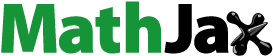
ABSTRACT
Understanding and managing land use and land cover (LULC) changes are crucial for addressing environmental challenges, promoting efficient utilization of natural resources, sustainability of environmental and ecological systems for the well-being of both present and future generations. This study was conducted to map the geo-physical features of Mampong municipality to assess the extent of LULC dynamism using Landsat 7 TOA (top-of-atmosphere) image of 2006 and Landsat 8 TOA (top-of-atmosphere) images of 2013 and 2020. Supervised random forest machine learning classification algorithm was applied to classify and examine the dynamics of land use changes in the study area. Markov chain simulation was adopted to examine the magnitude of transition of land cover changes. The results of the study revealed a substantial change in land cover uses in the study area over the period (2006–2020). The trend analysis in area percentage changes indicated that natural forest and deciduous forest land had reduced drastically mainly for the purpose of agriculture production and build-ups for human settlement as revealed by the negative change or reduction in typical biodiversity hotspot reservations: natural forest (−22.71%), deciduous forest (−26.74%) and water bodies (−32.11%) with a positive change in components such as built-up area (29.11%), barren land (4.96) and agriculture (37.91) in the study area. The study, however, revealed serious trend in water bodies extinction within the municipality as indicated by the dynamic change analysis results, suggesting that 7.86 km2 area that was originally under water pathways have been converted for human settlement and 0.76 km2 of agriculture lands are exposed to erosion (barren land). A Markov chain simulation prediction of the transition for the year 2032 revealed a positive probability increasing rate in urban and surface build-up and agriculture at a rate of 2.33% and 1.69%, respectively, with substantive reduction in natural biodiversity resources in future given the present scenario. Given the dynamics of LULC changes, we recommend that major stakeholders including environmental protection agency, forest guides, Ghana water company and municipal assembly authorities should develop feasibility holistic preventive measures that seek to ensure effective and efficient utilization of resources and promote the livelihood of individuals who depend on these resources for survival.
1. Introduction
Managing land use and land cover (LULC) changes is crucial for addressing environmental challenges due to the necessity to modify earth’s surfaces for the construction of social structures and facilities to meet the aesthetic lifestyle of the increasing contemporary globally geometric human population which is predicted to rise to 9.7 billion by 2050 (United Nations, Citation2022) and the sustainability of ecological systems. This rapid expansion in human population accompanied with its concomitant changes in lifestyle, taste and preferences has necessitated and induced the modification of land covers to increase agricultural production to an extent of 70% to provide the necessary quantum of food for the nutritional security and economic development of the growing population (Food and Agriculture Organization, Citation2022). However, the extent of these alterations to land surfaces has direct or indirect effects on the geo-physical properties with their adverse impacts on the environmental resources, biodiversity and global climate changes (Quintas-Soriano et al., Citation2016; Winkler et al., Citation2021), soil erosion and salinity of soils and permanent loss of land fertility and productivity (Foody, Citation2002; Hegazy & Kaloop, Citation2015; Liu & Yang, Citation2015), leaving most of the land covers sustainable for future generation. These transformations have been unprecedented in different geographical parts of the world due to large scale and extravagant utilization of natural resources by anthropogenic activities (Foley et al., Citation2005; Lambin et al., Citation2001), social, economic and political factors (Biney & Boakye, Citation2021; Wijitkosum, Citation2016). Managing LULC changes has received global attention and has been guided by international conventions because of climate change implications and management of earth surface and ecosystems to promote parsimonious use of available resources. The geophysical composition of many countries has undergone dramatic changes due to rapid expansion of built-up areas in various metropolis reducing vegetation cover dramatically as a result of the associated rise in commercial activities (Agidew & Singh, Citation2017; Banba & Shaw, Citation2017; David et al., Citation2016).
In Ghana, the change has been tremendous and rapid due to the rise in population from 6.7 million in 1960 just after independence to 18.9 million in 2000, 24.2 million in 2010, and 30.8 million in 2020 with an average annual rate growth of 2.15% (Ghana Statistical Service, Citation2021). This is projected to reach 34.4 million by 2025 and about 50 million by 2050 (United Nations, Citation2022). Moreover, significant high levels of migration, urbanization, mining pressures resulting in urban sprawl (Amenyo-Xa et al., Citation2017; Nyatuame et al., Citation2023), agriculture and herding activities (Yenibehit et al., Citation2024), cultural and technological factors (Sarfo et al., Citation2022) are critical factors leading to land deprivation, and climate variation which consequently trigger changes in LULC. Analysis on LULC changes and the rate of change provides critical information to enhance decision-making process in sustainable ecological management and environmental planning.
