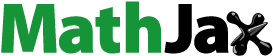
ABSTRACT
Malaria is a mosquito-borne disease. It is a life-threatening disease primarily found in tropical countries, like Ethiopia. The objective of this study was to assess the spatial distributions of malaria risk using geospatial techniques in Wabi Shebele River Sub-basin, Southeastern Ethiopia. Eleven factors, land use and land cover, topographic wetness index, slope, elevation, soil types, proximity to rivers, rainfall, population density, proximity to main roads, proximity to health services, and surface temperature were considered to produce a malaria risk map. GIS-based multi-criteria evaluation (MCE) technique was applied using weighted overlay analysis and produced three map layer factors—hazard, vulnerability, and element at risk maps. Malaria risk map results show that an area of 1867.8 km2 (33.4%), 2112.7 km2 (37.7%), and 1181.6 km2 (21.1%) were categorized under high, moderate, and low malaria risk levels, respectively. Results reveal that a large portion of the study area is classified under high and moderate spatial malaria risk levels. This indicates that communities living in the study area are prone to the malaria disease. Therefore, there is a critical need to progressively reduce malaria disease distribution through healthcare implementation in prioritized areas. This study is helpful for further study in combating malaria disease distribution and increases the efficiency of expectation efforts by skillfully targeting high and moderate-risk areas.
1. Introduction
Malaria is a serious health problem world-wide. Around 3.3 billion population is prone to malaria infection (World Health Organization [WHO], Citation2012). Sub-Saharan African countries are the most victims to malaria. It is an environmental based disease. It requires specific habitats like humidity for survival and surface water for reproduction (Chakarova et al., Citation2015; Felix, Citation2003; Sujoy & Tingneyuc, Citation2016).
Distribution of malaria in Ethiopia varies from place to place, and from time to time. A large portion of Ethiopia’s terrain is situated below 2000 meters, which is the most suitable altitude for the spread of malaria. Because of its impact on temperature, altitude greatly influences how malaria is distributed and spreads (Betemariam, Citation2002). This is the reason that over 54 million people who reside in these regions are susceptible to malaria (Mulugojjam et al., Citation2015). In addition to altitude, climatic modification and ecology are also the most principal reason for the increase of malaria in Ethiopia (Gebre et al., Citation2020).
In Ethiopia, malaria is a severe health issue and more prevalent in the months of August, September, October and November (Negassi, Citation2008; Olana et al., Citation2011). It is therefore a significant health concern in Ethiopia (FMoH, Citation2009). Malaria breeding and its transmission are highly associated with physical factors like altitude, accessibility to rivers, lake, swamps, temperature and rainfall (Ofgeha, Citation2023).
Several studies have shown that geospatial technologies can be used to analyze the specific factors that contribute to the heterogeneities in the distribution of mosquito-borne diseases. Among these geospatial technology, geographic information system (GIS) is one the most powerful tool for studying spatial distribution of malaria diseases (Gebre et al., Citation2020). It offers a wide range of possible uses in the research of mosquitoes and the diseases that they carry. GIS is therefore, a suitable tools to map and analyze the spatial distribution of mosquitoes and to assess the ecological factors that contribute to the diseases’ distribution (Hasyim et al., Citation2018; Workineh et al., Citation2019).
Previously, studies conducted by Betemariam (Citation2002); Olana et al. (Citation2011); Ahmed (Citation2014); Mulugojjam et al. (Citation2015) revealed that GIS and remote sensing play a pivotal role in mapping malaria risk prone areas. Recent study by Ofgeha (Citation2023) also reveals that malaria breeding and its transmission is associated with physical factors and can easily mapped using GIS and remote sensing techniques. Despite these efforts, in the Wabi Shebele River Sub-basin, there is a shortage of evidence regarding the spatial distribution of malaria risk. Apart from the extensive research carried out in specific regions of Ethiopia, the noteworthy impact of land surface temperature (LST) and topographic wetness index (TWI) on the spatial distribution of malaria remain underutilized.
Therefore, this study is aimed to assess and map malaria risk prone areas by integrating LST and TWI with other physical factors like land use and land cover, slope, elevation, soil types, proximity to rivers, rainfall, human population density, proximity to main roads and proximity to health services according to their risk level using geospatial techniques. The findings of this research are essential for setting priorities for interventions in medical facilities and for managing and tracking diseases. It could also enhance the efficiency of anticipation efforts; and will considerably reduce cost of prevention by proficiently targeting on high and moderate risk areas.
2. Materials and methods
2.1. Study area descriptions
Wabi Shebele River sub-basin is located in West Hararghe Zone of Oromia Regional State, eastern Ethiopia. Geographically, it is situated between 8°0′0′′ to 8°50′0′′ N latitude and 40°20′0′′ to 41°10′0′′ E longitude. The study area covers about 5596.34 km2 with an elevation ranging from 703 to 2988 meter above mean sea level (Figure ).
This study site was selected with strong assumption that the area (Wabi Shebele River Sub-basin) is one of the malaria hotspot areas due to its physical features. Wabi Shebe River Sub-basin encompasses six districts of West Hararghe zone. Namely, Habro, Daro Lebu, Boke, Oda Bultum, Hawi Gudina, Burka Dintu. The physical setting of the study area by itself facilitates the malaria parasite breeding with a dominant altitude of less than 2000 m ranging from 703 to 2988 meter above mean sea level.
2.1.1. Data types and sources
To achieve the stated objective, different data were collected from different sources. The main dataset for this study are landsat 8 OLI/TIRS (operational land imagery/thermal Infrared remote sensor) of the year 2023, shuttle radar topographic mission (SRTM 30 m resolution), soil type, climate data (temperature and rainfall) were used (Table ). These data were acquired from NASA website (https://earthexplorer.usgs.gov), open topography, Ethiopian ministry of agriculture, Ethiopian national metrological agency, respectively
Table 1. Data types and sources
2.1.2. Software used
Different types of software such as ArcGIS 10.5, IDRISI Selva 17, ERDAS IMAGINE 2015, Google Earth and Handheld GPS (global positioning system) were used to generate malaria risk area map. Specifically, ArcGIS 10.5 was used to visualize spatial distribution map of malaria risk area. IDRISI Selva 17 used for pairwise comparisons of all parameters. ERDAS IMAGINE 2015 was used for LULC types classification and accuracy assessment and Google Earth and Handheld GPS were used for ground truth to calculate accuracy assessment of LULC types.
