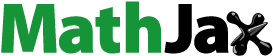
ABSTRACT
One of the byproducts of life is waste. Volume and complexity of solid waste have increased due to urbanization, economic growth, and rising living standards in cities. In most Ethiopian cities, residents do not pay for municipal solid waste management services. The study aims to examine Economic valuation and its’ determinates of improve solid waste management services in Gondar, Ethiopia. The study was conducted through face-to-face interviews with 222 randomly selected households. According to the survey results, 192 (87.3%) of the sampled households were willing to pay for better solid waste management services, with a mean willingness to pay of 34.48 and 17.0756 Ethiopian Birr (ETB) per month per household from the double-bounded dichotomous and open-ended contingent valuation methods, respectively. The total willingness to pay from open-ended and double-bounded contingent valuation methods is 941,361 and 1,900,848 ETB, respectively. Furthermore, the outcomes from the bivariate probit model indicated a positive and significant correlation between the willingness to pay bid amounts and both education and income. The study reveals that households’ inclination to contribute to enhanced solid waste management services is significantly and inversely affected by factors such as age, marital status, as well as the levels of Bid1 and Bid2. When allocating service charges, the city municipality should consider the amount of solid waste generated and the income level of the households. Awareness creation is important for better solid waste management. Additionally, the city municipality encouraged to facilitate awareness among urban households to minimize illegal waste disposal and encourage their participation.
1. Background of the study
Nowadays, in the 21st century, the world’s environment is becoming increasingly polluted and less conductive to life (Maskey & Singh, Citation2017b). Among these environmental issues, solid waste possess a significant problem due to the establishment of settled communities by humans (Gizaw et al., Citation2014). Solid waste is a continuously growing and challenging issue for local authorities in urban centers (Lami et al., Citation2019). Municipal solid waste generation and the variety of waste composition increase in line with the developmental rate of any country (Aleluia & Ferrão, Citation2016; Bhada-Tata & Hoornweg, Citation2012).
In 2012, cities around the world produced 1.3 billion tons of solid waste, and a number that increased to 2.01 billion tons annually by 2016 annually, equating to 1.2 kilograms per person per day. It is predicted that by 2025, municipal waste generation will increase to 2.2 billion tons and 3.40 billion tons by 2050, with households accounting for approximately 85.8% of the waste generated (Hoornweg & Bhada-Tata, Citation2012; Sanjeevi & Shahabudeen, Citation2015). In sub-Saharan Africa, waste generation is estimated at 62 million tons per year, ranging from 0.09 to 3.0 kilograms per person per day, with an average of 0.65 kilograms per person per day (Omran & Gebril, Citation2018; Zhu et al., Citation2007).
In Africa, municipal solid waste management (SWM) constitutes one of the most critical health and environmental problems facing governments in African cities (Akhtar et al., Citation2017; Fenta, Citation2017). It is expected that Africa will face the most sever challenges in SWM services due to rapid development, lack of proper planning, and inadequate waste disposal equipment management on the continent (Damtew & Desta, Citation2015; Mussa, Citation2015). Improper solid waste disposal practices have several implications for the environment, soil, air, water, public safety, health problems, and flooding during the rainy seasons (Ayilara et al., Citation2020). Waste generation is gradually increasing in developing nations, particularly in sub-Saharan Africa (SSA) (Kayamo, Citation2022). More than two-thirds of waste is disposed of in open dumps, leading to stagnant water that breeds vectors and causes flooding during the rainy seasons (Abubakar et al., Citation2022; Suryawan & Lee, Citation2023). By 2030, it is expected that Africa will have an urban population of over 50%, with an urban growth rate of 3.4% (Mohee & Simelane, Citation2015; Mombo & Bigirwa, Citation2017; Oyawole et al., Citation2016). The indiscriminate and improper dumping of municipal solid waste (MSW), particularly in water bodies and uncontrolled dump sites, worsens the problem of low sanitation levels across Africa (Gebreeyessus et al., Citation2019).
As shown in Figure below, waste generation in Sub Saharan Africa (SSA) is projected to nearly triple from 176 to 516 million tons per year if business continues as usual (Elisabeth Marie Constance, Citation2021). As cities become more developed and prosperous, citizens’ welfare improves, leading to an increase in consumption levels. Consequently, the volume of waste generated increases (Massoud et al., Citation2021).
Figure 1. Trend in global solid waste generation (in million tonns).

In Ethiopia, improper handling and disposal of solid waste is common in major cities. Waste is often dumped in unauthorized sites such as roadsides, drainage systems, and open spaces. Additionally, the amount of solid waste generated per capita in Ethiopia ranges from 0.28 to 0.83 kilograms per person per day (Gebreslassie et al., Citation2019; Mohammed & Elias, Citation2017). Unfortunately, Ethiopia lacks the financial resources necessary to improve sanitary facilities and institutional capacity for effective SWM (Getahun et al., Citation2012; Woretaw et al., Citation2017b). The collection and transportation of solid waste are the most crucial aspects of municipal SWM. They consume the majority of the municipal budget and have the greatest impact on urban life, placing a heavy burden on the country’s economy (Atinkut et al., Citation2020; Endalew et al., Citation2019). To mitigate these problems, the participation of local communities or service receivers is essential in finding solutions to SWM issues (Abate & Goshu, Citation2017; Bhattarai, Citation2015).
Gondar City, located in Ethiopia, is one of the fastest-growing cities in the region. However, it is currently facing the same problem as other rapidly expanding cities (Boateng et al., Citation2019; Maskey & Singh, Citation2017a; Selamawit; Mulat et al., Citation2019b). With an annual population growth rate of 6%, it is projected that the city’s population will double within 14 years (Belay et al., Citation2020; Bogale & Urgessa, Citation2012; Mezgebo & Ewnetu, Citation2015). This rapid growth has a significant impact on the generation and composition of solid waste (Alam, Citation2013; Chinasho, Citation2015).
However, SWM in Gondar City suffers from poor organization and relies on traditional methods of solid waste collection (Gedefaw, Citation2015). This problem primarily stems from the inability of cities and municipalities to keep up with the rapid pace of waste production (Gizaw et al., Citation2014). Furthermore, there is a lack of waste bins available, and the fees for waste collection services vary greatly. Waste operators charge households fees ranging from 2 to 15 ETB (US $0.10 to US $0.85) per week, depending on the size of the sack used for waste disposal (Gizaw et al., Citation2014; Mekuria et al., Citation2019). Despite this substantial share of SWM, municipalities are still not able to cover the total costs. Their financial capacities are limited and generally shared among other essential services (Olawade et al., Citation2023; Sharma & Jain, Citation2020).
It is essential to consider service users’ preferences in order to enhance the waste collection service, as the waste collection practice connects the service recipients and the service providers (Suryawan & Lee, Citation2023). Users’ positive attitudes and willingness to pay (WTP) for municipal SWM are crucial for ensuring the sustainability of service provision (Elisabeth Marie Constance, Citation2021). Additionally, Kaso et al. (Citation2022) reported that requesting service fees improves users’ waste management behavior, such as recycling, waste reduction, and segregation at the household level (Haileyesus Girma et al., Citation2022). Household WTP for waste management services depends on users’ preferences and participation (Kayamo, Citation2022; Lunojo, Citation2016). Therefore, the development and implementation of relevant SWM interventions and policies should take into account the behavior and readiness of the communities to contribute (Wang et al., Citation2014).
Non-market valuation methods can be used to determine the WTP for improvements in solid waste collection services (Nain & Kumar, Citation2023). The most commonly utilized non-market valuation method is the Contingent Valuation Method (CVM), which has been validated for use in developing countries (H. Alhassan et al., Citation2017; Suryawan & Lee, Citation2023). The CVM estimates the value of a good or service that has not been sold in the market by employing a survey-based approach (Mitchell & Carson, Citation2013). Factors that influence urban households’ WTP to improve municipal solid waste collection services have either very limited or non-existent in Gondar City. Understanding and designing effective policies and strategies that involve the active participation of residents is necessary due to budget limitations. Most studies on SWM in Ethiopia have focused solely on the country’s capital, Addis Ababa City (Amiga, Citation2002b), with only a few studies having been conducted in regional cities, such as Hawassa (Ayenew et al., Citation2019), Injibara (Selamawit Mulat et al., Citation2019b), Harar (Maskey & Singh, Citation2017a), Mekelle City (Dagnew et al., Citation2012), Bahir Dar City (Endalew et al., Citation2018), and Shashemene (Ayenew et al., Citation2019). Therefore, this study aims to fill these research gaps and provide valuable information on residents’ WTP that can inform SWM policymakers in Gondar City (see Table ).
Table 1. Summary of studies conducted on household’s willingness to pay to improve solid waste management services
2. Empirical literature review
3. Materials and methods
3.1. Description of the study area
The study was conducted in Gondar City, Amhara Regional State, which is located 739 km northwest of Addis Ababa, the capital city of Ethiopia. Gondar City is an ancient and historical city in Ethiopia, established by Emperor Fasiledes in 1636 (see Figure ). Currently, it serves as the capital city of the central Gondar zone. Gondar City is situated 738 km away from Addis Ababa and 182 km away from Bahir Dar City, the capital city of Amhara National Regional State. Its coordinates are 12°30’North and 37°20’ East, with an altitude of 1966 meters above sea level. The study area receives an average annual rainfall ranging from 1200 to 1600 mm and has a mean annual temperature of 26 °C (Gedefaw, Citation2015).