In Ghana, myriad of studies have been conducted with the concentration on impact of land use dynamics on urbanization and development of cities (Ampim et al., Citation2021; Iddrisu et al., Citation2023), sustainability of natural environment and biodiversity (Arfasa et al., Citation2023; Baidoo et al., Citation2023), watershed management (Abungba et al., Citation2022), and forest dynamics (Dembele et al., Citation2024; Frimpong, Citation2015; Kullo et al., Citation2021; Nyatuame et al., Citation2023; Yeaboah et al., Citation2017) as a means for justifying, validating, and supervising natural environment with the application of remote sensing (RS). The usage of RS and GIS (Geographic information system) in establishing and identifying the rate and extent of land cover change is to critically provide synoptic view of high-level management information for monitoring and mapping of ecological and biophysical properties, a vital input for the prioritization and rationalization of natural resource management as well as for the development of climate change adaptation strategies (Enoh et al., Citation2023; Patel et al., Citation2024; Zhang & Li, Citation2022). Monitoring LULC dynamics using geospatial techniques requires sophisticated multistage data processing skills with numerous, if not dozens or hundreds of, classifications, methods and algorithms to group image pixels into meaningful classification schemes (Mehmood et al., Citation2022; Zhao et al., Citation2024). However, the choice of techniques used depends on the availability of algorithms with the image processing software and experience of the researcher with the different methods. Previous geospatial scientists have established and used various LULC mapping techniques that vary from normal techniques like minimum distance and maximum likelihood (Abungba et al., Citation2022; Mahmoud et al., Citation2023; Norovsuren et al., Citation2019), convolutional neural network (Fang et al., Citation2019; Lui et al., Citation2018; Xie et al., Citation2019), artificial neural network (Buğday & E, Citation2019; Eshetie et al., Citation2023; Sisay et al., Citation2023), the Bayesian maximum likelihood (Ali et al., Citation2018), hybrid machine learning (ML) models: support vector machine and K-nearest neighbors (Akshya & Priyadarsini, Citation2019; Alimjan et al., Citation2018; Prasad et al., Citation2017), light gradient boosting (Abdi, Citation2020; Ali et al., Citation2018) and random forest [RF] (Ghayour et al., Citation2021; Maxwell et al., Citation2018; Tavares et al., Citation2019).
In Ashanti region, Mampong municipality is one of the fastest-growing areas where adequate information on LULC change over the period is almost non-existent. This leaves a gap in understanding the dynamics of LULC change in this municipality. Therefore, the need to research into the implications of LULC change in this municipality using RS and GIS (Geographic information system) is appropriate and cost-effective and has well-established technologies in understanding the landscape dynamics. Thus, this study was conducted to investigate land use changes and dynamics of terrestrial surface changes in terms of natural and man-built types in the municipality and to understand and monitor the overall dynamics of natural environment. This paper, therefore, contributes to previous studies (Frimpong, Citation2015; Kotei et al., Citation2013) by putting emphasis on implications of land use dynamics in Mampong using RS and advanced econometric modelling techniques. The remaining sections of the paper are organized as follows. Section 2 provides a brief description of data and the econometric modeling methodology, while section 3 describes the empirical analysis and results. Section 4 contains the concluding remarks.
2. Materials and methods
2.1. Study area
The present study focused on Mampong municipality located in the Ashanti Region of Ghana. The Mampong municipality was purposively selected from the rest of the districts in the region for the study as result of the rapid changes in land use due to upspring of universities and other tertiary institutions as well as its elevation into a municipality in 2007 and to police regional command, resulting in concomitant upsurge in population. The municipality as depicted in is bounded to the east by Sekyere Central, south by Sekyere South, and Ejura-Sekyedumasi to the West. The municipality is located within longitudes 0° 05″ W and 1° 30″ W and latitudes 6° 55″ N and 7° 30″ N, and covers a total land area of 782 km2 with 79 settlements of which about 61% being rural. The total population of the municipality is projected as 116,632 with an annual growth rate of 1.3% (Ghana Statistical Service, Citation2021). The municipality is generally low lying and rise gradually through rolling hills stretching southwards towards the Mampong scarp with the highest point of 2,400 m above sea level, while the lowest is about 135 m. The municipality has an average annual temperature of 27°C with variations in mean monthly temperature ranging between 22°C and 30°C (Ghana Meteorological Service, Citation2021). The mean annual rainfall, which is bimodal and fairly distributed, ranges between 800 and 1500 mm.
2.2. Data and image pre-processing
The study employed secondary datasets, which were derived from Landsat satellite imagery. Landsat TOA (top-of-atmosphere) satellite image datasets of 2006, 2013 and 2020 were used for the study in the municipality.
The images were selected over equal time intervals as dynamic changes in geomorphological land features are usually geological and gradual. Images were captured at approximately the same period to ensure that the selected image had the same reflectance values, which were derived from Google Earth Engine (GEE). The selected imagery was radiometrically corrected to eliminate possible noise of haze, and also geometrically corrected and projected to the Universal Transverse Mercator zone 30 North. According to Yu et al. (Citation2019) and Lu and Weng (Citation2007), band 4, 5 and 6 are the best three-band combination and band 1, 2, 5 and 7 are the best four-band combination which produces almost identical performance for LULC classification. Therefore, bands 1, 2, 5 and 7 of the images were stacked with the application of QGIS (Geographic information system), and R software algorithms were used for the classification of images in the present study, as shown in .
2.3. Vegetation cover analysis
The normalized difference vegetation index (NDVI) was adopted to study vegetation productivity or mapping of vegetation encroachment and land degradation in the study area. NDVI was estimated by following the mathematical expression:
where NIR and RED are spectral information in the near infra-red and red bands, respectively, of the satellite imagery. By design, the NDVI values varies between −1.0 and +1, with higher and positive values indicating higher photosynthetic activity or vegetation greenness. Values near zero (0) are usually non-vegetated areas and negative values indicate water bodies (Wegmann et al., Citation2016).
