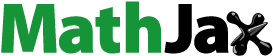
ABSTRACT
Oxidative potential (OP) reflects integratedtoxicity of PM2.5. Therefore, OP of PM2.5 samples collected from traditional central and suburban areas (Gulou and Xianlin) in Nanjing, a megacity of China, from Jan. 2020 to Jun. 2021 was analyzed by using two abiotic assays, DTT and AA assays (OPDTT and OPAA). Values of OPDTTv and OPAAv were 0.82 ~ 3.82 and 0.15 ~ 2.22 nmol/min/m3, respectively. The OP values of PM2.5 samples collected from the Xianlin were higher than those from the Gulou by using both the DTT assay and the AA assay in this study, suggesting the seasonal and spatial variation. Moreover, OP values analyzed by using DTT assay were higher than those by using AA assay. Based on Positive Matrix Factorization (PMF), the major pollution source of the OP of PM2.5 in Nanjing was combustion source. This study may be helpful for evaluating adverse health risks of PM2.5 in a megacity.
1. Introduction
Epidemiological studies show that long-term exposure to the atmospheric fine particulate matters (PM2.5) can result in various adverse health risks such as asthma, airway rhinitis, and congestive heart failure [Citation1–3]. Although the specific mechanisms of those toxic effects of the inhaled PM2.5 is currently unclear, it is widely accepted that the adverse health effects of PM2.5 are related to oxidative stress [Citation4–7]. Inhaled PM2.5 produces excess reactive oxygen species (ROS) in the human body, leading to an imbalance in the body’s antioxidant defenses, which will result in damage to DNA and lipids and inflammation in the body, and then ultimately cause various diseases [Citation8,Citation9]. Now, the ambient air quality standards established by governments around the world and World Health Organization (WHO) focus on the mass concentrations of airborne particles/gaseous pollutants (e.g. PM10, PM2,5, SO2, NOx, O3) and particle-bound contaminants (e.g. inorganic toxic elements such as Pb, F, As, Cd, and Hg and organic toxic compounds such as BaP) [Citation9–11]. These single indicators are hard to reflect the integrated toxicity of PM2.5. For example, oxidative stress posed by inhaled airborne particles may result from the integrated effects of the mass concentrations of airborne particles and particle-bound contaminants [Citation4]. Recently, oxidative potential (OP) has been advocated to assess the oxidation activity of airborne particles to reflect the integrated toxicity of airborne particles [Citation12,Citation13].
Oxidative potential of PM2.5 may be posed by PM2.5-bound persistent free radicals (e.g. semiquinone radical and aromatic radical), PM2.5-bound hazardous trace elements (e.g. As, Pb, Cd, and Hg), PM2.5-bound toxic organic compounds (e.g. PAHs and PCBs), and airborne microorganisms [Citation14–18]. For example, the previous studies show that persistent-free radicals, transition metals (such as copper), quinones, and polycyclic aromatic hydrocarbons have relatively higher oxidative potential than other compounds [Citation19,Citation20]. It is reported that bacterial cells significantly reduced the oxidative potential of particulate matter, while fungal spores increased the oxidative potential of particulate matter [Citation21]. Moreover, factors such as particle size, particle aging time, and particle-derived products may also alter particulate oxidative potential [Citation22]. As an index of the integrated toxicity of oxidation activity, OP of PM2.5 is a better indicator for potential inhalational health risk assessment of PM2.5 than mass concentrations of PM2.5 and/or PM2.5-bound toxic chemical components [Citation3]. Therefore, more investigations on OP of PM2.5 should be carried out in different cities, as extensive investigations of PM2.5-bound hazardous trace elements and organic compounds.
Recently, abiotic and biotic assays have been developed to assess the oxidation activity of atmospheric particulate matters [Citation13]. The most widely used abiotic assays are dithiothreitol assay (DTT) and ascorbic acid (AA) and they were labeled as OPDTT and OPAA [Citation23,Citation24]. It is reported that DTT assay was sensitive to transition metals (e.g. Cu and Mn) and organic compounds (e.g. water-soluble organic carbon and quinone compounds) [Citation25,Citation26], while the AA assay was more sensitive to transition metals (e.g. copper) [Citation27]. Therefore, abiotic DTT and AA assays may reflect different response of PM2.5-bound components to potential health risk. For example, there was a correlation between OPDTT of particulate matter and multiple adverse health outcomes, such as airway inflammation, asthma, and congestive heart failure [Citation1,Citation3]; however, no good correlation was reported between OPAA and asthma, cardiopulmonary emergency departments [Citation28]. So, the choice of assays may impact the performance of the oxidative potential of the particulate matter during the health risk assessment. Therefore, determination of OP using DTT and AA assay synchronously may offer more information on evaluating spatiotemporal variation of OP, which reflects the response of different PM2.5-bound components on the potential health risk assessment of PM2.5.
