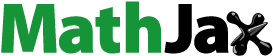
ABSTRACT
Using territorial- and consumption-based carbon emissions as proxies for carbon risk, the study examined the impact of innovation on carbon risk while controlling for institutional quality and financial development effects in the BRICS countries (Brazil, Russia, India, China, and South Africa) from 1986 to 2021. To address cross-sectional dependence and ensure robustness, we employed the augmented mean group (AMG) and cross-sectional autoregressive distributed lags (CS-ARDL) estimation techniques. Our findings show that innovation plays a key role in mitigating carbon risks, with a more pronounced effect on territorial carbon risk compared to consumption-based carbon risk. Furthermore, financial development exerts a positive influence on carbon risk, especially in the context of consumption-based emissions. Notably, institutional quality mitigates both forms of carbon risk. These outcomes suggest that BRICS countries should consider the types of carbon risk when formulating carbon emissions mitigation strategies.
1. Introduction
The challenge of climate change is attracting a lot of debate as it has become the single most serious environmental threat that affects all human endeavors globally (Khalfaoui et al., Citation2023; Liguo et al., Citation2022; Sheng & Lu, Citation2016; Usman et al., Citation2023; Zhou et al., Citation2023). In the two last decades, the emerging powers, particularly Brazil, Russia, India, China and South Africa (BRICS) have become dominant players in the world economy in terms of economic growth and carbon dioxide (CO2) emissions (proxy for carbon risk) resulting in shifts in the global economic and political order (Baloch & Wang, Citation2019). The Global Economic Prospects, for example, reports that the sluggish global growth in the past few years could be attributed to downturns in trade and investment in the BRICS economies.
The BRICS have become critical in the global governance of climate change because they have become the highest emitters of Greenhouse Gases (GHG). From the 1960s to date, on average, carbon risks for the BRICS have increased more than 10 times. Obviously, this is worth studying when one considers the fact that while carbon risks reduced in the developed countries from about 40% to 25%, it increased in the BRICS region from 27% to 42% from 1990 to date (BP Statistical Review of World Energy, Citation2019; The Global Energy and CO2 Status Report, Citation2019). This means that the BRICS are not only influencing but also being influenced by global energy markets and the clean energy transition and are described as having moved from the periphery to key players in global affairs (Downie & Williams, Citation2018; World Intellectual Property Organization WIPO, Citation2022). The current debate on climate mitigation and adaptation strategies have led many experts arguing for innovation to support technological progress to reduce carbon risk. Generally, innovation is measured in terms of research and development, patents, and trademarks (Liu, Citation2020; Mensah et al., Citation2018; Shahbaz et al., Citation2018). On average, there is an increase in the innovative capabilities of the BRICS economies (See World Intellectual Property Organization (WIPO), Citation2022). The Global Innovation Index (see Dutta et al., Citation2019) shows that China emits the most emissions, yet it is the most innovative among the BRICS nations. The Global Innovation Index (Citation2022) shows China ranked number 11 in the world (its highest rank ever) but number one for the BRICS. India is ranked at number 40 followed by Russia at 47, Brazil at 54 and South Africa at 61. The report notes that China is the middle-income economy within the top 30. It is important to also observe that South Africa is the least innovative among BRICS countries but the most innovative in Sub Sahara Africa.
China spends the most on R&D for the BRICS at 1.3% followed by Brazil at 1.1% and the least by India at 0.76% (World Bank, Citation2018). The averages mask the wide variation in R&D spending. For example, while China spent the least on R & D in the 1990s, by 2004, it spent more than any of the BRICS and by 2015, it was spending about two times (2.1% of GDP) while each of the countries was spending (0.9% of GDP on average). Also, available data suggests that the BRICS have an average of 110, 000 trademarks. For example, the average for South Africa (1960–2013) is just about 15, 000, compared to 70, 000 for Brazil (1964–2013), 42, 000 for Russia (1992–2013), 50, 000 for India (1963–2013), and over 380, 000 for China (1980–2013) (World Bank, Citation2018). Accordingly, the study seeks to examine how the increase in innovative capacity of the region is impacting on the evolution of carbon risk. Jiang et al. (Citation2022) describe the issue of the effect of innovation on carbon risk as the greatest problem facing the human society in recent times.
In addressing the research question, the study contributes to the extant literature in several ways. First, the study accounts for territorial- and consumption-based carbon risks. The territorial-based carbon risk captures the emissions from domestic activities only, while the consumption—based carbon risk is related to the domestic use of fossil fuels plus the embodied emissions from imports, minus exports (Hasanov et al., Citation2018, Adams & Opoku, Citation2020; Adam, Opoku and Aluko, Citation2021; Ulucak & Erdogan, Citation2022). Second, the paper is situated in the extant literature that indicates that the country or regional specific characteristics, particularly the institutional infrastructure matters in explaining environmental degradation. Indeed, the Global Innovation Index [GII] (Citation2018, Citation2019), WIPO (Citation2022) published by the World Intellectual Property Organization, Garrone and Grilli (Citation2010) reports that the positive effect of innovation is enhanced in a conducive institutional environment. Accordingly, the study accounts for institutional quality (government stability) to explain its independent and moderating effect on innovation, if any. Third, the estimation technique employed helps to identify the dynamic relationship between the variables of interest and the presence of nonlinearities to reduce estimation bias. Additionally, the study controls for the level of financial development as the credit-driven money provided by financial institutions allows firms and entrepreneurs to invest in innovation (Schumpeter, Citation1934). Also, financial development determines how the stability and structure of capital affect the intensity of carbon risk (Hillman & Baydoun, Citation2020; Omoke et al., Citation2023; Shahbaz et al., Citation2021). In this paper, financial development is defined basically as improvements in the efficiency, scope, and stability of and access to the financial system such that transaction cost is reduced. In the sections that follows, the literature review is presented and the methodology used is explained. The results are then discussed, conclusions given and policy implications offered.
2. Literature review
2.1. Theoretical review
Environmental innovations have been recognized to be critical in climate change mitigation (Liguo et al., Citation2022; Jiang & Khattak, Citation2023; Patterson & Huitema, Citation2019). Generally, energy innovations can measure both input (R&D) and output variables (patents and trademarks). Innovation systems usually consume less energy and consequently, produce less emissions (Ferreira et al., Citation2020; Monem & El Ghandour, Citation2020; Sinha et al., Citation2020). Innovation is assumed to have a positive effect on the environment because it is associated with advances in technology, which reduce carbon risk (Shi et al., Citation2023; Khan et al., Citation2020; Mensah et al., Citation2018). Cadez and Guilding (Citation2017) and Cadez and Czerny (Citation2016), for example, have argued that the lack of innovation can be described as the main reason for the continuous rise in carbon risks around the world. There is also the argument that technological innovations help to expand the scale and scope of economic activities that could lead to reduction in CO2 emissions (Georgatzi et al., Citation2020; Jiang & Khattak, Citation2023; Dinda, Citation2018; Wen et al., Citation2020; Yang & Li, Citation2019). Additionally, trademarks and patents provide benefits to organizations that give them a competitive edge, enhance firm value and sustain market positions (Castaldi, Citation2018). The enforcement of trademarks and patent rules support the commercialization of innovations and incentivizes firms to be more technologically oriented in promoting economic growth and environmental quality (Mensah et al., Citation2019; Wang et al., Citation2016).
