Abstract
Background
The mechanism of Chemotherapy-induced neuropathic pain (NP) remains obscure. This study was aimed to uncover the key genes as well as protein networks that contribute to Oxaliplatin-induced NP.
Material/Methods
Oxaliplatin frequently results in a type of Chemotherapy-induced NP that is marked by heightened sensitivity to mechanical and cold stimuli, which can lead to intolerance and discontinuation of medication. We investigated whether these different etiologies lead to similar pathological outcomes by targeting shared genetic targets or signaling pathways. Gene expression data were obtained from the Gene Expression Comprehensive Database (GEO) for GSE38038 (representing differential expression in the spinal nerve ligation model rats) and GSE126773 (representing differential expression among the Oxaliplatin-induced NP model rats). Differential gene expression analysis was performed using GEO2R.
Results
Protein-protein interaction (PPI) analysis identified 260 co-differentially expressed genes (co-DEGs). Subsequently, Kyoto Encyclopedia of Genes and Genomes (KEGG) analysis revealed three shared pathways involved in both models: Kaposi sarcoma-associated herpesvirus (KSHV) infection, Epstein-Barr virus (EBV) infection, and AGE-RAGE signaling pathway in diabetic complications. Further bioinformatics analysis highlighted eight significantly up-regulated genes in the NP group: Mapk14, Icam1, Cd44, IL6, Cxcr4, Stat1, Casp3 and Fgf2. Our results suggest that immune dysfunction, inflammation-related factors or regulating inflammation factors may also be related to Oxaliplatin-induced NP. Additionally, we analyzed a dataset (GSE145222) involving chronic compression of DRGs (CCD) and control groups. CCD model is a classic model for studying NP. We assessed these hub genes’ expression levels. In contrast with the control groups, the hub genes were up-regulated in CCD groups, the difference was statistically significant, except Stat1.
Conclusion
Our research significantly contributes to elucidating the mechanisms underlying the occurrence as well as the progression of Oxaliplatin-induced NP. We have identified crucial genes and signaling pathways associated with this condition.
Introduction
Chemotherapy-induced neuropathic pain (CINP) is a progressive, persistent, and challenging disease characterized by pain, numbness, tingling sensation, and sensitivity to cold. It affects about 50–90% of individuals undergoing chemotherapy treatment.Citation1 CIPN is mainly induced by first-line chemotherapy drugs such as oxaliplatin, paclitaxel, and vinblastine. With the accumulation of chemotherapy drug toxicity, peripheral neuropathological symptoms become increasingly severe, compelling patients to reduce the chemotherapy drug dosages, shorten the chemotherapy cycle, or even discontinue medication, ultimately impacting patient survival rates. Chemotherapy-induced neuropathic pain (CINP) remains a therapeutic challenge with no effective drugs and treatments currently available.
Oxaliplatin is a widely used chemotherapy-drug for the treatment of colorectal and advanced ovarian cancer.Citation2 However, one of the major side effects associated with oxaliplatin treatment is the development of neuropathic pain (NP). This condition is characterized by severe and painful peripheral neuropathy, which can often lead to dose reduction or early discontinuation of chemotherapy.Citation3,Citation4 Despite its clinical significance, the exact mechanism underlying Oxaliplatin-induced neurotoxicity and the subsequent development of NP remains unclear.
Inflammation of the dorsal root ganglions (DRGs) is the pivotal physiological basis for the occurrence of NP. During the process of pain generation, the DGRs serve as the primary neuron of pain input in the pain mechanism. So the DGRs are considered to be key participants within the pathogenesis that lead to NP.Citation5 Studies have found that the expression of neuroinflammatory markers is significantly increased in both the dorsal root ganglion (DRG) and the spinal cord (L4-L5 region) of rats.Citation6,Citation7 Other studies have found that the main target organ of oxaliplatin’s action is the DGRs,Citation8,Citation9 its specific mechanism is still unclear. Investigating the shared targets and signaling pathways associated with different etiologies leading to the same outcome can enhance our comprehension of the molecular mechanisms underlying NP.In this study, GSE38038 and GSE126773 datasets obtained from the GEO database were investigated. Combined bioinformatics analyses with enrichment methods, the objective of this study was to find out shared differentially expressed genes (DEGs) and elucidate the functions of them in NP induced by diverse etiologies. Additionally, the construction of a protein-protein interaction (PPI) network was carried out, and the STRING database and Cytoscape software were employed to uncover gene modules and identify central genes as hub genes. Finally, we collected expression data on central genes from CCD model rat induced by chronic compression of DRGs to validate our research findings against the experimental and control groups.
