Abstract
Objective: Patients with triple-class refractory (TCR) multiple myeloma (MM) have limited treatment options and poor prognoses. This high unmet need has prompted the development of new therapies allowing for improved outcomes for these patients. Recently, new targeted therapies for the treatment of patients with relapsed or refractory MM have been approved based on single-arm clinical trial results. Real-world (RW) data enable a better understanding of the effectiveness of new therapies in clinical practice and provide external controls for single-arm studies. However, using RW data to identify patients with TCR MM is challenging and subject to limitations. Methods: In this retrospective cohort study of an analysis of the COTA electronic health record (EHR) database, we used four algorithms to define refractory status and created four groups of patients with TCR MM initiating post-TCR therapy. Each algorithm relied on slightly different criteria to identify TCR patients, but all were based on the International Myeloma Working Group (IMWG)-derived and/or healthcare provider (HCP)-reported progressions within the database. Results: A total of 3815 patients with newly diagnosed MM met the eligibility criteria for this study. The choice of the algorithm did not impact the characteristics of identified patients with TCR MM (Algorithm 1 [n = 404], Algorithm 2 [n = 123], Algorithm 3 [n = 404], and Algorithm 4 [n = 375]), including their demographic and disease characteristics, MM treatment history, or treatment patterns received after becoming TCR. However, identifying TCR MM using a combination of IMWG-derived and HCP-reported progressions allowed up to a 70% increase in the size of the identified group of patients compared with using only IMWG-derived progressions. Conclusion: In RW settings, progressions from both IMWG-derived data and physician reports may be used to identify patients with TCR MM.
Introduction
Multiple myeloma (MM) is a rare hematologic malignancy with substantial mortality. In the United States, an estimated 32,270 new MM cases are diagnosed, and approximately 12,830 individuals die from the disease each yearCitation1. Over the course of the disease, patients are treated with successive lines of therapy (LOTs) that include complex regimens. Patients who are treated with and have disease refractory to at least one agent from all three standard-of-care classes of therapy used in MM (proteasome inhibitors [PIs], immunomodulatory agents [IMiDs], and anti-CD38 monoclonal antibodies) are considered to have triple-class refractory (TCR) MMCitation2. Treatment of TCR MM presents a therapeutic challenge because of the inherent clonal heterogeneity and genetic instability of MM tumor cells influencing the development of therapeutic resistanceCitation3. Because of limited therapeutic approaches, patients with TCR MM have a poor prognosis, with reported median overall survival (OS) of 9.2 to 10.8 monthsCitation4–6. Given high unmet needs due to poor prognosis and limited treatment options for patients with TCR MM, there has been a push in the past years for the development of new therapies that may improve patient outcomes while maintaining manageable levels of toxicityCitation7. Several treatment options for such patients are available commercially, and several treatments are under investigation in clinical trialsCitation1. While real-world (RW) evidence is needed to better understand the effectiveness of new therapies in clinical practice settings or to provide external controls for single-arm clinical trials, RW studies on patients with MM initiating treatment after becoming TCR remain scarce due to the difficulty in identifying such patients in most claims and electronic health record (EHR) data sets. Specifically, the definition of TCR assumes that a patient meets the International Myeloma Working Group (IMWG) criteria for progression requiring assessment of either serum protein electrophoresis (SPEP), 24-h urine protein electrophoresis (UPEP), serum-free light chain (sFLC) levels, radiological and bone marrow assessments, or serum calcium levelsCitation8. However, these measurements are frequently unavailable in most RW data. A study based on the analysis of the US nationwide Flatiron Health EHR-derived database reported that only 62% of patients with MM had at least one measurable SPEP or 24-h UPEPCitation9. Moreover, the documentation of the radiological and bone marrow assessments or the count of circulating plasma cells is not within a discrete field and therefore not easily extracted in EHRs. Because of these limitations, some studies based on the analysis of RW data used the healthcare provider (HCP) reports to ascertain progressionsCitation10.
In this study, we aimed to identify patients with TCR MM initiating new LOTs using four different algorithms applied to EHR data. Each algorithm relied on slightly different criteria to identify TCR, but all were based on IMWG-derived and/or HCP-reported progressions within the COTA database, a large US-based EHR data source. To characterize variability in patients identified using each of the four approaches, we produced descriptive statistics comparing patient demographics, clinical disease presentation, comorbidity profiles, and MM therapeutic patterns.