Contrary to the positive view of innovation, other studies suggest that the effect of technology on carbon risk is uncertain due basically to the rebound effect or the so-called Jevons’ paradox, which indicates that energy efficiency improvements do not necessarily result in reduction of emissions as it is offset by increased energy use (Cansino et al., Citation2022; Kong et al., Citation2023; Shao et al., Citation2019; York et al., Citation2022). Indeed, Shao et al. (Citation2019) claim that the rebound effect could be described as an obstacle to energy saving policies driven by innovation and consequently a hindrance to sustainable development. Kong et al. (Citation2023) also noted that though improving energy efficiency is one of the most effective ways to reduce energy waste and achieve carbon neutrality, it may also bring the energy rebound effect and thus fail to achieve the desired energy conservations. In a study of Beijing, China, Kong et al. (Citation2023) reported that energy savings at the sector level is not ideal, and that in most cases, sectors with low energy efficiency have a higher rebound effect than sectors with high energy efficiency. The rebound effect idea is consistent with the observation of Garrone and Grilli (Citation2010) that many of the developed countries that have vigorously supported R & D over the past decades have not experienced reductions in carbon risks commensurate with the investments made. The authors therefore conclude that R & D spending does not automatically lead to improvements in environmental quality. Equally, Brookes (Citation1990) and Khazzoom (Citation1980) explain that the assumption that energy efficiency can lead to carbon risk reduction is fundamentally illogical.
With respect to the institutional environment, Haseeb et al. (Citation2018) suggests poor governance infrastructure explain many of the environmentally related problems. Government stability is an assessment both of the government’s ability to carry out its declared program(s), and its ability to stay in office. Countries with low government stability usually face a high level of political uncertainty and high transaction costs (ICRG, Citation2020). Government stability is a measure of political risk, which is measured by three main components, including government unity, legislative strength, and popular support of the government. This means that the translation of environmental problems into remedial environmental policy depend on social and political factors at both national and regional levels (Sarkodie & Adams, Citation2018). Obviously, government’s responsiveness to the citizenry is a key mechanism for promoting social, economic and environmental development such that institutional stability is usually a prerequisite for achieving environmental quality (Adedoyin et al., Citation2022; Root et al., Citation2015; Shah et al., Citation2020; Zhang et al., Citation2022). Khan et al. (Citation2022) have suggested that good institutions help government to protect and management the environment to enhance its quality.
The third variable of concern is financial development. There are opposing views on how financial development affects the environment as some studies claim that it promotes environmental quality, while others suggest otherwise. The positive view of financial development is indicative of the fact that it promotes resource allocation and technological advancement that helps to reduce carbon risks (Mu, Citation2018; Shahbaz et al., Citation2021). Additionally, some studies suggest that financial development could enhance research and development to produce advances in technology that have an adverse effect carbon risks (Khan et al., Citation2022). Zhao et al. (Citation2021) have also argued for a mediation relationship between financial risk and carbon risk such that increased financial risk has an indirect effect by promoting technological innovations that reduce emissions. On the other hand, the negative view of financial development suggests that it usually augments FDI in improving economic growth which is usually associated with increased energy use and carbon risk (Khan et al., Citation2022; Shahbaz et al., Citation2020). In support of this view, Sadorsky (Citation2010) explains that promoting financial development enhances access to loans to consumers who are able to purchase equipment that are energy intensive and consequently degrade the environment.
2.2. Empirical review
Khan et al. (Citation2020) examine the innovation—carbon risk link for China spanning the period 1990Q1 – 2017Q2 based on Generalized Least Squares (GLS) and report that, innovation reduces emissions. Similarly, Cai et al. (Citation2023) and Temesgen Hordofa et al. (Citation2023) studied the relationship for China and find that green innovations have a net reducing effect on carbon risk. In another study of China, however, Chen et al. (Citation2023) report an inverted U- shaped relationship between green innovations and carbon. Other studies also report differential, negative or negligible effect of innovations on emissions. For instance, Dauda et al. (Citation2019) employ the FMOLS and DOLS techniques to examine the innovation—emissions nexus for 18 countries over the period 1990–2016 and show that innovation contributes to environmental quality in the G-6 but has adverse effects in the BRICS and MENA regions. However, Rahman et al. (Citation2019) study economies in BRICS and NAFTA for the period 1992–2016 using the Panel Group Mean technique to show that innovation did not have a significant effect on environmental quality. Cheng et al. (Citation2019) show similar results for the OECD countries based on panel quantile regressions and OLS, Cheng et al. (Citation2019) for the BRICS and Du et al. (Citation2019) for a panel of 71 economies. At the country level, Ali et al. (Citation2016) demonstrate that technological innovations had no influence on carbon risks in Malaysia using the ARDL technique, while Wang et al. (Citation2012) report same for China and Weina et al. (Citation2016) for Italy.
Like the innovation studies, financial development studies show contradictory results. For example, Zhao et al. (Citation2021) examine the financial risk- emissions relationship for 62 countries over the period 2003–2018 and report that financial risk reduces emission. Similarly, Liu (Citation2020) study the case of China spanning the period 2007–2016 and report that financial development contributes to environmental quality. Using a global panel, Khan et al. (Citation2022) report of a positive effect of financial development on carbon. Sethi et al. (Citation2021) in a study of India during the period 1980–2015 and demonstrate that an increase in the level of globalization and financial development while improving economic performance are inimical to the sustainability of the environment. Shahbaz et al. (Citation2021) report differential effects of financial development in the G-7 countries, for example, an M-shaped relationship in the US, Canada, and Japan, while inverted M-shaped relationship in Germany, and an inverted N-shaped relationship in the UK, Italy, and France. On the other hand, Sekali & Bouzahzah (Citation2019) employ the ARDL technique to show that financial development does not have a significant effect on carbon risk in Morocco.
Many other studies do report that the inconsistencies could be attributed to the country characteristics in terms of the governance infrastructure (Adams & Klobodu, Citation2017; Adams et al., Citation2016; Gamso, Citation2018a). Good institutional infrastructure facilitates better environmental protection outcomes. In support of this view, Baloch and Wang (Citation2019) observe that the mere increase in growth in the BRICS without the appropriate improvement in the institutional infrastructure will not necessarily lead to decline in carbon risks. However, the innovation and institutional quality complementarity has not been given the desired attention in the literature especially as it pertains to the BRICS. This gap motivates the study. The methodology and data employed are discussed next.