2.4. Classification algorithms and classes of land cover type mapping
In this study, supervised random forest (RF) machine learning (ML) classifier algorithm was adopted for the classification of the selected imagery. RF is a robust algorithm for classification and regression of data with high dimensionality and potentiality of discrimination and grouping of digital levels of image classification which produces good results that are suitable for the classification of multisource RS and geographic data. This is due to its ability to minimize the correlation between the classifiers in the ensemble by bootstrapping and aggregating o ensemble to form classification and regression tree (Kulkarni & Barrett, Citation2016; Wegmann et al., Citation2016). The RF classifier is not sensitive to noise or overtraining as the resampling is not based on weighting, and it is computationally much lighter based on boosting as well as Out of the Bag error estimate and evaluation of variable performance (Ghayour et al., Citation2021; Tavares et al., Citation2019). Moreover, the RF was adopted due to its ability to handle Hughes phenomenon as a result of the two-randomness dimensionality and, the capability to produce good classification results with a relatively reduced number of supervised training samples (Zhao et al., Citation2024). The land classes as described in were developed and served as the supervised training sites (Region of interest) fed to the RF algorithm for the classification of the geomorphological land use features in the present study area. The trained sites were developed using the Google Earth map along with expert knowledge on spectral signature of landmark features based on the geological composition of the area.
Table 1. Description of land class for classification
2.5. Assessment of accuracy
Assessing the accuracy of classification algorithms enables the evaluation of the error incurred during preparation of final thematic LULC maps. Therefore, the prepared LULC maps were subjected to an overall accuracy and Kappa coefficient statistical test. Kappa coefficient, a robust nonparametric statistical method that measures and describes the interrelationship between categorical variables in confusion (error) matrix (Olofsson et al., Citation2013; Rossiter, Citation2004), was determined by using the following equation:
The overall accuracy of image classification was determined by the following equation:
where n stands for total number of observations (pixels), while r is number of rows in matrix. represents number of observations in row i and column i. On the other hand, marginal sum of row i and column i is denoted by
, respectively (Blaschke, Citation2010; Islam et al., Citation2018).
2.6. Dynamics of land use and land changes
Markov chain analysis was used to examine the dynamics of LULC changes in the study area. Markov chain approach was based on the probability distribution that a given class of land change under current study depends on distribution in the previous period that could be represented by a stationary first order Markov chain. Given the classes of land use dynamics, stochastic matrix with the probability Aij of movement of area under a given land class i in period t to another land use class group j in period t +1 is called transition probability matrix (TPM) which is expressed as follows:
where and
Here, P is a square matrix (n × n), with n being the total number of land classes under study. Each element (Aij) represents the probability of moving from one land use class i to other j. Following Kemeny and Snell (Citation1976) and Tariq et al. (Citation2022), the final probability matrix (F) for each state through finite Markov chain theory was defined as follows:
Given the F matrix, a Markov chain simulation of the transition probability was carried out to project the area change for the selected land use class for next decade based on the assumption that the dynamics follow a constant nonlinear growth rate shift as defined in Equationequation 4(4)
(4) . The calculated land area of each class for the period under study (2006–2020) was converted into time series data for growth rate analysis to understand the dynamics of land use changes over years by fitting exponential growth model expressed as follows:
where is the estimated land area under each class,
is intercept,
is the regression coefficient,
is the time variable and
is the error term. The growth rate was obtained from the logarithmic form of the Equationequation (1)
(1)
(1) as
The percent growth rate (g) was derived using the following expression:
where is the growth rate in percentage.
3. Results
The study of the geo-anthropogenic effect on the LULC in the present study area began with examination of the biophysical properties and landmark features in the geographical study area to enable proper identification of physical objects of the earth. However, bands 5, 4 and 3 were combined to form false color composition (FCC) to examine the spectral signature and landmark features in the study area as depicted in . The FCC was used to identify the various land use class based on their respective spectral signatures.
3.1. Vegetation cover analysis
The study of the dynamics in vegetation was analyzed using normalized differenced vegetative index (NDVI) as presented in and . The results revealed that there has been significant change in vegetation due to the reduction in NDVI values over the study period. The reduction in the average NDVI value of 0.43 in 2006 to 0.26 in 2020 suggests that there has been a complete degradation of vegetation cover. However, changes cannot be identified with the changes in NDVI, and thus the exact changes are examined in the next sub-section.
Table 2. Summary of NDVI
3.2. LULC classification map
The classified images of the original multispectral image and its decomposition into land use thematic map of the various land classes under present study using the RF algorithms are shown in . The classification accuracy estimated for the thematic maps produced from the original image was determined by the overall train accuracy and Kappa coefficients. The validation of the thematic classified image produced an overall accuracy value ranging from 91% for the 2006 classification map to 96% for 2020 thematic map with the kappa coefficient of 0.88 and 0.94, respectively. The kappa coefficient of 0.94 in 2020 implies that the classified classes were 94% totally identical to the ground truth features, whilst 88% and 89% identicality were observed for 2006 and 2013 images, respectively.
The results from the visual inspection of the thematic maps as depicted in indicate a substantive change, suggesting land covers have undergone dramatic shift over the years due to anthropogenic effect in the study area, as depicted by colour changes in the map. However, further analysis was conducted to estimate the area covered by each class and the extent of change among the various classes of land cover features under present study as presented in . The results of percentage trend analysis revealed that forest land cover has reduced drastically by 22.71% at a growth rate of −0.97% per annum. This magnanimous change in forest has serious implication on loss of natural resources and biodiversity as indicated by recent remarkable changes in climate variables and rainfall pattern in the study area.
