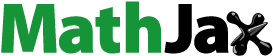
Abstract
The HIV/AIDS model was dynamically analyzed in this study. The model has seven compartments: the uneducated, the educated, the HIV-positive who take antiretroviral therapy (ART), the HIV-positive who do not take ART, people receiving ART treatment, people with AIDS who do not receive any treatment (full-blown AIDS), and the recovered. This model takes into account the analysis of the multiple interactions between the uneducated and the educated subpopulations, the HIV-positive who take and who do not take ART. The free-disease and endemic equilibrium points, as well as the basic reproduction number as a limit condition for infection-free and endemic occurrence, were produced by a mathematical analysis. The center-manifold hypothesis was used to prove that a backward bifurcation exists. The free-disease and endemic equilibrium points coexist when
This means that HIV/AIDS is still spreading. A basic reproduction number below one is insufficient to constitute a free-disease condition. In order to determine essential parameters that significantly contribute to HIV/AIDS transmission, we computed sensitivity index values using a sensitivity analysis. The HIV/AIDS model and bifurcation parameter both identified the rate of HIV transmission from uneducated individuals to HIV-positive individuals who do not receive ART as the most crucial parameter. A numerical simulation supports the dynamical analysis.
1. Introduction
A disease caused by the human immunodeficiency virus (HIV) is called HIV/AIDS (HIV/Acquired Immunodeficiency Syndrome). A person with an early HIV infection may not notice symptoms like a healthy person. As the infection progresses, it further damages the immune system. This infection’s ultimate symptom is acquired immunodeficiency syndrome (AIDS). HIV is mainly spread through unprotected sex, sullied blood transfusions, needles, pregnancy, mother-to-child transmission, childbirth, and breastfeeding.
Researchers proposed mathematical formulas to understand the spread of HIV/AIDS. The first model was constructed by Greenhalgh and Hay (Citation1997) with developed Kaplan’s model (1989). They used a dynamical analysis to simulate the spread of HIV/AIDS in a community of injectable drug users. Cai, Li, Ghosh, and Guo (Citation2009) investigated HIV/AIDS with a treatment in the SIAJ (Susceptible, Infected, AIDS, and Symptomatic) model. Huo, Chen, and Wang (Citation2016) proposed the SIATR (susceptible, infected, AIDS, treatment, and recovered) model and studied its dynamical analysis. As the traditional model, Naik, Zu, and Owolabi (Citation2021) created and examined a model of viral kinetics for HIV-1 primary infection in the presence of immune control and treatment. The literature has examined mathematical models in epidemiology to gain a better understanding of the processes underlying cancers connected to AIDS, giving us insight into viral oncogenesis and cancer immunity (Naik, Owolabi, Yavuz, & Zu, Citation2020).
Antiretroviral medication (ART) should be provided and started for those who have been diagnosed with HIV as soon as feasible following the diagnosis. Patients should also have routine clinical and laboratory evaluations, including tests to check for the presence of the virus in the blood (viral load). Regular use of ART prevents the spread of HIV to others (World Health Organization, Citation2023). By enhancing human abilities to process and comprehend the risks connected with the HIV/AIDS epidemic, education plays a significant role in enhancing population health. Insufficient knowledge makes it difficult for people to consider the potential health risks of their activities. A risk factor for the transmission of sexually transmitted illnesses is education. On the other hand, the incorrect portrayal of HIV/AIDS as a homosexual disease has increased the number of heterosexuals engaging in unsafe sexual conduct without taking transmission risk into account. Therefore, education becomes an essential factor to consider in the model. The HIV/AIDS model was developed by including an educated compartment (Ulfa, Trisilowati, & Kusumawinahyu, Citation2018; Wibowo, Hidayat, & Marsudi, Citation2016).
The number of new HIV infections is projected to decrease from 1.5 million in 2020 to 335,000 by 2030. The number of HIV-related deaths is also expected to decline, from 680,000 in 2020 to less than 240,000 by 2030. These projections are based on the WHO’s 2022-2030 global health sector strategy on HIV (World Health Organization, Citation2023). In accordance with the WHO mission, the Ministry of Health of the Republic of Indonesia planned to eradicate the HIV endemic by 2030 (Ministry of Health Republic of Indonesia, Citation2022). As a manifestation of this commitment, the Ministry of Health is making efforts to tackle HIV/AIDS by taking the 95-95-95 fast track, which refers to meeting the target indicators of 95% estimated people living with HIV (ODHIV) whose HIV status is known, 95% people living with HIV and treated, and 95% people living with HIV who received a treatment and experienced viral suppression. However, according to the 2018-2022 data, the achievement of these targets, especially for women, children, and adolescents, is still not optimal. This is because only 79% of people living with HIV (ODHIV) knew their HIV status, only 41% of people living with HIV were treated, and 16% of people living with HIV who received a treatment experienced viral suppression. In Habibah, Trisilowati, Pradana, and Villadystian (Citation2021), there are 640,633 people living with HIV in June 2018. By 2021, it decreased and was estimated that there would be 526,841 people living with HIV, with an estimated 27 thousand new cases.
