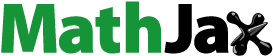
ABSTRACT
This study aimed to quantify and map habitat quality in the Dire and Legedadi watersheds between 1985 and 2022 using the InVEST habitat quality model. The purpose of this study was to assess the spatiotemporal changes in habitat quality in the Dire and Legedadi watersheds. The result showed, between 1985 and 2022, both watersheds experienced a significant decline in habitat quality. The extent of high habitat quality habitat in the Legadadi watershed has decreased significantly from 206 km2 in 1985 to 50.26 km2 in 2022. Similarly, high habitat quality habitat in the Dire watershed has gradually declined from 87.29 km2 in 1985 to 35.44 km2 in 2022. The construction of the Dire water reservoir in 1999 helped to maintain the extent of moderate habitat quality which increased from 6.93 km2 in 1995 to 25.36 km2 in 2010. The rapid expansion of farmland and settlement at the expense of natural vegetation was strongly linked to the decline in habitat quality. As a result, appropriate watershed management intervention is required. Therefore, the outcome of this research will provide a scientific basis for future ecosystem monitoring to monitor the impacts of watershed management interventions.
1. Introduction
A high-biodiversity ecosystem is regarded as a high-quality habitat that contributes to improved ecosystem processes (Johnson, Citation2007). The decline in biodiversity resources and intactness, on the other hand, is having a significant negative impact on habitat quality as a result of several unprecedented Land Use Land Cover (LULC) changes such as increased settlement, farming, and artificial monoculture plantations (Abreham et al., Citation2020; Hamere et al., Citation2021; H. Zhang et al., Citation2020). The degradation of habitat quality was primarily caused by intensive land use expansion associated with human activities (Liang and Liu, Citation2017). As a result, the proximity to and intensity of adverse land uses has a significant impact on ecosystem functioning and process (Fu et al., Citation2013; Yan et al., Citation2018), and the ability to support ecosystem services that are necessary to produce all ecosystem services can be deteriorated (Polasky et al., Citation2011; Thomas et al., Citation2021; Villamagna et al., Citation2013).
Similarly, the study watersheds have been significantly affected by LULC (Simeneh et al., Citation2023a). Natural forests and grassland were the dominant land cover types until the late 1980s when they were largely replaced by farmland and settlement (Addis Ababa Water and Sewerage Authority [AAWSA], Citation2016; Awraris, Citation2017). Due to the increased deterioration of natural habitats in both watersheds, the water quality in the reservoirs was reported to be very low (Helnata, Citation2019; Taye, Citation2009). Understanding landscape patterns, the interaction of human activities, and the effects of land conversion on natural phenomena is critical for proper land management and decision-making that contributes to the preservation of ecosystem integrity (Rawat & Kumar, Citation2015). The study of watersheds’ rapid LULC changes and their effects on the quality and quantity of ecosystem services are poorly known and less understood. Assessing the effects of LULC changes on habitat quality and Ecosystem Service Values (ESVs) in the Dire and Legedadi watersheds can help with proper ecosystem management intervention, which can support humans meet a variety of economic and environmental needs.
Assessments of habitat quality and ecological risk are required as part of sustainable ecosystem management to reduce the negative effects of anthropogenic disturbances (Y. Zhang et al., Citation2022). This study’s assessment model can be used as an effective decision-support tool to prioritize important ecological areas and can also be adapted for use in ecosystem management intervention (Y. Zhang et al., Citation2022). The availability of biodiversity resources in a specific environment and the environment’s ability to provide suitable living conditions are influenced by quality habitat (Hall et al., Citation1997). It is regarded as a critical representation of regional biodiversity and ecosystems, as well as a critical link in ensuring regional ecological security and enhancing human well-being (Chen et al., Citation2016;). Understanding the temporal and geographical aspects of habitat quality is the foundation for developing land-use planning and management (Liu et al., Citation2018).