Table 3. Area of land cover
Moreover, during the study time interval (2006–2020), there has been a considerable reduction in deciduous forest area at an exponential rate of −1.12% per annum translating into −26.74% change in total land area of deciduous forest. The drastic change and degradation in deciduous and typical natural forest are very likely to be associated with the rapid expansion of urbanization/built-ups which increased at an exponential rate of 2.23% per annum during the study period. The expansion of road network for easy accessibility to newly upcoming towns and villages within the municipality and intensification of agriculture which also witnessed an increase at an exponential rate of 2.69% during the period () to produce food to meet the demand of the population which is increasing geometrically at an annual growth rate of 1.3% (Ghana Statistical Service, Citation2021) within the municipality, and the increased exploitation of resources for gold and timber and firewood harvesting as a local source of energy for cooking. These results are in line with a study conducted by Boakye et al. (Citation2008), Frimpong (Citation2015)and Kullo et al. (Citation2021) who reported similar trend of forest resources degradation in an adjacent district to the present study area. The significant growth in urban settlements over the study period is inevitable and expected in a developing municipality where urbanization and migration exacerbate much pressure on existing land and land resources for urban structures, such as housing and commercial activities, as the expansion and development of these facilities depend directly on adjacent natural environment. This result is similar to a study conducted by Amenyo-Xa et al. (Citation2017) and Tuffor-Mills et al. (Citation2020) on LULC in Ghana who argue that built-up areas were one of the main principal factors responsible for reducing vegetation cover in Ghana due to upsurge in the demand for housing, and increasing commercial activities. However, barren land during the period increased substantially to 4.56% at an annual growth rate of 0.24%. The increase in barren land could be due to the activities of charcoal burning in the area as the activity dwells on forest product to meet the increasing demand of local energy for cooking resulting in deforestation as revealed by the drastic reduction in forest resources. Regarding croplands, agriculture land in the municipality is largely dispersed due to the nature of land ownership and type of farming practices, increased significantly by 37.92% at an annual exponential rate of 2.69%. This signifies the importance of crop production and availability of food in the municipality which is essential for food security, accounting for relatively low prices of locally produced food commodities within the municipality relative to other districts in Ghana. Moreover, water and river bodies in the district also reduced drastically at a rate of −1.30 per annum. This result supports a study conducted by Kotei et al. (Citation2013) on the effect of land use pattern on hydrological systems in the study area that reported a substantive 12.25% reduction in the annual mean streamflow in the study area. The drastic changes on water bodies could be due to the filling up of water bodies pathway, especially mushy areas within and around upcoming commercial area for building new structures for commercial activities such as car washing bays and sand wining. In this regard, further analysis was carried out to examine the dynamics of changes as discussed in the next sub-section.
3.3. Change detection analysis and dynamics of land use changes
The change detection in the LULC was analyzed by using the differences in the NDVI. The result is presented in the histogram depicted in . The distribution of the normalized differenced vegetation index indicates that there has been a reduction in vegetation cover as revealed by the negative NDVI.
To further examine the exact changes that has happened in areas which cannot be revealed by the changes in the NDVI, post classification analysis was conducted to examine the extent of land changes and tendency of change. The results are presented in .
Table 4. Area changes in land use changes (km2)
The results of the dynamic changes indicate that 8.0% of the total area change in forest, representing 9.04 km2 over the study period has been converted to agricultural land with transition probability of 23% (). Moreover, 1.41 km2 of forest area was converted into urban land use with 9% tendency of transition. The dynamic change results indicated that 8.43 km2 of deciduous forest has been transformed into urban usage, which has serious implication on soil fertility and destruction of natural ecosystem. Moreover, we found a clear tendency of 8.21 km2 of agricultural lands been converted in to urban with transition likelihood of 10% (). This could be due to the sale of relatively closer arable land to estate developer agents for urban expansion. The results, however, indicated that 7.86 km2 of the total area of water bodies were converted into urban settlement for commercial activities which might have serious implication on urban flooding in future. The transition matrix results () revealed that forest and deciduous degradation are purposely for urban settlement. However, the probability of agricultural land abandoned to barren land was about 2%. Therefore, utilization of urban space can be enhanced with proper urban planning and the application of modern urban planning architectural technology and design. The study results revealed a 33 % tendency of barren land areas to be rejuvenated into grassland with emergence of shrub. Other classes of land use are most likely to have changed by 2020, with a 13% probability of grassland being converted to farmland. The low likelihoods of farmland, forest and grassland areas being converted into barren land cover type (about 2%, 3% and 5%, respectively) are remarkable to ensure rejuvenation and protection of biodiversity in area. This can be attributed by recent efforts of policy makers in creating awareness on the need of conversation of natural reserves through the promotion of afforestation programmes.
Table 5. Transition matrix of land use dynamics
Simulation of the transition probability as depicted in was carried out to examine the changes that would be likely to happen by the next decade years (2032) among the various land use class using the percentage nonlinear rate of change (CGR) as per equation 6 as the baseline scenario due to rapid changes in population in municipality with higher demand for social amenities and food requirement to meet the developmental agenda in the municipality. The results of the simulation process are presented in .