Yangtze River Delta is one of the economically developed areas in China. Nanjing, one of the metropolitan cities in the Yangtze River Delta, is the capital city of Jiangsu Province locating in 118°22’ and 119°14ʹE and 31°14’ and 32°37ʹN. PM2.5 is an important atmospheric pollutant in Nanjing and may pose potential health risks to local residents [Citation29,Citation30]. Therefore, PM2.5 samples were collected from central and suburban areas (Gulou and Xianlin) in Nanjing, a Chinese megacity, from January 2020 to June 2021. The oxidative potentials of PM2.5 samples were analyzed and compared by using two abiotic assays, DTT and AA assays. The aim of this study is to evaluate the seasonal and spatial variations of OP determined by using two abiotic assays and to discuss the potential resources of OP based on Positive Matrix Factorization (PMF). The novelty of this study is to evaluate redox activity of PM2.5 in a typical metropolitan city in China and it may be helpful for assessing the potential impact of PM2.5 on adverse health risks, providing a scientific basis for the management and control of polluted atmospheric particulate matters in a megacity.
2. Materials and methods
2.1 Sampling of PM2.5
Therefore, PM2.5 samples were collected from central areas (Gulou campus of Nanjing University) and suburban areas (Xianlin campus of Nanjing University) shown in from January 2020 to June 2021. Concentrations of PM2.5, PM10, SO2, NO2, and O3 were recorded from Nanjing Environmental Monitoring Center Station. A total of 198 samples of PM2.5 were collected using a high-flow sampler (HY-1000, Qingdao Henyuan Instruments Co. Ltd., Qingdao, China) in Gulou sampling site and a medium-flow particle sampler (LB-120 F, Qingdao Henyuan Instruments Co. Ltd., Qingdao, China) in Xianlin sampling site, loaded with a quartz filter membrane with a daily air volume of 1000 and 120 m3, respectively. All Whatman quartz microfibre filters (Whatman International Ltd., Maidstone England) wer precombusted in a muffle furnace at 500°C for 2 h. The filter membrane is wrapped in tin foil and stored in a refrigerator. The mass of PM2.5 collected on quartz microfibre filters was obtained via the mass difference of the filter membrane before and after sampling after equilibrated in a desiccator for 48 h.
2.2 Measurement of oxidative potential
A sampling membrane was immersed in deionized water and sonicated for 30 min using an ultrasonic cleaner (SB-5200DTD, YMNL, China). The extraction solution was filtered with a 0.22 μm polyethersulfone membrane (Polyethersulfone Membrane, PES NAVIGATOR, Germany) to remove insoluble substances, and the filtrate was used for the measurement of oxidative potential. In this study, two abiotic assays (DTT and AA assays) were stored to measure the oxidative potential of water-soluble components of PM2.5. One method (reagent) blank was carried throughout the entire sample preparation and analytical process to identify possible contamination resulting from either the equipment used during sample processing or the reagents for each batch of samples processed. Each sample in triplicates was processed and if the relative percent difference of OP values in parallel samples was higher than 10%, the batch treatment would be redone.
DTT assay: DTT assay was performed following the protocol in previous reports [Citation31,Citation32]. Briefly, PM extraction solution, potassium phosphate buffer (0.2 M, pH 7.4), and DTT solution were mixed and incubated at 37°C, and a portion of the mixture was removed every 5 minutes into a centrifuge tube pre-filled with trichloroacetic acid (10%). Then DTNB solution and Tris-HCl (pH 8.8) buffer were added. The absorbance of 5-thio-2-nitrobenzoic acid (TNB) formed by the reaction of the remaining DTT and DTNB was measured at 412 nm using a UV-Vis spectrophotometer (UH5300, China). The OP values were calculated by the consumption rate of DTT, and then normalized by using sampling air volume.
AA assay: AA assay was based on the protocol of Pietrogrande’ report [Citation33]. Briefly, AA solution, PM extraction solution, and potassium phosphate buffer (0.2 M, pH 7.4) were mixed in a 96-well plate and incubated at 37°C. The absorbance of the mixture at 265 nm was measured every 5 minutes using a full-wavelength scanning multi-function reader (Thermo Fisher Scientific, Varioskan Flash, USA). The OP values were calculated by the consumption rate of AA, and then normalized by using sampling air volume.
2.3 Element determination
The quartz filters were immersed into 1 M dilute hydrochloric acid solution in centrifuge tubes. After sonication for 30 minutes, the tubes were transferred to a thermostatic graphite-digestion device and digested at 130°C for 1 h. After cooling to room temperature, those tubes were sonicated for 15 minutes again and the supernatant was separated via centrifugation and stored for further analyses. Soluble elements in the supernatant were determined by using an ICP-OES (Avio 500, PerkinElmer, USA). The measured elements include Al, As, Ca, Cr, Cu, Fe, K, Mg, Mn, Mo, Na, P, Pb, S, Sb, Sr, Ti and Zn. Each sample shall be tested 3 times in parallel. The analytical curve for each batch of sample analyses was set up via the dilution of Multi-Element Standard Solution (1000 mg/L) bought from SPEX CertiPrep, USA. One method (reagent) blank was set up and a continuing calibration verification standard (CCVS) prepared via the dilution of the Multi-Element Standard was determined every 30 of sample solution. If the relative percent difference of parallel samples or CCVS was higher than 10%, the batch treatment would be redone.