The environmental level that the ecological environment provides for the survival of individual organisms and populations is referred to as habitat quality (Yanan et al., Citation2022). It is a variable with a numerical range ranging from low to high (Sharp et al., Citation2020; Yanan et al., Citation2022). The higher the quality of the habitat, the more stable the ecosystem’s ecological structure and function (Sharp et al., Citation2020; Yanan et al., Citation2022). The manner and intensity with which human land use determines habitat quality, and the more intense the land use, the more pronounced the decline in habitat quality (Almpanidou et al., Citation2014). To quantify habitat quality, the Integrated Valuation of Ecosystem Services and Tradeoffs (InVEST) model has been widely used (Abreham et al., Citation2020; Hamere et al., Citation2021; Mohsen & Mohammadyari, Citation2023; H. Zhang et al., Citation2020). The model provides an excellent research method and perspectives (Romero‐Calcerrada & Luque, Citation2006; Terrado et al., Citation2016), as well as information on land use and threats to computing habitat quality. The spatial extent of habitat quality within the landscape was determined by the habitat’s proximity to human-dominated land use and the intensity of disturbance caused by the land use (Sharp et al., Citation2020). We hypothesize that habitat quality deteriorated over time as a result of threat factors present in the study area. As a result, the purpose of this study was to assess the spatiotemporal changes in habitat quality in the Dire and Legedadi watersheds using InVEST software. The Legedadi and Dire watersheds the main water supply for Addis Ababa city (AAWSA, Citation2011, Citation2016). Unlikely, the watershed’s natural environment has been under rapid changes (AAWSA, Citation2016; Awraris, Citation2017; Simeneh et al., Citation2023a) and impacted the eco-hydrological processes (Ajanaw & Miano, Citation2021; Simeneh et al., Citation2023b; Sisay, Citation2014), the quality and quantity of water (Helnata, Citation2019; Taye, Citation2009). Therefore, the establishment of sustainable watershed management intervention is highly essential. Since the development of a robust ecosystem monitoring system is crucial to monitor changes; the outcome of this study can be used as a baseline for future ecosystem service monitoring assessment in the study watersheds.
2. Materials and methods
2.1. The study area
The Legedadi and Dire are critical watersheds, covering 215 km2 and 97.5 km2 respectively. They are located in Oromia regional state, approximately 35 kilometers northeast of Addis Abeba, between 09 01’00“and 09 13’ 00” N latitude and 38 50’ 00“−39 07’ 00” E longitude (Figure ). They are sub-catchments of the Akaki River basin, which flows northeast-southwest, and are part of the drainage system that forms the northwest corner of the Awash River basin.
2.2. The InVEST habitat quality model
The InVEST model offers a good research method as well as perspectives (Abreham et al., Citation2020; Hamere et al., Citation2021; Romero‐Calcerrada & Luque, Citation2006; Sharp et al., Citation2020; Terrado et al., Citation2016; H. Zhang et al., Citation2020). To compute habitat quality, the model incorporates information on land use and threats. The spatial extent of habitat quality within the landscape was determined by the habitat’s proximity to human-dominated land use and the intensity of disturbance caused by the LULC (Sharp et al., Citation2020). The model considers LULC with higher habitat quality to be relatively intact and capable of supporting increased biodiversity, whereas LULC with lower habitat quality indicates reduced biodiversity support and denotes a degraded habitat (Baral et al., Citation2014). The model is based on the relative impact of threats to habitats, the distance between the threat source and the habitat, and the sensitivities of specific habitats to any potential threats that could lead to habitat degradation (Chen et al., Citation2016; Sharp et al., Citation2020).
For this study, five biodiversity threats were identified, namely settlement, farming, eucalyptus plantation, road, and land degradation, and the significance (weight) of each threat was prioritized based on the opinions of local key informants, experts’ knowledge, and review of the literature (Abreham et al., Citation2020; Hamere et al., Citation2021), using the approach developed by Terrado et al. (Citation2016) and Wu et al. (Citation2014) (Table ).
Table 1. Ecological habitat quality input data used for InVEST habitat quality model in the Dire and Legedadi watersheds
The key inputs of habitat quality in the InVEST model, according to Sharp et al. (Citation2020), are the suitability of each LULC type (Hj) for providing habitat for biodiversity; second, anthropogenic threats that originate at pixel x (rx) affecting habitat quality; and third, the sensitivity of each LULC type to each threat (Table ).