Materials and methods
Study design and patients
This was a population-based retrospective cohort study using de-identified data from a large US EHR database, COTACitation11. Patients with a diagnosis of MM and aged ≥18 years at the time of the first MM diagnosis available in the database any time before 1 January 2022, were eligible for inclusion in the study. COTA excludes those patients who before or at the date of their first MM diagnosis had concurrent primary malignancies or had a diagnosis of smoldering, asymptomatic MM, or monoclonal gammopathy of undetermined significance. We followed patients from the date of the first MM diagnosis until the earliest of the following: death, the latest available patient record, or the end of the study (30 June 2022), whichever occurred first. The event of interest was the initiation of a new LOT after becoming TCR (post-TCR). The TCR status was identified consecutively using four different algorithms, thus, four groups of patients with MM initiating a LOT post-TCR were identified in this study. summarizes the study design.
Figure 1. Study design. Abbreviations. LOT, line of therapy; MM, multiple myeloma; TC, triple-class refractory.
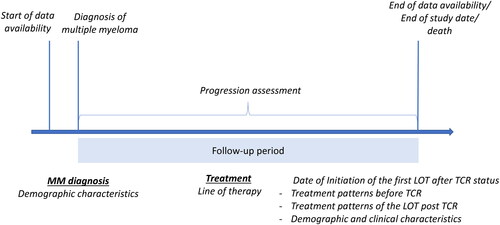
Approval from an institutional review board/independent ethics committee was not required for this study as only de-identified secondary data sources and anonymized medical record data from EHRs were used.
Data source
Patients with MM were identified within the COTA databaseCitation11. COTA collects information from the EHRs of oncology HCP sites, including academic institutions, community centers, and hospital systems representing approximately 500,000 patients from over 200 sites of care in the US. Information is derived from structured and unstructured data sources. Data elements are standardized across sources and ontologies to create a single, structured data set to cover the full longitudinal history of a patient’s clinical care. COTA has approaches to integrate data from variable clinical settings to collect information from the time of initial cancer diagnosis to the most recent documentation in the EHR. The data are curated to exclude patients with insufficient documentation data (e.g. no clinician notes available, data collection challenges due to the patient not being evaluated at an accessible oncology provider site) or those having significant gaps in their patient history in the EHRCitation12. For patients with MM, COTA provides specific databases that are dedicated to reporting factors relevant to the experience of a patient with MM and includes data sets containing abstracted information on bone lesions, plasmacytomas, radiation therapy, and adverse events. For disease progression, COTA provides derived data based on adapted IMWG criteria and data on progressions reported by HCPs as the reason for treatment discontinuation.
LOT definition according to COTA
COTA defines a LOT as a sequence of therapies received by a patient. A LOT may consist of a complete cycle of a single therapy, a combination of therapies (i.e. a regimen), or more than one regimen of anti-MM therapiesCitation12. A LOT may have multiple regimens because one regimen is considered maintenance, or because small changes in treatments were made from one regimen to the next (e.g. substituting one of the regimen therapies less than 30 days after the LOT started). A new LOT is initiated after discontinuation of a regimen because of HCP-reported progression or start of a new therapy (either after discontinuation of previous treatments or following a pre-determined gap period based on type of treatment). All non-supportive systemic treatments recorded in the EHR other than surgery are included in LOTs.
Information on disease progression and refractory status available in COTA
COTA identifies progression using two sources: IMWG-derived progressions and abstracted HCP-reported progressions.
IMWG-derived progressions are tied, by COTA, to a LOT, and a specific progression date is provided. COTA does not indicate which treatments within a LOT the progression is associated with. The markers/laboratory types prioritized by COTA to identify progression include SPEP, UPEP, the difference between involved and uninvolved FLC levels, or bone marrow plasma cell percentage. IMWG-derived progression is ascertained after comparing the values of the marker/laboratory values measured during the baseline time window and those measured during the progression time window. The progression time window begins 10 days following initiation of therapy in the current LOT or from the baseline value, whichever interval of time is longer, and ends at the time of initiation of the next LOT. In the case of the last LOT, the progression time window ends 60 days after the end of therapy in the final LOT.