3. Methodology and data
3.1. Theoretical model
The inspiring work of Tamazian et al. (Citation2009) has initiated a heated debate on determinants of CO2 emissions among researchers across the globe evidenced by both theoretical and empirical literature reviews of this study (Ferreira et al., Citation2020; Hillman & Baydoun, Citation2020, among others). Following Bose et al. (Citation2021), we proxy carbon dioxide emissions as carbon risk. On the one hand, environment innovation is expected to have a positive impact on the environment because it is associated with advances in technology, which reduce carbon risk. On the other hand, according to Jevons’ paradox, the effect of innovation on carbon risk is uncertain since the energy efficiency improvements do not necessarily result in carbon risk reduction as it is offset by increased energy use (Gillingham et al., Citation2016; Jevons, Citation1866; Vélez-Henao et al., Citation2019). Following Shahbaz et al. (Citation2020), we control for the effect of globalization and parameterize our empirical model as follows:
Where is carbon risk, innov is innovation, fin is financial development, and X is the set of controls such as energy consumption, foreign direct investments, trade, institutional quality, real GDP per capita, and globalization and
is the error term. EquationEquation (1)
(1)
(1) is restated in the estimable form as follows:
3.2. Empirical model
The paper investigates the determinants of territorial-based and consumption-based carbon risks while explicitly controlling for potential cross-sectional dependence to reduce estimation bias. Hence, we employ Equationequation (2)(2)
(2) in the natural logarithm form and specify standard autoregressive distributed lags (ARDL) with p and q optimal lag lengths for the dependent and independent variables in the spirit of Pesaran and Smith (Citation1995) and Pesaran et al. (Citation1999) (ARDL (p, q)) as given below:
where and
are the territorial-based and consumption-based carbon risks, respectively.
is the set of control variables and the coefficients
for country
to be estimated. The error terms are
and
. The errors can be reparametrized as (see Chudik & Pesaran, Citation2015; Pesaran, Citation2006; Chudik et al., Citation2011) in the following equations given below:
where and
are the cross-section specific error terms,
and
are the unobserved common factors and m is the number of factors. For parameter estimation to be possible, these factors must be covariance stationary and the autocovariances must also be summable. The common factors must induce cross-country dependence and estimating the parameters without explicitly controlling for the dependence may lead to spurious estimates. Specifically, these common factors could emanate from the trade integration (leading to technological transfers and financial integration, among others) among the BRICS countries. Hence, there is likelihood of cross-country (sectional) dependence among these countries, and failure to adjust the parameter estimates for this effect could lead to estimation bias. We adopt two estimators to correct for cross-sectional dependence such as cross-sectionally augmented ARDL (CS-ARDL) proposed by Chudik and Pesaran (Citation2015) and augmented mean group estimator (AMG) developed by Eberhardt and Teal (Citation2010). Firstly, we present CS-ARDL model that uses cross-sectional averages to account for cross-sectional dependence using Equationequations (5)
(5)
(5) and (Equation6
(6)
(6) ) as shown in the equation below:
where is either
or
as defined above. The terms
and
are averages of the carbon risks and the other regressors. The terms
and
are the optimal lag lengths of the lagged dependent and independent variables, respectively.
and
.Using the short-run coefficients in Equationequation (7)
(7)
(7) , long run coefficients are then indirectly estimated through an auxiliary regression as:
The error correction factor that adjusts temporary shorts to the long-run equilibrium is extracted from the equation below as in Chudik and Pesaran (Citation2015) and Chudik et al. (Citation2016):
EquationEquations (7)(7)
(7) and (Equation9
(9)
(9) ) are corrected for small sample size bias using the Chudik and Pesaran’s (Citation2015) Recursive Mean Adjustment technique. Testing for cross-country dependence, we use Pesaran (Citation2015) test of cross-sectional dependence that assumes a null hypothesis of cross-section dependence. Secondly, for robustness checks, we present the Augmented Mean Group (AMG) estimator proposed by Eberhardt and Bond (Citation2009) and Eberhardt and Teal (Citation2010) which includes a ‘common dynamic effect’ in the cross-section regressions to control for the cross-section dependence effects. The common dynamic effect is obtained from the year dummy (T-1 year dummies) parameter estimates of a pooled regression in first differences as follows:
Also, this variable is added to each of the N standard country regressions which contains linear trend terms to ‘capture omitted idiosyncratic processes which evolve in a linear fashion’ over time given by
where are the estimates of the year dummy regression coefficients and the group-specific parameter estimates are averaged across the countries as
.
3.3. Data
We use panel data of the BRICS to examine the drivers of territorial-based and consumption-based carbon risks over the periods 1984–2021 and 1990–2020, respectively.Footnote1 We employ two dependent variables namely territorial-based and consumption-based carbon risks measured in metric tons and sourced from the Carbon Dioxide Information Analysis Center (see https://globalcarbonbudget.org/carbonbudget/).Footnote2 The variables and sources of data are summarized in Table .
Table 1. Variable definition and sources
4. Empirical analysis
Section 4.1 presents the summary statistics and pairwise correlation matrix of all the variables, and principal component analysis of measures of financial development, while Section 4.2 offers panel unit root tests, cross-sectional dependence tests and all the CS-ARDL specifications, and Section 4.2 presents both the short-run and long-run relationships using the CS-ARDL estimator and the long-run coefficients of the AMG estimator for robustness checks.
4.1. Summary statistics, pairwise correlation matrix and principal component analysis
Table shows that the mean of each variable falls within the range (0.000, 1765.143), and all the values of the variables range between − 1.429 and 9838.754. The variables fdi and foss exhibit the highest and the lowest variations in their values, respectively. The terms tco2, cco2, renergy, rd, fd, and fdi are positively skewed, whilst the rest are negatively skewed. All the variables are normally distributed at 5% significance level. Except renergy, foss, rd, fdi, glob, and gstab, all the variables have negative excess kurtosis.
Table 2. Summary statistics
Table 3. Pairwise correlation matrix
Table 4. Principal component analysis of financial development
The pairwise correlation matrix is presented in Table . The regressors are not highly correlated, but territorial-based and consumption-based carbon risks are highly correlated since they are constituents of carbon risk. However, this will not pose any estimation problem because they are dependent variables in different equations.
Following Shahbaz et al. (Citation2015) and Shahbaz et al. (Citation2020), we employ principal component analysis to create a financial development index (Table ). The outcome reveals 85.68% of the variations in the dataset is accounted for by the first principal component. Therefore, the latter is employed as the composite index for financial development in the empirical analyses.
4.2. Panel unit root test, cross-country dependence test and CS-ARDL specifications
We conduct parameter estimation using CS-ARDL (refer to Chudik & Pesaran, Citation2013, Citation2015) and AMG (refer to Eberhardt & Teal, Citation2010) techniques. The stationarity of the variables is assessed using Im-Pesaran-Shin and Fisher-type tests, and the results are presented in Table . These tests confirm that all variables are either I(0) or I(1).