Materials and Methods
Data Source
We conducted a search of the public GEO database (https://www.ncbi.nlm.nih.gov/geo/) and retrieved two GSE datasets (GSE38038 and GSE126773) that pertain to the microarray changes in the dorsal root ganglia (DRGs) of rats. In total, four Oxaliplatin-induced NP samples (GSM3612644, GSM3612645, GSM3612646, GSM3612647) and three normal samples (GSM3612641, GSM3612642, GSM3612643) from GSE126773; four samples of NP induced by spinal nerve ligation (GSM932859, GSM932860, GSM932561, GSM932862), and four sham operation samples (GSM932855, GSM932856, GSM932857, GSM932858) from GSE38038 were selected.
Identification of DEGs
To differentiate DEGs of the diseased and control groups, we utilized the online analysis tool of GEO2R (http://www.ncbi.nlm.nih.gov/geo/geo2r)Citation10 on a basis of the R packages of GEOquery as well as Limma, which facilitate data reading and differential expression calculation, respectively. By comparing gene expression profiles across groups, we determined the DEGs associated with the disease condition. Probe sets lacking annotated gene identifiers were excluded from the analysis to ensure the validity and precision of the results, and genes exhibiting multiple probe sets were either eliminated or their expression levels were averaged. Genes were deemed to be significantly DEGs if they met the criteria of an adjusted P-value less than 0.05 and a |logFC (fold change) | of no less than 1.0. To identify common DEGs, a Venn diagram performed using R software.
Functional and Pathway Enrichment Analysis
The Gene Ontology (GO) database provides succinct annotations regarding the characteristics of gene products, encompassing their functional attributes, involvement in biological pathways, as well as cellular localization. Besides, the Kyoto Encyclopedia of Genes and Genomes (KEGG) Pathway database focuses on the compilation of gene pathway information across distinct species. Results of enrichment analysis were achieved utilizing the Pathview database (https://www.bioinformatics.com.cn).Citation11 Pathview represents an innovative toolkit designed for integrating and visualizing data based on pathways simplifies the process of mapping user-provided data onto relevant pathway graphs. Users are only required to provide their data and data file, after which the tool seamlessly integrates and maps the user data onto the corresponding pathway. It also generates pathway graphs with the mapped data, including the generation of Venn diagrams. A significance threshold of adjusted P < 0.05 was utilized to indicate statistical significance.
PPI Network Construction and Module Analysis
To explore the relationships within relevant proteins, including direct binding interactions and interconnected regulatory pathways both upstream and downstream, the Search Tool for the Retrieval of Interacting Genes (STRING; http://string-db.org) (version 11.0)Citation12 was utilized. The utilization of the STRING database facilitates the construction of a protein-protein interaction (PPI) network, which enables the representation and analysis of complex regulatory connections among proteins. Besides, interactions with a cumulative score more than 0.7 were deemed to have statistical significance. For visualizing this PPI network, we employed Cytoscape 3.7.2 (http://www.cytoscape.org).Citation13 To identify key functional modules, we employed Cytoscape’s molecular complex detection technology (MCODE). Selection criteria were were defined as follows: (1) a K-core value of 2; (2) a degree cutoff of 2; (3) a maximum depth of 100, (4) a node score cutoff of 0.2.