Unlike IMWG-derived progressions, HCP-reported progressions are tied to a specific regimen and not to a full LOT. HCP-reported disease progressions include progressions given as the reason for discontinuation of a regimen and no specific date for these progressions is provided. Some progressions reported by HCPs did not have corresponding IMWG-derived progressions. This might be due to the unavailability of laboratory measurements at baseline or response assessments or available laboratory measurements not meeting the criteria for IMWG progression. Similarly, some IMWG-derived progressions did not have corresponding HCP-reported progressions, likely due to differences in availability of HCP comments.
TCR definition
TCR patients were defined as those who were refractory to ≥1 IMiD (i.e. pomalidomide, lenalidomide, or thalidomide), ≥1 PI (i.e. bortezomib, carfilzomib, or ixazomib), and ≥1 anti-CD38 antibody treatment (i.e. daratumumab or isatuximab). The IMiD, PI, and anti-CD38 antibody treatments were allowed to be used as part of distinct LOTs or within the same line.
We used four algorithms to define the refractory status and create four groups of patients with MM initiating a post-TCR LOT (see and , for the summary of the algorithms). The algorithms applied different strategies for linking IMWG-derived progressions to the associated therapies, given the progression timing relative to the LOT (). Furthermore, the algorithms differed by considering or not considering the HCP-reported progressions provided by COTA as reasons for the regimen discontinuation (). For algorithms in which both IMWG-derived and HCP-reported progressions were considered, all available progression information was used to define refractory status for patients. , show two observed patient examples and demonstrate how these algorithms were applied to identify TCR MM. In the first example (), the patient was considered to have TCR MM with all algorithms during the same LOT. However, in the second example (), the patient was only considered to have TCR MM using three algorithms, and the timing of TCR identification varied with each algorithm.
Figure 2. Summary of similarities and differences in the determination of refractory status and TCR identification criteria for Algorithms 1-4 using IMWG-derived progressions (a) and HCP-reported progressions (b). Abbreviations. HCP, healthcare provider; IMWG, International Myeloma Working Group; N/A, not applicable; TCR, triple-class refractory.
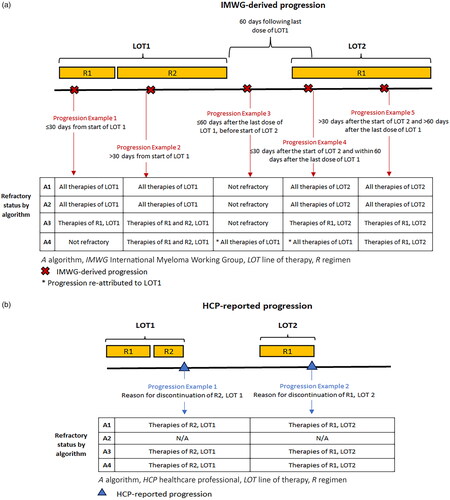
Figure 3. Patient examples of application of Algorithms 1–4 for TCR identification. Patient example 1 (a) and patient example 2 (b).
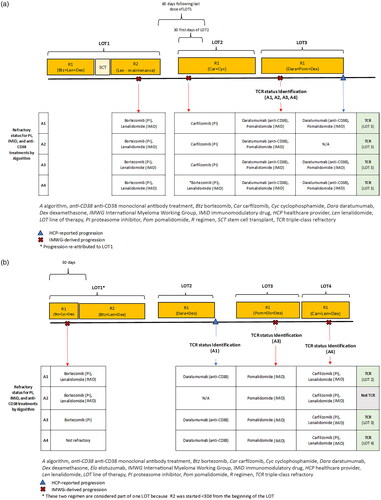
Table 1. Summary of algorithms for TCR identification.
Algorithm 1: refractory status was identified using both IMWG- and HCP-reported progressions as recorded in the COTA database
Patients were considered refractory to a given therapy if their refractory status was determined either through IMWG-derived or HCP-reported progressions, as described below.