Table 5. Panel unit root tests
We assess the cross-country dependence by using Pesaran’s (Citation2015) CD test and the outcomes presented in Table . The results indicate significant cross-sectional dependence at the 1% significance level within the BRICS dataset, and failure to explicitly adjust for this dependence may lead to estimation bias. Additionally, CS-ARDL specifications in Table confirms the existence of long-run equilibrium relationships
Table 6. Cross-country dependence test and CS-ARDL specifications
Table 7. CS-ARDL regression estimates
4.3. Parameter estimates discussions
Panel 1 and Panel 2 of Table present the short and long-run coefficients, respectively. Each specification in Table , we present two regression estimates, one for territorial-based carbon risk and the other for consumption-based carbon risk as shown in Table . T1 refers to model one for territorial-based carbon risk, while C1 corresponds to model one for consumption-based carbon risk. Generally, the energy consumption variables (renewable energy and fossil fuel) have the desired effects on carbon risks. The outcome of both models reveals that renewable energy consumption has a negative and significant impact on both carbon risks, while fossil fuel consumption has positive and significant impact on these risks. For instance, a 1% increase in renewable energy consumption in (i) model T1 diminishes territorial carbon risk by 0.513% in short-run and 0.729 % in the long-run, and (ii) in model C1 renewable energy reduces consumption carbon risk by 0.323% in the short-term and 0.544% in the long-term. For fossil fuel consumption, a 1% increase worsens territorial carbon risk by 1.054% (short run) and 1.499% (long run), and for consumption carbon risk, it worsens by 1.054% (short run) and 1.345% (long run). These findings are consistent with the studies of Khan et al. (Citation2020) for China and Mensah et al. (Citation2019) for OECD countries, which indicate that renewable energy has a positive effect on environmental quality, while fossil fuel has adverse effects. The negative and statistically significant coefficient of the error correction terms (ECT) (see Panel 2), 0.699 for T1 and 0.690 for C1, imply that there is a long-run equilibrium relationship among the variables in both models and any deviation from the relationship arising for temporary shocks is corrected by 69.9% in T1 and 69.0% in C1.
Regarding the substantive variables, we derive models T2 and C2 by incorporating adjustments for innovation, financial development, and government stability. Financial development exhibits a positive and significant impact in both models, with a more pronounced effect observed for consumption-based carbon risk. Comparable findings are documented by Xing et al. (Citation2017) for China and Churchill et al. (Citation2018) for G7 countries. Innovation has a negative significant effect on both carbon risks in the long-run. Specifically, a 1% increase in innovation decreases territorial-based and consumption-based carbon risks by 0.712% and 0.674%, respectively in the long term. This implies that innovation in the form of R&D expenditure enhances environmental quality, which is consistent with the study of Aldieri et al. (Citation2019) for Russia, Töbelmann and Wendler (Citation2020) for 27 EU countries, and Shahbaz et al. (Citation2018) for France. It is worth mentioning that the active pollution control mechanisms are more important in reducing carbon risks than innovation per se, while others also suggest that technological innovation may be able to mitigate the per capita income reductions and not the overall effect (Smulders and De Nooij, Citation2003).
We obtain models T3 and C3 by controlling for the persistent feedback effects of carbon risk by including the second lag of the risks in models T2 and C2. Government stability is negatively significant as in the original models of T2 and C2. Baloch and Wang (Citation2019) explain that government quality which is a measure of the institutional quality facilitates sustainable environment through the enforcement of rule of law. Indeed, Nwani and Adams (Citation2021) report that institutional quality plays a major role in reducing the environmental cost of natural resources and Adams and Nsiah (Citation2019) report that democratic states with high institutional quality are less likely to pollute the environment.
Financial development has adverse effect on carbon risk in both the short and long term. Additionally, the reductive effect of financial development on consumption-based carbon risk is higher than on territorial—based carbon risk. A 1% increase in financial development will lead to an increase of 0.136% in territorial carbon risk and 0.312% in consumption carbon risk in the long run. The results of the study are consistent with those of Shahbaz et al. (Citation2020), Koondhar et al. (Citation2021), Sethi et al. (Citation2021), and Shahbaz et al. (Citation2018) but contradict Shahbaz et al. (Citation2021), and Sekali and Bouzahzah (Citation2019). Shahbaz et al. (Citation2021) explain that the inconsistencies in the results of the various studies could be explained by time varying factors such that in the same country or region, financial development could have negative and positive effect on emissions at different periods. For example, the negative impact of financial development on emissions in the 1940–1957 sub-period in Italy was a result of hydroelectric energy investments, but in the 1960s, financial development’s effect on emissions was positive because of the shift to low priced fossil fuels.
In models T4 and C4, we investigate the predictive power of FDI and trade liberalization coupled with the variables in the above models. Foreign direct investments and trade liberalization are statistically insignificant for both carbon risks in the long-run. For model 5, we explicitly control for globalization in T4 and C4 and the results show that innovation, renewable energy consumption, fossil fuel consumption, and financial development have the expected impacts on both risks. A 1% increase in globalization efforts lead to reduction in only consumption-based carbon by 0.582% and 0.462% in the short-run and long-run, respectively. The negative effect of globalization of carbon risk is consistent with the findings of Shahbaz et al. (Citation2020) for the United Arab Emirates which indicates that a 1 per cent increase in economic globalization is associated with a decline in risk of 0.54–0.56 per cent. Similarly, Shahbaz et al. (Citation2015) demonstrate that the reduction in carbon risk associated with globalization is related to the fact that the composite effect is maintained by the dominant effect of the technique effect over the scale effect. Further, we investigated the effect of real GDP per capita on both risks, and the results show that GDP has no impact on carbon risks (Refer to Table in Appendix A for the parameter estimates).
4.4. Robustness checks
Following literature of using a different estimation technique (see e.g. Samargandi, Citation2019; Atsu & Adams, Citation2021; Shahbaz et al., Citation2020) to ensure the robustness of our findings, we present the long-run coefficients using the AMG estimator in Table below. Notably, renewable energy exhibits a negative effect on territorial carbon risk in models 1–3 (TAMG1, TAMG2, and TAMG3) and consumption carbon risk in only model 1 (CAMG1). This outcome is consistent with CS-ARDL results. Fossil fuel largely increases both types of carbon risk, while financial development is negative and significant only in Model 5 for both risks, while environmental innovation (research and development expenditure) does not significantly contribute to carbon risk. On the average, institutional quality exhibits a reducing effect on the risks suggesting that the variable enhances environmental quality. FDI contributes to both types of carbon risks, while the globalization variable does not. Overall, the AMG results support the robustness of the findings observed in CS-ARDL.
Table 8. Augmented mean group model estimates
In addition to cross-sectional dependence adjustment, the CS-ARDL corrects for small sample size bias using the Recursive Mean-Adjustment technique and improves on the long-run coefficients using the short-run coefficients in an auxiliary regression. This is not the same for the AMG even though it also corrects for cross-sectional dependence.
5. Conclusion
The study investigated the impact of innovation on carbon risk (measured by carbon dioxide) while accounting for institutional quality and financial development of the BRICS countries, using AMG and CS-ARDL estimators. The results indicate that while fossil fuels negatively impact the environment, renewable energy, innovation, financial development, and institutional quality mitigate both territorial and consumption carbon risks. Particularly, innovation predominantly affects territorial carbon risk, while financial development has a more pronounced impact on consumption carbon risk. Additionally, globalization significantly influences only consumption carbon risks. These findings yield three key implications.