Table 6. Projected land use changes by 2032
The results revealed that, given the above scenario, all thing being equal, natural forest, deciduous forest and grassland are likely to reduce at rates of −0.487%, −0.289% and −0.348% per annum, respectively, from their current state to other land transformation. Water bodies are likely to extinct at a rate of −0.128% which will pose serious threat to agriculture, urban livelihoodsand loss of important biodiversity hotspots. The former is essential so as to meet the food demand of the population as production of important commercial vegetables demands water during production especially in the dry seasons whilst the later could lead to flooding of settlement due to blockages of water pathway as recently observed in major urban settlements. Moreover, other areas are likely to be converted to urban area with 1% of agricultural land being barren land by 2032. The chances that there will be a change in other land cover for urbanization is 39.3% with 2% chance that other land type will be converted for agriculture production, resulting from changes in typical forest. These results provide the impetus for the requirements of immediate, stringent preventive measures on the utilization of land cover in the municipality.
4. Conclusion
Land use and vegetation cover changes, accompanied by recent climate changes, are the contemporary challenges facing humanity as they pose threat to ecosystem and biodiversity. Various methods have been developed to study the dynamics of changes, but RS has proven to be a viable method to study earth surface changes. With the application of remote sensing, the changes and transformation of land cover in Asante Mampong municipality have been examined. The results of the study showed that there has been a substantial change in the geo-physical features in the study area during the study period (2006–2020). The analysis revealed a negative change or reduction in natural forest (−22.71%) and deciduous forest (−26.74%), and positive change in components such as built-up area (29.11), barren land (4.56%) and agriculture (37.91%) under study. The dynamic change analysis results revealed that natural forest and deciduous forest land transformation were mainly for the purpose of agriculture production and build-ups for human settlement. The study, however, revealed serious trend in water bodies extinction within the municipality as the water bodies are left to be barren land due to urbanization and illegal activities of lumbers and sand wining with serious implication on food security and biodiversity. The use of spatiotemporal data on LULC change has revealed general picture of the main land use alterations in one of the most important districts of Ashanti Region. Our Markov chain simulation of the transition probability revealed positive increasing rate in urban and surface build-up and agriculture at a rate of 2.33% and 1.69%, respectively, with substantive reduction in natural biodiversity resources such as natural water bodies that are likely to be converted for other land form utilization by 2032. However, these adverse changes in land cover, which affect biodiversity and communities, if continued could pose serious environmental threat in rainy seasons for communities and food insecurity during the dry season. This study result will serve as fundamental information to local stakeholders on changes in LULC in the municipality during development planning for managing the use of land resources. Therefore, given the dynamics of LULC changes, we recommend that major stakeholders including environmental protection agency, forest guides, Ghana water company and municipal assembly authorities should develop feasibility holistic management preventive measures that seek to ensure effective and efficient utilization of resources for future generation and promote the livelihood of individuals who depend on these resources for survival.
Author contributions
All the authors contributed by conceiving, designing the research, analyzing the data, and critically reviewed and approved the final version of the manuscript.
Disclosure statement
No potential conflict of interest was reported by the author(s).
Data availability statement
Data used for this work is a public data that was downloaded from GEE. Landsat 5 TM+ image period of 2006 and Landsat 8 TOA (top-of-atmosphere) satellite image for the period 2013–2020. Code for data extraction was obtained through the link https://code.earthengine.google.com/. R-codes for data collection, processing and analysis are available on request.
References
- Abdi, A. M. (2020). Land cover and land use classification performance of machine learning algorithms in a boreal landscape using Sentinel-2 data. GIScience & Remote Sensing, 57(1), 1–12. https://doi.org/10.1080/15481603.2019.1650447
- Abungba, J. A., Adjei, K. A., Gyamfi, C., Odai, S. N., Pingale, S. M., & Khare, D. (2022). Implications of land use/land cover changes and climate change on Black Volta Basin future water resources in Ghana. Sustainability, 14(19), 12383. https://doi.org/10.3390/su141912383
- Agidew, A. M. A., & Singh, K. N. (2017). The implications of land use and land cover changes for rural household food insecurity in the Northeastern highlands of Ethiopia: The case of the Teleyayen sub-watershed. Agriculture & Food Security, 6(1). https://doi.org/10.1186/s40066-017-0134-4