IMWG-derived progressions
Patients with TCR MM were identified using the information on IMWG-derived progressions as provided by COTA. With this algorithm, patients with disease progression were considered refractory to all therapies in all regimens in the associated LOT ( and ). For example, in , a patient’s first LOT consisted of two regimens (R1: bortezomib, cyclophosphamide, dexamethasone; R2: bortezomib, lenalidomide, dexamethasone), and the patient experienced progression identified based on IMWG criteria after the initiation of their first regimen. Using Algorithm 1, this patient was considered refractory to all therapies in all regimens of the first LOT. For the purposes of TCR MM identification, they were considered refractory to a PI (bortezomib) and an IMID (lenalidomide) based on this progression.
HCP-reported progressions
Algorithm 1 also accounted for HCP-reported progressions. Patients were considered refractory only to therapies in the regimens that were discontinued due to the progression ( and ). For example, the second LOT of the patient shown in consisted of one regimen (R1: daratumumab, dexamethasone), which was discontinued due to an HCP-reported progression. This patient was considered refractory to an anti-CD38 monoclonal antibody (daratumumab) based on this progression.
Algorithm 2: refractory status identified using only IMWG progressions as recorded in the COTA database
Patients were considered refractory to a given therapy only if their refractory status was determined through the IMWG-derived progressions as HCP-reported progressions were not considered, as described below.
IMWG-derived progressions
The definition of IMWG-derived progressions was identical to that of Algorithm 1. Patients with IMWG-derived progression were considered refractory to all therapies in all regimens in the associated LOT ( and ).
HCP-reported progressions
HCP-reported progressions were not considered by Algorithm 2 (). Therefore, the patient example in would not have been identified as having TCR MM using Algorithm 2.
Algorithm 3: refractory status identified using both IMWG and HCP progressions with the modified definition of progression-associated regimens
Patients were considered refractory to a given therapy if their refractory status was determined through either IMWG-derived or HCP-reported progressions, as described below.
IMWG-derived progressions
To minimize potential misclassification of the attributable regimens, Algorithm 3 specified that when a progression was identified based on IMWG criteria, the patient was considered refractory only to therapies in regimens in the associated LOT received before the progression ( and ). For example, consider the patient’s first LOT in , which consisted of two regimens (R1: bortezomib, cyclophosphamide, dexamethasone; R2: bortezomib, lenalidomide, dexamethasone). The patient experienced a progression identified based on IMWG criteria while on their first regimen. Using Algorithm 3, this patient was considered refractory to all therapies of the first regimen but not refractory to therapies of the second regimen. This differs from both Algorithms 1 and 2, which would consider this patient to be refractory to all therapies of both regimens of the first LOT. Therefore, using this algorithm for TCR identification, the patient was only considered refractory to a PI (bortezomib) based on this progression. Whereas, using Algorithms 1 and 2 they were considered refractory to both a PI (bortezomib) and an IMID (lenalidomide).
HCP-reported progressions
Algorithm 3 also accounted for HCP-reported progressions. Patients were considered refractory only to therapies in the regimen that was discontinued due to the progression ( and ). Therefore, the patient example described in was considered to have disease refractory to an anti-CD38 monoclonal antibody (daratumumab) based on HCP-reported progressions using Algorithm 3.
Algorithm 4: refractory status identified using both IMWG and HCP progressions with a modified attribution of progression-associated LOT
Patients were considered refractory to a given therapy if their refractory status was determined through either IMWG-derived or HCP-reported progressions, as described below.
IMWG-derived progressions
Algorithm 4 was akin to Algorithm 3; however, to minimize potential misclassification of the attributable LOT and regimens, Algorithm 4 considered not only the timing of progressions within a given LOT but also the timing of progression relative to the initiation of the following LOT or time of discontinuation of the preceding LOT. Patients with recorded IMWG-derived progression while on a therapy, or up to 60 days following the completion of a therapy (if there was no subsequent LOT or the duration of the subsequent LOT was ≤30 days at the time of disease progression), were classified as refractory to only those regimens in the LOT that were received before the progression. If progression occurred within the first 30 days of the LOT initiation, it was re-attributed to all therapies in the previous LOT if the progression fell within the 60 days following the end of the previous LOT ( and ). For example, the patient shown in experienced an IMWG-derived progression at the beginning of their second LOT. As this progression occurred within 30 days of the start of their second LOT and within 60 days of the end of their first LOT, using Algorithm 4, this progression was re-attributed to the first LOT. Therefore, based on this progression the patient was considered refractory to a PI (bortezomib) and an IMID (lenalidomide). However, with Algorithms 1–3, where the progression is not re-attributed to a different LOT, the patient was considered refractory to a PI based on this progression (carfilzomib).