Investing in R&D is crucial for driving technological innovations that drive economic growth with emphasis on environmental quality. BRICS countries should strive for an innovation-driven economy to diversify energy sources, fostering a low-carbon economy through advanced technologies like carbon capture. Government-led innovation can shift the focus from manufacturing to service-oriented activities, promoting green growth. This aligns with the idea that technological progress reduces carbon risk, while growth through capital accumulation has the opposite effect. The dominance of innovation on territorial carbon risk suggests enhanced productive efficiency, while the influence of financial development and globalization on consumption-based carbon risk implies a potential association with environmental degradation as BRICS integrates into the global economy. Second, the increasing economic power of the BRICs, large population and supplier of nearly 40% of global primary energy offers them an opportunity to lead in the global energy transition agenda. Obviously, this bestows benefits on the BRICS as a group and as individual countries as they could help to lower the cost of clean energy deployment and other externalities in terms of overcoming the constraints of energy poverty, enhance energy security and more importantly environmental quality. Another peripheral benefit is the promotion of employment, particularly in green jobs. The IRENA (Citation2020) report indicates that energy transformation would boost GDP by as much as 2.5% and a total employment of 0.2% by 2050. This suggests that the BRICS should work on increasing the share of clean energy in its energy mix to improve energy efficiency. In this light, China’s commitment to the renewables agenda should be an example for the other countries in reducing environmental pollution. It is worthy of mention that China has been the biggest investor in clean energy over the last decade and currently the largest producer of solar panels and wind turbines.
Third, the adverse effect of institutional quality on carbon risk is supportive of the need for tough and legally binding rules at the national and international levels. This in a way also calls for action in the energy sector for strong environmental policy to promote and exchange local solutions and technologies for environmentally sustainable development. Indeed, the literature supports the view that economic instability and poor environmental quality in many countries could be attributed to weak governance infrastructure. The way forward for the BRICS governments is to enact and implement stern regulations as the situation demands and impose carbon taxes where necessary to mitigate carbon risk. This is in sync with the view that whether or not environmental quality is improved is driven mainly by government policies, which determine the functioning of markets and shape overall economic activity. In this light, it is worth mentioning that stable energy policies have helped Brazil in becoming the leading producer of biofuels and the energy generation from wind and solar through subsidies. Overall, strong government policies should focus on decarbonizing the economy and more importantly provide the requisite political environment conducive for clean energy development that is not only affordable but also accessible. Overall, the results of the study show that though the institutional quality is necessary for the BRICS countries, it is not enough and that it has to be complemented with investments in R & D, clean energy policies, and enhance its financial infrastructure to achieve its emissions reduction target.
In conclusion, the BRICS possess the potential to reshape the global agenda for clean energy development. This study is limited to the BRICS, and future research should explore country-specific contexts for a deeper understanding of carbon risk dynamics and a defined climate change mitigation strategy. Additionally, future research should utilize more specific environmental innovations data to reduce estimation bias.
Disclosure statement
No potential conflict of interest was reported by the author(s).
Data availability statement
The data that support the findings of this study are available from the corresponding author, F. A, upon reasonable request.
Notes
1. The difference in the periods is due to availability of data for the consumption-based carbon risk.
2. Consumption-based carbon risks reflect the consumption and lifestyle choices of a country’s citizens, while territorial-based carbon risks are those emitted within a country’s borders (Ritchie & Roser, Citation2017)
References
- Adams, S., Adom, P. K., & Klobodu, E. K. M. (2016). Urbanization, regime type and durability, and environmental degradation in Ghana. Environmental Science and Pollution Research, 23(23), 23825–16. https://doi.org/10.1007/s11356-016-7513-4
- Adams, S., & Klobodu, E. K. M. (2017). Urbanization, democracy, bureaucratic quality, and environmental degradation. Journal of Policy Modeling, 39(6), 1035–1051. https://doi.org/10.1016/j.jpolmod.2017.04.006
- Adams, S., & Nsiah, C. (2019). Reducing carbon dioxide emissions; does renewable energy matter?. Science of the Total Environment, 693, 133288.
- Adams, S., & Opoku, E. E. O. (2020). Trade and environmental pollution in Africa: Accounting for consumption and territorial-based emissions. Environmental Science and Pollution Research, 27, 44230–44239.
- Adams, S., Opoku, E. E. O., & Aluko, O. A. (2021). The foreign direct investment-environment nexus: Does emission disaggregation matter?. Energy Reports, 7, 778–787.
- Adedoyin, F. F., Bekun, F. V., Eluwole, K. K., & Adams, S. (2022). Modelling the nexus between financial development, FDI, and CO2 emission: Does institutional quality matter? Energies, 15(20), 7464. https://doi.org/10.3390/en15207464
- Aldieri, L., Kotsemir, M., & Vinci, C. P. (2019). Environmental innovations and productivity: Empirical evidence from Russian regions. Resources Policy, 74, 101444. https://doi.org/10.1016/j.resourpol.2019.101444
- Ali, W., Abdullah, A., & Azam, M. (2016). The dynamic linkage between technological innovation and carbon dioxide emissions in Malaysia: An autoregressive distributed lagged bound approach. International Journal of Energy Economics & Policy, 6(3), 389–400.
- Atsu, F., & Adams, S. (2021). Energy consumption, finance, and climate change: Does policy uncertainty matter? Economic Analysis and Policy, 70, 490–501. https://doi.org/10.1016/j.eap.2021.03.013
- Baloch, M. A., & Wang, B. (2019). Analyzing the role of governance in CO2 emissions mitigation: The BRICS experience. Structural Change and Economic Dynamics, 51, 119–125. https://doi.org/10.1016/j.strueco.2019.08.007
- Bose, S., Minnick, K., & Shams, S. (2021). Does carbon risk matter for corporate acquisition decisions? Journal of Corporate Finance, 70, 102058. https://doi.org/10.1016/j.jcorpfin.2021.102058
- BP Statistical Review of World Energy. (2019).
- Brookes, L. (1990). The greenhouse effect: The fallacies in the energy efficiency solution. Energy Policy, 18(2), 199–201. https://doi.org/10.1016/0301-4215(90)90145-T
- Cadez, S., & Czerny, A. (2016). Climate change mitigation strategies in carbon-intensive firms. Journal of Cleaner Production, 112, 4132–4143. https://doi.org/10.1016/j.jclepro.2015.07.099
- Cadez, S., & Guilding, C. (2017). Examining distinct carbon cost structures and climate change abatement strategies in CO2 polluting firms. Accounting Auditing & Accountability Journal, 30(5), 1041–1064. https://doi.org/10.1108/AAAJ-03-2015-2009
- Cansino, J. M., Ordóñez, M., & Prieto, M. (2022). Decomposition and measurement of the rebound effect: The case of energy efficiency improvements in Spain. Applied Energy, 306, 117961. https://doi.org/10.1016/j.apenergy.2021.117961
- Castaldi, C. (2018). To trademark or not to trademark: The case of the creative and cultural industries. Research Policy, 47(3), 606–616.