The total threat level in a grid cell x with LULCj is calculated as the relative habitat suitability score (Hj), which ranges from 0 to 1, with 1 indicating the highest suitability to species (Sharp et al., Citation2020). The model’s final input is the sensitivity of habitat type to different threats; this allows for the differentiated impacts of threats on different habitats to be accounted for. Threat impacts on habitat are determined by: 1) the threat’s effect over space (irxy); 2) the relative weight of each threat’s importance compared to the others (wr); and 3) a relative sensitivity of each habitat to respective threat (Sjr) (Sharp et al., Citation2020). The grid x’s stress level Dxj with land-use type j is calculated as follows.
where R= number of threat factors, yr = set of grid cells on r’s map, wr =relative effect of each threat, θx = level of accessibility to a grid cell x, and Sjr = relative sensitivity of each habitat types to each threat (Sharp et al., Citation2020).
The model’s output ranges from 0 to 1, with 1 representing the highest level of habitat quality (Sharp et al., Citation2020). Threats with higher destructive values (on a scale of 0–1) have higher impacts on habitat, and the more sensitive a habitat type is to a threat (higher Sjr), the more degraded the habitat type could be by the threat.
The degree of habitat degradation was used to calculate habitat quality, and the habitat quality score decreased as the degree of habitat degradation increased (Sharp et al., Citation2020; Yanan et al., Citation2022). The habitat quality calculation formula is as follows (Sharp et al., Citation2020).
where, Qxj is the habitat quality of grid cell x in land cover type j; Hj is the habitat suitability of land cover type j; Dz xj is the level of habitat threat for grid cell x in land cover type j; k is the half-saturation factor, which is generally taken as half of the maximum value of Dz xj; and x is a constant.
2.3. Data preparation and input for the InVEST-habitat quality model
The InVEST habitat quality model requires data inputs (both spatial and non-spatial) (Figure ). Thus, LULC maps, threat sources and impacts, habitat types, habitat sensitivity to each threat, and half-saturation constant were all required inputs (Sharp et al., Citation2020). The information on LULC was obtained from Simeneh’s (2023) previous study in the study area (Figures ). To run the habitat quality model, all of the required inputs were loaded, including LULC maps for the respective years, threat sources and impacts, habitat types, and habitat sensitivity to each threat. Finally, habitat quality maps for each year were created; the final habitat quality maps were classified into three categories (low, moderate, and high).
3. Results and discussions
Between 1985 and 2010, the watersheds were dominated by high habitat quality. The extent of high habitat quality in the Legadadi watershed has decreased significantly from 206 km2 (95.5%) in 1985 to 179.5 km2 (83.2%) in 1995 and then to 156.85 km2 (72.66%) in 2010. However, by 2022, the high habitat quality would have dropped to 50.26 km2 (23.28%). Low habitat quality, on the other hand, increased from 9.62 km2 (4.46%) in 1985 to 36.31 km2 (16.82%) in 1995, then to 59 km2 (27.34%) in 2010 and 165 km2 (76.72%) in 2022. (Table ; Figures ).
Table 2. Habitat quality changes in the Legedadi and Dire watersheds using InVEST habitat quality model (1985, 2022)
In the Dire watershed, the extent of high habitat quality has gradually declined between 1985 and 2022, from 87.29 km2 (89.84%) in 1985 to 78.23 km2 (80.56%) in 1995, 71.81 km2 (73.9%) in 2010, and 35.44 km2 (36.48%) in 2022. Low habitat quality, on the other hand, has increased from 9.87 km2 (10.16%) in 1985 to 11.95 km2 (12.31%) in 1995 and then to 49 km2 (50.44%) in 2022. (Table ; Figures ). The construction of the Dire water reservoir in 1999 helped to maintain the extent of moderate habitat quality. It increased from 6.93 km2 (7.14%) in 1995 to 25.36 km2 (26.1%) in 2010 but then decreased to 12.71 km2 (13.08%) in n 2022 (Table ; Figures ).
