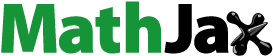
Abstract
In this article, we propose confidence intervals for the population coefficient of variation based on some robust estimators such as trimmed mean, winsorized mean, Hodges-Lehmann estimator and trimean. The proposed confidence intervals and their bootstrap versions were compared with the existing confidence intervals for the population coefficient of variation. The performances of the proposed confidence intervals were evaluated via a Monte-Carlo simulation study by considering the coverage probability, average width, standard deviation of widths, and coefficient of variation of widths as comparison criteria. The proposed confidence intervals performed well in terms of coverage probability on symmetrical distributions. In the case of skewed distributions, they were closer to the nominal confidence level and had narrower widths than the others. As a result, we proposed to use confidence intervals based on the Hodges-Lehmann estimator and winsorized mean for small sample sizes () and larger sample sizes for skewed distributions, respectively. The real-life datasets were analyzed to support the simulation results and confirm the practical applications of the proposed confidence intervals.
1. Introduction
The coefficient of variation (CV) is a statistical tool that is utilized extensively across various study subfields to measure the dispersion for a variable of interest. It is a unit-free measure, therefore, it can be used to compare the homogeneity of multiple variables scaled differently. This is a significant advantage of the CV over the variance or standard deviation, since when the latter are used to report variation, all variables must have the same means and be expressed in the same units. In such cases, it would be correct to use the population CV since it is not dependent on the measuring unit. The population CV is equal to ratio of the population standard deviation (σ) to the population mean The greater the CV value, the greater the dispersion, and the lower the value, the lower the risk. The lowest value indicates the highest level of safety (Banik & Kibria, Citation2011). It is commonly used in many fields, such as engineering, atmospheric, medical sciences and environmental data.
Confidence intervals or hypothesis testing can be used to inference about the CV of a population whose distribution is unknown. While many researchers have studied constructing confidence intervals based on normality assumption for the population CV (Miller, Citation1991; Panichkitkosolkul, Citation2015), others proposed confidence intervals based on the skewed distributions (Curto & Pinto, Citation2009). Thangjai, Niwitpong, and Niwitpong (Citation2020) obtained novel approaches to constructing confidence intervals for the common CV of several normal populations. Amiri and Zwanzig (Citation2010) stated that the shape of the distribution should be known to make inferences about the CV.
Most of the methods in the literature are generally based on parametric models. In addition, bootstrap methods can be considered alternative methods. On the other hand, extremely skewed distributions are common in real-life applications, as well. Niwitpong (Citation2013) presented the confidence intervals for the population CV under log-normal distribution and two theorems were proved for the approximated coverage probability and the expected length of confidence interval. Sangnawakij, Niwitpong, and Niwitpong (Citation2015) proposed confidence intervals for the ratio of the CVs of Gamma distributions. They used the method of variance of estimates recovery with the methods of Score and Wald intervals and compared the coverage probability and interval width via a Monte Carlo simulation. On the other hand, the literature provides confidence intervals for the CV ratio and the difference between two lognormal values (Nam & Kwon, Citation2017; Niwitpong, Niwitpong, & Thangjai, Citation2019). Consulin et al. (Citation2018) evaluated the performance of some CV estimators via Monte Carlo simulation and they highly recommended the use of the CV as a measure of relative dispersion through Ranked set sampling. Abu-Shawiesh, Akyüz, and Kibria (Citation2019) developed three confidence intervals based on variance for the CV of both symmetric and skewed distributions. They observed that the proposed augmented-large-sample (AA&K-ALS) confidence interval performed well in terms of coverage probability in all cases, while large-sample (A&A-LS) and adjusted degrees of freedom (AA&K-ADJ) confidence intervals had much lower coverage probability than the nominal level for skewed distributions. Forkman (Citation2009) conducted statistically inferences for the estimation of CV in normal distribution. This article discussed inference for the coefficient of variation when there was reason to believe that the data is normally distributed, but not lognormally distributed. La-Ongkaew, Niwitpong, and Niwitpong (Citation2022) proposed the confidence intervals for the ratio of coefficients of variation of two Weibull distributions and these intervals have been used on wind speed data, whereas Puggard, Niwitpong, and Niwitpong (Citation2022) compared some interval methods to estimate the population CV of several Birnbaum–Saunders distributions. Chankham, Niwitpong, and Niwitpong (Citation2022) analyzed an air pollution indicator using confidence intervals for the CV of inverse Gaussian distribution.