Selection and Analysis of Hub Genes
The cytoHubba plug-in incorporated within the Cytoscape software was utilized for the purpose of identifying hub genes. Eight commonly used algorithms, namely MCC, Degree, MNC, Stress, Closeness, BottleNeck, EPC, and Radiality, were utilized to choose these hub genes, followed by the construction of a co-expression network among the hub genes using GeneMANIA, a powerful tool for elucidating intrinsic relationships within sets of genes. The GeneMANIA website (http://www.genemania.org) was employed for this purpose. Mod Enrichr was utilized for enrichment analysis by introducing hub genes. Mod Enrichr is a suite of tools for gene set enrichment analysis(https://maayanlab.cloud/Enrichr/).Citation14
Hub Genes Validation Study
The expression of identified hub genes was verified in GSE145222, which contained 5 sham groups as well as 4 chronic compression of DRGs model groups. The comparison between groups was conducted using a T-test, a statistical test that assesses the significance of differences (P <0.05) between means.
Ethical Declaration
All data utilized in this study were sourced from publicly available databases. The study does not involve any investigations pertaining to animals or humans.
Results
Identification of DEGs
To identify genes exhibiting differential expression in the DRGs following NP, we retrieved two microarray datasets from the GEO database: GSE126773 () and GSE38038 (). These datasets provide information regarding gene alterations specifically observed in Rat DRGs after NP. The heap maps of GSE38038 as well as GSE126773 showed the expression levels of DEGs ( and ). After performing differential expression analysis on the GSE126773 and GSE38038 datasets, 260 DEGs were identified. These DEGs were found to be overlapping, as illustrated in .
Figure 1 Bioinformatics analysis of the DRGs microarray datasets, GSE126773 and GSE38038, after nucleus pulposus (NP). (A) Volcano plot depicting the differentially expressed genes (DEGs) in GSE126773. (B) Volcano plot illustrating the DEGs in GSE38038. (C) Heatmap displaying the top 1000 DEGs in GSE126773. (D) Heatmap showcasing the top 1000 DEGs in GSE38038.

Analysis of the Functional Characteristics of Common DEGs
To investigate the biological functions as well as pathways associated with the 260 common DEGs, enrichment analysis was conducted using GO and KEGG Pathway databases. The results of the GO analysis revealed that the enriched biological processes of these genes were primarily in relation with response to peptide and myeloid cell homeostasis (). Additionally, the analysis indicated enrichment in terms of neuron to neuron synapse, postsynaptic density (), growth factor receptor binding, and hormone receptor binding (). Regarding the KEGG Pathway analysis, results indicate significant enrichment of the 260 common DEGs in three key pathways, namely insulin resistance, EGFR tyrosine kinase inhibitor resistance, and toxoplasmosis ().
Figure 3 GO analysis and KEGG pathway analysis of the overlapping DEGs. (A) Enriched biological processes (BP) identified in GO analysis. (B) Cellular component (CC) terms enriched in GO analysis. (C) Molecular function (MF) terms enriched in GO analysis. (D) KEGG pathway enrichment analysis of the integrated DEGs.
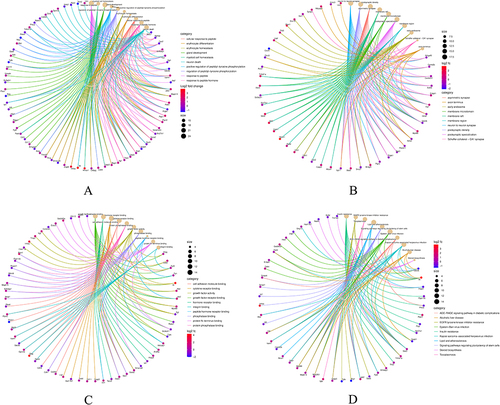
PPI Network Construction and Module Analysis
Using Cytoscape, a PPI network was constructed for the common DEGs. The network was generated by incorporating interactions with combined scores greater than 0.7, indicating a high level of confidence in the reliability of the interactions. Through the utilization of the MCODE plug-in within Cytoscape, six gene clustering modules were identified (). Among these modules, a total of twenty-five genes were found to be present in all of them, indicating their potential functional significance. Notably, the three modules with the highest scores encompassed sixteen genes, suggesting their potential importance in the biological context ().The GO analysis revealed that the identified genes are significantly associated with several biological processes and molecular functions, including sterol biosynthetic process, perinuclear endoplasmic reticulum localization, 3-keto sterol reductase activity, type 5 metabotropic glutamate receptor binding, CD4 receptor binding, and oxidoreductase activity. Furthermore, the oxidoreductase activity was characterized by the process of oxidizing a pair of donors, leading to the reduction of molecular oxygen as well as the production of two molecules of water (). According to the KEGG pathway analysis, the identified genes were found to be primarily associated with three key pathways: steroid biosynthesis, Epstein-Barr virus (EBV) infection, and the AGE-RAGE signaling pathway in diabetic complications ().