Gondar City is experiencing rapid expansion, with commercial centers, small industries, and residences scattered throughout all sectors of the town (See Figure ). Economically, it is one of the country’s surplus producing areas, with a high potential for sesame farming and market. Additionally, Gondar is a major center of tourism in Ethiopia, boasting attractions such as Fasiledes Castle and many historical churches. The city has a total population of 315,856, with 47% being male and the remaining 53% being female. In terms of religion, 84.2% of the population is Orthodox Christians, 11.8% are Muslims, and 1.1% follows other religions. The population density of the city continues to increase over time as rural residents migrate to urban areas.
3.2. Sample size and sampling technique
3.2.1. Sample size determination
The sample size was determined by considering resource constraints and the representativeness of the sample. Accordingly, the sample size of the study was calculated using the probability proportional to size (PPS) method by taking the population of the three Kebeles as the population for the study. This study applies a simplified formula provided by Yamane (Citation1967) to determine the required sample size at a 95% confidence level and a level of precision of 0.05. Through proportionate random sampling, 222 households were selected, and included in the study. This proportion was chosen because the estimated value of residents’ willingness to contribute to municipal SWM services in the study areas was unknown. The final sample size was determined to be 222 households. The number of households surveyed in each Kebele was determined using proportional allocation. A summary of the sample size from each Kebele is provided in Table .
Table 2. Sample size determination from sample kebeles and proportional sample size
3.2.2. Sampling procedures
This study used a cross-sectional design to evaluate the WTP of respondents for the improvement of SWM services. The study specifically targeted on households that had been living in their current location for at least one year, as most service fees, such as water supply and electricity, are paid at the household level (Delaney & O’Toole, Citation2006). The study population consisted of households in selected Kebeles, the smallest administrative divisions in Ethiopia. The choice of sampling technique, whether it is probability or nonprobability depends on the purpose and nature of the study. In this study, the objective was to estimate households’ WTP for improved SWM services and analyze the factors influencing their WTP. Therefore, a multi-stage random sampling technique was employed to achieve this objective. Initially, Gondar City was purposefully selected.Footnote1 Then, 21 Kebeles were classified into three strata based on the amount of household solid waste generated: high, medium, and low waste generation Kebeles (Sharma et al., Citation2011). While there may be general trends or similarities in waste generation patterns within a specific Kebele, it is crucial to acknowledge that individual households can vary significantly in their behaviors and waste production. Factors such as lifestyle, consumption patterns, and waste disposal habits can differ among households, even within the same Kebele. One sample Kebele was randomly selected from each stratum using simple random sampling, resulting in a total of three sample Kebeles. Finally, 222 urban households were randomly selected and allocated to the sample Kebeles using a probability proportional to the sample size. The random sampling method was used to select sample households from the sampling frame.
3.3. Elicitation method
According to Hoyos and Mariel (Citation2010), the economic valuation of environmental resources using stated preference information is known as the CVM. The CVM is one of the most advanced and commonly used techniques for valuing environmental resources (Jantzen, Citation2006), but this method is viewed with some skepticism, especially in situations where multiple options and several attributes are being considered. When designing a contingent valuation survey, it is important for the proposed scenario to clearly explain the characteristics of the goods and services being valued. This will enhance the credibility of the survey and ensure reliable results (Kwak et al., Citation2013). The questionnaire format should include the following sections: introductory questions about SWM service provision; WTP questions regarding the SWM service connection; and questionnaires gathering respondents’ information, including socioeconomic and demographic characteristics (Cameron & Quiggin, Citation1994a).
The CVM is a commonly used non-market valuation method for determining the economic value of environmental commodities. It can also be used to assess WTP for improvements in solid waste collection service. The most widely used non-market valuation method is the CVM, which has been validated for its application in developing countries (Alpizar et al., Citation2003). In the dichotomous or closed-ended format, respondents are asked to provide a yes or no answer to the question of whether they are willing to pay a certain amount (e.g. are you willing to pay X birr?”). If the answer is yes, a new question with a higher value for X is asked, and if the answer is no, a new question with a lower value for X is asked (Cameron & Quiggin, Citation1994a; Hoyos & Mariel, Citation2010). Table presents commonly used elicitation formats for WTP estimation. The CVMFootnote2 typically consists of a series of steps. First, the current state of waste management is described. Second, a scenario for a hypothetical market is formulated. The scenario includes describing the baseline (or status quo) condition (s), as well as the proposed change (s), in a simple, meaningful, and understandable way (Johnston et al., Citation2017). In this study, we formulated a hypothetical scenario called the ‘Gondar City SWM improvement program’ in the future that could be implemented by Gondar city municipality or other concerned bodies. The hypothetical program intends to increase the waste collection frequency and improve the waste disposal system from the current condition (status quo). Respondents were informed by stating, ‘ … the program would work to collect solid waste two times a week (house-to-house) and safely dispose of it into a waste disposal site outside the city to reduce health impacts related to poor urban SWM services’. If the new management plan (Option 2) below is the only possible alternative to the current waste management practice (Option 1). Do you prefer to choose Option 1(collection frequency, once weekly but irregular, no separation of waste at source needed, etc.), or Option 2 (collection frequency—once weekly every alternate days, separation at source needed but containers are provided free of charge, etc.)?
Table 3. The contingent valuation questionnaire
The payment is a service fee for solid waste collection services. The hypothetical market assumes that each response to hypothetically stated questions is comparable to the individual response to the actual market (Mitchell & Carson, Citation2013). The valuation exercise begins by asking respondents whether they are willing to pay using a dichotomous question (yes or no). Then, for those who answer ‘yes’, the double-bounded choice format ask how much they are willing to pay for the scenarios described in the hypothetical market (Cawley, Citation2008). Respondents who answered ‘no’ are then asked to explain why in a follow-up question. Please refer to Tables for the contingent valuation questionnaire used in the study.
Table 4. Examples of commonly used elicitation formats for WTP estimation
The study utilizes the double-bounded dichotomous choice (DBDC) elicitation format, recommended by (Cameron & Quiggin, Citation1998; Haab, Citation1998; Hanemann et al., Citation1991). In this format, each respondent is presented with a series of two bids and asked the question twice. The second question depends entirely on the response to the first question. Based on the initial response, respondents are then asked for new bids. If their initial response was ‘yes’, the new bids are higher, and if their response was ‘no’, the new bids are lower. Additionally, the increased number of responses allows for a better fit of the given function due to more observations. This study applied a DBDC format, as it efficiently elicits more information about respondents’ WTP (Haab & McConnell, Citation2002).
This study applied a double-bounded dichotomous choice format, as it efficiently elicits more information about respondents’ WTP. The DBDC formats used in the study are summarized in Figure above. Participants who answered no in the survey are required to provide their reasons for not being willing to pay, and these reasons are recorded in an open-ended format. The follow-up questionnaire helps identify the respondents who protested and were excluded from our estimation of the WTP model in the later section.
In a DBDC question, the individual was presented with a first bid and asked whether they would pay this price for the new urban SWM program considering their maximum subjective value. If the answer was yes, then a second higher bid was presented. If the answer was no, then a lower-second bid was presented.
A CVM using a DBDC format was used to elicit respondents’ WTP under a hypothetical scenario of improving the SWM system, particularly the collection service. This method produces four possible outcomes (Table ).
Table 5. Double-bounded dichotomous choice format to elicit WTP to improve SWM services
3.4. Data collection methods
Three professional enumerators were hired to collect households’ WTP for improved SWM service and its attributes using multistage sampling techniques. Face-to-face interviews were chosen as the preferred data collection method due to their ability to provide clarity and focus, reduce non-response rates, and ensure data completeness (Jianjun et al., Citation2016). During interactions with respondents, we explained the purpose of the study and assured them that their responses would be kept confidential. Consent and willingness to participate in the survey were obtained. Additionally, this study adheres to proper citation and crediting of all materials used and avoids improper paraphrasing.
To obtain initial estimates of the distribution of WTP, a pilot study was conducted. This involved determining initial bid values and checking for appropriateness and ambiguities in the study materials. Pre-test surveys were conducted with a total of 20 respondents. The questionnaire was also translated from English to the local language (Amharic) to ensure clear understanding for both enumerators and respondents. This study utilized a cross-sectional design to estimate respondents’ WTP for improved SWM services. Data for the study were collected from both primary and secondary sources. Qualitative primary data were gathered through face-to-face interviews, and key informant interviews were also conducted as part of the data collection method. The CVM typically consists of a series of steps. The questionnaire has two main sections. The first section includes socioeconomic and demographic questions, while the second part includes the valuation exercise. Questionnaires are designed to obtain the most precise data for econometric analysis of households’ WTP, determinants of WTP, and the amount of WTP, as well as assess SWM. Moreover, secondary data were collected from published and unpublished documents.