According to the above data, we derived a mathematical model of HIV/AIDS with compartments representing people living with HIV (ODHIV) whose HIV status is known,
representing people living with HIV who received a treatment and experienced viral suppression, T representing people living with HIV who are treated, and E representing educated individuals.
In the last couple of years, different stages of infection have become interesting topics in the HIV/AIDS model. Huo and Chen (Citation2015), Omondi, Mbogo, and Luboobi (Citation2018), Ulfa et al. (Citation2018), Mushayabasa and Bhunu (Citation2011), Naik, Owolabi, et al. (Citation2020) and Habibah, Trisilowati, Pradana, et al. (2021) studied mathematical analyses in the development of a model of HIV/AIDS infection with distinct stages. The model includes infections from the mildest stage to the most severe. Now, individuals with full-blown AIDS, which is the most serious stage of the infection, who are not receiving any treatment make up A. Habibah, Trisilowati, Muzaqi, Tania, and AlFaruq (Citation2021a), Habibah, Trisilowati, Muzaqi, Tania, and AlFaruq (Citation2021b), and Habibah, Owati, and Muzaqi (Citation2021) constructed an HIV/AID model by taking into account multiple-interactions between sub-populations. We call it the model. Here, people who alter their sexual behaviors and stick with this alteration for the remainder of their lives are known as R. The dynamics of the model were analyzed in several cases, but the analyses were based on the assumption that not all interactions were considered. The stability analysis of the model can be determined from the basic reproduction number (
). According to the findings, when
asymptotically, the endemic equilibrium point is stable. Conversely, it is unstable. A dynamic analysis involving the relationship between all compartments remains to be conducted.
In this paper, we analyzed the dynamics of the model comprehensively by considering multiple-interactions between all sub-populations, especially the multiple interactions between S, E,
and
sub-populations. The resultant model underwent a dynamical analysis. We demonstrate the positivity and bounds of the system’s solutions (Brauer & Chavez, Citation2016; Murray, Citation2002). Additionally, we determined the model’s solution, the basic reproduction number
and the disease-free and endemic equilibrium points (Abidemi, Owolabi, & Pindza, Citation2022; Heffernan, Smith, & Wahl, Citation2005; Karaagac & Owolabi, Citation2021; Mishra, Purohit, Owolabi, & Sharma, Citation2020). A mathematical analysis of the model was derived to determine the free-disease and endemic equilibrium points, existence, and local stability analysis. In this analysis, the reproduction number (
) is considered insufficient to meet the free-disease condition. It means that the infection can continue to spread.
Recently, the study of the backward bifurcation phenomenon has become an interesting problem. A backward bifurcation occurs when stable free-disease and endemic equilibria coexist. It happens when the basic reproduction number is below one. The center-manifold theory is used to analyze backward bifurcations. Castillo-Chavez and Song (Citation2004) were the first to investigate this method. The center-manifold theory was applied to the tuberculosis model. Gumel (Citation2012) studied the causes of backward bifurcations in some epidemiological models, such as the TB model, the dengue-fever model, and the West Nile virus model. There are several papers presenting backward bifurcation analyses, such as those by Buonomo & Vargas-De-León (Citation2013), Gerberrya and Philipa (Citation2016), Martcheva (Citation2015), Liu and Liu (Citation2018), Bi, Chen, Wu, and Ben-Arieh (Citation2020), and Fatimah, Aldila, and Handari (Citation2021). In this paper, we will show and explain a backward bifurcation in the model. The bifurcation parameter now plays a crucial function as a limit condition of a backward bifurcation. We calculated the sensitivity index values using a sensitivity analysis to obtain parameters that significantly contribute to HIV/AIDS transmission (Chitnis, Cushing, & Hyman, Citation2006; Rois, Trisilowati, & Habibah, Citation2021).
Finally, using the Runge-Kutta method with we numerically solved the model and simulated it with the given parameters to back up the findings of the mathematical analysis.