Hub Gene Selection and Analysis
Through the application of the cytoHubba plug-in and employing six algorithms, the top 10 hub genes were identified, as presented in . Through the analysis of the Venn diagrams, we have identified ten hub genes that are commonly shared, namely Stat1, II6, Stat3, Cd44, Icam1, Casp3, Fgf2, Jak2, Cxcr4, and Mapk14 (). In order to gain a deeper understanding of the 10 identified hub genes, we utilized the GeneMANIA database to conduct further analysis of their co-expression network and associated functions. The analysis revealed a complex PPI network, with physical interactions accounting for 84.50%, shared protein domains for 6.34%, co-expression for 30.45%, co-localization for 4.01%, and predicted interactions for 5.61% (). Function analysis conducted using GeneMANIA demonstrated that these genes are primarily involved in regulating endothelial cell migration, blood vessel endothelial cell migration, myeloid cell homeostasis, and response to cytokine stimulus regulation, among other functions. Notably, eight genes were found to appear in the three modules with the highest scores, indicating their potential significance (). Additionally, these genes exhibited significant expression differences when compared the control group to the treatment group (). For a comprehensive understanding, provides the complete names of these genes along with their associated functions. Furthermore, KEGG pathway analysis of these 10 hub genes has revealed their strong association with Kaposi sarcoma-associated herpesvirus (KSHV) infection, EBV infection, and the AGE-RAGE signaling pathway in diabetic complications ( and ).
Table 1 The Top 10 Hub Genes by Topological Analysis Methods of CytoHubba
Table 2 Details of the Hub genes
Figure 6 Venn diagram and co-expression network of hub genes. (A) The Venn diagram illustrates the overlapping hub genes identified by six different algorithms. (B) The co-expression network of hub genes and their associated genes was analyzed using GeneMANIA. (C) Module analysis revealed that eight genes were present in the three modules with the highest scores. (D) Expression patterns of the eight hub genes in the control and treatment groups. (E and F) Pathways associated with the hub genes.
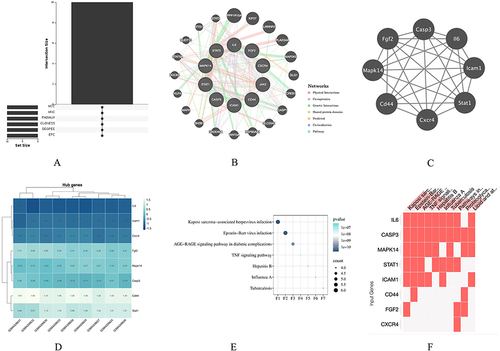
Validation of Hub Genes Expression
To assess the reliability of the expression levels of the identified hub genes (Mapk14, Icam1, Cd44, IL6, Cxcr4, Stat1, Casp3, and Fgf2), we selected a dataset (GSE145222) that includes both sham and chronic compression of DRGs (CCD) groups. The CCD model is a well-established model used to study nucleus pulposus (NP). We conducted an analysis of the expression levels of the aforementioned hub genes in the selected dataset. The results demonstrated that, in comparison to the control group, all of the hub genes showed up-regulation in the CCD groups, with statistically significant differences observed, except for Stat1 (). These findings serve as additional evidence to support the potential involvement of these hub genes in the context of NP.