2.1.3. Data analysis
To produce malaria risk map of the study area, identification and selection of the main parameters for malaria breeding such as LULC, topographic wetness index, slope, elevation (altitude), soil types, distance from rivers, rainfall and surface temperature factors were developed.
2.2. Factors for malaria risk area detection
To produce a malaria risk map, risk computational model was developed (EquationEquation 1(1)
(1) ).
Hazard is the probability of occurrence of a potential damaging natural phenomenon within a specified period and a given area. Element at risk (E) includes the population, public services, utilities etc., in a given area. Vulnerability (V) is the exposure of a given element at risk resulting from the occurrence of a damaging phenomenon of a given magnitude. Risk (R) is the expected degree of loss due to particular natural phenomenon. It may be expressed as the product of hazard (H), vulnerability (V) and element at risk (E).
2.2.1. Land use and land cover (LULC)
During the process of LULC classification, image pre-processing like image resampling and false color combination were computed in ERDAS IMAGINE V15 software. Supervised classification approach was applied to classify the images with a maximum likelihood classifier algorithm (MLC). MLC is one of the eminent parametric classifiers used for supervised image classification (Foody et al., Citation1992). The maximum likelihood classifier algorithm was used for LULC analysis. The LULC classes were grouped into five and labeled as farmland, forest, grassland, settlement and shrub land. The analysis of LULC classification and accuracy assessment was also processed in ERDAS Imagine 2015.
2.2.2. Accuracy assessments
To validate the result with ground truth, field data were collected using handheld global positioning system (GPS) and finally LULC classes were identified and mapped for further analysis.
Accuracy assessment was also made to evaluate the quality of classification output in this study. Error matrix based on assessment of the overall accuracy, and kappa coefficient was utilized. The main goal of accuracy assessment is to quantitatively assess how efficiently the pixels were tested into the correct land cover classes (Bhatta, Citation2008; Moisa & Gemeda, Citation2021; Negash et al., Citation2021).
The image classification accuracy is further assessed by calculating Kappa coefficient ‘k’. Kappa analysis generates a kappa coefficient or Khat statistics, the values of which range between 0 and 1.
Kappa coefficient (Khat) is a measure of the agreement between two maps staking into account all elements of error matrix (EquationEquation 3(3)
(3) ).
Where: OAC is over all accuracy, Khat is Kappa statistics, N is total number of samples, Xij is the diagonal values, Obs is accuracy reported in error matrix (overall accuracy) and Exp represents correct classification.
Land use and land cover is taken as an element at risk that affect malaria incidence. Land use has a big influence on Anopheles mosquito life cycle. It increases anopheles survival rate and shorten the population. Irrigated farming has positive effect on the survivorship and on the adult breeding (Munga et al.). In this study, LULC was classified into five categories and labeled as settlement area, farmland, grass land, shrub land and forest. Based on the value given by different scholars (Ahmed, Citation2014; Manoharan et al., Citation2020; Mulugojjam et al., Citation2015; Ofgeha, Citation2023) settlement areas and farmland are assigned as very high and high malaria risk areas respectively. The remaining classes, grass land shrub land and forest were assigned as moderate, low and very low malaria risk areas respectively.
2.2.3. Topographic wetness index (TWI)
TWI was calculated using topographic data from the shuttle radar topographic mission (SRTM), which was downloaded from the USGS website. TWI is a steady state wetness index which is commonly used to quantify topographic control on hydrological process. It is based on distribution of moisture across variable topography and is most relevant when conditions of infiltration rate exceed storage capacity (Wilson, Citation2018). Wetness index can affect the availability of mosquito in a given area. As the wetness of land increases, water holding capacity of the land increases, and that can create conducive environment for mosquito breeding. TWI gives an inexpensive quick view of potential moisture patterns where other data may not be available (Riihimäki et al., Citation2021). The TWI is mathematically expressed in the following formula (EquationEquation 4(4)
(4) ).
Where; A is the specific catchment area of a portion of land, and tanβ is the slope angle. It is estimated by dividing specific catchment areas by contour length. Slope indicates the effect of gravitational forces on water movement. It represents the tendency of land to shed water to lower adjoining areas. The catchment area represents the size of the area upstream per unit contour length (Gruber & Peckham, Citation2009; Wilson, Citation2018).
The wetness index was generated from digital elevation model (DEM) of 30 m spatial resolution. Considering the minimum and maximum index value, the raster was reclassified using natural break standards in ArcGIS 10.8 software into five sub-groups. Raster layer were ranked according to the wetness index value, which means areas of less wetness. In this re-class, low wetness index value were considered as less malaria risk level and high wetness index value are considered as high malaria risk level (Rohan, Citation2012).
2.2.4. Slope
Slope is one of the most important factors that may be associated with mosquito larval habitat formation and generated from digital elevation of 30 m spatial resolution. Slope raster layer was reclassified and ranked accordingly to the degree of suitability for malaria incidence. The steeper slope values are related to lesser malaria hazard and the gentler slope have high susceptibility for malaria incidence (Ahmed, Citation2014). Water appears to be logged in areas with low-lying slopes, and in such a case speed up the chances of water stagnation. Lack of proper water drainage can lead to the formation of stagnant water pools, which in effect, encourage mosquito breeding and survival (Thomson et al., Citation1999). Areas on flat ground are most likely to accumulate and dam rainwater thereby increasing the risk of malaria (Freeman, Citation1995; Ofgeha, Citation2023).