Recently, sampling plans based on CV have received increasing attention in the literature from many authors due to their importance in measuring product quality. Rao, Aslam, Sherwani, Shehzad, and Jun (Citation2022) obtained a generalized multiple dependent state (GMDS) sampling plan based on population CV and compared with the existing sampling plans. The study’s results indicate that GMDS sampling plan based on population CV provides the desired level of protection with a smaller sample size requirement, making it more cost-effective than existing plans. Albatineh, Kibria, Wilcox, and Zogheib (Citation2014) evaluated the performance of some confidence intervals for the population CV using ranked set sampling and simulated data that are generated fromnormal, skew normal, Gamma, log-normal, and Weibull distributions. The simulation results indicate that the confidence intervals based on ranked set sampling outperformed in terms of coverage probability and interval width. On the other hand, Shahzad et al. (Citation2023) developed some new estimators for the estimation of CV under double stratified random sampling. It was expressed that the traditional estimators of the population CV are based on conventional moments; and also, these are highly affected by the presence of extreme values. The proposed method has shown that estimators can be used in the presence of extreme observations.
Evaluating all the results together, we take into account the CV parameter, which is widely used in describing variation within a data set, is more informative than others among scale parameters, and is preferred over variance or standard deviation in many fields (Banik & Kibria, Citation2011).
When both standard deviation and mean of the population are unknown, the CV may be considered to obtain the interval estimation of the CV. Studies on the confidence interval for CV in such cases are limited. Considering all these, this study aims to obtain new confidence intervals for the population CV by modifying the robust confidence interval proposed by Abu-Shawiesh, Banik, and Kibria (Citation2011). The performance of confidence intervals and their bootstrap versions is compared with some known confidence intervals in terms of coverage probability (CP), average width (AW), standard deviation of width (SDW), and coefficient of variation of width (CVW).
This study is organised as follows: In Section 2, the statistical methodology for deriving the confidence intervals for the population CV is given. That section also includes a review for some existing confidence intervals for a population CV and an explanation for a robust confidence interval for the population standard deviation (). It also includes a derivation of the modified confidence intervals for the population CV and the bootstrap versions of the proposed confidence intervals. In Section 3, simulations are used to evaluate the performance of the proposed confidence intervals in terms of CP, AW, SDW, and CVW. Section 4 illustrates the use of these confidence intervals using three real-life dataset examples. Finally, Section 5 reports our conclusions.
2. Statistical methodology
Consider the independent and identically distributed random sample X1, X2, …, Xn from a distribution with a finite mean μ and variance σ2. We want to determine the (1 − α)100% confidence interval for the population CV under the probability of type I error (α). The population CV can be estimated by the sample CV, defined as
where S and
are sample standard deviation and sample mean, respectively.
2.1. Some selected confidence intervals for the population coefficient of variation
In this section, some confidence intervals for the population CV that are important and useful are reviewed.
2.1.1. Hendricks and Robey confidence interval (denoted by HR)
Hendricks and Robey (Citation1936) proposed a confidence interval as in EquationEq. (2.1)(2.1)
(2.1) .
(2.1)
(2.1)
where
is
percentile of the student t-distribution with degrees of freedom (n-1) and
is the standard error of
This confidence interval is very sensitive to minor violations of the assumption of normality.
2.1.2. Miller confidence interval (denoted by Mill)
Miller’s method has confidence interval limits (CIMill) given as follows (Miller, Citation1991):
(2.2)
(2.2)
where
is the
percentile of the standard normal distribution.