Discussion
Chemotherapy-induced peripheral neuropathic pain (CIPN) is a common and debilitating adverse reaction associated with anticancer medications. Oxaliplatin, an anticancer drug derived from platinum and utilized in advanced colorectal cancer, frequently induces a distinct type of CIPN marked by increased sensitivity to mechanical / cold stimuli. However, the mechanisms underlying the development and chronicization of Chemotherapy-induced peripheral neuropathy, as well as effective treatment options, remain poorly understood, highlighting the urgent need for therapeutic advancements in this area. In this study, our findings shed light on potential pathways implicated in the development of CIPN. Specifically, we identified KSHV infection, EBV infection, as well as the AGE-RAGE signaling pathway in diabetic complications as potentially crucial pathways in both animal models of peripheral neuropathy induced by spinal nerve ligation and oxaliplatin treatment. Moreover, we found that the genes Mapk14, Icam1, Cd44, IL6, Cxcr4, Stat1, Casp3, and Fgf2 may play significant roles during the development of CIPN.
Kaposi sarcoma-associated herpesvirus (KSHV) is known to cause various human malignancies and hyperproliferative diseases, particularly in individuals with compromised immune systems like those infected with human immunodeficiency virus (HIV). KSHV has the ability to modulate innate immune pathways, enabling infected cells to survive for extended periods following primary infection and during viral latency.Citation15 EBV primarily infects B cells, remaining dormant in the body until certain conditions disrupt the EBV-host balance and allow the virus to manifest its pathogenic potential. Studies have suggested that EBV can induce immune dysfunction in susceptible individuals, leading to neuroinflammation through autoimmunity or antiviral immune responses.Citation16 Diabetes is associated with increased production of advanced glycation end products (AGEs) resulting from reactive dicarbonyl compounds in a hyperglycemic environment. AGEs can trigger the expression of pro-inflammatory cytokines through their main receptor, receptor for advanced glycation end products (RAGE). The involvement of the AGE-RAGE signaling pathway has been suggested in diabetic peripheral neuropathy’s pathogenesis. Several studies have shown a connection between diabetes-related peripheral neuropathy and the AGE-RAGE signaling pathway in diabetic complications.Citation17–19 In the context of our study, we found that immune dysfunction and the AGE-RAGE signaling pathway in diabetes complications may also play a role in the development of oxaliplatin-induced peripheral neuropathy.
In our study, we observed significant upregulation of genes such as Mapk14, Icam1, CD44, IL6, Cxcr4, Stat1, Casp3, and Fgf2 in both Oxaliplatin-induced peripheral neuropathy (NP) and spinal nerve ligation-induced NP animal models compared to the control group. Mapk14, a member of the MAP kinase family, might be activated by various environmental pressures as well as pro-inflammatory cytokines. Previous research on microglia and NP has shown that Mapk14 is significantly upregulated and plays a role in microglial activation in NP animal models.Citation20 Icam1 is a glycoprotein located on the cell surface and acts as an adhesion receptor. It plays a crucial role in regulating the recruitment of white blood cells to areas of inflammation. It participates in important physiological processes such as cell signal transduction, immune response, and inflammatory response. In NP animal models, Icam1 has been found to be significantly increased compared to the control group.Citation21 Additionally, another study found upregulated expression of Icam1 in the spinal cord of rat models of NP compared to rat models of inflammatory pain.Citation22 CD44 is a transmembrane glycoprotein that is widely expressed in various cell types. It is involved in various biological processes including cell adhesion, migration, signal transduction, proliferation, and tumor metastasis. Recent studies focusing on CD44 and CIPN have demonstrated that CD44 acts as a mediator of HMWH-induced pain relief in cases of oxaliplatin and paclitaxel-induced CIPN.Citation23 These findings emphasize the significant role of CD44 signaling in HMWH-induced antihyperalgesia and establish it as a potential therapeutic target for inflammatory conditions and NP.Citation24 Interleukin-6 (IL-6) is an interleukin with dual functionality, serving as both a pro-inflammatory cytokine and an anti-inflammatory myokine. The inflammatory response, involving the propagation of inflammation and recruitment of neutrophils, is initiated when glial cells are activated by noxious stimuli and inflammation. Pro-inflammatory cytokines, including IL-6, play a crucial role in the initiation and maintenance of NP.Citation25 In a study, it was found that the expression levels of the inflammatory factor IL-6 in the ipsilateral L4-L6 spinal dorsal horn were increased in contrast with the contralateral side at 7 days following nerve crush injury.Citation26