HCP-reported progressions
Algorithm 4 also accounted for HCP-reported progressions. Patients were considered refractory only to therapies in the regimen that were discontinued due to progression ( and ).
Prognostic characteristics measured for patients identified by four algorithms
A variety of prognostic characteristics were measured for each identified patient with MM initiating a new LOT after becoming TCR. Demographics, including age and sex, were measured at the date of the first MM diagnosis and the date of initiation of post-TCR LOT. Disease characteristics (International Staging System [ISS] stage, Eastern Cooperative Oncology Group performance status, time since initial MM diagnosis until the date of initiation of post-TCR LOT, cytogenetic risk, extramedullary plasmacytoma [defined as plasmacytoma of soft tissue or unspecified plasmacytoma], and presence of bone lesions) and laboratory test results (aspartate aminotransferase, alanine aminotransferase, creatinine clearance, absolute neutrophil count, platelets, hemoglobin, calcium in serum or plasma, lactate dehydrogenase, beta-2 microglobulin, serum albumin, total bilirubin, serum M-protein [SPEP], urinary M-protein [UPEP], serum immunoglobulin kappa to lambda FLC ratio [sFLC K/L], and plasma cells in bone marrow), MM treatment history (duration of prior therapy, number of LOTs before TCR status, SCT, radiation therapy, prior exposure to B-cell maturation antigen [BCMA]–directed therapy (including belantamab mafodotin and idecabtagene vicleucel), prior treatment with selinexor, and history of any-grade peripheral sensory or motor neuropathy in those with prior BCMA-directed therapy), and Charlson Comorbidity Index score were measured during the period between the MM diagnosis and the date of initiation of the post-TCR LOT. Treatment patterns were also measured for the LOT initiated post TCR (therapies received, including BCMA-directed therapy or selinexor).
Data analyses
Descriptive statistics were used to characterize the demographics, baseline clinical characteristics, and treatment patterns of each group of patients with MM initiating a new LOT post TCR identified by four algorithms. Continuous variables were summarized using mean (standard deviation) and median (interquartile range). Categorical variables were described using frequencies and percentages. In addition, for each variable, the number of observations associated with a missing value was reported. To compare the distribution of continuous variables across the four groups of TCR patients, we used the Kruskal-Wallis test for not normally distributed variables or the analysis of variance test for normally distributed variables. To compare dichotomous and categorical variables, we used the Chi-square or Fisher’s exact test. A p value ≤0.05 was considered statistically significant.
Results
A total of 3815 patients newly diagnosed with MM who met the eligibility criteria were included in the study. The mean age at the first MM diagnosis was 64 years (±11 years), and 56% of patients were male. Among patients with MM, the number of identified patients initiating a new LOT post TCR was 404 with Algorithms 1 and 3, 375 with Algorithm 4, and 123 with Algorithm 2. The median time from the first MM diagnosis to the date of initiation of the first post-TCR LOT was approximately 4.5 years when using Algorithms 1, 3, and 4, and 5.4 years when using Algorithm 2 (p = 0.5500).
shows the baseline characteristics and comorbidity burden, shows MM characteristics, and shows treatment history of four groups of identified patients with TCR MM initiating a new LOT. See Supplementary Table 1 for additional demographics and laboratory results not shown in , and . Across all four groups, the mean age of identified patients with TCR MM was approximately 67 years, and 55% of patients were male (). The median Charlson Comorbidity Index score measured any time before the date of initiation of post-TCR LOT was 3.0 across all four groups of identified patients. TCR patients identified by all four algorithms had similar MM characteristics (): approximately 80% had Eastern Cooperative Oncology Group performance status scores measured at the date of initiation of post-TCR LOT of ≤2 (p = 0.9993), approximately 60% had a history of bone lesions (p = 0.9979), and 17%-18% had a history of extramedullary plasmacytoma during the period between the first MM diagnosis and the date of initiation of post-TCR LOT (p = 0.9719). For approximately two-thirds of identified patients in the four groups, information on the ISS stage was not possible to derive due to a lack of measurements on serum beta-2 microglobulin or serum albumin. For those with available ISS stage, the proportions of patients in each ISS stage were largely similar across all four groups (p = 0.9989): ISS stage 1 had 13% of patients identified by Algorithms 1, 3, and 4 and 15% of patients identified by Algorithm 2, ISS stage 2 had 11% of patients identified by Algorithms 1, 3, and 4 and 12% of patients identified by Algorithm 2, and ISS stage 3 had 6% of patients identified by Algorithms 1, 3, and 4 and 5% of patients identified by Algorithm 2. The distribution of ISS components, including serum beta-2 microglobulin and serum albumin, did not differ between groups (p = 0.9981 and p = 0.9848, respectively).