3.5. Method of data analysis
3.5.1. Descriptive statistics
Descriptive statistics (percentages and frequencies, means and standard deviations) and an econometric model were employed to analyze the collected data using statistical software (STATA version 15). These analyses were conducted after appropriate coding, editing, and registration of the collected data. The data was then the data entered into SPSS software.
3.5.2. Econometrics model specification
In most CVMFootnote3 studies, it is common to directly inquire about respondents’ WTP. However, using an open-ended method to ask about the payment amount is not recommended. This approach often leads to missing values as respondents find it difficult to provide an answer (Adamowicz et al., Citation1993).
This study applied a DBDC format, as it efficiently elicits more information about respondents’ WTP. In a DBDC question, the individual was presented with a first bid and asked whether they would pay this price for the new municipal SWM program when considering their maximum subjective value. If the answer was yes, then a second higher bid was presented. If the answer was no, then a lower second bid was presented. To identify the factors that affect the WTP of urban households’ for improving urban SWM services, a multivariate probit model was preferred over other econometric models such as multinomial logit, binary logit, and probit due to its advantages. Depending on the assumptions about the average values of error terms in relation to the individual covariates, several models can be used, including the bivariate probit model (Entele, Citation2020; Lee & Heo, Citation2016), the random effects probit model Lee and Heo (Citation2016) and the interval-data logit model (Cameron & Quiggin, Citation1994b; Hanemann et al., Citation1991). Additionally, Alberini (Citation1995) suggests that the estimate of the bivariate probit model is preferable to the interval data logit model when the correlation coefficient between the two consecutive bid error terms is close to zero.
The multivariate probit approach allows for the simultaneous modeling of the influence of explanatory variables on the choice of urban households’ WTP while also accounting for potential correlations between unobserved disturbances and the relationships between the choices of different bid values. Multinomial models are appropriate when individuals can choose only one outcome from a set of mutually exclusive alternatives. However, in this study, urban households’ WTP is not mutually exclusive, considering the possibility of simultaneous WTP choices and the potential correlations among these choices. The Tobit model is an alternative to other linear regression models like Ordinary Least Squares (OLS) when the dependent variable is not fully observed. This is because the OLS would give inefficient and inconsistent estimates. The multivariate probit model is preferred and applied in this study because of the assumption of independence of irrelevant alternatives (IIA). This allows for the capture of household variation in the choice of market outlets and the estimation of several correlated binary outcomes jointly (Bhatti & Al-Shanfari, Citation2017).
To identify the factors simultaneously determining the two WTP dependent variables, a seemingly unrelated (SUR) bivariate probit model for an individual i can be specified as follows by (Haab & McConnell, Citation2002).
Where; ith respondent’s, WTP
= are unknown parameters to be estimated in the first and second responses respectively,
= Explanatory variables that can affect amounts of offered prices WTP for improved solid waste management services, WTP1, WTP2 = unobservable random components in the first and second responses respectively,
and
, are normally distributed with mean zero and respective variances
and
, and have a bivariate normal distribution with correlation coefficient
.
According to (Entele, Citation2020); and Lin et al. (Citation2013), these four types can be expressed by the following equations.
Where; h1 =first bid price (initial bid) and h2 = second bid price (final bid or Bid2)
3.5.3. Estimation of the mean willingness to pay
After running a regression of the dependent variable of two equations (yes/no indicators), on a constant and independent variables consisting of bid levels, the mean WTP value was calculated using the approach developed by the formula specified by (Haab and McConnell (Citation2002).
Where = is the coefficient for the constant term and
= is a coefficient offered bids to the respondents
For open-ended contingent valuation survey responses, the maximum WTP figures reported by the respondents can simply be averaged to produce an estimate of mean WTP. The formula for calculating mean WTP is as follows:
MeanWTP
Where: n is the sample size and y, is the maximum WTP amount reported by households; yi is the maximum amount an individual is willing to pay for the proposed improvement
4. Result and discussions
4.1. Descriptive statistics
4.1.1. Socioeconomic and demographic characteristics of households
Out of the total 222 respondents, 2 observations were eliminated as invalid responses. The analysis in this research article was based on the remaining 220 sample units. Among the 220 remaining sample respondents, 100 (45.45%) were from male-headed households and 120 (54.55%) were from female-headedFootnote4 households. This may be due to the fact that women traditionally have more responsibility for SWM in the household.
Of all the respondents, 192 (87.27%) households were willing to pay for improved SWM services, while 28 (12.73%) households were not willing to pay. Among the non-willing to pay respondents, 22 (78.6%) were from male-headed households and 6 (21.4%) were from female-headed households (see Table ). This finding suggests that sex is an important variable in the WTP decisions of households because traditionally, females are more concerned about their environment than males due to their responsibility for general hygiene in the house.
Table 6. Descriptive statistics of socioeconomic and demographic characteristics of households
On average, the sampled household heads have 5 years of schooling. According to the categorical form summarized in Table , 19.09% of household heads have no formal education (illiterate), 4.09% have basic education, 12.27% have completed primary schooling, 28.64% have attained secondary schooling, and 35.91% have a certificate or above level of education. Regarding marital status, out of the total household heads surveyed, 145 (65.91%) were married, while 49 (22.27%), 16 (7.27%), and 10 (4.55%) were single, divorced, and widowed, respectively. Furthermore, out of the 195 respondents who were willing to participate, 132 (68.75%) were married, while 21.35%, 6.25%, and 3.65% were single, divorced, and widowed, respectively (see Table ).
In terms of access to SWM services once per week, only 153 (69.55%) household heads currently receive SWM services, while 67 (30.45%) household heads do not receive SWM services (see Table ).
To assess the level of awareness and understanding among households regarding solid waste and its management, selected samples were asked about their perception of solid waste. According to the data collected from 220 respondents, 50% stated that solid waste is completely useless. Additionally, 25% of the selected households believed that solid waste was somewhat useful, while the remaining 25% considered it to be useful.
The survey results show that 80 (36.36%) of the household heads experienced health problems due to improper SWM services, while 140 (63.64%) did not. Out of the 192 respondents who were willing to pay for an improved SWM program, 60.94% of households were not affected by any diseases caused by improper SWM practice. Among the 28 respondents who were not willing to pay, 39.06% were affected by diseases (see Table ). However, this study’s results align with a study conducted in Jimma town, Ethiopia, by Batu and Fikadu Tolosa (Citation2016), which reported a prevalence of 83.6%. The results are also higher than studies conducted in Tanzania by Mussa (Citation2015), South-east Nigeria by Oyawole et al. (Citation2016), Ghana by Awunyo-Vitor et al. (Citation2013), Nepal by Maskey and Singh (Citation2017a), Ethiopia (Addis Ababa city) by Amiga (Citation2002a), and (Injibara town) by Selamawit. The possible reason for these differences could be variation in study areas, periods, designs and demographics.
Regarding house ownership, 58 (23.18%) of households live in their own houses, while 169 (76.82%) live in rented houses. Additionally, out of 192 willing-to-pay respondents, 45 (23.44%) lived in their own house, while 147 (76.56%) lived in rented houses (see Table ). In terms of the age variable, the mean age for willing-to-pay household heads is 38.2 years, while for non-willing-to-pay respondents it is 41.5 years (Table ).
The average family size for both willing-to-pay and non-willing-to-pay respondents is 4.58, with a minimum of 1 and a maximum of 9 family members. The mean family size for willing-to-pay household heads is 4.68, while for non-willing-to-pay it is 3.928. The overall sample household mean family size is 4.58, which is lower than the national mean family size (Gashaw et al., Citation2014). This may be because household heads were not interested in disclosing their family size (see Table ).
Table 7. Descriptive statistics of socioeconomic and demographic characteristics of households
As indicated in Table , the mean income of the respondents was 4,451.76 ETB per month. The minimum and maximum aggregate income for both willing-to-pay and non-willing-to-pay respondents was 500 ETB and 15,000 ETB, respectively. Additionally, the mean income of willing-to-pay respondents was 4,695.51 ETB per month, while for non-willing-to-pay households; it was 2,780.36 ETB per month (see Table ).
The average length of stay in the town is 17.8 years, according to the survey data. Furthermore, the data reveals a strong correlation between the age of the household head and their duration of residency in the town. In other words, the older the household head, the longer they tend to stay in the city.
When examining the average years of stay for households that are willing to pay and those who are not willing-to-pay for improved SWM services, the data shows that the former group has stayed in the city for an average of 18.56 years, while the latter group has stayed for an average of 16.82 years. This suggests that there is not a significant difference in the length of stay between the two groups (Table ).
On average, each household generated 0.92 ‘Madaberiya’ per week, with amounts ranging from 0.25 to 3.0 ‘Madaberiya.Footnote5 This applies to both willing and non-willing households. Additionally, willing-to-pay household heads generated an average of 0.99 ‘Madaberiya’ of solid waste per week, while non-willing-to-pay household heads generated an average of 0.455 ‘Madaberiya’ of solid waste per week (Table ).
4.1.2. Households’ willingness to pay for improved solid waste management services
Households were then asked if they were willing to pay for improved urban SWM services. Out of the total sample of 220 households, described in the previous section, 192 (87.27%) were willing to support the government in financing the provision of improved SWM services. On the other hand, 28 (12.73%) households were not willing to contribute to the cost sharing for the planned service improvement.