2. Model analysis
2.1. Model formulation
A mathematical model of HIV/AIDS is proposed, with multiple interactions between uneducated people, educated people, HIV-positive people who take ARV, and HIV-positive people who do not take ARV. The model includes seven sub-populations: susceptible/uneducated people (S), educated people (E), people with HIV who are taking ARV (), people with HIV who are not taking ARV (
), people with AIDS who are not receiving any treatment (A), people who are receiving an ARV treatment (T), and people who have recovered (R). The compartment diagram of the HIV/AIDS
epidemic model, which includes multiple interactions, is shown in .
Figure 1. Diagram of formula (Habibah et al., Citation2021a).

The compartment diagram pertaining to HIV/AIDS is developed and can be found in Habibah et al. (Citation2021a). However, the model constructed did not account for the interaction between the infected people and
and the educated subpopulation (E). Since
was assumed, the model is rather straightforward. The dynamic of each sub-population follows the transmission diagram given in and is described as follows. The number of uneducated or susceptible individuals S increases with the rate of recruitment
and decreases due to interactions with
and
This sub-population decreases due to the natural death rate d and due to its receiving HIV/AIDS education at the rate
Therefore, the dynamic of uneducated or susceptible individuals is given by
The number of educated individuals (E) grows as susceptible individuals receive education at a rate The interactions with
and
as well as the natural death rate d, cause a decrease in the number of educated individuals (E). The dynamic of educated individuals is as follows.
The number of people with HIV who are taking ARV () grows as a result of interactions with uneducated people (S) and educated people (E); S and E now become infected people
A successful treatment contributes to an increase in
at a rate
On the other hand, the number of people with HIV who are taking ARV (
) decreases due to the natural death rate d. Individuals with HIV who are taking ARV (
) will have the worst health, so they require medical treatment at a rate
The dynamic of individuals with HIV who are taking ARV (
) is given by
Following that, the number of people with HIV who are not taking ARV () grows by interactions with uneducated people (S) and educated people (E); S and E now become infected people (
). On the other hand, the number of people with HIV who are not taking ARV (
) decreases due to the natural death rate d. Individuals with HIV who are not taking ARV (
) will have the worst health and develop full-blown AIDS (A) at a rate
so they will require medical treatment at a rate
The dynamic of individuals with HIV who are not taking ARV (
) is given by
The number of people receiving an ARV treatment (T) increases with the progression rates of people with HIV who are taking ARV () and people with HIV who are not taking ARV (
) who then receive a treatment. A Successful treatment, in turn, contributes to the decrease in T at a rate
with the individuals (T) entering the
compartment. A treatment failure contributes to a decrease in T at a rate
with the individuals entering the A compartment. It means that the individuals receiving treatment develop full-blown AIDS (A). The natural mortality rate d and the disease-related mortality rate for treatment individuals
both contribute to the reduction of treatment individuals. The dynamic of individuals who are receiving ARV treatment (T) is given by
The number of people with AIDS who are not receiving any treatment (full-blown AIDS) (A) rises in tandem with the progression rate of individuals with HIV who are not taking ARV () and treatment failure at a rate
On the other hand, the disease-related death rate
for AIDS (A) patients and the natural death rate d contribute to the decreasing number of AIDS patients (A). The dynamic of individuals who are not receiving any ARV treatment (A) is given by
The final compartment is occupied by recovered individuals (R). The R class consists of susceptible individuals (S) who adopt safe sexual habits and stick to them for the rest of their lives at a rate of This class decreases at the natural death rate d. The dynamic of recovered individuals R is given by
Based on the above description, the mathematical model of the following system of nonlinear differential equations describes HIV/AIDS.
(1)
(1)
with
and
The initial values of the variables are
and
All parameters in the system Equation(1)
(1)
(1) are positive values as given in .
Table 1. Parameter description of the model.
In the previous research (Habibah et al., Citation2021a), the interaction between the educated subpopulation (E) and infected individuals and
did not consider in the mathematical model and its mathematical stability, so that
This model presents multiple interactions between uneducated people (S), educated people (E), HIV-positive people who take ARV (
), and HIV-positive people who do not take ARV (
). Because education plays a significant role in preventing the spread of HIV to others, the interaction between educated individuals (E) and infected individuals
and
is less than the interaction between uneducated or susceptible individuals (S) and infected individuals
and
Furthermore, the infection rate can be expressed mathematically as
On the other hand, people who do not take ARV (
) have the potential to transmit the HIV virus to a greater extent than people who take ARV (
). Therefore, we can also say that the infection rate (
) among susceptible people
is greater than the infection rate among susceptible people
or we can put it as
It will be further discussed in the sensitivity analysis in sub-section 2.4.
2.2. Boundedness and positivity of model solution
The boundedness and positivity of model solutions will be proved in the following theorems.
Theorem 2.1
(Boundedness). All feasible solutions
and
of system Equation(1)
(1)
(1) are bounded by a region
Proof.