- Akshya, J., & Priyadarsini, P. L. K. (2019). A hybrid machine learning approach for classifying aerial images of flood-hit areas. Proceedings of the International Conference on Computational Intelligence in Data Science (ICCIDS), Chennai, India (pp. 1–5). https://doi.org/10.1109/ICCIDS.2019.8862138
- Alimjan, G., Sun, T., Liang, Y., Jumahun, H., & Guan, Y. (2018). A new technique for remote sensing image classification based on combinatorial algorithm of SVM and KNN. International Journal of Pattern Recognition and Artificial Intelligence, 32(7), 1859012. https://doi.org/10.1142/S0218001418590127
- Ali, M. Z., Qazi, W., & Aslam, N. (2018). A comparative study of ALOS-2 PALSAR and Landsat-8 imagery for land cover classification using maximum likelihood classifier. The Egyptian Journal of Remote Sensing & Space Science, 21, S29–S35. https://doi.org/10.1016/j.ejrs.2018.03.003
- Amenyo-Xa, M. S., Mariwah, S., & Osei, K. N. (2017). Urban land use/land cover changes in the Tema metropolitan area (1990–2010). Geo Journal, 82(2), 247–258. https://doi.org/10.1007/s10708-015-9672-0
- Ampim, P. A. Y., Ogbe, M., Obeng, E., Akley, E. K., & MacCarthy, D. S. (2021). Land cover changes in Ghana over the past 24 years. Sustainability, 13(9), 4951. https://doi.org/10.3390/su13094951
- Arfasa, G. F., Owusu-Sekyere, E., & Doke, D. A. (2023). Past and future land use/land cover, and climate change impacts on environmental sustainability in Vea catchment, Ghana. Geocarto International, 38(1), 1, 2289458. https://doi.org/10.1080/10106049.2023.2289458
- Baidoo, R., Arko-Adjei, A., Poku-Boansi, M., Quaye-Ballard, J. A., & Somuah, D. P. (2023). Land use and land cover changes implications on biodiversity in the Owabi catchment of Atwima Nwabiagya North District. Ghana Heliyon, 9(5), 1–16. https://doi.org/10.1016/j.heliyon.2023.e15238
- Banba, M., & Shaw, R. (2017). Land use management in disaster risk reduction: Practice and cases from a global perspective. Springer Nature Publishers. https://doi.org/10.1007/978-4-431-56442-3
- Biney, E., & Boakye, E. (2021). Urban sprawl and its impact on land use land cover dynamics of Sekondi-Takoradi metropolitan assembly, Ghana. Environmental Challenges, 4, 100168. https://doi.org/10.1016/j.envc.2021.100168
- Blaschke, T. (2010). Object based image analysis for remote sensing. ISPRS Journal of Photogrammetry and Remote Sensing, 65(1), 2–16. https://doi.org/10.1016/j.isprsjprs.2009.06.004
- Boakye, E., Odai, S. N., Adjei, K. A., & Annor, F. O. (2008). Landsat images for assessment of the impact of land use and land cover changes on the Barekese catchment in Ghana. European Journal of Scientific Research, 21(4), 617–626.
- Buğday, E. A. B., & E, S. (2019). Modeling and simulating land use/cover change using artificial neural network from remotely sensing data. CERNE, 25(2), 246–254. https://doi.org/10.1590/01047760201925022634
- David, K., Yetta, G., Agung, F., Sharon, H., & Alison, C. (2016). Land use planning for disaster risk reduction and climate change adaptation: Operationalizing policy and legislation at local levels. International Journal of Disaster Resilience in the Built Environment, 7(2), 158–172. https://doi.org/10.1108/IJDRBE-03-2015-0009
- Dembele, F., Guuroh, R. T., Ansah, P. B., Asare, D. B. M., Da, S. S., Aryee, J. N. A., & Adu-Bredu, S. (2024). Land use land cover change and intensity analysis of land transformation in and around a moist semi-deciduous forest in Ghana. Trees, Forests and People, 15, 100507. https://doi.org/10.1016/j.tfp.2024.100507
- Enoh, M. A., Njoku, R. E., & Okeke, U. C. (2023). Modeling and mapping the spatial–temporal changes in land use and land cover in Lagos: A dynamics for building a sustainable urban city. Advances in Space Research, 72(3), 694–710. https://doi.org/10.1016/j.asr.2022.07.042
- Eshetie, A. A., Wubneh, M. A., Kifelew, M. S., & Alemu, M. G. (2023). Application of artificial neural network (ANN) for investigation of the impact of past and future land use–land cover change on streamflow in the Upper Gilgel Abay watershed, Abay Basin, Ethiopia. Applied Water Science, 13(11), 209. https://doi.org/10.1007/s13201-023-02003-3
- Fang, J., Yuan, Y., Lu, X., & Feng, Y. (2019). Robust space-frequency joint representation for remote sensing image scene classification. IEEE Transactions on Geoscience and Remote Sensing, 57(10), 7492–7502. https://doi.org/10.1109/TGRS.2019.2913816
- Foley, J., Defries, R., Asner, G. P., Barford, C., Bonan, G., Carpenter, S. R., Chapin, F. S., Coe, M. T., Daily, G. C., Gibbs, H. K., Helkowski, J. H., Holloway, T., Howard, E. A., Kucharik, C. J., Monfreda, C., Patz, J. A., Prentice, I. C., Ramankutty, N., & Snyder, P. K. (2005). Global consequences of land use. Science, 309(5734), 570–574. https://doi.org/10.1126/science.1111772
- Food and Agriculture Organization. (2022). Food security and agricultural mitigation in developing countries: Options for capturing synergies. FAO.
- Foody, G. M. (2002). Status of land cover classification accuracy assessment. Remote Sensing of Environment, 80(1), 185–201. https://doi.org/10.1016/S0034-4257(01)00295-4
- Frimpong, B. F. (2015). Land use and cover changes in the Mampong municipality of the Ashanti Region. Kwame Nkrumah University of Science and Technology. Kumasi (M.Sc thesis published).