2.1.3. Gulhar, Kibria, Albatineh, and Ahmed confidence interval (denoted by GKAA)
Gulhar, Kibria, and Albatineh (Citation2012) proposed a confidence interval for population CV as follows:
(2.3)
(2.3)
where
and
are, respectively, the
and
percentiles of the Chi-square distribution with degrees of freedom
2.1.4. Bootstrap percentile confidence interval (denoted by percentile bootstrap)
This method is based on the percentile of the distribution of bootstrap replications. Doğan (Citation2017) stated that all bootstrap methods give similar confidence interval limit values for normally distributed data. On the other hand, these methods for skewed distributions produce different confidence intervals. The lower and upper limits of the confidence interval are obtained as follows:
Calculate estimator CV based on a random sample
Obtain a bootstrap sample
} of size n by simple random sampling with replacement.
Calculate
based on the bootstrap sample.
Repeat steps (2) to (3) b = 2000 times.
Sort the estimators
in ascending order.
The lower and upper values of the bootstrap confidence interval for the population CV are the
and
quantiles of the estimator,
and
respectively.
2.1.5. Bootstrap-t confidence interval (denoted by parametric bootstrap)
The CV of bootstrap samples is as:
(2.4)
(2.4)
where
and
Accordingly, the mean of CVs of bootstrap samples and standard error of statistics
are defined as follows:
(2.5)
(2.5)
(2.6)
(2.6)
Thus, the bootstrap-t confidence interval for population CV is defined as follows:
(2.7)
(2.7)
where
j = 1,2,…,b (Nam & Kwon, Citation2017; Santiago & Smith, Citation2013).
2.2. Robust confidence interval for the population standard deviation
The estimator Qn for a random sample X1, X2,…, Xn with distribution function F can be expressed as follows (Abu-Shawiesh et al., Citation2011):
(2.8)
(2.8)
where
and
Qn is the gth order statistic of the
interpoint distance. Let X1, X2,…, Xn and n be a random sample and sample size, respectively. xi and xj are values of random variable Xk, where k = 1,2,…,n, i < j; i = 1,2,…,n; j = 1,2,…n. dn is unbiasing factor. Its values for larger values of sample size are obtained as follows (Abu-Shawiesh et al., Citation2011):
(2.9)
(2.9)
Abu-Shawiesh et al. (Citation2011) proposed a robust confidence interval for the population standard deviation (σ) based on the estimator Qn. This confidence interval is given as in EquationEq. (2.10)(2.10)
(2.10) .
(2.10)
(2.10)
2.3. Modified confidence intervals for the population coefficient of variation
There are many studies in the literature based on modifications of confidence intervals (Abu-Shawiesh et al., Citation2011, Citation2019; Banik, Albatineh, Abu-Shawiesh, & Golam Kibria, Citation2014; Gulhar et al., Citation2012). It is known that the sample mean estimator does not show robust statistical properties in estimating the non-normal population mean, and the coverage probabilities of the confidence intervals have much lower values than the nominal confidence interval. The population mean is very sensitive to outliers and deviation from the normality assumption. In such cases, it would be a good choice to use robust location estimators for estimation of population mean. We propose alternatives for interval estimation of the population CV that is based on robust estimators preferred instead of the sample mean for skewed distributions.
Let’s divide both sides of the confidence interval in EquationEq. (2.10)(2.10)
(2.10) by µ for
We can write as:
(2.11)
(2.11)
It is obtained a confidence interval for the population CV as follows:
(2.12)
(2.12)
where
and
are the
and
percentiles of standard normal distribution, respectively.
Since the population mean is unknown in EquationEq. (2.12)(2.12)
(2.12) , we consider using as an estimate the trimmed mean, winsorized mean, Hodges-Lehmann estimator, and trimean instead of the population mean, respectively.
2.3.1. Confidence interval based on the trimmed mean (denoted by interval I)
Since μ is not known in EquationEq. (2.12)(2.12)
(2.12) , it can be replaced with the robust estimator of μ which is
=
(2.13)
(2.13)
where tm is trimmed mean. The random sample of size n is
and ith order statistics is shown with X(i). The equation of the trimmed mean changes depending on whether the data is symmetrical or skewed. For positively skewed distributions, it is as follows:
(2.14)
(2.14)
For the symmetrical distribution, it is as:
(2.15)
(2.15)
where ln and un are the number of terms to be removed from the low and high end of the consecutive data, respectively.