Total population of system Equationequation (1)(1)
(1) can be written as
(2)
(2)
It implies that
(3)
(3)
and by using the method to solve an ordinary differential equation, we obtain
(4)
(4)
with the initial value
Then, by taking limit
we get
(5)
(5)
We can write The system(1) is well defined in the region
Furthermore, we prove the system Equation(1)
(1)
(1) in region D has solutions with a non-negative sign. □
Theorem 2.2
(Positivity). If
, and
, then the solution of system Equation(1)
(1)
(1)
and
are positive for all
Proof.
We have from the first equation of Equation(1)(1)
(1)
(6)
(6)
where
Equation (2.2) is multiplied by
to result
(7)
(7)
which implies
(8)
(8)
Additionally, the derivative of with respect to t can be used to express the left side of EquationEquation (8)
(8)
(8) , to yield
(9)
(9)
calculating the integral with respect to q from 0 to t, then
(10)
(10)
EquationEquation (10)(10)
(10) is multiplied by
to get
(11)
(11)
Eventually, we achieve
(12)
(12)
signifies that the system Equation(1)
(1)
(1) solution for
is positive The solution for
T, A, and R can be obtained with the same procedures as follows
(13)
(13)
(14)
(14)
(15)
(15)
(16)
(16)
(17)
(17)
(18)
(18)
where
and
Consequently, we can state that
and
for all
and this completes the proof. □
2.3. Existence of equilibrium points and basic reproduction number
The equilibrium points are obtained by equating EquationEquation (1)(1)
(1) to zero. For simplicity of analysis, we exclude the R variable because this variable does not affect directly the spread of HIV in the model. We get equilibrium points, called free of infection and endemic points. Free of infection equilibrium point
is
(19)
(19)
The sign of each component of the point reveals a disease-free equilibrium point. The fact that the sign is positive indicates that the system’s problem has an existing solution because it displays the population. From the free-disease point obtained, we can say that there is no infection transmission from the infected subpopulation to all the subpopulations in the model. Next, we get the endemic equilibrium point as follows
(20)
(20)
with
Furthermore, can be obtained by solving the following equation:
(21)
(21)
with
Additionally, an endemic equilibrium point must show a positive sign. All points in the endemic point depend on the and
thus we need to find a solution that works for both. Since we established the viability of the solutions in the preceding section, we can affirm that the endemic equilibrium exists.
In dynamical analysis, the basic reproduction number is a unique number determining whether infectious disease occurs or not (Murray, Citation2002). The basic reproduction number
is calculated using the Next Generation Matrix (NGM) (Heffernan et al., Citation2005). From EquationEquation (1)
(1)
(1) , we consider only the infected individuals, so we have
where
and
By taking partial derivative with respect to
and
at point
we get the Jacobian matrix F and V as follows
and
Thus, by taking invers and multiply with
we end up the NGM as follows
(22)
(22)
Thus, by calculating we get eigen values as follows
(23)
(23)
and
(24)
(24)
The basic reproduction number is equal to the maximum modulus of matrix eigenvalues K, i.e.
max
Substituting
and
from free-disease equilibrium point Equation(19)
(19)
(19) into EquationEquations (23)
(23)
(23) and Equation(24)
(24)
(24) , as well as using the information described in subsection 2.1,
and
as a condition for the transmission of HIV, then we get
(25)
(25)
The particular cases of multi-interaction between subpopulations in the HIV/AIDS model and their analysis have been written in Habibah et al. (Citation2021a), Habibah, Trisilowati, Pradana, et al. (2021), and Habibah, Owati, and Muzaqi (Citation2021).
2.4. Sensitivity analysis
A sensitivity analysis was done to examine the effect of each parameter on infection spread depending on the which can be helpful for choosing the best control methods to stop an epidemic from spreading (Rois et al., Citation2021). The following formula can be used to determine the sensitivity index value:
where A is a differentiated function of a parameter p.
It is crucial to identify the virus’s primary route of transmission in order to decrease the number of HIV-related illnesses and fatalities. This sensitivity index can be used to determine how important certain factors are for the spread of the disease. As a result, this method was used to identify the parameters that need to be regulated and those that have a substantial impact on the The basic reproduction number
has the following parameters:
d,
and
Using the technique described by Erfanian and Noori Skandari (Citation2011) and Rois et al. (Citation2021), the parameter sensitivity analysis can be calculated as follows:
(26)
(26)
where
(27)
(27)
and
(28)
(28)
Considering the values of the parameters in , we obtained and the sensitivity index values of all parameters in the reproduction number
can be seen in . A visual representation of the data in can be seen in .