In the last 37 years, the quality of the habitat has deteriorated dramatically. In general, high-quality habitats in 1985 degraded into low-quality habitats by 2022 (Table ). This is due to an unprecedented increase in anthropogenic pressure, which has resulted in severe changes in the ecosystem’s healthy functioning. A similar finding was reported that only 1.96 % of the area has high environmental quality; the medium, poor extremely deteriorated quality environment consisted of 77%, 13.30%, and 8.12%, respectively (AAWSA, Citation2011). Rapid anthropogenic LULC changes associated with the expansion of human settlements and artificial plantations may result in a significant loss of habitat quality (Abreham et al., Citation2020; Hamere et al., Citation2021; Jie et al., Citation2015; Li et al., Citation2018; Liting et al., Citation2019), pose a threat to biodiversity (Sun et al., Citation2019) and led to a decline in the ESs (Mohammadyari et al., Citation2023). Similarly, rapid urbanization and agricultural expansion have destroyed previously dominant natural habitats, resulting in significant habitat quality degradation in watersheds. Natural habitat loss can have a variety of effects on the larger ecosystem and have a significant impact on human well-being. The magnitude of poor habitat quality has grown significantly as settlement and cultivation areas have expanded. With a declining trend, high habitat quality values have only occurred in areas where natural habitats and reservoirs are located. Human activities, particularly settlement and urbanization, unrestricted grazing, deforestation, and agricultural practices, have the potential to degrade habitat quality and disrupt ecological processes. Similar findings have been reported in the Winkie and Beressa watersheds (Abreham et al., Citation2020; Hamere et al., Citation2021). Furthermore, eucalyptus plantations’ dominance and land degradation have contributed to the watershed’s declining habitat quality (Hamere et al., Citation2021).
4. Limitations of the study
The assessment of habitat quality using the InVEST model has been successfully used for biodiversity conservation and is invaluable for landscape management and planning (Sharp et al., Citation2020). The major limitations of the InVEST habitat quality model are still insufficient information about species’ spatial and temporal distribution across the landscape (Stephen et al., Citation2011). To obtain ecologically valid and robust information on the distribution of quality habitat and species abundance across watersheds, it is critical to conduct a field-based habitat suitability assessment (Nagendra et al., Citation2013). Another disadvantage of the InVEST habitat quality model is that it does not account for the impact of soil, climate, hydropower generation, edge effect, and vegetation differences in the habitat (Y. Zhang et al., Citation2014). Furthermore, the maximum effective distance of the threat and the weights of each ecological threat factor are heavily reliant on the model dataset parameters and expert judgments (Baral et al., Citation2014; Terrado et al., Citation2016). As a result, the expert’s judgment has had a significant impact on the habitat quality modeling work, which is one of the model’s major limitations (Abreham et al., Citation2020).
5. Conclusion
By mapping critical habitats for ecosystem conservation, habitat quality modeling helps in sustainable land management initiatives. The rapid expansion of farmland and settlement in the study watersheds has largely resulted in a decline in habitat quality. The decline in habitat quality is expected to continue. A sustainable water management intervention should be implemented based on the level of habitat degradation to improve habitat quality in both watersheds. It is also critical to ensure that the watershed management intervention causes little or no economic loss to other land uses (mainly for agriculture) through implementing appropriate incentive-based watershed management practices in the study landscapes. Thus, this study will assist decision-makers in appropriately planning for sustainable watershed management practices in the study watersheds.
Authors’ contributions
‘SA designed the research, collects relevant data, analyzed data and wrote the draft manuscript and KY and MA contributed to the work through their persistent guidance, reviewing and editing. All authors read and approved the final manuscript’.
Supplemental Material
Download MS Word (26 KB)Acknowledgements
The authors are grateful for the support provided by Bereh woreda agriculture and natural resource protection office, and Addis Ababa Water and Sewage Authority (AAWSA). The journal’s anonymous reviewers and Editor in Chief are also thanked for their critical comments that improved the manuscript.
Disclosure statement
No potential conflict of interest was reported by the author(s).
Data availability statement
The authors want to declare that they can submit the data at whatever time based on request. The data used for the current study are available from the corresponding author upon reasonable request.