Table below shows the WTP for households belonging to different area stratifications, based on criteria explained in the previous sections. Out of the total sample households, 192 were willing to pay for improved SWM. Among these willing households, 23, 51, and 118 were from low, medium, and high solid waste-generating Kebelles, respectively.
Table 8. Willingness to pay for improved solid waste management services in Gondar City
Based on the number of households unwilling to cooperate with the government through cost sharing for improved solid waste management, it was found that the percentage of households living in low-waste-generating Kebelles (34.29%) was greater than those in medium-waste-generating Kebelles (20.21%) and high waste generating Kebelles (2.48%). Additionally, the percentage of households in medium solid waste generating Kebelles (20.21%) was greater than those in high solid-waste-generating Kebelles (2.48%).
On the other hand, approximately 12.73% of households were unwilling to pay for the solid waste management improvement program. A follow-up question was then asked of the respondents to state their reasons for being against the program, with most stating they could not pay due to budget constraints. The responses can be found in Table .
Table 9. Ranking of reasons of unwilling to pay
The table above shows that out of the 28 households unwilling to pay, 11 (39.29%) believed they generated less waste and could manage it themselves. 7 (25%) of the households unwilling to pay stated they couldn’t afford it. 6 (21.43 %) said SWM is the municipality’s duty, and 4 (14.28%) were satisfied with current services and didn’t see a need for improvement.
Moreover, based on this data, we can conclude that a significant number of households are willing to pay or have no objections to waste management because they produce less waste and feel capable of handling it on their own. This indicates that they may not fully appreciate the importance of a clean environment or do not prioritize it, despite the fact that their waste has environmental consequences. As a result, there is a need raise create awareness among the population.
As mentioned earlier, out of the total sample size of 222, 2 observations were excluded due to invalid responses. Among the remaining 220 observations, 28 (12.73%) are true zeros. Of these, 12 come from areas with low solid waste generation, while 13 and 3 are from areas with medium and high solid waste generation, respectively.
According to Banga et al. (Citation2011), sample units that were unwilling to participate due to monetary reasons were removed from the bivariate probit analysis. Therefore, we analyzed the factors influencing households WTP for improved SWM using the remaining 192 (87.27 %) households that provided positive WTP responses, ranging from 3 to 50 ETB per month.
The distribution of the DBDC response aligns with the law of demand theory. This means that the percentage of no-no responses increases as the initial bid price faced by respondents rises. As expected, higher prices lead to lower acceptance rates by respondents. Table illustrates that the percentages of no-no responses increase with the initial bid price for both electricity goods, while the percentages of yes-yes responses decrease.
Table 10. Household responses to the hypothetical improved solid waste management program
Table illustrates that approximately 28 households (12.73%) rejected both the initial offers and the follow-up discounted bids (no-no). Out of the 192 respondents who stated their WTP, 21 respondents (9.55%) did not accept the initial bid but accepted the second discounted bid (no-yes). The third category included 59 households (26.81%) that accepted the initial bid but rejected the higher second follow up bid (yes-no). Lastly, the last category represented households who responded positively to both the initial bid and the higher follow-up bid (yes-yes), totaling 98 households (51.04%). This indicates that the response to the first bid influenced the response to the follow-up question, which aligns with previous studies like Solomon (Citation2004) on the valuation of multi-purpose tree resources using a DBDC elicitation format and Banga et al. (Citation2011) on WTP for rural water supply services using a DBDC elicitation format.
4.1.3. Estimation of mean willingness to pay for improved solid waste management services
Both open-ended and DBDC methods were used to calculate the mean WTP for better SWM services. In the open-ended format, the question posed was, “What is the maximum amount you are willing to pay for better SWM services? The results from the open-ended contingent valuation revealed that households were willing to pay anywhere from 0 to 50 Ethiopian Birr (ETB) for better SWM services. The calculated mean and median WTP values were 17.077 ETB ($058) and 15.25 ETB ($0.52), respectively.
These findings suggests that the average WTP of the respondents is significantly higher than the current SWM service charge of 7 ETB per month, indicating a potential mismatch between the current charge and the demand. Therefore, implementing a demand-based SWM service could potentially boost the municipality’s revenue and enhance satisfaction among urban households. Consequently, it is recommended that a demand-based SWM service be developed and implemented in Gondar city.
The results of this study were lower than those reported in a study conducted in Nepal ($0.72) by Maskey and Singh (Citation2017a), which found WTP values of 29.7 ETB and 11.89 ETB by Mulat et al. (Citation2019). However, they were higher than the WTP values reported in a study conducted in Ethiopia (Addis Ababa and Mekelle), which found values of 7.07 ETB and 11.89 ETB by Amiga (Citation2002a).
Another interesting result is that the error correlation is estimated to be 0.89, justifying the use of the bivariate probit model. The success probability of WTP only in the first response (WTP1) by the household head is about 86.78%. Similarly, the probability of WTP only in the second response (WTP2) by the household head is about 57.96%. The joint probability of success, where household heads WTP to both responses (WTP1 and WTP2) simultaneously, is not much different from the probability of WTP only in the second response (WTP2), at only 57.85%. On the other hand, the joint probability that household heads fail to WTP in both responses is about 13.11%, suggesting that they are more likely to fail to WTP both responses (WTP1 and WTP2) simultaneously (Table ).
Table 11. Parameter estimates of bivariate probit model from double bounded dichotomous choice format
In addition to the open-ended format, the mean WTP from the DBDC format was computed following the bivariate probit estimation. The likelihood ratio test of the model confirms the interdependence between the two probit equations at a significance level of less than 1%. This indicates that the two equations were estimated concurrently. As the model results indicated, both the initial and follow-up bids had statistically significant and negative effects on responses to the stated bids at a significance level less than 1%. This implies that an increase in the amount of bids decreases the respondents’ WTP. Using these coefficients in Table , the mean WTP for improved SWM service was estimated using the formula by Haab and McConnell (Citation2002). Thus, the mean of the WTP estimate using Bid1 and Bid2 was 28.74 and 40.22 ETB per month, respectively. On average, for both bids, the mean WTP was about 34.48 ETB per month, which is greater than the mean WTP from open-ended elicitation.
4.1.4. Estimated total willingness to pay
The total amount households are willing to pay (aggregate benefits) to improve solid waste management is used as a measure of the value of the cost of poor solid waste management to the city. Therefore, how much households are willing to pay represents the economic cost of services improvement in the city. The results of the open-ended questions were categorized into class marks following the steps to convert ungrouped frequency distribution data into a grouped frequency distribution. The class mark of each class limit was calculated using statistical procedures. To arrive at this, the mean WTP from the sample was extrapolated across the population. Using the population mean allows us to extrapolate the estimates for the entire population. Therefore, the estimated aggregate WTP for Gondar City is shown in Table . In the estimation we used the following two steps:
Table 12. Total monthly willingness-to-pay for improved solid waste management services
To estimate the number of households willing-to-pay in each interval, we multiplied the percentage of households in each interval by the total number of households in the city (Column 5 of Table ), resulting in 55,129 willing-to-pay households in the study area. Based on national census data, the city has a total population of 63,171 and 87.27% of the sample have been willing to pay for an improved SWM program. Therefore, the total number of households that are willing to pay = 63,171 × 0.8727 = 5,5129.
The results of the study show that 192 (87.27%) of the households are willing-to-pay for improved SWM services, while the remaining sample households are not 28 (12.73%). The midpoint of each WTP interval is the mean WTP of each row calculated by the sum of lower and upper bound and dividing by two (column two). When we multiply the number of households by this mean WTP, we can estimate the total WTP of each row (column six of Table ). Out of the total households in the study area, approximately 8,042 = 0.1273 × 63,171 (are not willing to pay for better SWM service provision by the city municipality.
The total number of households (Column 5) is obtained by taking the proportion of sample households falling in that boundary and multiplying it by the total number of households. To calculate the total willingness to pay (Column 6), we multiply the class mark (Column 2) by the total households willing to pay that amount (Column 5). The total monthly WTP of the city is estimated using the midpoint WTP. The total number of households (in Column 5) is calculated by multiplying the proportion of sample households within that range by the total number of households willing to pay (55,129).
The total amount that willing households are willing to pay (Column 6) is calculated by multiplying the midpoint WTP (Column 2) by the total number of willing households (Column 5). The overall total WTP (941,361 ETB)Footnote6 is obtained by adding up the total amounts at each class mark. This is the amount that all households in Gondar City are expected to pay per month if the proposed plan for improved SWM is implemented. Annually, this amounts to 11,296,332 ETB (941,361 ETB multiplied by 12).
These calculations are presented in the tables below, which include the demand curve.Footnote7 For instance, Haji and Tesfaye (Citation2014) estimated the total WTP of farm households for the restoration of Lake Haremaya. The author followed similar steps to estimate the total WTP, but she included all observations for estimation. However incorporating both willing and unwilling respondents may overestimate the total WTP. On the contrary, this study only considered willing sample households to estimate the total WTP. Similarly, Endalew et al. (Citation2018) estimated the total WTP based on the proportion of willing households.