Table 2. Sensitivity index of parameters in the reproduction number
Based on or , four parameters have positive sensitivity index values (
and
), while the others are negative. A positive mark of sensitivity index value means that if the parameter value is increased (decreased), then the
will increase (decrease). This causes the spread of HIV/AIDS to increase (decrease). As with positive values, a negative mark of sensitivity index value (
d,
and
) means that if the parameter value is increased (decreased), then the
will decrease (increase). As a result, the spread of HIV/AIDS decreases (increases). Positive and negative sensitivity index values reflect opposite parameter behavior. Now, we focus on the positive sensitivity index values.
has a higher value of sensitivity index, but it does not contribute to the transmission of infection. Therefore, we avoid this parameter. On the other hand,
is the parameter that has contributed to the increase in infection the most. The discussion of the parameters that affect how this disease spreads were discovered using parameter sensitivity analysis can be seen in Sangsawang, Humphries, Khan, and Pongsumpun (Citation2023).
2.5. Stability analysis
The free-of-infection equilibrium point can be analyzed for its stability by calculating those of the Jacobian matrix’s eigenvalues of the system Equation(1)(1)
(1) . If all the eigenvalues have negative values, then the equilibrium point
is asymptotically stable. To show this, first, the system Equation(1)
(1)
(1) should be linearized to have the Jacobian matrix at
as follows
(29)
(29)
By solving we get the characteristic equation of Equation(29)
(29)
(29) . Furthermore, we obtain eigenvalues as follows
where value of
can be written as
Value of
is negative when
The eigenvalue
and
can be obtained by calculating the following equation
(30)
(30)
we get characteristic equation
(31)
(31)
where
The EquationEquation (31)
(31)
(31) has negative roots (
and
) when satisfies the discriminant of EquationEquation (31)
(31)
(31) is positive,
and
Finally, we obtain all of the eigenvalues that are negative which can conclude that the disease-free equilibrium point
is locally asymptotically stable when
The stability of the endemic equilibrium point is obtained using the Routh-Hurwitz criteria (Martcheva, Citation2015; Tunc, Citation2007, Citation2002; Citation2009). Firstly, we find the eigenvalues of the Jacobian matrix of the system Equation(1)
(1)
(1) at
The following is the Jacobian matrix at
(32)
(32)
with
(33)
(33)
(34)
(34)
(35)
(35)
(36)
(36)
The equation of characteristic of matrix is yielded by calculating
(37)
(37)
hence we obtain the following eigenvalues
and
that meets
(38)
(38)
with
Since the characteristic equation has a complicated formula, it is rather difficult to determine the eigenvalues. Hence, we apply the Routh-Hurwitz criteria (Martcheva, Citation2015; Tunc, Citation2009, Citation2007, Citation2002). The characteristics equation has negative roots if and only if and
In the next subsection, we present a bifurcation analysis of the HIV/AIDS model.
2.6. Backward bifurcation analysis
To analyze the backward bifurcation, we apply the Center-Manifold Theorem which is introduced in Castillo-Chavez and Song (Citation2004). In the Center-Manifold Theorem, we should calculate two important values, P and Q. These values as a key to determining the type of bifurcation when A forward bifurcation occurs when
and
On the other hand, a backward bifurcation occurs when
and
Theorem 2.3
(Backward Bifurcation). If and
(39)
(39)
then
model exhibits a backward bifurcation at
. Oppositely, if
then
model exhibits a forward bifurcation at
Proof.
First, let the system Equation(1)(1)
(1) excluding the last equation, be redefined as follows
(40)
(40)
(41)
(41)
(42)
(42)
(43)
(43)
(44)
(44)
(45)
(45)
Let be the bifurcation parameter. We choose
as a bifurcation parameter since it shows the interaction between uneducated and HIV-positive without consuming ARV individuals that give the most contribution to the spread of HIV. It is consistent with the real phenomena in the spread of HIV/AIDS. The value of
is obtained from the basic reproduction number and evaluated when
such that we have
(46)
(46)
By substituting and
into the Jacobian matrix Equation(29)
(29)
(29) , we have
(47)
(47)
Then, we calculate the characteristic equation of to yield
and
that satisfies
(48)
(48)
which has the same form as the eigenvalues in the free-of-infection stability. From the above calculation, we obtain zero eigenvalues (
) and the other eigenvalues are negative real parts. It means this meets the condition of the Center-Manifold Theorem.
Furthermore, we need to calculate the left and right eigenvectors related to the zero eigenvalues. Then, the right eigenvector is where
On the other hand, the left eigenvector is where
Since
we only need to calculate the second partial derivative of
Hence, we have
(49)
(49)
(50)
(50)
It is difficult to determine a backward bifurcation occurs when and
directly from the EquationEquations (49)
(49)
(49) and Equation(50)
(50)
(50) , so we will show them in the numerical simulation by using MAPLE software.