Supplementary material
Supplemental data for this article can be accessed online at https://doi.org/10.1080/27658511.2023.2242137
Additional information
Funding
References
- Abreham, B., Noszczyk, T., Soromessa, T., & Elias, E. (2020). The InVEST habitat quality model associated with land use/cover changes: A qualitative case study of the winike watershed in the Omo-Gibe Basin, Southwest Ethiopia. Remote Sensing, 12(7), 1103. https://doi.org/10.3390/rs12071103
- Addis Ababa Water and Sewerage Authority. (2011). Master plan review, catchment rehabilitation, and awareness creation for geffersa, legedadi, and dire catchments. Addis Ababa.
- Addis Ababa Water and Sewerage Authority. (2016). Urban water supply and sanitation; Legedadi and Dire dam rehabilitation project. Addis Ababa.
- Ajanaw, N., & Miano, T. M. (2021). Impacts of land use and land cover change on soil erosion and hydrological responses in Ethiopia. Applied & Environmental Soil Science Article ID 6669438, 2021, 1–9. https://doi.org/10.1155/2021/6669438
- Almpanidou, V., Mazaris, A. D., Mertzanis, Y., Avraam, I., Antoniou, I., Pantis, J. D., & Sgardelis, S. P. (2014). Providing insights on habitat connectivity for male brown bears: A combination of habitat suitability and landscape graph-based models. Ecological Modelling, 286, 37–44. https://doi.org/10.1016/j.ecolmodel.2014.04.024
- Awraris, M., (2017). Analysis of the impact of land use land cover dynamics on soil erosion using the RUSLE model: The case of water supply reservoir watersheds around Addis Ababa [ M.Sc thesis]. Addis Ababa University.
- Baral, H., Keenan, R. J., Sharma, S. K., Stork, N. E., & Kasel, S. (2014). Spatial assessment and mapping of biodiversity and conservation priorities in a heavily modified and fragmented production landscape in north-central Victoria, Australia. Ecological Indicators, 36, 552–562. https://doi.org/10.1016/j.ecolind.2013.09.022
- Chen, Y., Qiao, F., & Jiang, L. (2016). Effects of land use pattern change on regional scale habitat quality based on InVEST modeld—a case study in Beijing. Acta Scientiarum Naturalium Universitatis Pekinensis, 52, 553–562. https://doi.org/10.13209/j.0479-8023.2016.057
- Fu, B., Wang, S., Su, C., & Forsius, M. (2013). Linking ecosystem processes and ecosystem services. Current Opinion in Environmental Sustainability, 5(1), 4–10. https://doi.org/10.1016/j.cosust.2012.12.002
- Hall, L. S., Krausman, P. R., & Morrison, M. L. (1997). The habitat concept and a plea for standard terminology. Wildl Soc Bull, 25, 171–182.
- Hamere, Y., Soromessa, T., Argaw, M., & Dewan, A. (2021). Spatio-temporal change in habitat quality and linkage with landscape characteristic in the Beressa watershed of Ethiopian highlands. Journal of Environmental Management, 281, 11188. https://doi.org/10.1016/j.jenvman.2020.111885
- Helnata Tilahun. (2019). Key drivers of water quality in Dire and Legedadi reservoirs, Ethiopia [ M.Sc thesis]. Addis Ababa University.
- Jie, G., Feng, L., Hui, G., Zhou, C., & Zhang, X. (2015). The impact of land‐use changes on water‐related ecosystem services: A study of the Guishui River Basin, Beijing, China. Journal of Cleaner Production, 163, S148–S155. https://doi.org/10.1016/j.jclepro.2016.01.049
- Johnson, M. D. (2007). Measuring habitat quality: A review. The Condor, 109(3), 489–504. https://doi.org/10.1093/condor/109.3.489
- Liang, Y., & Liu, L. (2017). Simulating land use change and its effect on biodiversity conservation in a watershed in northwest China. Ecosystem Health & Sustainability, 3, 1335933.