Table provides information on the percentage of households from the sample willing to pay by income group established in this study, the corresponding total number of households in the population by solid waste generating group, the number of households from the population willing to pay by solid waste generating group, mean WTP by solid waste generating group, and the expected revenue (in ETB) by solid waste generating groups.
Table 13. Estimated total willingness to pay from open ended and double bounded format
For example, to achieve an aggregate monthly revenue of ETB 361,599 for the high solid waste generating group, we first calculate 61.46% of the total number of households in the population from that specific solid waste generating group (since the study indicates that 61.46% of households from the high solid waste generating group are willing to pay). In this study 34,932 households belong to the high solid waste generating group, and 61.46% of 34,932 is 33,883. This means that 33,883 households from the high solid waste generating group are willing to pay if we consider the total population. By multiplying this number 33,883, by the mean WTPETB 34.48 (see Table ); we obtain the monthly expected revenue from that solid waste generating group which is ETB 1,168,286. This method can be used to calculate the expected revenue from other solid waste generating groups. By summing the expected revenues from the three different solid waste generating groups we arrive at aggregate revenue of ETB 1,900,848. Since households are not charged for waste disposal based on willingness and ability to pay, the estimated aggregate revenue serve as a proxy for the potential revenue that can be generated from user charges.
The main objective of this section is to compare the total amount of WTP obtained from the open-ended and DBDC methods. The grand total WTP was then calculated by summing the WTP of all households in each stratum. In terms of cash payment, the total WTP is 941,361ETB for the open-ended format and 1,900,848 ETB for the DBDC format (see Table ). This indicates that the DBDC format provides better results compared to the open-ended contingent valuation format.
4.2. Analysis factors associated with willingness to pay for improved solid waste management
Among the ten explanatory variables used in the first and second response regression six variables were found to be statistically insignificant. Additionally, the significant variables are discussed in Table .
Table 14. Bivariate probit regression results
4.2.1. Age of the respondent (AGEHH)
The coefficient of age is negative, as expected, and is significant at 5% and 10% probability levels in both the first and second responses. The result indicates that young people are willing to pay a higher amount for improved SWM services compared to older individuals. This may be because older people are less informed about environmental topics compared to younger people. It may also suggest that younger people are more concerned about the aesthetics of their city.
The marginal-effect results also show that an increase in the respondent’s age by one year will decrease the probability of WTP for the given amount of the offered price by 1.28% in both the first and second responses, holding other variables constant (see Table ). This negative sign confirms the findings of Lunojo (Citation2016), Adenike and Titus (Citation2009), and Adebo and Ajewole (Citation2012) who also found that age is an important factor in determining households WTP for improved SWM services.
4.2.2. Family size of the household (FAMSIZE)
The estimated coefficient for the family size of the household does not show a clear effect on the amount of the offered price that individuals are willing to pay in their first response. However, it is negative and significant at a 10% probability level for the second equation (see Table ). Moreover, the marginal effect clearly shows that the family size of the household has a negative and significant effect on the amount individuals are willing to pay for improved SWM service provision.
The marginal effect result indicates that as the total family size increased by one person, the probability of the household’s WTP at a given offered price (bid value) decreased by 3.09%, holding other variables constant. This is the reason why households with larger family sizes are willing to pay a relatively smaller amount, due to associated high running costs (i.e. budgetary constraints) (Table ). Similar findings have been observed in studies conducted in different parts of the world, such as Ethiopia (Oyawole et al., Citation2016), Nepal (Maskey & Singh, Citation2017a), and Ghana (Awunyo-Vitor et al., Citation2013). This may be because respondents with larger family sizes have a greater demand for SWM and are willing to pay more.
4.2.3. Total household income (HHINCOME)
The estimated total household income has a positive sign as expected, and is statistically significant at the 1% probability level in the first and second responses, respectively. This shows that the demand for improved SWM services moves in the same direction as changes in income. Therefore, income is a strong determinant variable in explaining, WTP and, any attempt to introduce user charges for the provision of the service should take into account the ability of the users in addition to their WTP.
The marginal effect result also indicates that households with higher incomes are willing to pay more for improved SWM services compared to those with lower incomes. If the income of the household increases by 1%, the probability of being willing to pay the offered price will increase by 0.0064%, assuming all other factors remain constant. This finding is consistent with the general demand theory, which states income is positively related to the demand for normal goods (see Table ). The results align with the findings of Yusuf et al. (Citation2007b), Ezebilo (Citation2013), and Rahji and Oloruntoba (Citation2009),which also showed a positive correlation between income and respondents WTP. Theoretically, they explained that the participation of people in SWM in higher-income areas is high, while the participation in lower-income areas is low. These results demonstrating, the correlation between income and participation in improved SWM services. This result is also in line with the theory and other research findings. According to Kassa and Teshome (Citation2016) contractors with higher paid-up capital, indicating higher income returns, showed a higher WTP for improved SWM services. This indicates a positive relationship between income and WTP for improved SWM services.
4.2.4. Education status of the household head (YEARSEDUC)
Among the five categories of educational status in the sample households, the illiterate group is used as the control group. The remaining four educational dummies show the expected positive sign. The religious-based education dummy has a positive sign but is not significant in the first and second responses, indicating that it is not a major determining factor for WTP in the analysis. On the other hand, household heads with primary education status (1–8) have a significant effect at a 10% probability level in the first responses, while those with secondary education (9–12) have a significant effect at a 5% probability level in the first responses. Furthermore, household heads with certificates and above education status have a positive and significant effect at a 5% and 10% probability level in the first and second responses, respectively. This suggests that higher levels of education lead to a higher WTP for a given amount of the offered price.
The marginal effect results indicate that, as compared to the base category (illiterate) and holding other variables constant, having a certificate and above education status would increase the probability of willing-to-pay for the given amounts of bid values for improved SWM service provision by 11.06% (Table ). This finding confirms the results of other studies conducted by Amiga (Citation2002a), Yusuf et al. (Citation2007b), Anjum (Citation2013), and Rahji and Oloruntoba (Citation2009), which show that individuals with higher levels of education are willing to pay higher values for improved SWM services. One possible explanation for this is that educated individuals can easily understand the consequences of waste mismanagement.
4.2.5. Marital status of the respondent (MARITSTA)
Among the four categories of marital status, the married group is used as the base category. Among the remaining three marital status dummy variables, only marista2 (single) household head is not statistically significant in the first response. However, in the second response, it has a negative sign as expected and is statistically significant at a 10% probability level for the given amounts of offered price (bids) for improved SWM services. The remaining two marital status dummy variables are not statistically significant. Therefore, marital status dummy variables (marista3 and marista4) do not significantly influence a household’s WTP for improved SWM services.
The marginal-effect results also indicate that all marital status dummy variables do not have a clear effect on WTP compared to the base category (married) when keeping the influence of other variables constant (see Table ).
4.2.6. The initial bid (Bid1)
It has a negative coefficient and is statistically significant at a 1% probability level in the first response. This means that the Bid1 coefficient indicates a negative correlation with the probability of accepting the amount of Bid1 in the first response, as expected. It means that as the amount of the offered price (Bid1) in the first response increases, the respondents will be less willing to pay for it. This is consistent with the law of demand.
However, the marginal effect does not clearly show the effect of Bid1 in the first response on the amount of the offered price for WTP for improved SWM service provision (see Table ).
4.2.7. The second bid (Bid2)
It has a negative coefficient as expected and is statistically significant at the 1% probability level for the follow-up question or in the second response. As the amount of Bid2 increases, respondents show a lower WTP, which is consistent with the law of demand. Additionally, the marginal effect in the second response reveals that if the offered price (Bid2) to the household increases by 1%, the probability of the WTP amount decreasing by 1.18% remains constant while keeping all other factors the same (Table ).
5. Conclusion and policy implications
This study aimed to determine households’ WTP for an improved SWM system in Gondar City using a double bounded contingent valuation followed by open ended questions. The results indicated that 192 (87.27%) of the sample respondents were willing to pay for improved SWM services, with a mean WTP of 17.0756 ETB for the open-ended format and 34.48 ETB for double bounded CVM format. Therefore, the double bounded format provided better results than the open-ended format. Consequently, more attention should be given to the double bounded format to elicit respondents’ WTP for better SWM services. Additionally, the results imply that the average WTP of the respondents is much higher than the current SWM service charge. Therefore, it would be better to design and implement an SWM service that collects more funds and delivers better service for the residents of Gondar City.
The estimated total monthly aggregated WTP of the city is 941,361 ETB for the open-ended format and 1,900,848 ETB for the double bounded format. The actual WTP of households in Gondar City may fall between these two figures. This WTP is much higher than the current sanitary fees. The citizens are eager for improved SWM, so there is potential to increase the fee and acquire sufficient funds to substantially improve and modernize SWM in Gondar City. To determine a more appropriate sanitation fee, municipal officials can compare the mean WTP to what a private solid waste collector currently charges a household for its service (7 ETB per month).
To ensure sustainable SWM services, reduce pollution and minimize health impacts through environmentally sound waste management throughout the product life cycle, this study provides various recommendations and policy implications to improve the situation in the study area.
The government and other stakeholders interested in waste management should invest more in education and awareness about the importance of waste management for the general public. This can be done through various mechanisms including social media. Additionally, there should be a mechanism in place to charge waste fees based on weight to avoid the issues of free riders.