- Cheng, C., Ren, X., & Wang, Z. (2019). The impact of renewable energy and innovation on carbon emission: An empirical analysis for OECD countries. Energy Procedia, 158, 3506–3512. https://doi.org/10.1016/j.egypro.2019.01.919
- Cheng, C., Ren, X., Wang, Z., & Yan, C. (2019). Heterogeneous impacts of renewable energy and environmental patents on CO2 emission-evidence from the BRIICS. Science of the Total Environment, 668, 1328–1338. https://doi.org/10.1016/j.scitotenv.2019.02.063
- Chen, H., Yi, J., Chen, A., Peng, D., & Yang, J. (2023). Green technology innovation and CO2 emission in China: Evidence from a spatial-temporal analysis and a nonlinear spatial durbin model. Energy Policy, 172, 113338. https://doi.org/10.1016/j.enpol.2022.113338
- Chudik, A., Mohaddes, K., Pesaran, M. H., & Raissi, M. (2016). Long-run effects in large heterogeneous panel data models with cross-sectionally correlated errors essays in honor of Aman Ullah. 85–135.
- Chudik, A., & Pesaran, M. H. (2013). Large panel data models with cross-sectional dependence: A survey. CAFE Research Paper, 13–15.
- Chudik, A., & Pesaran, M. H. (2015). Common correlated effects estimation of heterogeneous dynamic panel data models with weakly exogenous regressors. Journal of Econometrics, 188(2), 393–420. https://doi.org/10.1016/j.jeconom.2015.03.007
- Chudik, A., Pesaran, M. H., & Tosetti, E. (2011). Weak and strong cross‐section dependence and estimation of large panels.
- Churchill, S. A., Inekwe, J., Ivanovski, K., & Smyth, R. (2018). The environmental kuznets curve in the OECD: 1870–2014. Energy Economics, 75, 389–399. https://doi.org/10.1016/j.eneco.2018.09.004
- Dauda, L., Long, X., Mensah, C. N., & Salman, M. (2019). The effects of economic growth and innovation on CO 2 emissions in different regions. Environmental Science and Pollution Research, 26(15), 15028–15038. https://doi.org/10.1007/s11356-019-04891-y
- Dinda, S. (2018). Production technology and carbon emission: Long‐run relation with short‐run dynamics. Journal of Applied Economics, 21(1), 106–121. https://doi.org/10.1080/15140326.2018.1526871
- Downie, C., & Williams, M. (2018). After the Paris agreement: What role for the BRICS in Global climate governance? Global Policy, 9(3), 398–407. https://doi.org/10.1111/1758-5899.12550
- Du, K., Li, P., & Yan, Z. (2019). Do green technology innovations contribute to carbon dioxide emission reduction? Empirical evidence from patent data. Technological Forecasting and Social Change, 146, 297–303. https://doi.org/10.1016/j.techfore.2019.06.010
- Dutta, S., Escalona, R., Garanasvili, A., Vincent, S., León, L., Hardman, C., & Guadagno, F. (2019). Creating healthy lives–The future of medical innovation. Global innovation index, 41–58.
- Eberhardt, M., & Bond, S. (2009). Cross-section dependence in nonstationary panel models: A novel estimator.
- Eberhardt, M., & Teal, F. (2010). Productivity analysis in global manufacturing production. Discussion Paper 515, Department of Economics, University of Oxford. http://www.economics.ox.ac.uk/research/WP/pdf/paper515.pdf
- Ferreira, J. J., Fernandes, C. I., & Ferreira, F. A. (2020). Technology transfer, climate change mitigation, and environmental patent impact on sustainability and economic growth: A comparison of European countries. Technological Forecasting and Social Change, 150, 119770. https://doi.org/10.1016/j.techfore.2019.119770
- Gamso, J. (2018a). Environmental policy impacts of trade with China and the moderating effect of governance. Environmental Policy & Governance, 28(6), 395–405. https://doi.org/10.1002/eet.1807
- Garrone, P., & Grilli, L. (2010). Is there a relationship between public expenditures in energy R&D and carbon emissions per GDP? An empirical investigation. Energy Policy, 38(10), 5600–5613.
- Georgatzi, V. V., Stamboulis, Y., & Vetsikas, A. (2020). Examining the determinants of CO2 emissions caused by the transport sector: Empirical evidence from 12 European countries. Economic Analysis & Policy, 65, 11–20.
- Gillingham, K., Rapson, D., & Wagner, G. (2016). The rebound effect and energy efficiency policy. Review of Environmental Economics and Policy, 10(1), 68–88. https://doi.org/10.1093/reep/rev017
- The Global Energy and CO2 Status Report. (2019). Latest trends in energy and emissions in 2018. IEA.
- Global Innovation Index. (2018). Energizing the world with innovation. World Intellectual Property Organization.
- Global Innovation Index. (2019). Creating healthy lives–The future of medical innovation. World Intellectual Property Organization.
- Gygli, S., Haelg, F., Potrafke, N., & Sturm, J. E. (2019). The KOF globalisation index–revisited. The Review of International Organizations, 14(3), 543–574. https://doi.org/10.1007/s11558-019-09344-2
- Hasanov, F. J., Liddle, B., & Mikayilov, J. I. (2018). The impact of international trade on CO2 emissions in oil exporting countries: Territory vs consumption emissions accounting. Energy Economics, 74, 343–350. https://doi.org/10.1016/j.eneco.2018.06.004
- Haseeb, M., Hassan, S., Azam, M., & Suryanto, T. (2018). The dynamics of governance, tourism and environmental degradation: The world evidence. International Journal of Global Environmental Issues, 17(4), 340–363. https://doi.org/10.1504/IJGENVI.2018.095155
- Hillman, J. R., & Baydoun, E. (2020). An overview of innovation and entrepreneurship to address climate change. Higher Education in the Arab World: Building a Culture of Innovation and Entrepreneurship, 141–181.
- ICRG. (2020). International country risk Guide (ICRG).
- IRENA. (2020). Renewable energy statistics 2020. The International Renewable Energy Agency.
- Jevons, W. S. (1866). The coal question: An inquiry concerning the progress of the nation, and the probable exhaustion of our coal-mines. Macmillan.
- Jiang, Q., & Khattak, S. I. (2023). Modeling the impact of innovation in marine energy generation-related technologies on carbon dioxide emissions in South Korea. Journal of Environmental Management, 326, 116818.
- Jiang, P., Li, M., Zhao, Y., Gong, X., Jin, R., Zhang, Y., & Liu, L. (2022). Does Environmental Regulation Improve Carbon Emission Efficiency? Inspection of panel data from inter-provincial provinces in China. Sustainability, 14(16), 10448.