3. Numerical simulation
In this part, we give some simulations to illustrate the analytical results. Numerical simulations are carried out using the Runge-Kutta 4th order since this method is suitable for solving the system of ordinary differential equations. Furthermore, we show the dynamics of the HIV/AIDS mathematical formula with the parameter values as in . The local stability of the disease-free equilibrium point is presented in . The basic reproduction number is calculated and we obtain shows that with
as initial values, the model solutions lead to the no-infection equilibrium point
We can see that for a long time, there is no HIV infection in the population. This numerical solution is consistent with the analytical results. If
then the free-of-infection equilibrium point
is locally asymptotically stable. As demonstrated by mathematical analysis, the solutions are bounded and positive, as is evident. However, we found that when
the model was unstable disease-free equilibrium point
In , we see the model converges to the endemic equilibrium point. In this case, bi-stability occurs.
In addition, we simulate multiple parameters in order to provide effect of varying
to the basic reproduction number
that complies with the sensitivity analysis outcome. The rate of transmission from S to
or
was discovered to be the most crucial component of both the bifurcation parameter and the HIV/AIDS model. The dynamics of the
and
subpopulations when we employ a different value of parameter
are displayed in . The graph illustrates how raising
results in rising
This indicates that
is involved in the HIV/AIDS epidemic. As can be seen in ,
is greater than
in . Consequently, reducing the rate of transmission from S to
for example, by refraining from HIV/AIDS-related sexual contact ().
shows the existence of bi-stability phenomena. The condition in this work allows for the existence of a stable free-disease and endemic equilibrium points, one of which is stable (blue line) and the other unstable (red-dashed line), despite the fact that In this instance, reverse bifurcation causes a bi-stability event. The initial state of the population may affect the final state of the population when backward bifurcation occurs. A backward bifurcation parameter now plays a crucial function as a limit condition of a backward bifurcation. In this paper,
is a bifurcation parameter. Using the parameter values presented in , we calculate the values
and
where it satisfies the requirement for backward bifurcation as in the analysis. We calculate P and Q by using MAPLE software. As can be observed, system Equation(1)
(1)
(1) tends to two different final states, two stable equilibrium points, namely free-disease (FDE) and endemic (EE) equilibrium points, when
for the same set of parameter values. Related phenomena of a backward bifurcation can be seen in Fatimah et al. (Citation2021).
4. Conclusion
The HIV/AIDS model has seven compartments: the uneducated (S), the educated (E), the HIV-positive who are taking ARV (), the HIV-positive who are not taking ARV (
), the people receiving an ARV treatment (T), the people with AIDS who are not receiving any treatment (full-blown AIDS (A), and the recovered (R). The interactions between S, E,
and
are taken into account in this model. There are both disease-free points and endemic equilibrium points. Whether the equilibrium points are locally stable was investigated using the Routh-Hurwitz criteria. Bi-stability exists when the basic reproduction number is
The basic reproduction number below one is insufficient to achieve a disease-free condition because HIV/AIDS is continuously spreading.
The center manifold theory can be used to identify the model’s backward bifurcation. The bifurcation parameter now plays a crucial function as a limit condition of a backward bifurcation. The basic reproduction number was equalized to one before being used to determine the bifurcation parameter value. The result is the rate of HIV transmission from uneducated people to HIV-positive people who do not take antiretroviral therapy (ART) was found as the most important parameter by the HIV/AIDS model. The bifurcation parameter is
We calculated the sensitivity index values using a sensitivity analysis to obtain parameters that significantly contribute to HIV/AIDS transmission. Again, (the rate of transmission from S to
) was found to be the most important factor in the HIV/AIDS model and bifurcation parameter as well. Therefore, lowering the rate of transmission from S to
for instance by avoiding sexual contact between HIV/AIDS infection subpopulations could be a successful intervention to stop the spread of HIV virus. Backward bifurcation is a key component of many mathematical models and is essential for creating control schemes (Buonomo & Vargas-De-León, Citation2013; Fatimah et al., Citation2021). For further work, we add some interventions to the model to stop the spread of HIV, by using a control optimal approach.
The dynamical analysis and proposed model suggest that community social health professionals can raise public awareness of HIV/AIDS to prevent the spread of HIV/AIDS. This can be accomplished through various outreach and education initiatives, including campaigns, counseling, and informational media releases. Comprehensive education and outreach programs must include information on HIV/AIDS transmission, prevention, and treatment.