2.4 Statistical analysis
Descriptive statistics and correlation analysis of experimental data were processed by using IBM SPSS Statistics 26 software. The potential resources of OP were identified by using a model of EPA PMF5.0. Positive Matrix Factorization (PMF) model, a mathematical receptor model based on factor analysis method, is advocated and developed by United States Environmental Protection Agency (US EPA), which has been widely used in environmental samples including ambient and indoor airborne particles, soil, sediments, and surface water to reveal potential source types and source contributions. PMF model does not need to the source profiles or fingerprints and only files of sample species concentrations and uncertainties of receptor samples and the number of sources need to be provided by PMF users. The fundamentals and technical support of PMF model have been interpreted detailly in the US EPA website (https://www.epa.gov/air-research/positive-matrix-factorization-model-environmental-data-analyses). OP values and inorganic elements of PM2.5 were run on EPA PMF 5.0 for 100 times to discuss the potential sources in Nanjing. The basic principle of the model is that the environmental sample X is assumed to be an n × m matrix, n is the number of samples, and m is the chemical composition, so X can be decomposed into the pollution source contribution matrix G (n × p. P is the number of pollution sources) and the pollution source component spectrum matrix F (p × m), the measured sample concentration can be expressed as [Citation34]:
Where, xij is the sample concentration matrix X, that is, the concentration of the jth species in the ith sample, p is the number of pollution sources, gik is the contribution of the kth pollution source to the ith sample, fkj is the concentration of the jth species in the kth pollution source, and eij is the residual.The model imposes non negative constraints on G and F matrices (gik≥0 and fkj≥0). When the weighted value Q of the square of the ratio of residual error to uncertainty reaches the minimum, it can be considered that the factorization of the model achieves the optimal result. The PMF algorithm determines matrices G and F by continuously minimizing Q:
with
with uij corresponding to the uncertainty of the concentration xij.
3. Results and discussion
3.1 Major ambient air contaminants in Nanjing
Concentration variation of major ambient air contaminants (24-hour average for PM2.5, PM10, SO2, and NO2 and 8-hour average for O3) from 1 January 2020 to 1 July 2021 in Nanjing is shown in . shows that concentrations of PM2.5 and PM10 varied greatly among different date, even in the same season. For example, concentrations of PM2.5 and PM10 ranged from 6 to 133 μg m3 with a medium of 27 μg m3 and from 13 to 168 μg m3 with a medium of 51 μg m3, respectively, in 2020 (from 1 January 2020 to 31 December 2020). shows that the lowest 24-hour average concentrations of PM2.5 and PM10 were observed frequently in summer while PM2.5 and PM10 had higher 24-hour average concentrations in winter, which was due to urban central heating in winter. 24-hour average threshold values of PM2.5 and PM10 are 35/75 and 50/150 μg m3 (Level 1/Level 2 standard), respectively, in ‘Ambient air quality standards of China (GB-3095-2012)’. So, PM2.5 and PM10 are beyond the threshold value of Level 1 standard) frequently, while lower than the threshold value of Level 2 standard in most days of a year (). PM2.5/PM10 values in winter was obvious higher than those in summer (). Great variation on the average concentrations of SO2, NO2 and O3) was observed (). However, regular temporal variation on 24-hour average concentrations of SO2 and NO2 was not observed (); while 8-hour average concentrations of O3 has higher values in summer and lower values in winter (), which was opposite to PM2.5 and PM10. The average threshold values of SO2, NO2, and O3 are 20/50, 80/80, and 100/160 μg m3, respectively, in ‘Ambient air quality standards of China (GB-3095-2012)’. Average concentrations of NO2 fall into its threshold value and those of SO2 are beyond its threshold value in some days (). Average concentrations of O3 are beyond its threshold value frequently in summer. So O3 is the main ambient atmospheric contaminants in summer while PM2.5 and PM10 are the main ambient atmospheric contaminants in winter, spring, autumn in Nanjing. So PM2.5 is an important ambient atmospheric contaminant in Nanjing and the potential health risks posed by airborne particles cannot be ignored, especially PM2.5 which can go into the alveoli.
3.2 Oxidative potential of PM2.5
shows volume-normalized DTT activity (OPDTTv, nmol/min/m3). The seasonal variation of OPDTTv in Xianlin sampling site was in the order of summer> autumn> spring> winter. The average values of OPDTTv were 2.67 nmol/min/m3 (2.00 ~ 3.82 nmol/min/m3) for summer, 2.48 nmol/min/m3 (1.58 ~ 3.67 nmol/min/m3) for autumn, 2.32 nmol/min/m3 (1.55 ~ 3.02 nmol/min/m3) for spring, and 2.31 nmol/min/m3 (1.50 ~ 3.42 nmol/min/m3) for winter. The average values of OPDTTv were 1.56 nmol/min/m3 (1.25 ~ 2.08 nmol/min/m3) for winter, 1.23 nmol/min/m3 (0.82 ~ 1.75 nmol/min/m3) for summer, and 1.32 nmol/min/m3 (0.88 ~ 1.82 nmol/min/m3) for spring. The order of OPDTTv of the samples from Gulou was winter> summer> spring. Therefore, OPDTTv in PM2.5 of Xianlin and Gulou sampling sites indicated the seasonal difference of different sampling seasons and spatial difference between Xianlin and Gulou. Our previous study reported seasonal and spatial variation of mass concentrations of TSP and PM2.5 and TSP/PM2.5 bound toxic chemical components (e.g. As, Pb, Cd, and Hg) in the traditional center district (Gulou) and the suburb (Pukou) in Nanjing [Citation35]. These may be the important reason for seasonal and spatial variation of OPDTTv of PM2.5 in the traditional center district and the suburb (Pukou) in Nanjing.