2.3.2. Confidence interval based on the winsorized mean (denoted by interval II)
We consider winsorized mean (wm) as a robust estimator of the population mean.
(2.16)
(2.16)
Winsorized mean is defined for positively skewed distributions as follows:
(2.17)
(2.17)
and
replacement percentage [.] expression indicates the largest integer function. When replacement is made on both ends of the consecutive data, it is obtained as:
(2.18)
(2.18)
where ln and un are the number of terms to be replaced from the low and high end of the consecutive data, respectively.
2.3.3. Confidence interval based on the Hodges-Lehmann estimator (denoted by interval III)
where HL refers to Hodges-Lehmann and This estimator is obtained from Wilcoxon signed-rank statistic and based on the median of all pairwise differences (Rosenkranz, Citation2010).
2.3.4. Confidence interval based on the trimean (denoted by interval IV)
where is sample trimean (tr). Also, md, Q1, and Q3 are median, first quantile, and third quantile, respectively. Trimean is resistant to extreme values and is preferred to mean and median for extremely skewed distributions (Bonett, Citation2006).
2.4. Bootstrap versions of proposed confidence intervals
In some studies, it has been suggested to use the critical value obtained by the bootstrap method instead of the table value in the confidence intervals (Abu-Shawiesh et al., Citation2011; Banik et al., Citation2014; Gulhar et al., Citation2012). Even if it is known that the sampling distribution of a statistic is approximately normally distributed, this may not apply to real-life problems. Thus, we also propose to examine confidence intervals based on the critical value obtained from bootstrap samples. The lower and upper limits are as follows:
(2.21)
(2.21)
(2.22)
(2.22)
where
and
are the
and
quantiles of statistics
(2.23)
(2.23)
where
is the sample CV of the bootstrap samples,
and
In this study, we denoted the bootstrap confidence intervals as bootstrap intervals I-IV.
3. Performance comparison
In this section, the simulation study was performed to compare the performance of the confidence intervals. We examined the proposed confidence intervals and their bootstrap versions to estimate the population CV. Also, these confidence intervals were compared with bootstrap percentile, parametric bootstrap, and some known confidence intervals in terms of CP and AW. In order to compare the performance of the various intervals, other criteria were the SDW and CVW. The reason for choosing the HR, Mill, and GKAA confidence intervals was to use intervals that studied both the normal and non-normal distribution. Since a theoretical comparison was not possible, a simulation study was conducted to compare the performance of the intervals. All simulations were performed using codes written in MATLAB. We note that trimming and replacement ratios are prefered as in Bonett (Citation2006). The simulation study was designed as follows:
Sample sizes n = 10, 15, 20, 30, 50, 100,
Probability of type I error α = 0.05,
The number of bootstrap and simulation replications are b = 2000 and s = 5000, respectively,
Trimming and replacement ratios
Normal (10,2) with skewness = 0,
Beta (2,2) with skewness = 0,
Beta (2,4) with skewness= 1.32,
Gamma (6.25,2) with skewness= 0.8,
Gamma (4,1) with skewness= 1,
Chi-square (2) with skewness = 2,
Lognormal (0,1) with skewness = 6.18.
The CP, AW, SDW, and CVW were measured for each case. AWs were obtained by dividing the total differences between the lower (l) and upper limits (u) found for each replication by the number of replications. The CPs were determined as the proportion of cases where the population CV was between the lower and upper interval limit. It is always desirable that the CP be as close as possible to the nominal level and the AW be as narrow as possible. SDW and CVW were obtained as the standard deviation of the widths and the ratio of SDW to AW, respectively. Thus, they are obtained by using the following four formulas:
(3.1)
(3.1)
(3.2)
(3.2)
(3.3)
(3.3)
(3.4)
(3.4)
If we need to rephrase, it can be said that s is number of simulation replications, l and u are the lower and upper limits of confidence interval, and Wi, i = 1,2,…,s are interval widths.
The lower and upper limits of proposed confidence intervals in MATLAB are based on the following algorithm:
Step-1: The sample sizes n = 10, 15, 20, 30, 50, and 100 are generated from the known distributions.