Cxcr4 is a specific receptor for the chemokine stromal cell-derived factor-1 (SDF-1) and exerts a strong effect on the promotion of chemotaxis, particularly for lymphocytes. It has been suggested that Cxcr4 is closely related to the occurrence of NP. Research has shown that Cxcr4 expression increases in spinal glial cells of mice with peripheral nerve injury-induced NP. Blocking Cxcr4 can alleviate pain behavior, while overexpressing Cxcr4 can induce pain hypersensitivity.Citation27 Stat1 belongs to the STAT family, which is critical for regulating gene expression within cells and important in immune responses. In the context of chronic NP, a study identified specific blood biomarkers for chronic NP by comparing patients with chronic pain (neuropathic and nociceptive) to painless controls, and Stat1 was among the identified biomarkers.Citation28 Casp3, a downstream effector protease in the apoptosis cascade, plays a crucial role in cell apoptosis. In a study, IIK-7 was found to significantly alleviate mechanical allodynia and glial activation while inhibiting casp-3 proteins. This suggests that IIK-7 reduces NP by inhibiting glial activation and suppressing proteins associated with inflammation as well as apoptosis.Citation29 Fgf-2 belongs to the fibroblast cytokine family, which is significant for various processes such as tendon-to-bone healing, cartilage repair, bone repair, and nerve regeneration. Research has shown that spinal cord astrocytes upregulate Fgf-2, a neurotrophic and gliogenic factor, in response to the ligation of spinal nerves L5 and L6. Studies have revealed that endogenous astroglial Fgf-2 plays a role in sustaining neuropathic pain (NP) tactile allodynia, which is linked to the reactivity of spinal cord astrocytes. Inhibiting spinal Fgf-2 has been shown to ameliorate NP symptoms.Citation30 These findings suggest that factors involved in inflammation and regulation of inflammation may also play a role in oxaliplatin-induced NP. Understanding the involvement of these factors could provide insights into potential therapeutic targets for managing NP.
The objective of this study was to utilize bioinformatics technology to identify common factors in oxaliplatin-induced NP animal models and spinal nerve ligation-induced NP animal models. By identifying DEGs and hub genes, the study aimed to enhance our understanding of the underlying mechanisms of oxaliplatin-induced NP. However, there were several limitations to consider in this study. Firstly, the study design is retrospective, which means that the findings should be validated using external sources to ensure their reliability and reproducibility. Additional studies with independent datasets are necessary to confirm the identified DEGs and hub genes. Secondly, further investigations are needed to validate the functional roles of the identified hub genes using in vitro models. Experimental studies should be conducted to explore the mechanisms by which these hub genes contribute to oxaliplatin-induced NP.
Conclusions
In conclusion, our study utilized bioinformatics analysis to identify common DEGs in two independent datasets related to oxaliplatin-induced NP and spinal nerve ligation-induced NP. Through enrichment and PPI network analysis, we discovered several hub genes that are potentially involved in the shared mechanisms underlying these two types of NP.
Author Contributions
All authors made a significant contribution to the work reported, whether that is in the conception, study design, execution, acquisition of data, analysis and interpretation, or in all these areas; took part in drafting, revising or critically reviewing the article; gave final approval of the version to be published; have agreed on the journal to which the article has been submitted; and agree to be accountable for all aspects of the work.
Disclosure
The authors declare that there are no conflicts of interest in this work.
Data Sharing Statement
The data that support the findings of this study are downloaded from the Gene Expression Omnibus (GEO) database (https://www.ncbi.nlm.nih.gov/geo/query/acc.cgi; GEO accession: GSE38038; GSE126773).