The economic status of households, specifically their income, was found to be statistically significant and linked to their WTP for improved SWM. Households with unreliable sources of income are less likely to pay for better SWM services. Therefore, authorities should pay special attention to implementing strategies, which cater to the needs of low-income groups.
Compared to the current sanitary fees, the WTP for improved SWM is much higher. This indicates that citizens are eager for better SWM services, providing an opportunity to increase fees and acquire sufficient funds to substantially improve and modernize SWM in Gondar City. Municipal officials can use the mean WTP as a starting point to determine a more appropriate sanitation fee.
Community participation in terms of service charges can be a sustainable financing method and help avoid the free-rider problem. To achieve this, a socially acceptable fee should be set, which the majority of people are willing to pay. Therefore, the local government must work on convincing and engaging the community to pay a service fee for house-to-house waste collection, which can alleviate financial constraints.
Acknowledgements
The authors thanks the enumerators, and Gondar city residents and the city municipality for their genuine contribution during cross sectional survey data collection
Disclosure statement
The writers attest that they have no known financial conflict of interest that could have seemed to influence the work reported in this manuscript
Notes
1. Because it is the place where solid waste is a serious problem from time to time, there is a lack of background information about households’ WTP and its determinants for improved SWM services, an increase in solid waste disposal-induced problems in the city, and a high rate of migration from rural areas in all directions. These factors increases pressure to manage solid waste effectively in order to avoid outbreaks of diseases.
2. There are different elicitation methods that can be used in a CVM application, and the choice of a technique depends on the type of resource being valued and the characteristics of the sample.
3. CVM is a method that uses hypothetical survey questions to elicit people’s preferences for public good by finding out what they are the willing to pay for specified improvements in them finding out what they are willing-to-pay for specified improvements. The design of the CVM questionnaire used to elicit the WTP of respondents was done following the recommendations of the NOAA panel.
4. Not all of the female respondents were heads of households. Some were wives and others were elders interviewed when the head of the household was not available for the interview.
5. A material that looks like suck and used is to store solid waste at the household level.
6. Note that the total monthly WTP of the city can be calculated using the open-ended elicitation format, i.e. the maximum WTP of the respondents, as follows: using one of the aggregation methods of WTP. First, i.e. before the aggregation of benefits, class boundaries for the results of the open-ended questions are set. Then, the mid-WTP or class mark is determined. That is, mid-WTP or class mark is the average of the WTP interval or class boundaries. The total WTP for the class is derived by multiplying the mid-WTP or class mark by the total number of households in the class. Then this is aggregated across all classes.
7. ‘Demand’ - The desire and ability to acquire a good or service, or the quantity of a good or service those economic agents are willing to buy at a given price.
References
- Abate, B., & Goshu, G. (2017). Waste management in lake tana basin—case of rapidly urbanizing Bahir Dar city. In Social and Ecological System Dynamics: Characteristics, Trends, and Integration in the Lake Tana Basin (pp. 581–23). Springer.
- Abed Al Ahad, M. J. (2018). Factors associated with people’s willingness to pay for better solid waste management services in Lebanese rural areas: The case of Jdeidet Ghazir.
- Abubakar, I. R., Maniruzzaman, K. M., Dano, U. L., AlShihri, F. S., AlShammari, M. S., Ahmed, S. M. S., Al-Gehlani, W. A. G., & Alrawaf, T. I. (2022). Environmental sustainability impacts of solid waste management practices in the global south. International Journal of Environmental Research and Public Health, 19(19), 12717. https://doi.org/10.3390/ijerph191912717
- Adamowicz, W. L., Bhardwaj, V., & Macnab, B. (1993). Experiments on the difference between willingness to pay and willingness to accept. Land Economics, 69(4), 416. https://doi.org/10.2307/3146458
- Addai, K. N., & Danso-Abbeam, G. (2014). Determinants of willingness to pay for improved solid waste management in Dunkwa-on-Offin, Ghana. Journal of Agriculture and Environmental Sciences, 3(1), 01–09.
- Adebo, G., & Ajewole, O. (2012). Gender and the urban environment: Analysis of willingness to pay for waste management disposal in Ekiti-State, Nigeria. American International Journal of Contemporary Research, 2(5), 228–236.
- Adenike, A. A., & Titus, O. B. (2009). Determinants of willingness to pay for improved water supply in Osogbo Metropolis; Osun State, Nigeria. Research Journal of Social Sciences, 4(1), 1–6. https://www.researchgate.net/publication/283498209
- Akerele, D., Afolayan, S., Oyawole, F., & Sanusi, R. (2017). Socioeconomic determinants of food waste among households in Abeokuta, Ogun State, Nigeris. Nigerian Journal of Agricultural Economics, 7(2066–2018–1341), 25–35.
- Akhtar, S., Ahmad, A. S., Qureshi, M. I., & Shahraz, S. (2017). Households willingness to pay for improved solid waste management.
- Alam, P. A. K. (2013). Impact of solid waste on health and the environment. International Journal of Sustainable Development and Green Economics (IJSDGE), 2(1), 165–168.
- Alam, S., & Fatima, A. (2022). Analysing household willingness and ability to pay for solid waste: A case study of Karachi. Pakistan development review, 6(1), 169–184. https://doi.org/10.30541/v61i2pp.169-184
- Alberini, A. (1995). Efficiency vs bias of willingness-to-pay estimates: Bivariate and interval-data models. Journal of Environmental Economics and Management, 29(2), 169–180. https://doi.org/10.1006/jeem.1995.1039
- Aleluia, J., & Ferrão, P. (2016). Characterization of urban waste management practices in developing Asian countries: A new analytical framework based on waste characteristics and urban dimension. Waste Management, 58, 415–429. https://doi.org/10.1016/j.wasman.2016.05.008
- Alhassan, H., Asante, F. A., Oteng-Ababio, M., & Bawakyillenuo, S. (2017). Do socio-psychological factors influence households’ willingness-to-pay for improved solid waste management services? Evidence from Ghana. International Journal of Green Economics, 11(3–4), 183–203. https://doi.org/10.1504/IJGE.2017.089854
- Alhassan, M., & Mohammed, J. (2013). Households’ demand for better solid waste disposal services: Case study of four communities in the new Juaben municipality, Ghana. Journal of Sustainable Development, 6(11), 16. https://doi.org/10.5539/jsd.v6n11p16
- Alpizar, F., Carlsson, F., & Martinsson, P. (2003). Using choice experiments for non-market valuation. Economic Issues-Stoke on Trent-, 8(1), 83–110.
- Amiga, A. (2002a). Households’ willingness to pay for improved solid waste management: The case of Addis Abab. Addis Ababa University].
- Amiga, A. (2002b). Households’ willingness to pay for improved solid waste management: The case of Addis Ababa. Addis Ababa University.
- Anjum, R. (2013). Willingness to pay for solid waste management services: A case study of Islamabad. Pakistan Institute of Development Economics.
- Atinkut, H. B., Yan, T., Arega, Y., & Raza, M. H. (2020). Farmers’ willingness-to-pay for eco-friendly agricultural waste management in Ethiopia: A contingent valuation. Journal of Cleaner Production, 261, 121211. https://doi.org/10.1016/j.jclepro.2020.121211
- Awunyo-Vitor, D., Ishak, S., & Seidu, J. G. (2013). Urban households’ willingness to pay for improved solid waste disposal services in Kumasi Metropolis. Urban Studies Research, 2013, 1–8. https://doi.org/10.1155/2013/659425
- Ayenew, B., Tilahun, A., Erifo, S., & Tesfaye, P. (2019). Household willingness to pay for improved solid waste management in Shashemene town, Ethiopia. African Journal of Environmental Science and Technology, 13(4), 162–171. https://doi.org/10.5897/AJEST2019.2663
- Ayilara, M. S., Olanrewaju, O. S., Babalola, O. O., & Odeyemi, O. (2020). Waste management through composting: Challenges and potentials. Sustainability, 12(11), 4456. https://doi.org/10.3390/su12114456
- Balasubramanian, M. (2019). Household willingness to pay for improved solid waste management services: Using contingent valuation analysis in India. Municipal Solid Waste Management, 10(48). https://doi.org/10.5772/intechopen.83597
- Banga, M., Lokina, R. B., & Mkenda, A. F. (2011). Households’ willingness to pay for improved solid waste collection services in Kampala city, Uganda. The Journal of Environment & Development, 20(4), 428–448. https://doi.org/10.1177/1070496511426779
- Batu, M. M., & Fikadu Tolosa, E. (2016). Determinants of households’ willingness to pay for improved solid waste management in Ethiopia: The case study of Jimma town. Journal of Environment & Earth Science, 6(2224–3216), 75.
- Belay, G., Ketema, M., & Hasen, M. (2020). Households’ willingness to pay for soil conservation on communal lands: Application of the contingent valuation method in north eastern Ethiopia. Journal of Environmental Planning and Management, 63(12), 1–19. https://doi.org/10.1080/09640568.2020.1717933
- Bhada-Tata, P., & Hoornweg, D. A. (2012). What a waste?: A global review of solid waste management.