Step-2: The trimming/winsorizing percentages based on these samples are obtained (percent=
). If the distribution is symmetrical, trimming/winsorizing is performed from both ends of the data. Otherwise (which is right-skewed distribution), it is obtained only from the top end.
Step-3: The samples are sorted in ascending order and trimmed mean, winsorized mean, HL estimator, and trimean are calculated.
Step-4: The lower and upper limits of confidence intervals in EquationEqs. (2.12)
(2.12)
(2.12) , Equation(2.15)
(2.15)
(2.15) , Equation(2.18)
(2.18)
(2.18) and Equation(2.19)
(2.19)
(2.19) are obtained.
Step-5: Bootstrap samples of size n are generated by simple random sampling with replacement following Step 3.
Step-6: The CV is obtained based on the bootstrap samples.
Step-7: Steps (5) to (6) are repeated 2000 times.
Step-8: The z critical value is obtained from the bootstrap samples as in Eq. (22).
Step-9: The limits of bootstrap confidence intervals are get using bootstrapped critical values in EquationEqs. (2.13)
(2.13)
(2.13) , Equation(2.16)
(2.16)
(2.16) , Equation(2.19)
(2.19)
(2.19) , and Equation(2.20)
(2.20)
(2.20) .
Step 10: The values of CP, AW, SDW, and CVW in EquationEq. (3.1)–(3.4) are calculated based on these limits.
Simulation results based on random samples generated from Normal, Beta and some skewed distributions are presented in .
Table 1. Simulated CP, AW, SDW and CVW values of confidence intervals for N (10,2) and CV = 0.2.
Table 2. Simulated CP, AW, SDW and CVW values of confidence intervals for Beta (2,2) and CV = 0.4472.
Table 3. Simulated CP, AW, SDW, and CVW values of confidence intervals for Beta (2,4) and CV = 0.5345.
Table 4. Simulated CP, AW, SDW and CVW values of confidence intervals for Gamma (4,1) and CV = 0.5.
Table 5. Simulated CP, AW, SDW and CVW values of confidence intervals for Gamma (6.25, 2) and CV = 0.4.
Table 6. Simulated CP, AW, SDW and CVW values of confidence intervals for chi-square (2) and CV = 1.
Table 7. Simulated CP, AW, SDW and CVW values of confidence intervals for Lognormal (0,1) and CV = 1.3108.
Firstly, we compare the performance of the confidence intervals for the some symmetric distributions. The simulation results for random samples generated from both N (10,2) and Beta (2,2) are given in and . The results show that the proposed confidence intervals are quite close to the nominal confidence level (1-α) except for sample sizes n = 10, 15, and The percentile and parametric bootstrap confidence intervals have the narrowest widths for all sample sizes. We noticed that bootstrap intervals I–IV that were obtained with bootstrapped critical value are narrower than intervals I–IV. On the other hand, we note that proposed confidence intervals give higher AWs than the others for symmetrical distributions. The existing confidence intervals are not close to nominal level except GKAA for n = 10, 15 and they aren’t close to nominal level except HR, Mill, and GKAA for n = 20, 30 under the normal distribution. The SDWs of the proposed confidence intervals are larger because of these results. It is also evident from the CV of the widths. Interval IV has the highest AWs in symmetrical distributions ( and ).
In the study, we preferred some skewed distributions in the range skewness coefficient 0.8 − 6.18 to evaluate the performance of the confidence intervals. Because we also want to see the effect of the severity of the skewness on the confidence intervals. We reported them in .
shows the results for moderate skewness. It can say the CPs of all proposed confidence intervals and bootstrap versions are closer to the nominal confidence level than HR, Mill, GKAA, percentile and parametric bootstrap confidence intervals for sample sizes n = 20, 30, 50, and 100. Also, they have narrower widths for similar CPs. Bootstrap interval III performed well in small sample sizes (n = 10,15) in terms of CP and its AWs were narrower than Mill confidence interval for n = 20, 30. It is determined that bootstrap interval II that the confidence interval based on the winsorize mean gives the narrowest widths with sample sizes for moderate skewness. However, it was obtained to be narrowest except n = 10, 15 under distributions where the skewness coefficient value was two or more ().