shows volume-normalized AA activity (OPAAv, nmol/min/m3). The seasonal variation of OPAAv in Xianlin sampling site was in the order of Autumn> Summer> Spring> Winter. The average values of OPAAv were 1.41 nmol/min/m3 (0.75 ~ 1.90 nmol/min/m3) for Autumn; 1.37 nmol/min/m3 (0.15 ~ 1.85 nmol/min/m3) for summer, 1.24 nmol/min/m3 (0.47 ~ 2.22 nmol/min/m3) for spring, and 0.93 nmol/min/m3 (0.44 ~ 1.93 nmol/min/m3) for winter. The average values of OPAAv were 0.70 nmol/min/m3 (0.45 ~ 1.16 nmol/min/m3) for winter, 1.22 nmol/min/m3 (0.47 ~ 1.80 nmol/min/m3) for summer, and 0.78 nmol/min/m3 (0.45 ~ 1.03 nmol/min/m3) for spring. The order of OPAAv of the samples from Gulou was summer> spring> winter. Therefore, these indicated the seasonal and spatial variation of OPAAv in PM2.5. The potential reasons may be consistent with these for OPDTTv discussed above.
To DTT assay, the average OPDTTv value was 2.42 nmol/min/m3 (1.50 ~ 3.82 nmol/min/m3 with a median value of 2.39 nmol /min/m3) for PM2.5 in Xianlin, while the average OPDTTv of was 1.33 nmol/min/m3 (0.82 ~ 2.08 nmol/min/m3 with a median value of 1.34 nmol/min/m3) for PM2.5 in Gulou (). The average OPDTTv value of PM2.5 in Xianlin was 1.8 times higher than that in Gulou. To AA assay, the average OPAAv value was 1.17 nmol/min/m3 (0.15 ~ 2.2 nmol/min/m3 with a median value of 1.17 nmol/min/m3) for PM2.5 in Xianlin, while the average OPAAv of was 0.93 nmol /min/m3 (0.45 ~ 1.80 nmol/min/m3 with a median value of 0.86 nmol/min/m3) for PM2.5 in Gulou. The average OPAAv value of PM2.5 in Xianlin was 1.26 time higher than that in Gulou. These indicated the spatial variation of OPDTTv and OPAAv in of PM2.5 between sampling sites. PM2.5-bound Zn (average: 507 ng m−3) in Xianlin were significantly higher that (126 ng m−3) in Gulou and there were no significant differences on concentrations of As, Pb, Cd, Mn, Cu and Fe between Gulou and Xianlin in Nanjing. PM2.5-bound As, Pb, Cd, Cr, and Zn in the suburb (Pukou) were generally higher these in the traditional center district (Gulou) in Nanjing [Citation35]. Moreover, Xianlin sampling site is located in the downwind of Jinling Petrochemical Group and about 200 meters away from the highway around the city; while Gulou sampling site is situated at the traditional commercial and living areas and low speed traffic exhaust and atmospheric diffusion within the city are the main local pollution source. These may result in the differences on OP between Gulou and Xianlin sampling sites. The average OPDTTv value were higher than the average OPAAv values in PM2.5 of both sampling sites, suggesting the sensitivity of the selected method (). Therefore, OP values analyzed by using DTT assay were higher than those by using AA assay. The previous investigations reported that DTT assay was sensitive to transition metals and organic compounds while the AA assay was more sensitive to transition metals [Citation25–27]. A sampling membrane was extracted with deionized water and the extraction solution was use for the determination of OP using DTT and AA assay. So water-soluble organic carbon and quinone compounds may involve into the formation extract OPDTTv. Moreover, this study confirms the seasonal and spatial variation of OPDTTv and OPAAv of PM2.5 in the traditional center district and the suburb in Nanjing.
The average OP of atmospheric particulate matter in this study was compared with those in other regions in the related literatures (). The average DTT activity in Nanjing was 2.03 nmol/min/m3, higher than those for Beijing (0.19 nmol/min/m3), Delhi (1.57 nmol/min/m3), Xi’an (0.51 nmol/min/m3), Toronto (0.03 nmol/min/m3), Athens (0.33 nmol/min/m3), Ala (0.61 nmol/min/m3), Trento (0.58 nmol/min/m3) and Lecce (0.40 nmol/min/m3), respectively, but lower than that for Sichuan (9.6 nmol/min/m3), Patiala (3.8 nmol/min/m3) (). Therefore, OP values in this study differed from those in the related literatures, revealing the regional characteristics of OP. So, more investigations should be carried out in different regions to accumulate abundant data to reveal how OP works.