References
- Chahal SK, Sodhi RK, Madan J. Duloxetine hydrochloride loaded film forming dermal gel enriched with methylcobalamin and geranium oil attenuates paclitaxel-induced peripheral neuropathy in rats. IBRO Rep. 2020;9:85–95. doi:10.1016/j.ibror.2020.07.006
- Misset JL. Oxaliplatin in practice. Br J Cancer. 1998;77 Suppl 4(Suppl 4):4–7. doi:10.1038/bjc.1998.428
- Kang L, Tian Y, Xu S, et al. Oxaliplatin-induced peripheral neuropathy: clinical features, mechanisms, prevention and treatment. J Neurol. 2021;268(9):3269–3282. doi:10.1007/s00415-020-09942-w
- Hershman DL, Lacchetti C, Dworkin RH, et al. Prevention and management of chemotherapy-induced peripheral neuropathy in survivors of adult cancers: American Society of Clinical Oncology clinical practice guideline. J Clin Oncol. 2014;32(18):1941–1967. doi:10.1200/JCO.2013.54.0914
- Veluchamy A, Hébert HL, Meng W, et al. Systematic review and meta-analysis of genetic risk factors for neuropathic pain. Pain. 2018;159(5):825–848. doi:10.1097/j.pain.0000000000001164
- Gadepalli A, Ummadisetty O, Akhilesh A, et al. Peripheral mu-opioid receptor activation by dermorphin [D-Arg2, Lys4] (1-4) amide alleviates behavioral and neurobiological aberrations in rat model of chemotherapy-induced neuropathic pain. Neurotherapeutics. 2023;21(1):e00302. doi:10.1016/j.neurot.2023.10.012
- Gadepalli A, Ummadisetty O, Akhilesh A, et al. Loperamide, a peripheral Mu-Opioid receptor agonist, attenuates chemotherapy-induced neuropathic pain in rats. Int Immunopharmacol. 2023;124(Pt B):110944. doi:10.1016/j.intimp.2023.110944
- Cavaletti G, Tredici G, Petruccioli MG, et al. Effects of different schedules of oxaliplatin treatment on the peripheral nervous system of the rat. Eur J Cancer. 2001;37(18):2457–2463. doi:10.1016/S0959-8049(01)00300-8
- McKeage MJ, Hsu T, Screnci D, et al. Nucleolar damage correlates with neurotoxicity induced by different platinum drugs. Br J Cancer. 2001;85(8):1219–1225. doi:10.1054/bjoc.2001.2024
- Barrett T, Wilhite SE, Ledoux P, et al. NCBI GEO: archive for functional genomics data sets--update. Nucleic Acids Res. 2013;41(Database issue):D991–995. doi:10.1093/nar/gks1193
- Luo W, Brouwer C. Pathview: an R/Bioconductor package for pathway-based data integration and visualization. Bioinformatics. 2013;29(14):1830–1831. doi:10.1093/bioinformatics/btt285
- Franceschini A, Szklarczyk D, Frankild S, et al. STRING v9.1: protein-protein interaction networks, with increased coverage and integration. Nucleic Acids Res. 2013;41(Database issue):D808–15. doi:10.1093/nar/gks1094
- Smoot ME, Ono K, Ruscheinski J, et al. Cytoscape 2.8: new features for data integration and network visualization. Bioinformatics. 2011;27(3):431–432. doi:10.1093/bioinformatics/btq675
- Kuleshov MV, Jones MR, Rouillard AD, et al. Enrichr: a comprehensive gene set enrichment analysis web server 2016 update. Nucleic Acids Res. 2016;44(W1):W90–W97. doi:10.1093/nar/gkw377
- Dittmer DP, Damania B. Kaposi sarcoma associated herpesvirus pathogenesis (KSHV)--an update. Curr Opin Virol. 2013;3(3):238–244. doi:10.1016/j.coviro.2013.05.012
- Aloisi F, Giovannoni G, Salvetti M. Epstein-Barr virus as a cause of multiple sclerosis: opportunities for prevention and therapy. Lancet Neurol. 2023;22(4):338–349. doi:10.1016/S1474-4422(22)00471-9
- Abdelkader NF, Ibrahim SM, Moustafa PE, et al. Inosine mitigated diabetic peripheral neuropathy via modulating GLO1/AGEs/RAGE/NF-κB/Nrf2 and TGF-β/PKC/TRPV1 signaling pathways. Biomed Pharmacother. 2022;145:112395.