In , we present the performance of confidence intervals for the Lognormal (0,1) distribution that is highly skewed. It was obtained that all proposed confidence intervals are closer to the nominal confidence level than the others for all sample sizes and they have narrower AWs except n = 10,15. Also, bootstrap interval II has the narrowest AWs according to HR, Mill, GKAA, percentile, and parametric bootstrap confidence intervals for n = 20, 30, 50, and 100. On the other hand, bootstrap interval III performed better for small sample sizes n = 10,15. We obtained that their SDWs were smaller than the others. CVW values also support these results. Interval I which is based on trimmed mean has AWs higher than intervals II-IV in skewed distributions ().
4. Applications using real data
In this section, three real-life data sets will be analyzed to illustrate the application of all considered confidence intervals CIs for the population CV. These data have different sample sizes and different degrees of skewness.
4.1. Dataset I: survival times
The data were obtained from Lawless (Citation2011). They include the survival times in weeks for 20 male rats exposed to high levels of radiation. Descriptive statistics and normality test results are as in .
Table 8. Descriptive statistics and goodness of fit test results for the survival time.
The histogram and Q-Q plot of survival times are shown in . The goodness of fit test for normality has a p-value greater than 0.05 (SW test statistic = 0.961, p-value = 0.571). We can conclude that the data are normally distributed. Thus, the widths based on trimmed and winsorized means are obtained using both ends (trimming/replacement). The calculated 95% confidence intervals are reported in .
Table 9. The 95% confidence intervals for the CV of the survival time.
includes the confidence interval limits for the CV of the survival time which is 0.3155. All confidence intervals cover this value. The percentile and parametric bootstrap confidence intervals differ slightly in terms of width and they give the narrowest interval widths. Interval I has the widest widths. This result supports the simulation outputs for symmetrical distributions ().
4.2. Dataset II: mosquito survival rates
The real-life data used in the second application are on mosquito survival rates in wet climates compiled by Johnson and McFarland (Citation1993). Descriptive statistics and goodness of fit test results for dataset II are given in .
Table 10. Descriptive statistics and goodness of fit test results for the mosquito survival rate.
According to and , we can say that the hypothesis data come from a normal distribution that is rejected at the 0.05 level (SW test statistic= 0.704, p-value < 0.05). All confidence interval limits and widths for dataset II are as in .
Table 11. The 95% confidence intervals for the CV of mosquito survival rate.
In , it was obtained that bootstrap interval III has the narrowest width. This result is consistent with the simulation result obtained with small sample sizes.
4.3. Dataset III: urinary tract infections
Data were obtained from the study by Santiago and Smith (Citation2013). They are on the duration of male patient urinary tract infections in days. The histogram and Q-Q plot were shown in . Also, descriptive statistics and goodness of fit test results for the urinary tract infection are obtained as in .
Table 12. Descriptive statistics and goodness of fit test results for the urinary tract infection.
According to the histogram, Q-Q plot, and goodness of fit test of urinary tract infection, the data have a non-normal distribution (). The limits of 95% confidence intervals and widths for the CV of urinary tract infection are reported in . We can see that all confidence intervals include the CV value. Bootstrap interval II has a narrower width than the others. This result supports the simulation results obtained for large sample sizes ().
Table 13. The 95% confidence intervals for the CV of the urinary tract infection.