Table 1. OP values in the related literatures.
3.3 Correlation between OP and element concentration
listed the correlation coefficients between OP values and element concentrations in PM2.5 collected from Xianlin and Gulou. To Xianlin sampling site, OPDTTv was moderately correlated with S (0.461) and weakly correlated with Cr (0.275), Cu (0.280), Pb (0.205), Sb (0.209), and O3 (0.317). This indicates that secondary sulfate may play an important role in OP, which is consistent with the previous research result that sulfate driven metal dissolution plays a key role in the OP of particulate matter [Citation45]. There is no significant positive correlation between OPAA and the measured elements. OPDTTv was correlated with various elements, including S, Ca, Mn, Fe, Cu, and Zn; while OPAAv was nearly exclusively correlated with Cu [Citation28]. Romano et al. reported that OPDTTv was strongly correlated with metals from anthropogenic sources (e.g. Cr, Ni, Cu, and Zn) and with mineral elements related to natural sources (e.g. K and P) in winter while less element mass concentrations (Pb, P, Ca, Fe, and Zn) were correlated with OPDTTv in spring; no correlation between OPAAv and element mass concentrations was observed [Citation46]. These were consistent with our research.
Table 2. Correlation coefficients between OP values and concentrations of PM2.5-bound elements and major ambient air contaminants.
To Gulou sampling site, OPDTTv had a significant correlation with Al (0.470), Ca (0.489), Cu (0.555), K (0.611), Mg (0.459), Mn (0.566), Pb (0.495), S (0.401), Sb (0.310), Sr (0.454), Ti (0.488), Zn (0.601), SO2 (0.481), and NO2 (0.488). K is a marker of biomass combustion [Citation47–49] and particles emitted from combustion sources may contain abundant persistent free radicals which have high reactivity. So combustion sources may have a great impact on OP in the region. The good correlation between transition metals (Cu, Pb, Mn and Zn) and OPDTT shows that transition metals may have an important contribution to the OP of particulate matter. These correlation results are consistent with previous studies, for example, Yang reported that the correlation coefficients between OPDTT and Cu, Fe, Mn and Zn ranged from 0.61 to 0.68 [Citation50] and Gao et al. reported the similar results [Citation32]. The correlation between OPAA values and element concentrations showed that OP was highly negatively correlated with Na (−0.638) and P (−0.625), and no good correlation between OPAA and the measured elements. In a word, the significant correlation between OPDTTv and the elements measured was observed while weak and/or no good correlation between OPAA and the elements measured was found for PM2.5 from both Xianlin and Gulou sampling sites.
3.4 Source apportionment of OP via PMF
153 observation samples and 17 variable species were inputted into the PMF model as the input basis to infer their possible sources. The uncertainty is jointly determined by the error coefficient and the method detection limit. The error coefficient is set to 0.1, and the method detection limit is determined by three times of the standard deviation of seven blank tests. The solution of 4 factors is determined as the optimal solution, and the number of factors is determined by Q (goodness of fit parameter) and the expected knowledge of sampling points. Q (Robustness) is the goodness of fit parameter for excluding outliers, and Q (True) includes all data points. The consistency of the two parameters shows that the model fits the input data well.
Announcement of source apportionment of PM2.5 in Nanjing announced by Environmental Protection Bureau of Nanjing shows that the main pollution sources of PM2.5 in Nanjing in 2015 were coal-burning emissions (27.4%), automobile exhaust (24.60%), industrial emissions (19.0%), dust (14.1%), and other sources. So 4 factors were set up when using PMF model in this study (Figure 4). As shown in Figure 4, potential sources in Nanjing were identified based on particle-bound elements. Factor 1 (F1) contains more Al, Ca, Fe, Mg, P, Sr, and Ti. Al, Fe, Mg, Sr, and Ti are crustal elements, which are generally related to site dust [Citation51]. Therefore, F1 is defined as soil dust. Factor 2 (F2) was dominated by S, Sb, Zn and Pb. It has been reported that Zn and Sb were associated with lubricating oil, fuel additives, tire and brake wear of traffic sources [Citation52,Citation53]. S is included in the source profiles of coal and oil combustion in PM2.5 is general secondary sulfate and sulfate particles mainly comes from SO2 which is oxidized to sulfate in the atmosphere in clouds. The combustion of oil and coal is the main source SO2, which was oxidized to sulfate in air via through gas-phase homogeneous and heterogeneous oxidation. Fine particles emitted from oil and coal combustion also contain abundant Zn and Pb [Citation54–57]. Hence, F2 was assigned as a combustion source of fossil fuel. Factor 3 (F3) had the higher contribution of As, Cu, Mn, and Zn. According to relevant literature reports, Chinese coal contains high contents of As [Citation58], which is considered as a tracer of the coal combustion source (power plant boilers, industrial boilers and residential stoves) [Citation59], So F3 was classified as coal combustion source. Factor 4 (F4) mainly contained Cr, Cu, and P. Cr and Cu many come from a wide range of sources, including metallurgy, vehicle exhaust, coal burning and rubber tire wear [Citation60]. So F4 was the mixed source of metal smelting and traffic-related source.