- Bhattarai, K. (2015). Households’ willingness to pay for improved solid waste management in Banepa municipality, Nepal. The Environment and Natural Resources Journal, 13(2), 14–25.
- Bhatti, M. I., & Al-Shanfari, H. (2017). Econometric analysis of model selection and model testing. Routledge.
- Boateng, K. S., Agyei-Baffour, P., Boateng, D., Rockson, G. N. K., Mensah, K. A., & Edusei, A. K. (2019). Household willingness-to-pay for improved solid waste management services in four major metropolitan cities in Ghana. Journal of Environmental and Public Health, 2019, 1–9. https://doi.org/10.1155/2019/5468381
- Bogale, A., & Urgessa, B. (2012). Households’ willingness to pay for improved rural water service provision: Application of contingent valuation method in Eastern Ethiopia. Journal of Human Ecology, 38(2), 145–154. https://doi.org/10.1080/09709274.2012.11906483
- Cameron, T. A., & Quiggin, J. (1994a). Estimation using contingent valuation data from a “dichotomous choice with follow-up” questionnaire. Journal of Environmental Economics and Management, 27(3), 218–234. https://doi.org/10.1006/jeem.1994.1035
- Cameron, T. A., & Quiggin, J. (1994b). Estimation using contingent valuation data from a“dichotomous choice with follow-up” questionnaire. Journal of Environmental Economics and Management, 27(3), 218–234. https://doi.org/10.1006/jeem.1994.1035
- Cameron, T. A., & Quiggin, J. (1998). Estimation using contingent valuation data from a “dichotomous choice with follow-up” questionnaire: Reply. Journal of Environmental Economics and Management, 35(2), 195–199. https://doi.org/10.1006/jeem.1998.1026
- Cawley, J. (2008). Contingent valuation analysis of willingness to pay to reduce childhood obesity. Economics & Human Biology, 6(2), 281–292. https://doi.org/10.1016/j.ehb.2008.05.003
- Chinasho, A. (2015). Review on community based municipal solid waste management and its implication for climate change mitigation. American Journal of Scientific and Industrial Research, 6(3), 41–46.
- Dagnew, H., Alemu Mekonnen, A. M., & Zenebe Gebreegziabher, Z. G.(2012). Households’ willingness to pay for improved urban waste management in Mekelle City, Ethiopia. Discussion Paper Series Apri l 2012. EfD DP 12–16.
- Damtew, Y. T., & Desta, B. N. (2015). Micro and small enterprises in solid waste management: Experience of selected cities and towns in Ethiopia: A review. Pollution, 1(4), 461–427.
- Delaney, L., & O’Toole, F. (2006). Willingness to pay: Individual or household? Journal of Cultural Economics, 30(4), 305–309. https://doi.org/10.1007/s10824-006-9021-8
- Elisabeth Marie Constance, N. (2021). Households willingness to pay for solid waste collection services in secondary cities of Rwanda. University of Rwanda].
- Endalew, B., Tassie, K., & Ayalew, Z. (2019). Non-market measurement techniques of willingness to pay, the case of environmental resources: A review. Journal of Agriculture and Environmental Sciences, 3(2), 1–22.
- Endalew, B., Tassie, K., & Nzeadibe, T. (2018). Urban households’ demand for improved solid waste management service in Bahir Dar city: A contingent valuation study. Cogent Environmental Science, 4(1), 1426160. https://doi.org/10.1080/23311843.2018.1426160
- Entele, B. R. (2020). Analysis of households’ willingness to pay for a renewable source of electricity service connection: Evidence from a double-bounded dichotomous choice survey in rural Ethiopia. Heliyon, 6(2), e03332. https://doi.org/10.1016/j.heliyon.2020.e03332
- Ezebilo, E. (2013). Willingness to pay for improved residential waste management in a developing country. International Journal of Environmental Science and Technology, 10(3), 413–422. https://doi.org/10.1007/s13762-012-0171-2
- Fenta, B. A. (2017). Waste management in the case of Bahir Dar city near lake tana shore in Northwestern Ethiopia: A review. African Journal of Environmental Science and Technology, 11(8), 393–412. https://doi.org/10.5897/AJEST2017.2340
- Gashaw, T., Bantider, A., & Mahari, A. (2014). Population dynamics and land use/land cover changes in Dera District, Ethiopia. Global Journal of Biology, Agriculture & Health Sciences, 3, 137–140.
- Gebreeyessus, G., Berihun, D., & Terfassa, B. (2019). Characterization of solid wastes in higher education institutions: The case of Kotebe Metropolitan University, Addis Ababa, Ethiopia. International Journal of Environmental Science and Technology, 16(7), 3117–3124. https://doi.org/10.1007/s13762-018-1953-y
- Gebreslassie, M. G., Gebreyesus, H. B., Gebretsadik, M. T., Bahta, S. T., & Birkie, S. E. (2019). Assessment of the generation rate and Composition of municipal solid waste in Mekelle City. Branna Journal of Engineering and Technology, 1(2), 185–197.
- Gedefaw, M. (2015). Assessing the current status of solid waste management of Gondar town. International Journal of Scientific & Technology Research, 4(9), 28–36.
- Getahun, T., Mengistie, E., Haddis, A., Wasie, F., Alemayehu, E., Dadi, D., Van Gerven, T., & Van der Bruggen, B. (2012). Municipal solid waste generation in growing urban areas in Africa: Current practices and relation to socioeconomic factors in Jimma, Ethiopia. Environmental Monitoring and Assessment, 184(10), 6337–6345. https://doi.org/10.1007/s10661-011-2423-x
- Girma, H., Geremew, A., Alemayehu, M., Mulatu, G., Gebrehiwot, M., & Defloor, B. (2022). Urban Households’ willingness to pay to Improve Municipal Solid Waste Collection Services and associated factors: A double-bounded contingent valuation study in Harar City, Ethiopia. Environmental Health Insights, 16, 11786302221134937. https://doi.org/10.1177/11786302221134937
- Gizaw, Z., Gebrehiwot, M., Teka, Z., & Molla, M. (2014). Assessment of occupational injury and associated factors among municipal solid waste management workers in Gondar town and Bahir Dar City, Northwest Ethiopia, 2012. Journal of Medicine and Medical Sciences, 5(9), 181–192.
- Haab, T. C. (1998). Estimation using contingent valuation data from a “dichotomous choice with follow-up” questionnaire: A comment. Journal of Environmental Economics and Management, 35(2), 190–194. https://doi.org/10.1006/jeem.1998.1025
- Haab, T. C., & McConnell, K. E. (2002). Valuing environmental and natural resources: The econometrics of non-market valuation. Edward Elgar Publishing.
- Hagos, D., Mekonnen, A., & Gebreegziabher, Z. (2013). Households willingness to pay for improved urban solid waste management: The case of Mekelle city, Ethiopia. Ethiopian Journal of Economics, 22(1), 107–138.
- Haji, J., & Tesfaye, S. (2014). Determinants of farm households willingness to pay for restoration of Lake Haramaya Eastern Ethiopia. Haramaya University].
- Hanemann, M., Loomis, J., & Kanninen, B. (1991). Statistical efficiency of double-bounded dichotomous choice contingent valuation. American Journal of Agricultural Economics, 73(4), 1255–1263. https://doi.org/10.2307/1242453
- Haron, N. F., Kamaludin, M., Mahmood, S., & Amirah, N. A. (2023). Analysis of households’willingness to pay for solid waste management services in Kuala Nerus, Terengganu. Journal of Sustainability Science and Management, 18(10), 35–48. https://doi.org/10.46754/jssm.2023.10.003
- Hoornweg, D., & Bhada-Tata, P. (2012). What a waste: A global review of solid waste management.
- Hoyos, D., & Mariel, P. (2010). Contingent valuation: Past, present and future. Prague Economic Papers, 4(2010), 329–343. https://doi.org/10.18267/j.pep.380
- Jantzen, J. (2006). The economic value of natural and environmental resources. Institute for Applied Environmental Economics, Netherland.
- Jianjun, J., Wenyu, W., Ying, F., & Xiaomin, W.(2016). Measuring the willingness to pay for drinking water quality improvements: Results of a contingent valuation survey in Songzi, China. Journal of Water and Health, 14(3), 504–512.
- Johnston, R. J., Boyle, K. J., Adamowicz, W., Bennett, J., Brouwer, R., Cameron, T. A., Hanemann, W. M., Hanley, N., Ryan, M., Scarpa, R., Tourangeau, R., & Vossler, C. A. (2017). Contemporary guidance for stated preference studies. Journal of the Association of Environmental and Resource Economists, 4(2), 319–405. https://doi.org/10.1086/691697
- Kaso, A. W., Hareru, H. E., Ashuro, Z., Soboksa, N. E., & Chupradit, S. (2022). Assessment of households’ willingness to join and pay for Improving Waste Management Practices in Gedeo Zone, Southern Ethiopia. BioMed Research International, 2022, 1–10. https://doi.org/10.1155/2022/9904665
- Kassa, B. A., & Teshome, Y. M. (2016). Smallholder Farmer’s willingness to pay for improved soil and water conservation practice: A contingent valuation study in Abaro-Toga Watershed Ethiopia. American Journal of Business, Economics and Management, 3(6), 432.