- Wang X, Li Q, Han X, et al. Electroacupuncture Alleviates Diabetic Peripheral Neuropathy by Regulating Glycolipid-Related GLO/AGEs/RAGE Axis. Front Endocrinol. 2021;12:655591. doi:10.3389/fendo.2021.655591
- Oshitari T. Advanced Glycation End-Products and Diabetic Neuropathy of the Retina. Int J Mol Sci. 2023;24(3):2927. doi:10.3390/ijms24032927
- Liang YH, Chen GW, Li XS, et al. Guanosine-5’-triphosphate cyclohydrolase 1 regulated long noncoding RNAs are potential targets for microglial activation in neuropathic pain. Neural Regen Res. 2021;16(3):596–600. doi:10.4103/1673-5374.290914
- Kwon SY, Yeom JH, Joo JD. Ketamine reduces the induced spinal p38 MAPK and pro-inflammatory cytokines in a neuropathic rats. Korean J Anesthesiol. 2014;66(1):52–58. doi:10.4097/kjae.2014.66.1.52
- Rodriguez Parkitna J, Korostynski M, Kaminska-Chowaniec D, et al. Comparison of gene expression profiles in neuropathic and inflammatory pain. J Physiol Pharmacol. 2006;57(3):401–414.
- Ferrari LF, Khomula EV, Araldi D, et al. CD44 Signaling Mediates High Molecular Weight Hyaluronan-Induced Antihyperalgesia. J Neurosci. 2018;38(2):308–321. doi:10.1523/JNEUROSCI.2695-17.2017
- Bonet IJM, Staurengo-Ferrari L, Araldi D, et al. Second messengers mediating high-molecular-weight hyaluronan-induced antihyperalgesia in rats with chemotherapy-induced peripheral neuropathy. Pain. 2022;163(9):1728–1739. doi:10.1097/j.pain.0000000000002558
- Denes A, Thornton P, Rothwell NJ, et al. Inflammation and brain injury: acute cerebral ischaemia, peripheral and central inflammation. Brain Behav Immun. 2010;24(5):708–723. doi:10.1016/j.bbi.2009.09.010
- Wei J, Su W, Zhao Y, et al. Maresin 1 promotes nerve regeneration and alleviates neuropathic pain after nerve injury. J Neuroinflammation. 2022;19(1):32. doi:10.1186/s12974-022-02405-1
- Pan Z, Shan Q, Gu P, et al. miRNA-23a/CXCR4 regulates neuropathic pain via directly targeting TXNIP/NLRP3 inflammasome axis. J Neuroinflammation. 2018;15(1):29. doi:10.1186/s12974-018-1073-0
- Islam B, Stephenson J, Young B, et al. The Identification of Blood Biomarkers of Chronic Neuropathic Pain by Comparative Transcriptomics. Neuromolecular Med. 2022;24(3):320–338. doi:10.1007/s12017-021-08694-8
- Kuthati Y, Goutham Davuluri VN, Yang CP, et al. Melatonin MT2 receptor agonist IIK-7 produces antinociception by modulation of ROS and suppression of spinal microglial activation in neuropathic pain rats. J Pain Res. 2019;12:2473–2485. doi:10.2147/JPR.S214671
- Madiai F, Goettl VM, Hussain SR, et al. Anti-fibroblast growth factor-2 antibodies attenuate mechanical allodynia in a rat model of neuropathic pain. J Mol Neurosci. 2005;27(3):315–324. doi:10.1385/JMN:27:3:315