2.2.5. Elevation
Elevation is a significant factor for malaria transmission. This is mainly because of elevation highly determines the amount of temperature. Temperature affects mosquito breeding as length of immature stage in life cycle (Ofgeha, Citation2023). In this study, elevation map was produced by processing DEM − 30 m resolution using ArcGIS software. The elevation raster layer was reclassified continuous scale in order of malaria hazard rating.
Altitude is related to the air temperature an area that affects the breeding of malaria vector. Malaria disease vector lives in an ideal area that is not too high that is between 100 and 500 m above sea level. The higher altitude of an area then the air temperature will tend to be lower. It will cause malaria vector inactive to bite, and affect to the lower transmission level of malaria disease.
2.2.6. Rainfall
Rainfall map was produced from secondary data processing of mean annual rainfall data of rainfall stations in and around the study area. The mean annual rainfall data was processed using interpolation inverse distance weight (IDW). The higher intensity of rainfall will increase the relative humidity that supports the survival of Anopheles mosquito, which resulted in an increase of the mosquito density (Ofgeha, Citation2023).
Rainfall data for the year 2022 for five stations: Bedesa, Gelemso, Abomsa, Sheki-Husen, and Seru were gained from National Meteorological Agency. These data were interpolated using inverse distance weight techniques in ArcGIS environment. Accordingly, the rainfall distribution of the study area was reclassified into five (760-847 mm, 848-9904 mm, 905-952 mm, 953-994 mm, and 995-1076 mm) and were assigned as very high, high, moderate, low and very low rainfall-based malaria risk level respectively.
In the study area, the annual average rainfall fluctuates between 760 and 1200 mm, with the major rainy season lasting from July to September and a minor wet season lasting from February to April. June, July, and August get the most rainfall, while May, June, March, September, and October have the least. The rain in the area is heavy and showery, with little volumes and a late start. The weather in the winter is usually quite warm.
2.2.7. Soil type
Soil type of the study area was generated from FAO classification based on their ability to hold moisture or being permeable or impermeable (Manoharan et al., Citation2020). There are four different types of soil in the study area. These include Calcaric Regosols, Haplic Yermosols, Eutric Cambisols and Haplic Xerosols. Based on their level of water holding capacity, they were reclassified and assigned as high, moderate, low and very low malaria risk area
2.2.8. Proximity to rivers
Rivers are ideal locations for malaria vector breeding places. The closest area to the rivers causes the risk of malaria disease to be increased. If the river has a steady flow, it will make the risk of malaria higher because the larvae of Anopheles prefer a steady flow to breathe through the spiracles (Gebre et al., Citation2020; Negassi, Citation2008; Ra et al., Citation2012). Classification and score of distance to rivers are made based on several literatures.
Proximity to rivers was generated by using Euclidean distance in spatial analyst tools of arcmap. Several studies (Kaufmann & Briegel, Citation2004; Negassi, Citation2008; Ra et al., Citation2012) confirmed that the maximum flight range of Anopheles mosquito is 2 km. thus, based on the flight range of mosquito the study area was reclassified into five (0-500 m, 500–1000, 1000–1500, 1500–2000 m, and >2000 m) were assigned as very high, high, moderate, low and very low river proximity based malaria risk level, respectively.
2.2.9. Land surface temperature
Step 1: Conversion to radiance
Near surface air temperature is a consequence of complex effects of the turbulent heat transports produced by nearby heated surfaces (Merga et al., Citation2022). After spectral radiance is converted to radiance, the raw digital numbers of the thermal bands were converted to top of atmosphere (TOA), which are the effective temperature viewed by the satellite under an assumption of emissivity (EquationEquation 5(5)
(5) )
Where;
Step 2: Conversion to brightness temperature
In the present study, LST was computed by using brightness temperature based on land surface emissivity. Band 10 was converted from spectral radiance to brightness temperature in landsat OLI/TIRS of 2023 (EquationEquation 6(6)
(6) ).
,
Step 3: Estimation of land surface emissivity (LSE) by using NDVI
The NDVI was used to calculate the abundance of vegetation cover on the surface of the earth. The NDVI value was derived using multispectral bands from Landsat images of 2023. Band 5 and 4 were used to assess NIR and red, respectively and NDVI was calculated using (EquationEquation 7(7)
(7) ).
A proportional vegetation (Pv) calculation was done based on the NDVI measurements to calculate land surface emissivity. The vegetation proportion was calculated using (EquationEquation 8(8)
(8) ).
Where;
An estimation of LST was accomplished and calculated using (EquationEquation 9(9)
(9) ).
Where;
Surface emissivity
The computed LST was corrected for emissivity using (EquationEquation 10(10)
(10) ).
Where;
,
,
,
,
,
.
Temperature above 30°C has negative impact on survival of the vector (Teklehaimanot et al., Citation2004). On the other hand for temperature below 16°C, the development of Anopheles gambiae, the main malaria vector in most parts of Africa, is completely stopped, and the larvae die in wet temperature below 14°C (Afrane et al., Citation2011). Accordingly, the study area’s temperature map was reclassified into five classes as < 15°C, 15–22°C, 23–32°C, 33–42°C, and > 42°C and assigned as very low, low, moderate, high and very high risk level respectively.
2.2.10. Human population density
Population data for this study was obtained from central statistical agency (CSA, Citation2022). The population shapefile of Ethio-GIS data x and y coordinate of the Oromia regional state was calculated from attribute. The gross population density method was used to calculate the number of persons per square kilometer. Population shapefile was converted to raster layer and then re-classified using equal interval method. The population density re-classification was based on the assumption that the denser the population, the more it will be at risk of malaria (Gebre et al., Citation2020).