- Khalfaoui, R., Tiwari, A. K., Khalid, U., & Shahbaz, M. (2023). Nexus between carbon dioxide emissions and economic growth in G7 countries: Fresh insights via wavelet coherence analysis. Journal of Environmental Planning and Management, 66(1), 31–66. https://doi.org/10.1080/09640568.2021.1978062
- Khan, Z., Ali, M., Kirikkaleli, D., Wahab, S., & Jiao, Z. (2020). The impact of technological innovation and public‐private partnership investment on sustainable environment in China: Consumption‐based carbon emissions analysis. Sustainable Development, 28(5), 1317–1330. https://doi.org/10.1002/sd.2086
- Khan, H., Weili, L., & Khan, I. (2022). Institutional quality, financial development and the influence of environmental factors on carbon emissions: Evidence from a global perspective. Environmental Science and Pollution Research, 29(9), 13356–13368. https://doi.org/10.1007/s11356-021-16626-z
- Khazzoom, J. D. (1980). Economic implications of mandated efficiency in standards for household appliances. The Energy Journal, 1(4), 21–40. https://doi.org/10.5547/ISSN0195-6574-EJ-Vol1-No4-2
- Kong, L., Mu, X., Hu, G., & Tu, C. (2023). Will energy efficiency improvements reduce energy consumption? Perspective of rebound effect and evidence from Beijing. Energy, 263, 125665. https://doi.org/10.1016/j.energy.2022.125665
- Koondhar, M. A., Shahbaz, M., Ozturk, I., Randhawa, A. A., & Kong, R. (2021). Revisiting the relationship between carbon emission, renewable energy consumption, forestry, and agricultural financial development for China. Environmental Science and Pollution Research, 28(33), 45459–45473. https://doi.org/10.1007/s11356-021-13606-1
- Liguo, X., Ahmad, M., & Khattak, S. I. (2022). Impact of innovation in marine energy generation, distribution, or transmission-related technologies on carbon dioxide emissions in the United States. Renewable and Sustainable Energy Reviews, 159, 112225. https://doi.org/10.1016/j.rser.2022.112225
- Liu, X. (2020). Upcoming 14th five –year plan policy package attracts wide attention. Global Times Published, 23(38), 40.
- Mensah, C. N., Long, X., Boamah, K. B., Bediako, I. A., Dauda, L., & Salman, M. (2018). The effect of innovation on CO 2 emissions of OCED countries from 1990 to 2014. Environmental Science and Pollution Research, 25(29), 29678–29698. https://doi.org/10.1007/s11356-018-2968-0
- Mensah, C. N., Long, X., Dauda, L., Boamah, K. B., & Salman, M. (2019). Innovation and CO 2 emissions: The complimentary role of eco-patent and trademark in the OECD economies. Environmental Science and Pollution Research, 26(22), 22878–22891. https://doi.org/10.1007/s11356-019-05558-4
- Mensah, C. N., Long, X., Dauda, L., Boamah, K. B., Salman, M., Appiah-Twum, F., & Tachie, A. K. (2019). Technological innovation and green growth in the organization for economic cooperation and development economies. Journal of Cleaner Production, 240, 118204. https://doi.org/10.1016/j.jclepro.2019.118204
- Monem, M. A. A., & El Ghandour, I. A. (2020). Role of Science, technology and innovation in addressing climate change challenges in Egypt. In E. E. Omran, & A. M. Negm (Eds.), Climate change impacts on agriculture and food security in Egypt (pp. 59–79). Springer.
- Mu, R. (2018). Bounded rationality in the developmental trajectory of environmental target policy in China, 1972–2016. Sustainability, 10(1), 199. https://doi.org/10.3390/su10010199
- Nwani, C., & Adams, S. (2021). Environmental cost of natural resource rents based on production and consumption inventories of carbon emissions: Assessing the role of institutional quality. Resources Policy, 74, 102282.
- Omoke, P. C., Nwani, C., & Effiong, E. L. (2023) Globalization and its environmental effects: Assessing the role of de facto and de jure conditions in trade, financial and information (ICT) developments in West Africa, climate and development. https://doi.org/10.1080/17565529.2022.2154123
- Patterson, J. J., & Huitema, D. (2019). Institutional innovation in urban governance: The case of climate change adaptation. Journal of Environmental Planning and Management, 62(3), 374–398. https://doi.org/10.1080/09640568.2018.1510767
- Pesaran, M. H. (2006). Estimation and inference in large heterogeneous panels with a multifactor error structure. Econometrica, 74(4), 967–1012. https://doi.org/10.1111/j.1468-0262.2006.00692.x
- Pesaran, M. H. (2015). Time series and panel data econometrics. Oxford University Press.
- Pesaran, M. H., Shin, Y., & Smith, R. P. (1999). Pooled mean group estimation of dynamic heterogeneous panels. Journal of the American Statistical Association, 94(446), 621–634. https://doi.org/10.1080/01621459.1999.10474156
- Pesaran, M. H., & Smith, R. (1995). Estimating long-run relationship from dynamic heterogeneous panels. Journal of Economics, 68(1), 79–113.
- Rahman, Z. U., Cai, H., Khattak, S. I., & Hasan, M. M. (2019). Energy production-income-carbon emissions nexus in the perspective of NAFTA and BRIC nations: A dynamic panel data approach. Economic Research-Ekonomska Istraživanja, 32(1), 3378–3391. https://doi.org/10.1080/1331677X.2019.1660201
- Ritchie, H., & Roser, M. (2017). CO₂ and greenhouse gas emissions. Our World in Data.
- Root, L., Van Der Krabben, E., & Spit, T. (2015). Bridging the financial gap in climate adaptation: Dutch planning and land development through a new institutional lens. Journal of Environmental Planning and Management, 58(4), 701–718. https://doi.org/10.1080/09640568.2014.885412
- Sadorsky, P. (2010). The impact of financial development on energy consumption in emerging economies. Energy Policy, 38(5), 2528–2535. https://doi.org/10.1016/j.enpol.2009.12.048
- Samargandi, N. (2019). Energy intensity and its determinants in OPEC countries. Energy, 186, 115803.
- Sarkodie, S. A., & Adams, S. (2018). Renewable energy, nuclear energy, and environmental pollution: Accounting for political institutional quality in South Africa. Science of the Total Environment, 643, 1590–1601. https://doi.org/10.1016/j.scitotenv.2018.06.320
- Schumpeter, J. A. (1934). The theory of economic development. Harvard University Press.
- Sekali, J., & Bouzahzah, M. (2019). Financial development and environmental quality: Empirical evidence for Morocco. International Journal of Energy Economics & Policy, 9(2), 67.
- Sethi, P., Bhattacharjee, S., Chakrabarti, D., & Tiwari, C. (2021). The impact of globalization and financial development on India’s income inequality. Journal of Policy Modeling, 43(3), 639–656.