5. Some concluding remarks
Finding the mean and variance of the parameter estimates is a fundamental step in establishing the confidence interval (CI) for a parameter of interest. A different method will be used to generate the confidence interval when determining the precise mean and variance is challenging. Although there are many studies for the population CV under the assumption of normal distribution, there are insufficient studies to obtain confidence intervals for CV in skewed distributions and in cases where the population standard deviation is unknown. We proposed modified confidence intervals based on robust estimators for the population CV. Also, we examined the bootstrap versions of these confidence intervals that were obtained from bootstrapped critical values. These intervals were compared to some existing confidence intervals. Simulation results showed that the proposed confidence intervals and bootstrap versions were close to the nominal confidence level but performed poorly in terms of AW, SDW, and CVW on symmetrical distributions. Interval I had the weakest overall performance for the normal distribution. For moderate and high skewness, their CPs are closer to the nominal confidence level and they have narrower widths than the others in large sample sizes In addition, bootstrap interval II has the narrowest average widths. The SDWs and CVWs for this interval are smaller than the others. On the other hand, the proposed confidence intervals did not perform well for small sample sizes (
) except for interval III and bootstrap interval III. Consequently, we propose bootstrap interval III for small sample (
) sizes and bootstrap interval II for larger sample sizes under skewed distributions. Three real data sets were analyzed to illustrate the findings of the study and the simulation results were verified. Furthermore, the proposed confidence intervals are easy to compute and can be recommended for practitioners in various fields of industry, engineering, medical and physical sciences.
Authors’ contributions
All authors contributed equally and significantly in writing of this article. All authors have read and agreed the last version of the paper.
Disclosure statement
The authors declare that they have no known competing financial interests or personal relationships that could have appeared to influence the work reported in this paper.
Data availability statement
The information that assists the conclusions of the present research are included in the paper’s application section.
Additional information
Funding
References
- Abu-Shawiesh, M. O. A., Akyüz, H. E., & Kibria, B. M. G. (2019). Performance of some confidence intervals for estimating the population coefficient of variation under both symmetric and skewed distributions. Statistics, Optimization and Information Computing, 7, 277–290. doi:10.19139/soic.v7i2.630
- Abu-Shawiesh, M. O. A., Banik, S., & Kibria, B. M. G. (2011). A simulation study on some confidence intervals for the population standard deviation. SORT, 35, 83–102.
- Albatineh, A. N., Kibria, B. M. G., Wilcox, M. L., & Zogheib, B. (2014). Confidence interval estimation for the population coefficient of variation using ranked set sampling: A simulation study. Journal of Applied Statistics, 41(4), 733–751. doi:10.1080/02664763.2013.847405
- Amiri, S., & Zwanzig, S. (2010). An improvement of the nonparametric bootstrap test for the comparison of the coefficient of variations. Communications in Statistics - Simulation and Computation, 39(9), 1726–1734. doi:10.1080/03610918.2010.512693
- Banik, S., Albatineh, A. N., Abu-Shawiesh, M. O. A., & Golam Kibria, B. M. (2014). Estimating the population standard deviation with confidence interval: A simulation study under skewed and symmetric conditions. International Journal of Statistics in Medical Research, 3(4), 356–367. doi:10.6000/1929-6029.2014.03.04.4
- Banik, S., & Kibria, B. M. G. (2011). Estimating the population coefficient of variation by confidence intervals. Communications in Statistics - Simulation and Computation, 40(8), 1236–1261. doi:10.1080/03610918.2011.568151
- Bonett, D. G. (2006). Robust confidence interval for a ratio of standard deviations. Applied Psychological Measurement, 30(5), 432–439. doi:10.1177/014662160527955
- Chankham, W., Niwitpong, S. A., & Niwitpong, S. (2022). Measurement of dispersion of PM 2.5 in Thailand using confidence intervals for the coefficient of variation of an inverse Gaussian distribution. PeerJ, 10, e12988. doi:10.7717/peerj.12988
- Consulin, C. M., Ferreira, D., Rodrigues de Lara, I. A., De Lorenzo, A., di Renzo, L., & Taconeli, C. A. (2018). Performance of coefficient of variation estimators in ranked set sampling. Journal of Statistical Computation and Simulation, 88(2), 221–234. doi:10.1080/00949655.2017.1381959
- Curto, J. D., & Pinto, J. C. (2009). The coefficient of variation asymptotic distribution in the case of non-iid random variables. Journal of Applied Statistics, 36(1), 21–32. doi:10.1080/02664760802382491
- Doğan, C. D. (2017). Applying bootstrap resampling to compute confidence intervals for various statistics with R. Eurasian Journal of Educational Research, 17(68), 1–18. doi:10.14689/ejer.2017.68.1
- Forkman, J. (2009). Estimator and tests for common coefficients of variation in normal distributions. Communications in Statistics - Theory and Methods, 38(2), 233–251. doi:10.1080/03610920802187448
- Gulhar, M., Kibria, B. M. G., & Albatineh, A. N. (2012). A comparison of some confidence intervals for estimating the population coefficient of variation: A simulation study. SORT, 36, 45–68.