- Li, X., Hou, X., Song, Y., Shan, K., Zhu, S., Yu, X., & Mo, X. (2018). Assessing habitat quality changes for shorebirds in stopover sites: A case study in yellow river Delta, China. Wetlands, 39(1), 67–77. https://doi.org/10.1007/s13157-018-1075-9
- Liting, X., Shuang Chen, S., Xu, Y., Guangyu, L., & Weizhong, S. (2019). Impacts of land-use change on habitat quality during 1985–2015 in the Taihu Lake Basin. Sustainability, 11(13), 3513. https://doi.org/10.3390/su11133513
- Liu, C. F., Wang, C., & Liu, C. L. (2018). Spatio-temporal variation on habitat quality and its mechanism within the transitional area of the three natural zones: A case study in Yuzhong county. Geographical Research, 37, 419–432. https://doi.org/10.3390/ijerph19063594
- Mohammadyari, F., Zarandian, A., Mirsanjari, M. M., Suziedelyte Visockiene, J., & Tumeliene, E. (2023). Modelling impact of urban expansion on ecosystem services: A scenario-based approach in a mixed natural/urbanised landscape. Land, 12, 291. https://doi.org/10.1007/s11252-022-01297-6
- Mohsen, T., & Mohammadyari, F. (2023). Modeling the spatial distribution of multiple ecosystem services in Ilam dam watershed, Western Iran: Identification of areas for spatial planning. Urban Ecosystems, 26(2), 459–478. https://doi.org/10.1007/s11252-022-01297-6
- Nagendra, H., Lucas, R., Honrado, J. P., Jongman, R. H. G., Tarantino, C., Adamo, M., & Mairota, P. (2013). Remote sensing for conservation monitoring: Assessing protected areas, habitat extent, habitat condition, species diversity, and threats. Ecological Indicators, 33, 45–59. https://doi.org/10.1016/j.ecolind.2012.09.014
- Polasky, S., Nelson, E., Pennington, D., & Johnson, K. A. (2011). The impact of land-use changes on ecosystem services, biodiversity and returns to Landowners: A case study in the state of Minnesota. Environmental & Resource Economics, 48(2), 219–242. https://doi.org/10.1007/s10640-010-9407-0
- Rawat, J., & Kumar, M. (2015). Monitoring land use/cover change using remote sensing and GIS techniques: A case study of Hawalbagh block, district Almora, Uttarakhand, India. The Egyptian Journal of Remote Sensing & Space Science, 18(1), 77–84. https://doi.org/10.1016/j.ejrs.2015.02.002
- Romero‐Calcerrada, R., & Luque, S. (2006). Habitat quality assessment using Weights‐of‐Evidence based GIS modelling: The case of Picoides tridactylus as species indicator of the biodiversity value of the Finnish forest. Ecological Modelling, 196(1–2), 62–76. https://doi.org/10.1016/j.ecolmodel.2006.02.017
- Sharp, R., Douglass, J., Wolny, S., Arkema, K., Bernhardt, J., Bierbower, W., Chaumont, N., Denu, D., Fisher, D., Glowinski, K., Griffin, R., Guannel, G., Guerry, A., Johnson, J., Hamel, P., Kennedy, C., Kim, C. K., Lacayo, M. & Wyatt, K. (2020). The natural capital project, stanford university, university of Minnesota, the nature conservancy and world wildlife fund. InVEST User’s Guide.
- Simeneh, A., Yeshitela, K., & Argaw, M. (2023a). Impact of land use land cover changes on ecosystem service values in the Dire and Legedadi watersheds, central highlands of Ethiopia: Implication for landscape management decision making. Heliyon, 9(4), e15352. https://doi.org/10.1016/j.heliyon.2023.e15352
- Simeneh, A., Yeshitela, K., & Argaw, M. (2023b). Impacts of land use land cover changes and climate variability on water yield in the dire and legedadi watersheds central Ethiopia. Water Conservation Science and Engineering, 8(1), 14. https://doi.org/10.1007/s41101-023-00188-x
- Sisay Habtegebreal Mogesie. (2014). Impact of land use and land cover change on soil erosion potential in Legedadi reservoir watershed [ M.Sc thesis]. Addis Ababa University.