- Shahbaz, M., Destek, M. A., Dong, K., & Jiao, Z. (2021). Time-varying impact of financial development on carbon emissions in G-7 countries: Evidence from the long history. Technology Forecasting and Social Change, 171, 1–10. https://doi.org/10.1016/j.techfore.2021.120966
- Shahbaz, M., Haouas, I., Sohag, K., & Ozturk, I. (2020). The financial development-environmental degradation nexus in the United Arab Emirates: The importance of growth, globalization and structural breaks. Environmental Science and Pollution Research, 27(10), 10685–10699. https://doi.org/10.1007/s11356-019-07085-8
- Shahbaz, M., Mallick, H., Mahalik, M. K., & Loganathan, M. N. (2015). Does globalization impede environmental quality in India? Ecological Indicators, 52, 379–393. https://doi.org/10.1016/j.ecolind.2014.12.025
- Shahbaz, M., Nasir, M. A., & Roubaud, D. (2018). Environmental degradation in France: The effects of FDI, financial development, and energy innovations. Energy Economics, 74, 843–857. https://doi.org/10.1016/j.eneco.2018.07.020
- Shah, S. Z., Chughtai, S., & Simonetti, B. (2020). Renewable energy, institutional stability, environment and economic growth nexus of D-8 countries. Energy Strategy Reviews, 29, 100484. https://doi.org/10.1016/j.esr.2020.100484
- Shao, S., Guo, L., Yu, M., Yang, L., & Guan, D. (2019). Does the rebound effect matter in energy import-dependent mega-cities? Evidence from Shanghai (China). Applied Energy, 241, 212–228. https://doi.org/10.1016/j.apenergy.2019.03.007
- Sheng, P., & Lu, D. (2016). Low-carbon development and carbon reduction in China. Climate and Development, 8(5), 472–479. https://doi.org/10.1080/17565529.2015.1064812
- Shi, Q., Liang, Q., Wang, J., Huo, T., Gao, J., You, K., & Cai, W. (2023). Dynamic scenario simulations of phased carbon peaking in China's building sector through 2030–2050. Sustainable Production and Consumption, 35, 724–734.
- Sinha, A., Sengupta, T., & Alvarado, R. (2020). Interplay between technological innovation and environmental quality: Formulating the SDG policies for next 11 economies. Journal of Cleaner Production, 242, 118549. https://doi.org/10.1016/j.jclepro.2019.118549
- Smulders, S., & De Nooij, M. (2003). The impact of energy conservation on technology and economic growth. Resource and Energy Economics, 25(1), 59–79.
- Tamazian, A., Chousa, J. P., & Vadlamannati, K. C. (2009). Does higher economic and financial development lead to environmental degradation: Evidence from BRIC countries. Energy Policy, 37, 246–253. https://doi.org/10.1016/j.enpol.2008.08.025
- Temesgen Hordofa, T., Minh Vu, H., Maneengam, A., Mughal, N., The Cong, P., & Liying, S. (2023). Does eco-innovation and green investment limit the CO2 emissions in China? Economic Research-Ekonomska Istraživanja, 36(1), 634–649. https://doi.org/10.1080/1331677X.2022.2116067
- Töbelmann, D., & Wendler, T. (2020). The impact of environmental innovation on carbon dioxide emissions. Journal of Cleaner Production, 244, 118787. https://doi.org/10.1016/j.jclepro.2019.118787
- Ulucak, R., & Erdogan, S. (2022). The effect of nuclear energy on the environment in the context of globalization: Consumption vs production-based CO2 emissions. Nuclear Engineering and Technology, 54(4), 1312–1320. https://doi.org/10.1016/j.net.2021.10.030
- Usman, M., Balsalobre-Lorente, D., Jahanger, A., & Ahmad, P. (2023). Are Mercosur economies going green or going away? An empirical investigation of the association between technological innovations, energy use, natural resources and GHG emissions. Gondwana Research, 113, 53–70. https://doi.org/10.1016/j.gr.2022.10.018
- Vélez-Henao, J. A., Vivanco, D. F., & Hernández-Riveros, J. A. (2019). Technological change and the rebound effect in the STIRPAT model: A critical view. Energy Policy, 129, 1372–1381. https://doi.org/10.1016/j.enpol.2019.03.044
- Wang, S., Li, Q., Fang, C., & Zhou, C. (2016). The relationship between economic growth, energy consumption, and CO2 emissions: Empirical evidence from China. Science of the Total Environment, 542, 360–371.
- Wang, Z., Yang, Z., Zhang, Y., & Yin, J. (2012). Energy technology patents–CO2 emissions nexus: An empirical analysis from China. Energy Policy, 42, 248–260. https://doi.org/10.1016/j.enpol.2011.11.082
- Weina, D., Gilli, M., Mazzanti, M., & Nicolli, F. (2016). Green inventions and greenhouse gas emission dynamics: A close examination of provincial Italian data. Environmental Economics and Policy Studies, 18, 247–263.
- Wen, Q., Chen, Y., Hong, J., Chen, Y., Ni, D., & Shen, Q. (2020). Spillover effect of technological innovation on CO2 emissions in China’s construction industry. Building and Environment, 171, 106653. https://doi.org/10.1016/j.buildenv.2020.106653
- World Bank. (2018). World Development Indicators.
- World Intellectual Property Organization (WIPO). (2022). Global innovation Index 2022: What is the future of innovation-driven growth?. WIPO. https://doi.org/10.34667/tind.46596
- Xing, T., Jiang, Q., & Ma, X. (2017). To facilitate or curb? The role of financial development in China’s carbon emissions reduction process: A novel approach. International Journal of Environmental Research and Public Health, 14(10), 1222. https://doi.org/10.3390/ijerph14101222
- Yang, X., & Li, C. (2019). Industrial environmental efficiency, foreign direct investment and export-evidence from 30 provinces in China. Journal of Cleaner Production, 212, 1490–1498. https://doi.org/10.1016/j.jclepro.2018.12.156
- York, R., Adua, L., & Clark, B. (2022). The rebound effect and the challenge of moving beyond fossil fuels: A review of empirical and theoretical research. Wiley Interdisciplinary Reviews: Climate Change, 13(4), e782. https://doi.org/10.1002/wcc.782
- Zhang, D., Ozturk, I., & Ullah, S. (2022). Institutional factors-environmental quality nexus in BRICS: A strategic pillar of governmental performance. Economic Research-Ekonomska Istraživanja, 35(1), 5777–5789. https://doi.org/10.1080/1331677X.2022.2037446
- Zhao, J., Shahbaz, M., Dong, X., & Dong, K. (2021). How does financial risk affect global CO2 emissions? The role of technological innovation. Technological Forecasting and Social Change, 168, 120751. https://doi.org/10.1016/j.techfore.2021.120751
- Zhou, K., Yang, J., Yang, T., & Ding, T. (2023). Spatial and temporal evolution characteristics and spillover effects of China’s regional carbon emissions. Journal of Environmental Management, 325, 116423. https://doi.org/10.1016/j.jenvman.2022.116423
Appendix. A
Parameter estimates
Table A1. Short-run parameter estimates.
Table A2. Long-run parameter estimates.