2.2.11. Proximity to health institutions and roads
The two identified proxy indicators of access to treatment and care in this study area are proximity to road and health facilities. To identify health institutions accessibility, spatial locations of health institutions within the study area were collected with handheld global positioning system from woreda health office and processed in AcrMap 10.5. Hence, the study area’s distance to roads and health facilities was reclassified into five classes as <1000 m, 1001 – 2000 m, 2001 –3000 m, 3001 – 4000 m and >4000 m and labeled as very low, low, moderate, high and very high susceptible area to the risk of malaria. Several studies confirmed that environmental variable that influence the transmissions of malaria had applied best fit models with distance to roads and distance to health facilities (Feikin et al., Citation2009; Platt et al., Citation2018; Rutherford et al., Citation2010)
2.3. Multi criteria evaluation (MCE) for malaria risk mapping
MCE decision techniques with GIS methods are effective tools to swiftly and easily analyze secondary and primary large data. Consequently, it was used to generate optimum malaria risk maps by using multiple environmental and social data. In addition, in GIS, Multi Criteria Evaluation (MCE) looks into how land should be allocated for a certain use based on a range of characteristics that the places that are chosen have. Compromise alternatives and attractiveness-based ranking of alternatives can be produced with MCE (Gebre et al., Citation2020).
Using the proposed 1 to 9 scientific ratio scale, the AHP method of MCE analysis was used to calculate the criteria weights of the spatial layers to evaluate the potential site for malaria risk. During this study, eight physical parameters were assigned for accessing malaria risk prone areas. For each of targeted parameters, weighted value have been calculated by using analytical hierarchy process (AHP) method and multi-criteria decision analysis (MCDA) tool based on a stays 9-point scale. The reliability of the parameters weighted value was evaluated based on the relative importance value assigned by experts for each parameter (Table ).
Table 2. Pairwise comparison
Pairwise comparison matrix has been performed by using assigned relative importance value of each parameter in IDRISI Selva 17 to evaluate the malaria risk vulnerability. The pair wise comparison acceptability was based on the calculated value of consistency ratio (CR) which must be less than 0.1 (Moisa et al., Citation2023) and the consistence ratio in the present study was 0.08. The consistency ratio for parameters pairwise comparison was determined as (EquationEquation 11(11)
(11) ).
Where CI represents consistency Index and RI represents random consistency Index.
Consistency index was the measure of how all targeted parameters were interrelated and calculated as (EquationEquation 12(12)
(12) ).
Where n indicate the number of parameters, refers to is the principal Eigen value of parameters that can be calculated from multiplication of the total horizontal summation of given intensity importance value and parameters weighted value (Table ). The normalized principal Eigen vector was obtained from averaging the normalized relative weight of the parameters.
Table 3. Pairwise comparison of the parameters
The random consistency index was an assigned constant number based on the number of parameters used in the study (Table ).
Table 4. Random index value table
2.4. Parameters rating scale for malaria risk assessment
The weight assigned to each factors was calculated based on the pairwise comparisons of the three parameters. Hence, 55%, 15%, 30% were given for malaria hazard, vulnerability and elements at risk respectively. The scale variation in all targeted parameters may cause the presences of malaria risk incidence in the study area (Table ). Estimation of such like variation enables the concerned bodies to identify the malaria risk prone area and prioritizing for future resistance.
Table 5. Weight assigned for parameters (hazard, vulnerability and element at risk)
2.5. Weight overlay analysis
All criteria map of the parameters were overlaid by using parameters suitability index to calculate malaria risk prone areas. According to (Manoharan et al., Citation2020; Mihiretie, Citation2022; Ofgeha, Citation2023) malaria risk area was calculated as (EquationEq 13(13)
(13) ).
Where; SI is suitability index, Wi is weight of factor I, and Xi is normalized criterion of factors and ∑ is their the summation. General work-flow diagram for this study is presented on Figure .
3. Results and discussion
3.1. Accuracy assessment
Accuracy assessment was done from land use land cover types of 2023 for spatial analysis malaria risk distribution. Accordingly, the overall accuracy is 96% and the Kappa coefficient is 0.947 which is acceptable for further analysis.
3.2. Land use and land cover (LULC)
Accuracy assessment was done for spatial malaria risk distribution. Accordingly, the overall accuracy is 96% and the Kappa coefficient is 0.947 which is acceptable for further analysis.
In this study, LULC was classified into five categories and labeled as forest, shrub land, grass land, farm land and settlement. Land use and land cover has big influence on Anopheles mosquito life cycle. The study has been reclassified as very high, high, moderate, low and very low malaria risk level. From the total area, settlement 827.7 km2 (14.8%) followed by farm land 894.6 km2 (16.0%) were assigned as very high and high LULC-based malaria risk level. Shrub land 129.5 km2 (2.3%) and forest 1983.8 km2 (35.4%) in the study area are favor mosquito breeding at low and very low respectively (Table ). Residential areas are more prone to the risk of malaria disease (Figure ). It affects the temperature of larval habitats directly and food conditions indirectly. The prevalence of a major malaria disease is closely matched with the naturally occurring environmental and climatic conditions. Several studies also justify that increasing of more settlement can lead to increase of malaria incidence (Mihiretie, Citation2022). The incidence, intensity and spread of malaria diseases are significantly influenced by human land use changes.
Table 6. Land use and land cover (LULC)
3.3. Malaria hazard analysis
3.3.1. Topographic wetness index (TWI)
The TWI of the study area was classified into five classes and labeled as very high (>8.8), high (7.01–8.8), moderate (6.01–7.0), low (5.0–6.0) and very low (<5.2) malaria risk level. Accordingly, the results of this classification reveals that an area of 101.6 km2 (1.8%), 470.6 km2 (8.4%) and 980.5 km2 (17.5%) were fall under very high, high and moderate malaria risk level, respectively. The remaining areas of 2538.5 km2 (45.4%) and 1505.4 km2 (26.9%) were categorized under low and very low malaria risk (Table ). Result from this finding reveals that large portion of the study area is grouped under low TWI based malaria risk areas.
Table 7. Topographic wetness index (TWI) range
Topographic wetness index was calculated as the ratio of the area upslope i.e. from where water would flow into that point from any given point on the landscape to the local slope at that point thus corresponding to the amount of water that should enter a given spatial unit divided by the rate at which the rate at which the water should flow out of that unit (Nelson & Haksama, Citation2018; Rohan, Citation2012). TWI is an appealing measure because it indicates a simple, biologically meaningful description of how topographic characteristics, like slope and surface curvature may affect malaria risk via suitability for mosquito breeding (Ahmed, Citation2014; Nelson & Haksama, Citation2017).