- Hendricks, W. A., & Robey, K. W. (1936). The sampling distribution of the coefficient of variation. The Annals of Mathematical Statistics, 7(3), 129–132. doi:10.1214/aoms/1177732503
- Johnson, R. E., & McFarland, B. H. (1993). Antipsychotic drug exposure in a health maintenance organization. Medical Care, 31(5), 432–444. doi:10.1097/00005650-199305000-00005
- La-Ongkaew, M., Niwitpong, S. A., & Niwitpong, S. (2022). Estimation of the confidence interval for the ratio of the coefficients of variation of two Weibull distributions and its application to wind speed data. Symmetry, 15(1), 46. doi:10.3390/sym15010046
- Lawless, J. F. (2011). Statistical models and methods for lifetime data. Hoboken, NJ: John Wiley and Sons.
- Miller, E. G. (1991). Asymptotic test statistics for coefficient of variation. Communications in Statistics - Theory and Methods, 20(10), 3351–3363. doi:10.1080/03610929108830707
- Nam, J. M., & Kwon, D. (2017). Inference on the ratio of two coefficients of variation of two lognormal distributions. Communications in Statistics - Theory and Methods, 46(17), 8575–8587. doi:10.1080/03610926.2016.1185118
- Niwitpong, S. (2013). Confidence intervals for coefficient of variation of lognormal distribution with restricted parameter space. Applied Mathematical Sciences, 7, 3805–3810. doi:10.1007/978-3-030-04263-9_27
- Niwitpong, S-a., Niwitpong, S., & Thangjai, W. (2019). Simultaneous confidence intervals for all differences of coefficients of variation of log-normal distributions. Hacettepe Journal of Mathematics and Statistics, 48, 1–17. doi:10.15672/hujms.454804
- Panichkitkosolkul, W. (2015). Confidence interval for the coefficient of variation in a normal distribution with a known population mean after a preliminary t test. KMITL Science and Technology Journal, 15, 34–46.
- Puggard, W., Niwitpong, S. A., & Niwitpong, S. (2022). Confidence intervals for common coefficient of variation of several Birnbaum–Saunders distributions. Symmetry, 14(10), 2101. doi:10.3390/sym14102101
- Rao, G. S., Aslam, M., Sherwani, R. A. K., Shehzad, M. A., & Jun, C. H. (2022). Generalized multiple dependent state sampling plans for coefficient of variation. Communications in Statistics - Theory and Methods, 51(20), 6990–7005. doi:10.1080/03610926.2020.1869989
- Rosenkranz, G. K. (2010). A note on the Hodges–Lehmann estimator. Pharmaceutical Statistics, 9(2), 162–167. doi:10.1002/pst.387
- Sangnawakij, P., Niwitpong, S., & Niwitpong, S. (2015). Confidence intervals for the ratio of coefficients of variation of the gamma distributions. In Integrated uncertainty in knowledge modelling and decision making. Lecture Notes in Computer Science (vol. 9376, pp. 193–203). Cham: Springer. doi:10.1007/978-3-319-25135-6_19
- Santiago, E., & Smith, J. (2013). Control charts based on the exponential distribution: Adapting runs rules for the t chart. Quality Engineering, 25(2), 85–96. doi:10.1080/08982112.2012.740646
- Shahzad, U., Ahmad, I., García-Luengo, A. V., Zaman, T., Al-Noor, N. H., & Kumar, A. (2023). Estimation of coefficient of variation using calibrated estimators in double stratified random sampling. Mathematics, 11(1), 252. doi:10.3390/math11010252
- Thangjai, W., Niwitpong, S. A., & Niwitpong, S. (2020). Adjusted generalized confidence intervals for the common coefficient of variation of several normal populations. Communications in Statistics - Simulation and Computation, 49(1), 194–206. doi:10.1080/03610918.2018.1484138