–Saunders distribution. Journal of Applied Statistics, 45(14), 2499–2520. doi:10.1080/02664763.2018.1426740
- Kalyani, K., Rao, S. G., Rosaiah, K., & Sivakumar, D. C. U. (2021). Repetitive acceptance sampling plan for odds exponential log-logistic distribution based on truncated life test. Journal of Industrial and Production Engineering, 38(5), 395–400. doi:10.1080/21681015.2021.1931492
- Kannan, G., Jeyadurga, P., & Balamurali, S. (2022). Economic design of repetitive group sampling plan based on truncated life test under Birnbaum–Saunders distribution. Communications in Statistics - Simulation and Computation, 51(12), 7334–7350.
- Kannan, G., Jeyadurga, P., & Balamurali, S. (2022). Determination of multiple deferred state repetitive group sampling plan for lifetime assurance under Birnbaum–Saunders distribution. Journal of Statistical Computation and Simulation, 92(1), 145–158. doi:10.1080/00949655.2021.1934838
- Kannan, G., Jeyadurga, P., & Balamurali, S. (2022). Economical design of multiple deferred (dependent) state repetitive group sampling plan based on truncated life test under exponentiated half-logistic distribution. Sequence Analysis, 41(1), 20–34. doi:10.1080/07474946.2022.2043046
- Kaviyarasu, V., & Sivakumar, P. (2022). Optimization of Bayesian repetitive group sampling plan for quality determination in Pharmaceutical products and related materials. International Journal of Industrial Engineering Computations, 13(1), 31–42. doi:10.5267/j.ijiec.2021.9.001
- Lawless, J. F. (2003). Statistical models and methods for lifetime data. New York: Wiley.
- Liaqat, M., Saeed, N., Saleem, K., Aslam, M., & Sherwani, R. A. K. (2023). A modified group chain sampling plan for lifetime following kumaraswamy generalized power weibull distribution with minimum angle approach. Scientia Iranica. doi:10.24200/sci.2023.59768.6413
- Lio, Y., Tsai, T., & Wu, S. (2009). Acceptance sampling plans from truncated life tests based on the Birnbaum–Saunders distribution for percentiles. Communications in Statistics - Simulation and Computation, 39(1), 119–136. doi:10.1080/03610910903350508
- Lio, Y., Tsai, T., & Wu, S. (2010). Acceptance sampling plans from truncated life tests based on the Burr type XII percentiles. Journal of the Chinese Institute of Chemical Engineers, 27(4), 270–280. doi:10.1080/10170661003791029
- Mansoor, M., Tahir, M., Cordeiro, G., Provost, S., & Alzaatreh, A. (2019). The marshall-olkin logistic exponential distribution. Communications in Statistics - Theory and Methods, 48(2), 220–234. doi:10.1080/03610926.2017.1414254
- Mughal, A., Zain, Z., & Aziz, N. (2015). Time truncated generalized chain sampling plan for Pareto distribution of the 2nd kind. Research Journal of Applied Sciences, Engineering and Technology, 11(3), 343–346. DOI1019026/rjaset.11.1726 doi:10.19026/rjaset.11.1726
- Obulezi, O., Igbokwe, C. P., & Anabike, I. C. (2023). Single acceptance sampling plan based on truncated life tests for zubair-exponential distribution. Earthline Journal of Mathematical Sciences, 13(1), 165–181. doi:10.34198/ejms.13123.165181
- Periyasamypandian, J., & Balamurali, S. (2023). Determination of new multiple deferred state sampling plan with economic perspective under Weibull distribution. Journal of Applied Statistics, 50(13), 2796–2816. doi:10.1080/02664763.2022.2091526
- Rao, G. (2011). Double acceptance sampling plans based on truncated life tests for Marshall-Olkin extended Lomax distribution. Journal of Modern Applied Statistical Methods, 10(1), 12.
- Rao, G. S., Jilani, S. D., & Rao, A. V. (2021). Designing of multiple dependent state repetitive sampling plan for type-II generalized half logistic distribution. International Journal of System Assurance Engineering and Management, 12(6), 1397–1409. doi:10.1007/s13198-021-01338-x
- Rao, G., & Naidu, C. (2014). Acceptance sampling plans for percentiles based on the exponentiated half logistic distribution. Applications and Applied Mathematics, 9(1), 39–53.
- Rao, G., Rosaiah, K., & Prasad, S. (2019). New acceptance sampling plans based on percentiles for type-II generalized log logistic distribution. American Journal of Applied Mathematics and Statistics, 7(4), 131–137. doi:10.12691/ajams-7-4-2
- Rao, S. G., Rosaiah, K., & Naidu, C. R. (2023). Multiple dependent state repetitive sampling plans for exponentiated half logistic distribution. International Journal of Management Science and Engineering Management, 18(2),118–125 .
- Rasay, H., Pourgharibshahi, M., & Fallahnezhad, M. (2018). Sequential sampling plan in the truncated life test for weibull distribution. Journal of Testing and Evaluation, 46(2), 20170022. doi:10.1520/JTE20170022
- Singh, N., Singh, N., & Kaur, H. (2019). Acceptance sampling plan for truncated life test having generalized Pareto distribution. Journal of Life Cycle Reliability and Safety Engineering, 8(2), 151–156.
- Singh, N., Sood, A., Singh, N., & Buttar, G. S. (2020). Acceptance sampling plan for truncated life tests based on generalized pareto distribution using mean life. Industrial Engineering & Management Systems, 19(3), 694–703. doi:10.7232/iems.2020.19.3.694
- Subramani, K., & Haridoss, V. (2012). Development of multiple deferred state sampling plan based on minimum risks using the weighted Poisson distribution for given acceptance quality level and limiting quality level. International Journal of Quality Engineering and Technology, 3(2), 168–180. doi:10.1504/IJQET.2012.049699
- Tsong, Y., Chen, W., & Chen, C. W. (2003). ANCOVA approach for shelf-life analysis of stability study of multiple factor designs. Journal of Biopharmaceutical Statistics, 13(3), 375–393. doi:10.1081/BIP-120022761
- Vijayaraghavan, R., & Pavithra, A. (2022). Construction of Life test sampling inspection plans by attributes based on Marshall Olkin Extended Exponential Distribution. Reliability: Theory & Applications, 17(1 (67), 421–429.
- Yen, C., Chang, C., Aslam, M., & Jun, C. (2018). Multiple dependent state repetitive sampling plans based on one-sided process capability indices. Communications in Statistics - Theory and Methods, 47(6), 1403–1412. doi:10.1080/03610926.2017.1321121
- Yigiter, A., Hamurkaroglu, C., & Danacıoglu, N. (2023). Group acceptance sampling plans based on time truncated life tests for compound Weibull-exponential distribution. International Journal of Quality & Reliability Management, 40(1), 304–315.