3.3.2. Slope
Slope plays an important role that enhances the malaria breeding site in a certain locality. Based on the suitability of the slope for mosquito breeding, the reclassified slopes map was produced. Accordingly, area of 766.5 km2 (13.7%), 1253.4 km2 (22.4%), 2878.6 km2 (51.4%), 527.7 km2 (9.4%) and 170.4 km2 (3.0%) are very high, high, moderate, low and very low malaria risk area respectively. Areas labeled as very high and high have slope less than 5° and 5–11° that allow water stagnation and create suitable conditions for mosquito breeding. This clearly shows that large portion (22.4%) of the study area is highly vulnerable to the risk of malaria (Table ).
Table 8. Slope
Considering the relationship between slope and malaria case, water appears to be logged in areas with low-lying slopes, in such a case speeds up the chance of water stagnation. Several studies (Freeman, Citation1995; Bian et al., , Wondim et al. 2017; Manoharan et al., Citation2020; Ofgeha, Citation2023) justified that areas on flat ground are most likely to accumulate and dam rainwater thereby increases the risk of malaria.
3.3.3. Elevation
In this study, areas within an altitude of 703 – 1185 m above mean sea level was assigned as very high, whereas, areas range between 1,844–2,988 m above mean sea level was assigned as very low elevation based malaria risk area. Hence, an area of 106.6 km2 (1.9%), 1586.8 km2 (28.4), 3362.7 km2 (60.1%), 157.8 km2 (2.8%) and 382.8 (6.8%) very high, high, moderate, low and very low malaria risk areas due to altitudinal factor respectively (Table ). Altitude play an important role in limiting malaria in the tropical highlands by negatively influencing the breed of vector species. According to FMoH (Citation2009) malaria transmission in Ethiopia mainly occurs up to 2000 meter elevation but, can also occasionally affects areas up to 2300 m elevation. Based on this standard, map of the study area greater than 2300 was identified as very low risk level of malaria occurrences. There is a negative relationship between altitude and mosquito abundance. Fertility of mosquito became decrease with an increase of elevation (Ebi et al., Citation2005).
Table 9. Elevation (altitude)
3.3.4. Rainfall
The amount and distribution of rainfall experienced in the sub-basin indicate the spatial variations for malaria breeding suitability. Therefore, the ideal place for rainfall based mosquito breeding is the area that lies within 994.1 – 1076 mm of rainfall which is assigned as very high malaria risk area followed by rainfall 952 – 994 mm which is assigned as high malaria risk area cover an area of 191.7 km2 (3.4%) and 476.5 km2 (8.5%) of total area, respectively. The remaining 994.0 km2 (17.8%), 2477.6 km2 (44.3%) and 1456.6 km2 (26.0%) of the total areas were fell under moderate, low and very low malaria risk areas (Table ). This clearly indicates that rainfall distribution of the sub-basin facilitates mosquito growth and development which favors malaria prevalence. The abundance of Anopheles mosquito is strongly affected by rainfall. High precipitation increases water surface that harbor the malaria vector. Mosquito breed in freshwater pools, temporal and polluted water surface (Ofgeha, Citation2023).
Table 10. Rainfall distribution
3.3.5. Soil type
In this study, soil type was reclassified based on water holding capacity of soils. Accordingly, poorly drained soils leveled as high. Eutric Cambisols soil has good water holding capacity and reclassifies as high risk level. It covers an area of 548.8 km2 (9.8%) in the study area. Calcaric Regosols is a deep, well drained medium textured and have low water holding capacity and reclassified as very low risk level, and it covers an area of 409.6 km2 (7.3%) in the study area. More than half percent 3814.0 km2 (68.1%) of the study area is covered by Haplic Xerosols which is reclassified as moderate risk level of malaria occurrences. The remaining 824.2 (14.7%) of the total area is covered by Haplic Yermosols which is assigned as low factor for malaria risk (Table ).
Table 11. Soil types
Several studies (Manoharan et al., Citation2020; Ofgeha, Citation2023; Thomson et al., Citation1999) also explain that soil moisture content as one of the factors for mosquito breeding. Soils with greater water retention capacity assist malaria prevalence in the study area. Therefore, the soil drainage-based malaria risk was relatively low as compared to other parameters.
3.3.6. Proximity to rivers
In this study, areas closest to the streams less 500 m was assigned as very high; and areas beyond 2501 m far from rivers were assigned as very low spatial malaria risk. Accordingly, out of the total malaria vulnerable area, 273.3 km2 (4.9%) is highly vulnerable due to its closes distance to the rivers (Table ). An area of 273.3 (4.9%), 716.7 (12.8%), 1357.3 (24.3%), 1775.7 (31.7%) and 1473.6 (26.3%) mapped as very high, high, moderate, low and very low causes for malaria risk distribution as the distance from the river as risk factor respectively. This indicates that areas closer to water bodies are more vulnerable to the risk of malaria incidence.
Table 12. Proximity to rivers (m)
Several studies also report that the proximity to water bodies has direct implication on the community’s exposure to malaria hazards (Manoharan et al., Citation2020; Ofgeha, Citation2023). According to Santos et al. (Citation2016), people who live close to rivers or water bodies are prone to high spatial malaria risk.
3.3.7. Land surface temperature (LST)
LST based malaria risk occurrence show that temperature is one of the determinant factors facilitating malaria breeding in the study area. Accordingly, from the total area, 407.5 km2 (7.3%) and 764.2 km (13.6%) lie under very high and high level of malaria risk areas respectively. Area of 2148.5 km2 (38.4%), 2033.8 km2 (36.3%), and 242.6 km2 (4.3%) is categorized under moderate, low and very low level of malaria risk area respectively (Table ). Several studies (Afrane et al., Citation2011; Ofgeha, Citation2023) also justify that temperature play great role in determining malaria distribution. Development stage of mosquitoes is much dependent on temperature.