According to the results of the PMF model (Figure 4), the contribution of sources to OPDTTv was 5.6% for F1, 75.7% for F2, 0.5% for F3, 18.2% for F4, respectively; the contribution of sources to OPAA was 66.4% for F2 and 33.6% for F4, respectively. So more than 50% of OP of ambient PM2.5 in Nanjing was resulted from F2, indicating that combustion source of fossil fuel was the main contributor of OP of ambient PM2.5 in Nanjing. Strategies of control reducing toxicity of PM2.5 should pay more attention to the combustion sources of fossil fuel.
4. Conclusions
OP of PM2.5 collected from the unban traditional center district and the suburb in Nanjing from Jan. 2020 to Jun. 2021 was determined by using DTT and AA assays. The seasonal and spatial variation of both OPDTTv and OPAAv of PM2.5 in the unban traditional center district and the suburb in Nanjing were observed. OP values analyzed by using DTT assay were higher than those by using AA assay. Moreover, different correlation for OPDTT and OPAA with PM2.5-bound components was found, which may be the reason for higher OPDTT values than OPAA. The PMF analysis shows that the main potential source for OP in Nanjing was the combustion sources of fossil fuel. This study shows that OP provide a good index for evaluating the integrated toxicity of PM2.5 in different district (e.g. the unban traditional center district and the suburb) and OP using AA assay may underestimate the potential health risks posed by PM2.5.
Data availability
All data reported here can be made available on request
Disclosure statement
No potential conflict of interest was reported by the author(s).
Additional information
Funding
References
- Delfino RJ, Staimer N, Tjoa T, et al. Airway inflammation and oxidative potential of air pollutant particles in a pediatric asthma panel[J]. J Expo Sci Environ Epidemiol. 2013;23(5):466–10.
- Janssen NAH, Strak M, Yang A, et al. Associations between three specific a-cellular measures of the oxidative potential of particulate matter and markers of acute airway and nasal inflammation in healthy volunteers[J]. Occup Environ Med. 2015;72(1):49–56.
- Bates JT, Weber RJ, Abrams J, et al. Reactive oxygen species generation linked to sources of atmospheric particulate matter and cardiorespiratory effects[J]. Environ Sci Technol. 2015;49(22):13605–13612.
- Leni Z, Kunzi L, Geiser M. Air pollution causing oxidative stress[J]. Curr Opin Toxicol. 2020;20-21:1–8.
- Lim CC, Thurston GD. Air pollution, oxidative stress, and diabetes: a life course epidemiologic perspective[J]. Curr Diab Rep. 2019;19(8):58.
- Vo TTT, Wu C-Z, Lee IT. Potential effects of noxious chemical-containing fine particulate matter on oral health through reactive oxygen species-mediated oxidative stress: promising clues[J]. Biochem Pharmacol. 2020;182:114286.
- Yang L, Hou X-Y, Wei Y, et al. Biomarkers of the health outcomes associated with ambient particulate matter exposure[J]. Sci Total Environ. 2017;579:1446–1459.
- Lee C-W, Vo TTT, Wu C-Z, et al. The inducible role of ambient particulate matter in cancer progression via oxidative stress-mediated reactive oxygen species pathways: a recent perception[J]. Cancers (Basel). 2020;12(9):2505.
- EPA US. National primary and secondary ambient air quality standards[S]. Washington DC: United States Environmental Protection Agency; 2015.
- MEEC, GAQSIQC. Ambient air quality standards (GB 3095-2012)[S]. Beijing: Ministry of environment protection of China (MEEC), General administration of quality supervision, inspection and quarantine of China (GAQSIQC). 2012 (In Chinese) .
- WHO global air quality guidelines: particulate matter (PM2.5 and PM10), ozone, nitrogen dioxide, sulfur dioxide and carbon monoxide. Licence: CC BY-NC-SA 3.0 IGO. World Health Organization: Geneva, Switzerland. 2021.
- Gao D, Ripley S, Weichenthal S, et al. Ambient particulate matter oxidative potential: chemical determinants, associated health effects, and strategies for risk management[J]. Free Radical Bio Med. 2020;151:7–25.
- Rao LF, Zhang LY, Wang XZ, et al. Oxidative potential induced by ambient particulate matters with acellular assays: a review[J]. Processes. 2020;8(11):1410
- Guo X, Zhang N, Hu X, et al. Characteristics and potential inhalation exposure risks of PM2.5–bound environmental persistent free radicals in Nanjing, a mega–city in China[J]. Atmos Environ. 2020;224. 117355.
- Dong H, Xu D, Hu L, et al. Evaluation of N-acetyl-cysteine against tetrachlorobenzoquinone-induced genotoxicity and oxidative stress in HepG2 cells[J]. Food Chem Toxicol. 2014;64:291–297.