Acknowledgments
The authors would like to thank Brawijaya University for giving support through Hibah Doktor 2021, No: 1629/UN10.F09/PN/2021.
Disclosure statement
No potential conflict of interest was reported by the authors.
Additional information
Funding
References
- Abidemi, A., Owolabi, K. M., & Pindza, E. (2022). Modelling the transmission dynamics of Lassa fever with nonlinear incidence rate and vertical transmission. Physica A, 597, 127259. doi:10.1016/j.physa.2022.127259
- Bi, K., Chen, Y., Wu, C., & Ben-Arieh, D. (2020). A Memetic algorithm for solving optimal control problems of Zika virus epidemic with equilibriums and backward. Communications in Nonlinear Science and Numerical Simulation, 84, 105176. doi:10.1016/j.cnsns.2020.105176
- Brauer, F., & Chavez, C. C. (2016). Mathematical models in population biology and epidemiology (2nd ed.). New York: Springer Verlag.
- Buonomo, B., & Vargas-De-León, C. (2013). Stability and bifurcation analysis of a vector-bias model of malaria transmission. Mathematical Biosciences, 242(1), 59–67. doi:10.1016/j.mbs.2012.12.001
- Cai, L., Guo, S., & Wang, S. (2014). Analysis of an extended HIV/AIDS epidemic model with treatment. Applied Mathematics and Computation, 236, 621–627. doi:10.1016/j.amc.2014.02.078
- Cai, L., Li, X., Ghosh, M., & Guo, B. (2009). Stability analysis of an HIV/AIDS epidemic model with treatment. Journal of Computational and Applied Mathematics, 229(1), 313–323. doi:10.1016/j.cam.2008.10.067
- Castillo-Chavez, C., & Song, B. (2004). Dynamical models of tuberculosis and their applications. Mathematical Biosciences and Engineering: MBE, 1(2), 361–404. doi:10.3934/mbe.2004.1.361
- Chitnis, N., Cushing, J. M., & Hyman, J. M. (2006). Bifurcation analysis of a mathematical model for malaria transmission. SIAM Journal on Applied Mathematics, 67(1), 24–45. doi:10.1137/050638941
- Erfanian, H. R., & Noori Skandari, M. H. (2011). Optimal control of an HIV model. Journal of Mathematics and Computer Science, 02(04), 650–658. doi:10.22436/jmcs.02.04.09
- Fatimah, M., Aldila, D., & Handari, B. D. (2021). Backward bifurcation arises from the smoking transmission model considering media campaign. Journal of Physics: Conference Series, 1722(1), 012004. doi:10.1088/1742-6596/1722/1/012004
- Gerberrya, D. J., & Philipa, A. M. (2016). The effect of the incidence function on the existence of backward bifurcation. Letters in Biomathematics, 1(3), 181–199.
- Greenhalgh, D., & Hay, G. (1997). Mathematical modelling of the spread of HIV/AIDS amongst injecting drug users. IMA Journal of Mathematics Applied in Medicine and Biology, 14(1), 11–38.
- Gumel, A. B. (2012). Cute paper that expands on previous results. Journal of Mathematical Analysis and Applications. 395(1), 355–365. doi:10.1016/j.jmaa.2012.04.077
- Habibah, U., Trisilowati, T., & Muzaqi, M. H. (2021d). Stability analysis of HIV/AIDS model with interaction between educated and infected (not consuming ARV) subpopulations. International Journal of Mathematics Trends and Technology, 67(8), 103–111. doi:10.14445/22315373/IJMTT-V67I8P512
- Habibah, U., Trisilowati, D. I., Muzaqi, M. H., Tania, T. R., & AlFaruq, L. U. (2021b). Stability analysis of HIV/AIDS model with educated subpopulation. CAUCHY: Jurnal Matematika Murni Dan Aplikasi, 6(4), 188–199. doi:10.18860/ca.v6i4.10275
- Habibah, U., Trisilowati, Muzaqi, M. H., Tania, T. R., & AlFaruq, L. U. (2021c). Mathematical HIV/AIDS model with multi-interaction between infected and educated subpopulations and its local stability. Advances in Engineering Research, 209, 272–280.
- Habibah, U., Trisilowati, Pradana, Y. L., & Villadystian, W. (2021a). Mathematical model of HIV/AIDS with two different stages of infection subpopulation and its stability analysis. Engineering Letters, 29(1), 1–9.
- Heffernan, J. M., Smith, R. J., & Wahl, L. M. (2005). Perspectives on the basics reproductive Ratio. Journal of the Royal Society, Interface, 2(4), 281–293. doi:10.1098/rsif.2005.0042
- Huo, H. F., & Chen, R. (2015). Stability of an HIV/AIDS treatment model with different stages. Discrete Dynamics in Nature and Society, 2015, 630503.