- Ghana Meteorological Service. (2021). Mampong Climate Summary, Ghana. https://www.meteo.gov.gh/gmet/regional-weather-ashanti-region/
- Ghana Statistical Service. (2021). Ghana 2021 population and housing census: Population of regions and districts, general report. GPHC: Accra, Ghana
- Ghayour, L., Neshat, A., Paryani, S., Shahabi, H., Shirzadi, A., Chen, W., Al-Ansari, N., Geertsema, M., Amiri, M. P., Gholamnia, M., Dou, J., & Ahmad, A. (2021). Performance evaluation of sentinel-2 and Landsat 8 OLI data for land cover/use classification using a comparison between machine learning algorithms. Remote Sensing, 13(7), 1349. https://doi.org/10.3390/rs13071349
- Hegazy, I. R., & Kaloop, M. R. (2015). Monitoring urban growth and land use change detection with GIS and remote sensing techniques in Daqahlia Governorate Egypt. International Journal of Sustainable Built Environment, 4(1), 117–124. https://doi.org/10.1016/j.ijsbe.2015.02.005
- Iddrisu, S., Siiba, A., Alhassan, J., & Abass, K. (2023). Land-use and land cover change dynamics in urban Ghana: Implications for peri-urban livelihoods. International Journal of Urban Sustainable Development, 15(1), 80–96. https://doi.org/10.1080/19463138.2023.2184822
- Islam, K., Jashimuddin, M., Nath, B., & Nath, T. K. (2018). Land use classification and change detection by using multi-temporal remotely sensed imagery: The case of Chunati wildlife sanctuary, Bangladesh. Egyptian Journal of Remote Sensing and Space Science, 21(3), 37–47. https://doi.org/10.1016/j.ejrs.2016.12.005
- Kemeny, J. G., & Snell, J. L. (1976). Finite Markov Chains. Springer - Verlag.
- Kotei, R., Ofori, E., Kyei-Baffour, N., & Agyare, W. A. (2013). Land use changes and their impacts on the hydrology of the Sumampa catchment in Mampong-Ashanti, Ghana. International Journal of Engineering Research & Technology, 2(8), 2533–2549.
- Kulkarni, A. D., & Barrett, L. (2016). Random forest algorithm for land cover classification. International Journal on Recent and Innovation Trends in Computing and Communication (IJRITCC), 4(3), 58–63.
- Kullo, E. D., Biney, E., Harris, E., & Forkuo, E. K. (2021). The impact of land use and land cover changes on socioeconomic factors and livelihood in the Atwima Nwabiagya district of the Ashanti region. Ghana., Environmental Challenges. https://doi.org/10.1016/j.envc.2021.100226
- Lambin, E. F., Turner, B. L., Geist, H. J., Agbola, S. B., Angelsen, A., Bruce, J. W., Coomes, O. T., Dirzo, R., Fischer, G., Folke, C., George, P., Homewood, K., Imbernon, J., Leemans, R., Li, X., Moran, E. F., Mortimore, M., Ramakrishnan, P. S. & Vogel, C. (2001). The causes of land-use and land-cover change: Moving beyond the myths. Global Environmental Change, 11(4), 261–269. https://doi.org/10.1016/S0959-3780(01)00007-3
- Liu, T., & Yang, X. (2015). Monitoring land changes in an urban area using satellite imagery, GIS and landscape metrics. Applied Geography, 56(4), 42–54. https://doi.org/10.1016/j.apgeog.2014.10.002
- Liu, Y., Zhong, Y., & Qin, Q. (2018). Scene classification based on multiscale convolutional neural network. IEEE Transactions on Geoscience and Remote Sensing, 56(12), 7109–7121. https://doi.org/10.1109/TGRS.2018.2848473
- Lu, D., & Weng, Q. (2007). A survey of image classification methods and techniques for improving classification performance. International Journal of Remote Sensing, 28(5), 823–870. https://doi.org/10.1080/01431160600746456
- Mahmoud, R., Hassanin, M., Feel, H. A., & Badry, R. M. (2023). Machine learning-based land use and land cover mapping using multi-spectral satellite imagery: A case study in Egypt. Sustainability, 15(12), 9467. https://doi.org/10.3390/su15129467
- Maxwell, A. E., Warner, T. A., & Fang, F. (2018). Implementation of machine-learning classification in remote sensing: An applied review. International Journal of Remote Sensing, 39(9), 2784–2817. https://doi.org/10.1080/01431161.2018.1433343
- Mehmood, M., Shahzad, A., Zafar, B., Shabbir, A., Ali, N., & Ahmad, A. (2022). Remote sensing image classification: A comprehensive review and applications. Hindawi Mathematical Problems in Engineering, 2022, 1–24. https://doi.org/10.1155/2022/5880959
- Norovsuren, B., Tseveen, B., Batomunkuev, V., Renchin, T., Natsagdorj, E., Yangiv, A., & Mart, Z. (2019). Land cover classification using maximum likelihood method (2000 and 2019) at Khandgait valley in Mongolia. IOP Conference Series: Earth and Environmental Science, 381(1), 381. https://doi.org/10.1088/1755-1315/381/1/012054
- Nyatuame, M., Agodzo, S., Amekudzi, L. K., & Mensah-Brako, B. (2023). Assessment of past and future land use/cover change over Tordzie watershed in Ghana. Frontiers in Environmental Science, 11, 1139264. https://doi.org/10.3389/fenvs.2023.1139264
- Olofsson, P., Foody, G. M., Stehman, S. V., & Woodcock, C. E. (2013). Making better use of accuracy data in land change studies: Estimating accuracy and area and quantifying uncertainty using stratified estimation. Remote Sensing of Environment, 129(6), 122–131. https://doi.org/10.1016/j.rse.2012.10.031