Table 13. Land surface temperature (LST)
Findings from this study clearly reveal that large portion of the study area is reasonably has a high probability of a percentage of influence for malaria hazard level (). There is high chance of malaria hazard occurrence in southwestern, southeastern and in eastern parts of the study area.
3.4. Malaria vulnerability analysis
Figure shows the vulnerability level to the risk of malaria. In this study, distance to health institutions, human population density and proximity to main roads were considered.
3.4.1. Proximity to health centers
Proximity to health institutions of the study area was considered as valuable since the areas furthest from the health centers set population to be vulnerable to malaria risk due the absence of health services. Existences of health institutions in a given area are essential for the prevention of malaria disease mainly because of its easily accessibility and fair cost of treatment. Distant health institutions result in difficulties in accessibilities and high cost of treatment (Manoharan et al., Citation2020). People who are near to health institutions are safer relative to those who are at farther places and takes lower risk level. Accordingly, of all the total areas, 1170.2 km2 (20.9%), 922.5 km2 (16.5%) 1198.7 km2 (21.4%) 1027.4 km2 (18.4%) 1277.8 km2 (22.8%) are mapped as very high, high, moderate, low and very low malaria vulnerable areas respectively (). Access to health services especially for children under the age of five (5) has the potential of reducing significantly the number of malaria cases and death (Rutherford et al., Citation2010). People who live far away from health institutions are highly susceptible to the risk of malaria (Stratton et al., Citation2008).
Table 14. Proximity to health centers
3.4.2. Proximity to roads
In this study, an assumption was made that the shorter the distance from the roads, the higher the probability of access to prevention and control, thus its vulnerability to malaria risk is lower.
Accordingly, an area of 2246.82 km2 (40%), 1678.8 km2 (30.0%), 1058.4 km2 (18.9) 361.7 km2 (6.5%) 250.6 km2 (4.5%) are subjected to very low, low, moderate, high and very high susceptible to the risk of malaria respectively (Table ). According to the experts, poor transportation makes difficult to perform blood smear verification. Distance of a place from roads could determine the effectiveness of measures to be taken to control the risk of malaria infestation. Study conducted by Feikin et al. (Citation2009) reported that severely ill cases traveled further for clinic visit, and the rate of clinic visits decreased linearly with the number of kilometers from the residence to clinic.
Table 15. Proximity to roads
3.4.3. Population density
Human population density was found to be a significant predictor of malaria risk as it provides a consistent metric to adjust for the patterns of malaria risk in densely populated areas (Kabaria et al., Citation2017). The population density was reclassified in the assumption that the denser the population density, the more the venerable to the risk of malaria. As a result, an area of 634.41 (11.3), 1098.12 (19.6), 691.89 (12.4), 2169.19 (38.8%), 1002.76 (17.9%) are mapped as very highly, highly, moderately, low and very low vulnerable to the risk of malaria respectively (Table ). High population density areas are prone to malaria manifestation mainly due to the development conditions that would influence the transmission of vector-borne diseases resulted from unprotected water storage and poor waste disposal system (Santos et al., Citation2016). Malaria incidence has a strong correlation with population size and area of the village (Kreuels et al., Citation2008).
Table 16. Population density risk level
3.5. Analysis of malaria risk occurrences using geospatial techniques
The fast, efficient, and economical determination of malaria risk areas would be made possible by the incorporation of advanced geospatial technologies into malaria management strategies. Planning, controlling, and managing the malaria disease more effectively is made possible by the forecast of malaria risk areas. The malaria risk map is the result of the overlaid maps of all parameters i.e. hazards, vulnerability and element at risk (Figure ). The malaria risk map of the study area was produced using weighted overlay method. Accordingly, 1867.8 km2 (33.4%), 2112.7 km2 (37.7%) and 1181.6 km2 (21.1%) were categorized under high, moderate and low level of malaria risk in Wabi Shebele River Sub-basin. Geographically, northern, eastern, western and some southern parts of study area were captured high level of malaria risk distribution. However, central, some southern and some northern parts of the study area were captured with low and very low malaria risk level of distribution (Figure ). This shows that majority of the study area fell in moderate followed by low risk level. Low and very low malaria risk level covers small areas, which occupies only 1181.6 km2 (21.1%) and 434.5 km2 (7.8%) respectively (Table ). Malaria risk level in this study area is relatively lower than reported by Abdulahia et al. (Citation2020) in Koahey zone of Somali regional state which was more than 70.5% of the study area fell in the high risk level. In contrast to this, study conducted by Mihiretie (Citation2022) in East Gojjam indicated that 24.49% of the total area was subjected to high level of malaria risk. The results of this study is in line with study conducted by Gebre et al. (Citation2020) in Didessa district southwestern Ethiopia where there is a high rate of malaria prevalence and incidence.
Table 17. Malaria risk level
4. Conclusion
Malaria is spread by mosquitoes and affects people and other animals. It is brought into the body through the bite of an infected female Anopheles mosquito, which carries parasites from the Plasmodium genus. This study assessed malaria hazard and risk using geospatial techniques in Wabi Shebele River Sub-basin, Southeastern Ethiopia. It revealed that GIS and remote sensing play great role to map malaria hazard and risk areas. Physical factors like LULC, topographic wetness index, slope, elevation (altitude), soil types, proximity to rivers; rainfall and surface temperature were developed and reclassified based on their suitability for mosquito breeding and malaria incidence. For each of targeted parameters, weighted value have been calculated by using analytical hierarchy process method and multi-criteria decision analysis. All criteria map of the parameters were overlaid by using parameters suitability index to calculate malaria risk prone areas. The result revealed that, about 33.4%, 37.7% and 7.8% were high, moderate and very low risk for malaria distribution. This shows that, large portion of the study area is moderately vulnerable to the risk of malaria. Health officers other organization should pay great attention to prioritize areas those highly and moderately vulnerable to the risk of malaria; and take a measurement action. Based on the result, we suggest that, socio-economic data and other parameters should be added to sufficiently provide best model for malaria disease management for all concerned bodies in the study area.