Table 2a. Demographics characteristics of TCR samples identified using four algorithms.
Table 2b. Disease characteristics of TCR samples identified using four algorithms.
Table 2c. Treatment history of TCR samples identified using four algorithms.
Patients identified by different algorithms had similar MM treatment histories before the date of initiation of the post-TCR LOT (). Thus, with all algorithms, approximately 60% of patients received SCT during the period between MM diagnosis and the date of initiation of the post-TCR LOT (57%, 60%, 57%, and 57%, for Algorithms 1–4, respectively; p = 0.9978). The median number of LOTs used before becoming TCR was 6 for all groups (p = 0.4998). The median duration of treatment for these prior LOTs was 3.2 years for the group identified with Algorithms 1 and 3, 3.5 years for Algorithm 2, and 3.3 years for Algorithm 4 (p = 0.8105). The median time from diagnosis to initiation of the first LOT after becoming TCR, which includes time on treatment and any gaps between treatment, was 4.5 years for the group identified with Algorithms 1 and 3, 5.4 years for Algorithm 2, and 4.6 years for Algorithm 4 (p = 0.5500). Algorithms 2 and 4 identified one patient who received BCMA-directed therapy before becoming TCR. Cytogenetic abnormalities associated with poor outcomes in patients with MM, including the deletion of 17p and presence of t(14,16) or t(4,14), were found in 22% of patients identified by Algorithms 1 and 3, 20% identified by Algorithm 2, and 21% identified by Algorithm 4 (p = 0.9124).
A significantly higher proportion of patients identified by Algorithm 2 had recorded information on SPEP, UPEP, and sFLC K/L compared with patients identified by Algorithms 1, 3, and 4 (SPEP: 91%, 72%, 72%, and 71%, p < 0.0001; UPEP: 43%, 35%, 35%, and 35%, p = 0.3443; FLC K/L: 94%, 76%, 76%, and 74%, p < 0.0001, respectively; ). Patients identified with Algorithm 2 had significantly higher median SPEP levels compared with patients identified using Algorithms 1, 3, and 4 (median SPEP 1.9 g/dL for Algorithm 2 and 0.8 g/dL for Algorithms 1, 3, and 4; p < 0.0001).
shows treatments received in the LOT initiated post TCR for each group of patients identified with the four algorithms. There was no statistically significant difference in treatment patterns received in the LOT initiated after TCR between the four groups of patients. The three most common regimens of the LOT initiated after TCR were daratumumab-pomalidomide-dexamethasone (7%, 6%, 7%, and 7% for Algorithms 1–4, respectively), elotuzumab-pomalidomide-dexamethasone (5%, 0%, 5%, and 5%, respectively), and carfilzomib-cyclophosphamide-dexamethasone (5%, 7%, 5%, and 5%, respectively). For all algorithms, fewer than 5% of patients received SCTs in the LOT initiated at the index date (p = 1.0000). A higher proportion of patients identified with Algorithm 2 received selinexor as part of their index treatment compared with patients identified using Algorithms 1, 3, and 4; however, the difference in the proportion of patients with selinexor was not statistically significant (p = 0.4530). The number of patients treated with BCMA-directed therapies during their index LOT ranged from 2 (using Algorithm 2) to 4 (using Algorithms 1 and 3).
Table 3. Treatment patterns of the first LOT initiated post TCR in TCR samples identified using four algorithms.