- Huo, H. F., Chen, R., & Wang, X. Y. (2016). Modelling and stability of HIV/AIDS epidemic model with treatment. Applied Mathematical Modelling, 40(13-14), 6550–6559. doi:10.1016/j.apm.2016.01.054
- Karaagac, B., & Owolabi, K. M. (2021). Numerical analysis of polio model: A mathematical approach to epidemiological model using derivative with Mittag–Leffler Kernel. Mathematical Methods in the Applied Sciences, 2021, 1–18.
- Liu, Y., & Liu, X. (2018). Global properties and bifurcation analysis of an HIV-1 infection mode; with two target cells. Computational and Applied Mathematics, 37(3), 3455–3472. doi:10.1007/s40314-017-0523-0
- Martcheva, M. (2015). An introduction to mathematical epidemiology. Applied Mathematics, 61. Springer New York: Springer Verlag.
- Ministry of Health Republic of Indonesia. (2022). Cegah HIV-AIDS, Kemenkes Perluas Akses Pencegahan Pada Perempuan. Kementerian Kesehatan Republik Indonesia: Anak dan Remaja.
- Mishra, A. M., Purohit, S. D., Owolabi, K. M., & Sharma, Y. D. (2020). A nonlinear epidemiological model considering asymptotic and quarantine classes for SARS CoV-2 virus. Chaos, Solitons, and Fractals, 138, 109953. doi:10.1016/j.chaos.2020.109953
- Murray, J. D. (2002). Mathematical biology: An introduction (3rd ed.). New York: Springer-Verlag.
- Mushayabasa, S., & Bhunu, C. P. (2011). Modeling HIV transmission dynamics among male prisoners in Sub-Saharan Africa. IAENG Inter. J. Appl. Math, 41(1), 62–67.
- Naik, P. A., Owolabi, K. M., Yavuz, M., & Zu, J. (2020). Chaotic dynamics of a fractional order HIV-1 model involving AIDS-related cancer cells. Chaos, Solitons and Fractals, 140, 110272. doi:10.1016/j.chaos.2020.110272
- Naik, P. A., Zu, J., & Owolabi, K. M. (2020). Global dynamics of a fractional order model for the transmission of HIV epidemic with optimal control. Chaos, Solitons, and Fractals, 138, 109826. doi:10.1016/j.chaos.2020.109826
- Naik, P. A., Zu, J., & Owolabi, K. M. (2021). Modeling the mechanics of viral kinetics under immune control during primary infection of HIV-1 with treatment in fractional order. Physica A, 545, 123816. doi:10.1016/j.physa.2019.123816
- Omondi, E. O., Mbogo, R. W., & Luboobi, L. S. (2018). Mathematical analysis of sex-structured population model of HIV infection in Kenya. Letters in Biomathematics, 1(5), 174–194.
- Rois, M. A., Trisilowati, & Habibah, U. (2021). Local sensitivity analysis of COVID-19 epidemic with quarantine and isolation using normalized index. Telematika, 14(1), 13–24. doi:10.35671/telematika.v14i1.1191
- Sangsawang, S., Humphries, U. W., Khan, A., & Pongsumpun, P. (2023). Sensitivity analysis of cassava mosaic disease with saturation incidence rate model. AIMS Mathematics, 8(3), 6233–6254. doi:10.3934/math.2023315
- Tunc, C. (2002). A study of the stability and boundedness of the solutions of nonlinear differential equations of the fifth order. Indian Journal of Pure and Applied Mathematics, 33(4), 519–529.
- Tunc, C. (2007). About uniform boundedness and convergence of solutions of certain non-linear differential equations of fifth-order. Bulletin of the Malaysian Mathematical Sciences Society, 30(1), 1–12.
- Tunc, C. (2009). A new result on the boundedness of solutions to a nonlinear differential equation of fifth-order with delay. Kuwait Journal of Science & Engineering, 36, 15–31.
- Ulfa, B., Trisilowati., & Kusumawinahyu, W. M. (2018). Dynamical analysis of HIV/AIDS epidemic model with treatment. Journal of Experimental Life Science, 8, 1.
- Wibowo, Ratno Bagus Edy, Hidayat, Noor, Marsudi. (2016) A Sensitivity analysis of the impact of educational campaign, screening and therapy on the spread of HIV infection. Nonlinear Analysis and Differential Equations, 4, 327–341. doi:10.12988/nade.2016.63126
- World Health Organization. (2023). HIV-AIDS. https://www.who.int/health-topics/hiv-aids.