- Saed GM, Jiang ZL, Fletcher NM, et al. Exposure to polychlorinated biphenyls enhances lipid peroxidation in human normal peritoneal and adhesion fibroblasts: a potential role for myeloperoxidase[J]. Free Radical Bio Med. 2010;48(6):845–850.
- Zhang S, Yang T, Xu X, et al. Oxidative stress and nitric oxide signaling related biomarkers in patients with pulmonary hypertension: a case control study[J]. BMC Pulm Med. 2015;15(1):50.
- Dong H, Shi Q, Song X, et al. Polychlorinated biphenyl quinone induces oxidative DNA damage and repair responses: the activations of NHEJ, BER and NER via ATM-p53 signaling axis[J]. Toxicol Appl Pharm. 2015;286(1):10–16.
- Saffari A, Daher N, Shafer MM, et al. Seasonal and spatial variation in dithiothreitol (DTT) activity of quasi-ultrafine particles in the Los Angeles Basin and its association with chemical species[J]. J Environ Sci Heal A. 2014;49(4):441–451.
- Guo HB, Li M, Lyu Y, et al. Size-resolved particle oxidative potential in the office, laboratory, and home: evidence for the importance of water-soluble transition metals[J]. Environ Pollut. 2019;246:704–709.
- Samake A, Uzu G, Martins JMF, et al. The unexpected role of bioaerosols in the oxidative potential of PM[J]. Sci Rep-UK. 2017;7(1):10978.
- Fang T, Zeng L, Gao D, et al. Ambient size distributions and lung deposition of aerosol dithiothreitol-measured oxidative potential: contrast between soluble and insoluble particles[J]. Environ Sci Technol. 2017;51(12):6802–6811.
- Bates JT, Fang T, Verma V, et al. Review of acellular assays of ambient particulate matter oxidative potential: methods and relationships with composition, sources, and health effects[J]. Environ Sci Technol. 2019;53(8):4003–4019.
- Calas A, Uzu G, Kelly FJ, et al. Comparison between five acellular oxidative potential measurement assays performed with detailed chemistry on PM10 samples from the city of Chamonix (France)[J]. Atmos Chem Phys. 2018;18(11):7863–7875.
- Visentin M, Pagnoni A, Sarti E, et al. Urban PM2.5 oxidative potential: importance of chemical species and comparison of two spectrophotometric cell-free assays[J]. Environ Pollut. 2016;219:72–79.
- Vreeland H, Weber R, Bergin M, et al. Oxidative potential of PM2.5 during Atlanta rush hour: measurements of in-vehicle dithiothreitol (DTT) activity[J]. Atmos Environ. 2017;165. 169–178.
- Janssen NAH, Yang A, Strak M, et al. Oxidative potential of particulate matter collected at sites with different source characteristics[J]. Sci Total Environ. 2014;472:572–581.
- Fang T, Verma V, Bates JT, et al. Oxidative potential of ambient water-soluble PM2.5 in the Southeastern United States: contrasts in sources and health associations between ascorbic acid (AA) and dithiothreitol (DTT) assays[J]. Atmos Chem Phys. 2016;16(6):3865–3879.
- Wu L, Luo X-S, Li H, et al. Seasonal levels, sources, and health risks of heavy metals in atmospheric PM2.5 from four functional areas of Nanjing city, Eastern China[J]. Atmosphere. 2019;10(7):419.
- Zheng H, Yi W, Ding Z, et al. Evaluation of life expectancy loss associated with submicron and fine particulate matter (PM1 and PM2.5) air pollution in Nanjing, China[J]. Environ Sci Pollut R. 2021;28(48):68134–68143.
- Cho AK, Sioutas C, Miguel AH, et al. Redox activity of airborne particulate matter at different sites in the Los Angeles Basin[J]. Environ Res. 2005;99(1):40–47.
- Gao D, Fang T, Verma V, et al. A method for measuring total aerosol oxidative potential (OP) with the dithiothreitol (DTT) assay and comparisons between an urban and roadside site of water-soluble and total OP[J]. Atmos Meas Tech. 2017;10(8):2821–2835.
- Pietrogrande MC, Bertoli I, Manarini F, et al. Ascorbate assay as a measure of oxidative potential for ambient particles: evidence for the importance of cell-free surrogate lung fluid composition[J]. Atmos Environ. 2019;211. 103–112.
- Laurent YA, Laure L, Esperanza P, et al. PM10 metal concentrations and source identification using positive matrix factorization and wind sectoring in a French industrial zone[J]. Atmos res. 2010;96(4): 612–625
- Hu X, Ding Z, Zhang Y, et al. Size distribution and source apportionment of airborne metallic elements in Nanjing, China[J]. Aerosol Air Qual Res. 2013;13:1796–1806.
- Brehmer C, Lai A, Clark S, et al. The oxidative potential of personal and household PM2.5 in a rural setting in Southwestern China[J]. Environ Sci Technol. 2019;53(5):2788–2798.
- Liu Q, Baumgartner J, Zhang Y, et al. Oxidative potential and inflammatory impacts of source apportioned ambient air pollution in Beijing[J]. Environ Sci Technol. 2014;48(21):12920–12929.