Discussion
In this study based on the analysis of EHR data, we identified patients with MM initiating a new LOT after becoming TCR using four algorithms to define TCR MM. We compared descriptive statistics and profiles of patients identified with each of the four algorithms. The study found that the choice of the algorithm did not impact the characteristics of patients with TCR MM, including their demographic and disease characteristics, MM treatment history, or treatment patterns received after becoming TCR.
Using Algorithm 1, patients with IMWG-derived progression were defined as being refractory to all therapies in the given LOT regardless of the timing of the progression. This may lead to the misclassification of assigning refractory status to the therapies within the cycles that had been completed after the date of progression. Algorithms 3 and 4 were designed to minimize potential misclassification of either the progression-attributable treatments in the same LOT (Algorithm 3) or progression-attributable treatments in the immediately preceding or following LOT (Algorithm 4). We did not find evidence of significant misclassification of the progression-attributable regimens within the same LOT, as both Algorithm 1 and Algorithm 3 yielded the same number of identified patients with similar distributions of measured characteristics. Compared with Algorithms 1 and 3, the group of identified patients was approximately 7% smaller when using Algorithm 4, which accounts for possible misclassification of progression-attributable LOT and regimens. However, we determined that identifying patients with TCR MM using a combination of IMWG-derived progressions and HCP-reported progressions (Algorithms 1, 3, and 4) allowed up to a 70% increase in the size of the identified group of patients compared with using only IMWG-derived progressions (Algorithm 2). Previous studies reported that the OS of patients with MM with progression diagnosed using clinical features may be worse compared with patients with progression diagnosed based on laboratory resultsCitation13,Citation14. However, in our study, the distribution of prognostic characteristics identified by previous research as strongly and consistently associated with efficacy outcomes in patients with MM did not differ meaningfully across all four groups. Thus, patients identified by the four algorithms described here had a similar distribution of age, which may be a predictor of OSCitation15–17 and progression-free survival (PFS)Citation17,Citation18; sex, which may be associated with OSCitation19,Citation20; cytogenetic abnormalities such as deletion 17p, t(14,16), and t(4,14), which have been shown to be predictors of OSCitation21–23 and PFSCitation24–26; prior number of LOTs, which may be a predictor of OSCitation23,Citation27,Citation28 and PFSCitation16,Citation20,Citation23,Citation25,Citation28; and ISS stage and ISS components (serum beta-2 microglobulin and serum albumin), which have been found to be associated with OSCitation20,Citation29,Citation30 and PFSCitation17,Citation20.
Our study was based on the analysis of the COTA database. The markers/laboratory types prioritized by COTA for IMWG-derived progression include SPEP, UPEP, the difference between involved and uninvolved FLC levels, and/or bone marrow plasma cell percentageCitation11. This may explain our finding that in the group of patients identified using only IMWG-derived progressions (Algorithm 2), a significantly higher proportion had recorded information on SPEP, UPEP, and sFLC K/L compared with patients identified by algorithms that used both IMWG-derived and HCP-reported progressions. Among those with available laboratory results, patients identified using only IMWG-derived progressions had significantly higher median SPEP levels compared with patients identified using IMWG-derived and HCP-reported progressions. This may be explained by the fact that some HCP-reported progressions may have been diagnosed based on IMWG criteria that are not prioritized by the COTA definition (radiological assessments or hypercalcemia) or based on clinical features.
The IMWG criteria to assess the treatment response in patients with MM allow a direct comparison of efficacy endpoints between clinical trials testing new treatment strategies for MMCitation8. Some RW data studies implemented the IMWG criteria to identify patients with relapsed or refractory MMCitation5,Citation6,Citation31,Citation32. For example, the KarMMa-RW study, a noninterventional retrospective cohort study, compared efficacy outcomes in patients with relapsed or refractory MM treated with idecabtagene vicleucel in the KarMMa clinical study with those treated with standard of care in RW settings in Europe and the US (from RW clinical sites, the Connect MM Registry, and external research databases)Citation32. The MAMMOTH study, based on the analysis of RW data collected from 14 US academic institutions, described the outcomes in patients with MM who were refractory to anti-CD38 therapies daratumumab and isatuximabCitation5. Another example is the study by Kumar et al.Citation31 on the natural history of myeloma refractory to IMiDs and PIs in patients treated in multiple centers across North America, Europe, and Asia-Pacific. However, limitations and methodological challenges are associated with the operationalization of the IMWG criteria for disease progression when using RW data. A key limitation is the quality and completeness of the recorded data on laboratory tests or radiological assessmentsCitation9. Therefore, some studies based on the analysis of RW data use all data readily available in EHRs to capture the progressions, including physician-reported progressionCitation10,Citation33,Citation34. The results of our study support this strategy showing that physician-reported progression can generate additional evidence of disease progression without compromising the characteristics of identified TCR patients.