- Patel, A., Vyas, D., Chaudhari, N., Patel, R., Patel, K., & Mehta, D. (2024). Novel approach for the LULC change detection using GIS & Google Earth Engine through spatiotemporal analysis to evaluate the urbanization growth of Ahmedabad city. Results in Engineering. https://doi.org/10.1016/j.rineng.2024.101788 21 101788
- Prasad, S. V. S., Savithri, T. S., & Krishna, M. I. (2017). Comparison of accuracy measures for RS image classification using SVM and ANN classifiers. International Journal of Electrical and Computer Engineering (IJECE), 7(3), 180. https://doi.org/10.11591/ijece.v7i3.pp1180-1187
- Quintas-Soriano, C., Castro, A. J., Castro, H., & García-Llorente, M. (2016). Impacts of land use change on ecosystem services and implications for human well-being in Spanish drylands. Land Use Policy, 54(20), 534–548. https://doi.org/10.1016/j.landusepol.2016.03.011
- Rossiter, D. G. (2004). Statistical methods for accuracy assessment of classified thematic maps. International Institute for Geo-information Science and Earth, Department of Earth Systems Analysis, Enschede. http://www.itc.nl/~rossiter/teach/R/R_ac.pdf
- Sarfo, I., Shuoben, B., Otchwemah, H. B., Darko, G., Kedjanyi, E. A. G., Oduro, C., Folorunso, E. A., Abdallah, M., Alriah, A., Amankwah, S. O. Y., & Ndafira, G. C. (2022). Validating local drivers influencing land use cover change in Southwestern Ghana: A mixed-method approach. Environmental Earth Sciences, 81(14), 367. https://doi.org/10.1007/s12665-022-10481-y
- Sisay, G., Gessesse, B., Fürst, C., Kassie, M., & Kebede, B. (2023). Modeling of land use/land cover dynamics using artificial neural network and cellular automata Markov chain algorithms in Goang watershed, Ethiopia. Heliyon, 9(9), e20088. https://doi.org/10.1016/j.heliyon.2023.e20088
- Tariq, A., Mumtaz, F., Majeed, M., & Zeng, X. (2022). Spatio-temporal assessment of land use land cover based on trajectories and cellular automata Markov modelling and its impact on land surface temperature of Lahore district Pakistan. Environmental Monitoring and Assessment, 195(114). https://doi.org/10.1007/s10661-022-10738-w
- Tavares, P. A., Beltrão, N. E. S., Guimarães, U. S., & Teodoro, A. C. (2019). Integration of Sentinel-1 and sentinel-2 for classification and LULC mapping in the urban area of Belém, eastern Brazilian Amazon. Sensors (Switzerland), 19(5), 19. https://doi.org/10.3390/s19051140
- Tuffour-Mills, D., Antwi-Agyei, P., & Addo-Fordjour, P.(2020). Trends and drivers of land cover changes in a tropical urban forest in Ghana. Trees Forests People, 2. https://doi.org/10.1016/j.tfp.2020.100040
- United Nations. (2022). World population prospects 2022: Summary of results. Department of Economic and Social Affairs Population Division. https://population.un.org/wpp/
- Wegmann, M., Leutner, B., & Dech, S. (2016). Remote sensing and GIS for Ecologist: Using open-source software. Pelagic Publishing. U.K.
- Wijitkosum, S. (2016). The impact of land use and spatial changes on desertification risk in degraded areas in Thailand. Sustainable Environment Research, 26(2), 84–92. https://doi.org/10.1016/j.serj.2015.11.004
- Winkler, K., Fuchs, R., Rounsevell, M., & Herold, M. (2021). Global land use changes are four times greater than previously estimated. Nature Communications, 12(1), 2501. https://doi.org/10.1038/s41467-021-22702-2
- Xie, J., He, N., Fang, L., & Plaza, A. (2019). Scale-free convolutional neural network for remote sensing scene classification. IEEE Transactions on Geoscience and Remote Sensing, 57(9), 6916–6928. https://doi.org/10.1109/TGRS.2019.2909695
- Yeboah, F., Awotwi, A., Forkuo, E. K., & Kumi, M.(2017). Assessing the land use and land cover changes due to Urban growth in Accra, Ghana. Journal of Basic and Applied Research International, 22(2), 43–50.
- Yenibehit, N., Abdulai, A., Amikuzuno, J., & Blay, J. K. (2024). Impacts of farming and herding activities on land use and land cover changes in the north eastern corridor of Ghana: A comprehensive analysis. Sustainable Environment, 10(1), 2307229. https://doi.org/10.1080/27658511.2024.2307229
- Yu, Z., Liping, D., Ruixing, Y., Junmei, T., Li, L., Zhang, C., Rahman, M. S., Zhao, H., Gaigalas, J., Yu, E. G., & Sun, Z. (2019). Selection of Landsat 8 OLI band combinations for land use and land cover classification. The Eighth International Conference on Agro-Geoinformatics (pp. 16–19). Istanbul, Turkey.
- Zhang, C., & Li, X. (2022). Land use and land cover mapping in the era of big data. The Land, 11(10), 1692. https://doi.org/10.3390/land11101692
- Zhao, Z., Islam, F., Waseem, L. A., Tariq, A., Nawaz, M., Islam, I. U., Bibi, T., Rehman, N. U., Ahmad, W., Aslam, R. W., Raza, D., & Hatamleh, W. A. (2024). Comparison of three machine learning algorithms using Google Earth Engine for land use land cover classification. Rangeland Ecology & Management, 92, 129–137. https://doi.org/10.1016/j.rama.2023.10.007