Consent for publication
The authors agreed to publish on journal of environment, development and sustainability.
Author contribution
BBM was involved in all stages of this study including field work, analysis and writing the manuscript. MBM and DOG were engaged in methodology development and data analysis. All authors read and approved the final manuscript.
Acknowledgements
The authors would like to acknowledge Oda Bultum University, Wollega University; and Jimma University for providing facilities to conduct this research.
Disclosure statement
No potential conflict of interest was reported by the author(s).
Additional information
Funding
References
- Abdulahia, A., Wudad, A., Abajihad, M., & Mohammed, F. (2020). Malaria hazard and risk analysis using GIS and remote sensing in Korahey Zone of Somali regional state, Eastern Ethiopia. American Scientific Research Journal for Engineering Technology, and Sciences (ASRJETS) 68(1), 20–18. http://asrjetsjournal.org/
- Afrane, Y., Githeko, K., & Yan, G. (2011). Malaria transmission in the African highlands in a changing climate situation: Perspective from Kenyan highlands, global warming impacts: - case studies on the economy, human health, and on urban and natural environments (Stefano Casalegno, Ed.). https://doi.org/10.5772/24946
- Ahmed, A. (2014). Geographic information science and remote sensing for malaria risk mapping, Ethiopia. The International Archives of the Photogrammetry, Remote Sensing and Spatial Information Sciences, Volume XL-8, 2014 ISPRS Technical Commission VIII Symposium (Vol. 40, pp. 155–161).
- Betemariam, G. A. Y. (2002). Severe malaria among children in Gambella region, western Ethiopia. Ethiopian Journal of Health Development, 16(1), 61–70. https://doi.org/10.4314/ejhd.v16i1.9827
- Bhatta, B. (2008). Remote sensing and GIS. Oxford University Press.
- Chakarova, B., Kichukova, K., & Karaslavov, G. (2015). A short historical overview of malaria in worldwide and in Bulgaria. The fight against malaria - the main task of sanitary epidemiological station in stara zagora in the first year of its opening. Trakia Journal of Sciences, 13(Suppl. 2), 151–154. https://doi.org/10.15547/tjs.2015.s.02.032
- CSA. (2022). National census 2005. Ethiopian Central Statistical Agency.
- Ebi, K. L., Hartman, J., Chan, N., McConnell, J., Schlesinger, M., & Weyant, J. (2005). Climate suitability for stable malaria transmission in Zimbabwe under different climate change scenarios. Climatic Change, 73(3), 375–393. https://doi.org/10.1007/s10584-005-6875-2
- Feikin, D. R., Nguyen, L. M., Adazu, K., Ombok, M., Audi, A., Slutsker, L., & Lindblade, A. K. (2009). The impact of distance of residence from a peripheral health facility on pediatric health utilisation in rural western Kenya. Tropical Medicine & International Health: TM & IH, 14(1), 54–61. https://doi.org/10.1111/j.1365-3156.2008.02193.x
- Felix, P. A. (2003). Towards a risk map of malaria for Sri Lanka: The importance of house location relative to vector breeding sites. International Journal of Epidemiology, Great Britain, 32, 280–285.
- FMoH. (2009). National strategic plan for malaria prevention, control and elimination in Ethiopia 2010–2015. Fed Ministry Health Addis Ababa Ethiop Geosci Geom, 1(1), 8–14.
- Foody, G. M., Campbell, N. A., Trodd, N. M., & Wood, T. F. (1992). Derivation and applications of probabilistic measures of class membership from the maximum-likelihood classification. Photogrammetric Engineering and Remote Sensing, 58(9), 1335–1341.
- Freeman, T. W. (1995). Binga district - base line survey of the malaria situation. Save The Children.
- Gebre, S. L., Nasreddin, T., & Alemayehu, R. (2020). Spatial analysis and mapping of malaria risk areas using multi-criteria decision making in Didessa district, South West Ethiopia. Cogent Environmental Science, 6(1), 1860451. https://doi.org/10.1080/23311843.2020.1860451
- Gruber, S., & Peckham, S. (2009). Land-surface parameters and objects in hydrology. In T. Hengl,& H. I. Reuter (Eds.), Developments in soil science (Vol. 33, pp. 171–194). Elsevier.
- Hasyim, H., Nursafingi, A., Haque, U., Montag, D., Groneberg, D. A., Dhimal, M., Kuch, U., & Müller, R. (2018). Spatial modelling of malaria cases associated with environmental factors in South Sumatra, Indonesia. Malaria journal, 17(1). https://doi.org/10.1186/s12936-018-2230-8
- Kabaria, C. W., Gilbert, M., Noor, A. M., Snow, R. W., & Linard, C. (2017). The impact of urbanization and population density on childhood Plasmodium falciparum parasite prevalence rates in Africa. Malaria journal, 16(1), 49. https://doi.org/10.1186/s12936-017-1694-2
- Kaufmann, C., & Briegel, H. (2004). Flight performance of the malaria vectors anopheles gambiae and anopheles atroparvus. Journal of Vector Ecology: Journal of the Society for Vector Ecology, 29(1), 140–153. http://www.ncbi.nlm.nih.gov/pubmed/15266751
- Kreuels, B., Kobbe, R., Adjei, S., Kreuzberg, C., Von Reden, C., Bäter, K., Klug, S., Busch, W., Adjei, O., & May, J. (2008). Spatial variation of malaria incidence in young children from a geographically homogeneous area with high endemicity. The Journal of Infectious Diseases, 197(1), 85–93. https://doi.org/10.1086/524066
- Manoharan, R., Alemu, M., Legesse, B., & Abajihad, M. (2020). Malaria hazard and risk analysis using geospatial techniques in the case of selected woredas of Jimma Zone, Oromia Region, Ethiopia. Earth Systems and Environment, 5(1), 115–126. https://doi.org/10.1007/s41748-020-00170-w
- Merga, B. B., Moisa, M. B., Negash, D. A., Ahmed, Z., & Gemeda, D. O. (2022). Land surface temperature variation in response to landuse and land-cover dynamics: A case of Didessa river sub-basin in western Ethiopia. Earth Systems and Environment, 6(4), 803–815. https://doi.org/10.1007/s41748-022-00303-3
- Mihiretie, A. A. (2022). Assessment of malaria risk using GIS and multi criteria: The case study of East Gojjam Zone, Ethiopia. International Journal of Environment & Geoinformatics, 9(1), 074–078. https://doi.org/10.30897/ijegeo.781219
- Moisa, M. B., & Gemeda, D. O. (2021). Analysis of urban expansion and land use/land cover changes using geospatial techniques: A case of Addis Ababa city, Ethiopia. Applied Geomatics, 13(4), 853–861. https://doi.org/10.1007/s12518-021-00397-w
- Mulugojjam, A., Tamiru, M. A., & Yeshanew, A. G. (2015). Assessment of control measures and trends of malaria in BurieZuria district, West Gojjam Zone, amhara region, North West Ethiopia. Malaria Research and Treatment, 2015, 1–5. https://doi.org/10.1155/2015/302194
- Negash, D. A., Moisa, M. B., Merga, B. B., Sedeta, F., & Gemeda, D. O. (2021). Soil erosion risk assessment for prioritization of sub-watershed: The case of chogo watershed, Horo Guduru Wollega, Ethiopia. Environmental Earth Sciences, 80(17), 589. https://doi.org/10.1007/s12665-021-09901-2
- Negassi, F. (2008). Identifying, mapping and evaluating environmental factors affecting malaria transmission using GIS and RS in selected Kebeles of Adama district, Oromia region. Addis Ababa university.