- Kayamo, S. E. (2022). Willingness to pay for solid waste management improvement in Hawassa city, Ethiopia. Journal of environmental management, 302, 113973. https://doi.org/10.1016/j.jenvman.2021.113973
- Kwak, S.-Y., Yoo, S.-H., & Kim, C.-S. (2013). Measuring the willingness to pay for tap water quality improvements: Results of a contingent valuation survey in Pusan. Water, 5(4), 1638–1652. https://doi.org/10.3390/w5041638
- Lami, A., Fada, A., & Sanda, H. (2019). Assessment of Challenges Associated with waste disposal in Zuru Town, Kebbi State. Asian Journal of Geographical Research, 1–9. https://doi.org/10.9734/ajgr/2019/v2i430090
- Lee, C.-Y., & Heo, H. (2016). Estimating willingness to pay for renewable energy in South Korea using the contingent valuation method. Energy Policy, 94, 150–156. https://doi.org/10.1016/j.enpol.2016.03.051
- Lin, Y.-M., Fu, T.-T., Yeh, P., & Huang, M.-Y. (2013). Assessing the economic value of public service broadcasting in Taiwan using the contingent valuation approach. Journal of Media Economics, 26(4), 186–202. https://doi.org/10.1080/08997764.2013.842921
- Lunojo, E. (2016). Household willingness to pay for improved solid waste management services in Njombe town council, Tanzania. Mzumbe University].
- Maskey, B., & Singh, M. (2017a). Households’ willingness to pay for improved waste collection service in Gorkha municipality of Nepal. Environments, 4(4), 77. https://doi.org/10.3390/environments4040077
- Maskey, B., & Singh, M. (2017b). Households’ willingness to Pay for Improved Waste Collection Service in Gorkha Municipality of Nepal. Environments, 4(4), 77. https://doi.org/10.3390/environments4040077
- Massoud, M., Lameh, G., Bardus, M., & Alameddine, I. (2021). Determinants of waste management practices and willingness to pay for improving waste services in a low-middle income country. Environmental management, 68(2), 198–209. https://doi.org/10.1007/s00267-021-01472-z
- Mekuria, T., Muralitharan, J., & Ali, Y. (2019). GIS and remote sensing based suitable site selection for solid waste disposal: A case study of Gondar Town. North West Ethiopia.
- Mezgebo, G. K., & Ewnetu, Z. (2015). Households willingness to pay for improved water services in urban areas: A case study from Nebelet town, Ethiopia. Journal of Development and Agricultural Economics, 7(1), 12–19. https://doi.org/10.5897/JDAE2014.0604
- Mitchell, R. C., & Carson, R. T. (2013). Using surveys to value public goods: The contingent valuation method. Rff press.
- Mohammed, A., & Elias, E. (2017). Domestic solid waste management and its environmental impacts in Addis Ababa City. Journal of Environment and Waste Management, 4(1), 194–203.
- Mohee, R., & Simelane, T. (2015). Future directions of municipal solid waste management in Africa. Africa Institute of South Africa.
- Mombo, F., & Bigirwa, D. (2017). The role of sub-saharan Africa countries’ households waste charges on sustainable cities development. International Journal of Waste Resources, 07(1). https://doi.org/10.4172/2252-5211.1000265
- Mulat, S., Worku, W., & Minyihun, A.(2019). Willingness to pay for improved solid waste management and associated factors among households in Injibara town, Northwest Ethiopia. BMC Research Notes, 12, 1–6.
- Mulat, S., Worku, W., & Minyihun, A. (2019b). Willingness to pay for improved solid waste management and associated factors among households in Injibara town, Northwest Ethiopia. BMC Research Notes, 12(1), 1–6. https://doi.org/10.1186/s13104-019-4433-7
- Mussa, J. (2015). Residents’ willingness to pay for improved solid waste management in Dodoma municipality, Tanzania. Sokoine University of Agriculture].
- Nain, P., & Kumar, A. (2023). Understanding manufacturers’ and consumers’ perspectives towards end-of-life solar photovoltaic waste management and recycling. Environment, Development and Sustainability, 25(3), 2264–2284. https://doi.org/10.1007/s10668-022-02136-6
- Olawade, D. B., Wada, O. Z., Ore, O. T., David-Olawade, A. C., Esan, D. T., Egbewole, B. I., & Ling, J. (2023). Trends of solid waste generation during COVID-19 pandemic: A review. Waste Management Bulletin, 1(4), 93–103. https://doi.org/10.1016/j.wmb.2023.10.002
- Omilani, O., Abass, A. B., & Okoruwa, V. O. (2019). Smallholder agroprocessors’ willingness to pay for value-added solid-waste management solutions. Sustainability, 11(6), 1759. https://doi.org/10.3390/su11061759
- Omran, A., & Gebril, A. O. (2018). Municipal solid waste management practices in the central part of Libya. In A. Omran & O. Schwarz-Herion (Eds.), The impact of climate change on our life (pp. 149–165). Springer. https://doi.org/10.1007/978-981-10-7748-7
- Oyawole, F., Ajayi, O., Aminu, R., & Akerele, D. (2016). Willingness to pay for improved solid waste management services in an urbanizing area in South-East Nigeria. Ethiopian Journal of Environmental Studies and Management, 9(6), 793–803. https://doi.org/10.4314/ejesm.v9i6.11
- Rahji, M., & Oloruntoba, E. O. (2009). Determinants of households’ willingness-to-pay for private solid waste management services in Ibadan, Nigeria. Waste Management & Research, 27(10), 961–965. https://doi.org/10.1177/0734242X09103824
- Sanjeevi, V., & Shahabudeen, P. (2015). Development of performance indicators for municipal solid waste management (PIMS): A review. Waste Management & Research, 33(12), 1052–1065. https://doi.org/10.1177/0734242X15607428
- Sharma, H., Abebe, T., Admassu, M., Teshaye, T., Aseffa, T., & Emana, M. (2011). Municipal solid waste management and community awareness and involvement in management practices: An overview and a case study from Gondar town of Ethiopia. International Journal of Environment and Waste Management, 7(3–4), 294–304. https://doi.org/10.1504/IJEWM.2011.039470
- Sharma, K. D., & Jain, S. (2020). Municipal solid waste generation, composition, and management: The global scenario. Social Responsibility Journal, 16(6), 917–948. https://doi.org/10.1108/SRJ-06-2019-0210
- Solomon, J. (2004). Contingent valuation of multi-purpose tree resources: The case of Arsi Zone, Ethiopia [ MSc Thesis]. School of Graduate Studies of Addis Ababa University,
- Suryawan, I. W. K., & Lee, C.-H. (2023). Citizens’ willingness to pay for adaptive municipal solid waste management services in Jakarta, Indonesia. Sustainable Cities and Society, 97, 104765. https://doi.org/10.1016/j.scs.2023.104765
- Tassie, K., Endalew, B., & Behzadian, K. (2020). Willingness to pay for improved solid waste management services and associated factors among urban households: One and one half bounded contingent valuation study in bahir dar city, Ethiopia. Cogent Environmental Science, 6(1), 1807275. https://doi.org/10.1080/23311843.2020.1807275
- Wang, H., He, J., Kim, Y., & Kamata, T. (2014). Municipal solid waste management in rural areas and small counties: An economic analysis using contingent valuation to estimate willingness to pay for Yunnan, China. Waste Management & Research, 32(8), 695–706. https://doi.org/10.1177/0734242X14539720
- Woretaw, E., Woubishet, D., & Asmare, W. (2017a). Households’ preferences and willingness to pay for improved solid waste management interventions using choice experiment approach: Debre Tabor Town, Northwest Ethiopia. Ethiopian Economics Associat and Ethiopian Strategy Support P (ESSP) of IFPRI, 1(215).
- Woretaw, E., Woubishet, D., & Asmare, W. (2017b). Households’ preferences and willingness to pay for improved solid waste management interventions using choice experiment approach: Debre Tabor Town, Northwest Ethiopia. Journal of Economics & Sustainable Development, 8(7), 16–32.
- Yamane, T.(1967). Statistics: An introductory analysis. New York University.
- Yasin, A. S. (2021). Assessing households’ willingness to pay for improved solid waste management services in Jigjiga, Ethiopia. Environment and Ecology Research, 9(2), 39–44. https://doi.org/10.13189/eer.2021.090201
- Yusuf, S., Ojo, O., & Salimonu, K. (2007a). Households’ willingness to pay for improved solid waste management in Ibadan North local government area of Oyo state, Nigeria. Journal of Environmental Extension, 6, 57–63. https://doi.org/10.4314/jext.v6i1.2766
- Yusuf, S., Ojo, O., & Salimonu, K. (2007b). Households’ willingness to pay for improved solid waste management in Ibadan North local government area of Oyo state, Nigeria. Journal of Environmental Extension, 6(1), 57–63. https://doi.org/10.4314/jext.v6i1.2766
- Zhu, D., Asnani, P., Zurbrugg, C., Anapolsky, S., & Mani, S. K. (2007). Improving municipal solid waste management in India: A sourcebook for policymakers and practitioners. The World Bank.