- Stephen, P., Jeremy Nelson, E., Pennington, D. N., & Johnson, K. A. (2011). The impact of land-use changes on ecosystem services, biodiversity and returns to landowners: A case study in the state of Minnesota. Environmental & Resource Economics, 48(2), 219–242. https://doi.org/10.1007/s10640-010-9407-0
- Sun, X., Jiang, Z., Liu, F., & Zhang, D. (2019). Monitoring spatio-temporal dynamics of habitat quality in Nansihu Lake basin, Eastern China, from 1980 to 2015. Ecological Indicators, 102, 716–723. https://doi.org/10.1016/j.ecolind.2019.03.041
- Taye, A., (2009). Impact of LULC change on catchment hydrology and water quality of Legedadi-Dire catchments [ M.Sc Thesis]. Addis Ababa University.
- Terrado, M., Sabater, S., Chaplin‐Kramer, B., Mandle, L., Ziv, G., & Acuna, V. (2016). Model development for the assessment of terrestrial and aquatic habitat quality in conservation planning. The Science of the Total Environment, 540, 63–70. https://doi.org/10.1016/j.scitotenv.2015.03.064
- Thomas, E., Jansen, M., Chiriboga-Arroyo, F., Wadt, L. H. O., Corvera-Gomringer, R., Atkinson, R. J., Bonser, S. P., Velasquez-Ramirez, M. G., & Ladd, B. (2021). Habitat quality differentiation and consequences for ecosystem service provision of an Amazonian hyperdominant tree species. Frontiers in Plant Science, 12, 621064. https://doi.org/10.3389/fpls.2021.621064
- Villamagna, A. M., Angermeier, P. L., & Bennett, E. M. (2013). Capacity, pressure, demand, and flow: A conceptual framework for analyzing ecosystem service provision and delivery. Ecological Complexity, 15, 114–121. https://doi.org/10.1016/j.ecocom.2013.07.004
- Wu, C.-F., Lin, Y. P., Chiang, L. C., & Huang, T. (2014). Assessing highway’s impacts on landscape patterns and ecosystem services: A case study in Puli township, Taiwan. Landscape and Urban Planning, 128, 60–71. https://doi.org/10.1016/j.landurbplan.2014.04.020
- Yanan, L., Duo, L., Zhang, M., Yang, J., & Guo, X. (2022). Habitat quality assessment of mining cities based on InVEST model—a case study of Yanshan County, Jiangxi Province. Jiangxi Province International Journal of Coal Science & Technology, 9(1), 28. https://doi.org/10.1007/s40789-022-00498-w
- Yan, S., Wang, X., Cai, Y., Li, C., Yan, R., Cui, G., & Yang, Z. (2018). An integrated investigation of spatiotemporal habitat quality dynamics and driving forces in the upper basin of Miyun Reservoir, North China. Sustainability, 10(12), 1–17. https://doi.org/10.3390/su10124625
- Zhang, Y., Qi, W., Zhou, C., Ding, M., Liu, L., Gao, J., Bai, W., Wang, Z., & Zheng, D. (2014). Spatial and temporal variability in the net primary production of alpine grassland on the Tibetan Plateau since 1982. Journal of Geographical Sciences, 24(2), 269–287. https://doi.org/10.1007/s11442-014-1087-1
- Zhang, H., Zhang, C., Hu, T., Zhang, M., Ren, X., & Hou, L. (2020). Exploration of roadway factor and habitat quality in InVEST. Transportation Research, Part D: Transport & Environment, 87, 102551. https://doi.org/10.1016/j.trd.2020.102551
- Zhang, Y., Zhang, C., Zhang, X., Wang, X., Liu, T., Li, Z., Lin, Q., Jing, Z., Wang, X., Huang, Q., Sun, W., Zhai, J., Tan, L., Wang, J., Zhou, G., Tian, Y., Hao, J., Song, Y., & Fei, M. (2022). Habitat quality assessment and ecological risks prediction: An analysis in the Beijing-hangzhou grand canal (Suzhou Section). Water, 14(17), 2602. https://doi.org/10.3390/w14172602