- Nelson, L. I., & Haksama, S. (2017). Spatial analysis of the correlation between topographic wetness index with annual parasites incidence malaria in South Central Timor district âA ¸S Indonesia. https://doi.org/10.5220/0007543303760380
- Nelson, L. I., & Haksama, S. (2018). Spatial analysis of the correlation between topographic wetness index with annual parasites incidence malaria in South Central Timor district 2017 âA ¸S Indonesia. In Proceedings of the 2nd International Conference Postgraduate School (ICPS 2018), (p. 376–380). https://doi.org/10.5220/0007543303760380.
- Ofgeha, G. Y. (2023). Spatial analysis of malaria hotspots in dilla sub-watershed: Western Ethiopia. Public Library of Science One, 18(4), e0273271. https://doi.org/10.1371/journal.pone.0273271
- Olana, D., Chibsa, S., Teshome, D., Mekasha, A., Graves, P. M., & Reithinger, R. (2011). Malaria, Oromia regional state, Ethiopia, 2001–2006. Emerging Infectious Diseases, 17(7), 1336–1337. PMID: 21762615. https://doi.org/10.3201/eid1707.100942
- Platt, A., Obala, A. A., MacIntyre, C., Otsyula, B. O., & Meara, W. P. (2018). Dynamic malaria hotspots in an open cohort in western Kenya. Scientific Reports, 8(1), 647. https://doi.org/10.1038/s41598-017-13801-6
- Ra, P. K., Nathawat, M. S., & Onagh, M. (2012). Application of multiple linear regression model through GIS and remote sensing for malaria mapping in Varanasi District. India Health Science Journal, 6(4), 731–749.
- Riihimäki, H., Kemppinen, J., Kopecký, M., & Luoto, M. (2021). Topographic Wetness Index as a Proxy for Soil Moisture: The Importance of Flow-Routing Algorithm and Grid Resolution. Water Research, 57(10), e2021WR029871. https://doi.org/10.1029/2021WR029871
- Rohan, F. (2012). SAGA GIS training materials display and analysis of satellite imagery with a focus on NTT. 5–9.
- Rutherford, M. E., Mulholland, K., & Hill, P. C. (2010). How access to health care relates to under-five mortality in sub-saharan Africa: Systematic review. Tropical Medicine and International Health, 15(5), 508–519. https://doi.org/10.1111/j.1365-3156.2010.02497.x
- Santos, V. M., Bouma, M. J., Kohli, V., Pascual, M., & Maheu-Giroux, M. (2016). Population density, climate variables and poverty synergistically structure spatial risk in urban malaria in India. PLoS Neglected Tropical Diseases, 10(12), e0005155. https://doi.org/10.1371/journal.pntd.0005155
- Stratton, L., O’Neill, M., Kruk, M., & Bell, M. L. (2008). The persistent problem of malaria: Addressing the fundamental causes of a global killer. Social Science & Medicine, 67(5), 854–862. https://doi.org/10.1016/j.socscimed.2008.05.013
- Sujoy, K., & Tingneyuc, S. (2016). Integrating remote sensing and GIS for investigating malaria risk areas in morobe province-papua new guinea. International Journal of Current Research, 8(8), 37476–37483.
- Teklehaimanot, H. D., Lipsitch, M., Teklehaimanot, A., & Schwartz, J. (2004). Weather based prediction of Plasmodium falciparum malaria in epidemic-prone regions of Ethiopia I. Patterns of lagged weather effects reflect biological mechanisms. Malaria Journal, 3(1), 41. https://doi.org/10.1186/1475-2875-3-41
- Thomson, M. C., Connor, S. J., & D’Alessandro, U. (1999). Predicting malaria infection in Gambian children from satellite data and bednet use surveys: The importance of spatial correlation in the interpretation of results. The American Journal of Tropical Medicine and Hygiene, 61(1), 2–8. https://doi.org/10.4269/ajtmh.1999.61.2
- Wilson, J. P. (2018). Environmental applications of digital terrain modeling. Wiley Blackwell.
- Workineh, B., Mekonnen, F. A., Sisay, M., & Gonete, K. A. (2019). Malaria outbreak investigation and contracting factors in Simada district, Northwest Ethiopia: A case–control study. BMC Research Notes, 12(1). https://doi.org/10.1186/s13104-019-4315-z
- World Health Organization (WHO). (2012). WHO recommended insecticides for indoor residual spraying against malaria vectors.