- Puthussery JV, Singh A, Rai P, et al. Real-time measurements of PM2.5 oxidative potential using a dithiothreitol assay in Delhi, India[J]. Environ Sci Tech Let. 2020;7(7):504–510.
- Chen Q, Wang M, Wang Y, et al. Oxidative potential of water-soluble matter associated with chromophoric substances in PM2.5 over Xi’an, China[J]. Environ Sci Technol. 2019;53(15):8574–8584.
- Weichenthal S, Shekarrizfard M, Traub A, et al. Within-city spatial variations in multiple measures of PM2.5 oxidative potential in Toronto, Canada[J]. Environ Sci Technol. 2019;53(5):2799–2810.
- Pietrogrande MC, Dalpiaz C, Dell’anna R, et al. Chemical composition and oxidative potential of atmospheric coarse particles at an industrial and urban background site in the alpine region of northern Italy[J]. Atmos Environ. 2018;191. 340–350.
- Chirizzi D, Cesari D, Guascito MR, et al. Influence of Saharan dust outbreaks and carbon content on oxidative potential of water-soluble fractions of PM2.5 and PM10[J]. Atmos Environ. 2017;163. 1–8.
- Paraskevopoulou D, Bougiatioti A, Stavroulas I, et al. Yearlong variability of oxidative potential of particulate matter in an urban Mediterranean environment[J]. Atmos Environ. 2019;206. 183–196.
- Patel A, Rastogi N. Oxidative potential of ambient fine aerosol over a semi-urban site in the Indo-Gangetic Plain[J]. Atmos Environ. 2018;175:127–134.
- Fang T, Guo H, Zeng L, et al. Highly acidic ambient particles, soluble metals, and oxidative potential: a link between sulfate and aerosol toxicity[J]. Environ Sci Technol. 2017;51(5):2611–2620.
- Romano S, Becagli S, Lucarelli F, et al. Oxidative potential sensitivity to metals, Br, P, S, and Se in PM10 samples: new insights from a monitoring campaign in Southeastern Italy[J]. Atmosphere. 2020;(2020(11):367.
- Cheng Y, Engling G, He KB, et al. Biomass burning contribution to Beijing aerosol[J]. Atmos Chem Phys. 2013;13(15):7765–7781.
- Boreddy SKR, Kawamura K, Okuzawa K, et al. <atl>Temporal and diurnal variations of carbonaceous aerosols and major ions in biomass burning influenced aerosols over Mt. Atmos Environ. 2017;154. 106–117.
- Chuang MT, Chou CCK, Sopajaree K, et al. Characterization of aerosol chemical properties from near-source biomass burning in the northern Indochina during 7-SEAS/Dongsha experiment[J]. Atmos Environ. 2013;78. 72–81.
- Yang A, Jedynska A, Hellack B, et al. Measurement of the oxidative potential of PM2.5 and its constituents: the effect of extraction solvent and filter type[J]. Atmos Environ. 2014;83. 35–42.
- Fang GC, Huang YL, Huang JH. Study of atmospheric metallic elements pollution in Asia during 2000–2007[J]. J Hazard Mater. 2010;180(1–3):115–121.
- Hjortenkrans DST, Bergb€ack BG, H€aggerud AV. Metal emissions from brake linings and tires: case studies of Stockholm, Sweden 1995/1998 and 2005[J]. Environ Sci Technol. 2007;41(15):5224–5230.
- Pant P, Baker SJ, Shukla A, et al. The PM10 fraction of road dust in the UK and India: characterization, source profiles and oxidative potential[J]. Sci Total Environ. 2015;530-531. 445–452.
- Verma SK, Masto RE, Gautam S, et al. Investigations on PAHs and trace elements in coal and its combustion residues from a power plant[J]. Fuel. 2015;162:138–147.
- Xu B, Song Y, Tian Y, et al. Sulfur content in coal formed during different geologic periods in the Guangxi Province and the relationship with the depositional environment[J]. Arab J Geosci. 2020;13(7):294.
- Chu VT, Ristovski Z, Surawski N, et al. Effect of sulphur and vanadium spiked fuels on particle characteristics and engine performance of auxiliary diesel engines[J]. Environ Pollut. 2018;243:1943–1951.
- Winnes H, Moldanová J, Anderson M, et al. On-board measurements of particle emissions from marine engines using fuels with different sulphur content[J]. P I Mech Eng M-J Eng. 2016;230(1): 45–54
- Kunli L, Xinmin Z, Changhe C, et al. Estimate of arsenic emission amount from the coal power stations in China[J]. Chin Sci Bull. 2004;49:2183–2189.
- Tian HZ, Wang Y, Xue ZG, et al. Trend and characteristics of atmospheric emissions of Hg, As, and Se from coal combustion in China, 1980–2007[J]. Atmos Chem Phys. 2010;10:11905–11919.
- Harrison RM, Jones AM, Gietl J, et al. Estimation of the contributions of brake dust, tire wear, and resuspension to nonexhaust traffic particles derived from atmospheric measurements[J]. Environ Sci Technol. 2012;46(12):6523–6529.