The 2015 guidelines presented by Rajkumar et al. established clear criteria for determining number of prior LOTs in MM patientsCitation35. The COTA database has its own definition of LOTs that generally aligns well with the guidelines, though it includes more specifications to account for the various treatment patterns observed in RW settings. As LOTs are constructed by COTA, these LOTs are standardized for all MM research conducted using this data source. In practice, there are two main differences between the two sources. The first is in the management of SCTs, where the guidelines in Rajkumar et al. suggest that only one SCT be included per LOT. The COTA definition, however, allows for the possibility of more than one SCT per LOT. The second difference is in regard to progressions. Both the COTA and Rajkumar et al. LOT definitions state that the LOT should be advanced when all therapies in the current regimen are discontinued and a new regimen is started. However, if therapies are discontinued due to HCP-reported progression, COTA’s definition specifies that the LOT advances regardless of whether all regimen therapies were discontinued. Given the similarity of LOTs defined using the COTA and Rajkumar et al. approaches, our findings are relevant for researchers seeking to identify TCR MM patients for RW research, including creation of external controls for single-arm clinical trials.
This study has several limitations. First, there was no gold standard for TCR to validate the performance of the algorithms. Instead, the four algorithms were compared with each other, and the performance was assessed based on expert knowledge. Second, missing data and the accuracy of recorded data on disease characteristics, laboratory results, and comorbidities may introduce an information bias. As these data are collected from RW EHRs from individual oncology practices, patients tend to have a very short clinical history before their first MM diagnosis. Although COTA has implemented the data curation process to span the entire patient’s journey after the MM diagnosis, conditions that are part of the patient’s clinical history before MM diagnosis are likely underestimated for patients with TCR MM in all four groups. Next, because COTA provides only the quarter and the year of diagnosis, we define the day of MM diagnosis as the midpoint in the quarter. Therefore, the time between the first MM diagnosis and the index date may be misclassified. However, most likely this misclassification was non-differential across four groups of patients with TCR MM, as it was non-differential between patients identified using IMWG-derived or HCP-reported progression. Furthermore, as all of our analyses were performed using the COTA databases, this may limit the generalizability of our findings to other EHR databases because COTA has its specific algorithms for defining LOT and progression. However, these algorithms are based on standardized definitions and align well with established guidelines, which increases the generalizability of our resultsCitation8,Citation35.
Conclusion
Overall, in RW settings, progressions from both IMWG-derived data and physician reports may be used to identify patients with TCR MM. Decisions regarding the modification of progression-associated regimens or progression-associated LOTs did not impact the demographic and disease characteristics of identified patients with TCR MM in this analysis of the COTA EHR database. Identification of other refractory populations (e.g. double-refractory, penta-refractory) should also be tested and compared using this algorithmic approach. Further validation studies are needed to evaluate the validity of physician-reported progressions and IMWG-derived progressions in other EHR databases.
Transparency
Declaration of funding
This study was supported by research funding from Pfizer.
Author contributions
LS, AM, PH, SMAJ, MD, FV, JR, and AS designed and wrote the study protocol, AF was responsible for extracting and analyzing data. All authors interpreted the data and contributed to the development of the manuscript.
Sinyavskaya (CMRO)_TCR algorithm MS_Suppl Table 1.docx
Download MS Word (20.3 KB)Acknowledgements
Editorial support was provided by Nucleus Global and funded by Pfizer.
Declaration of financial/other relationships
AM, PH, MD, JR, and AS are employed by Pfizer. LS, AF, SMAJ, and FV are employees of STATLOG, a consultancy company that received funds from Pfizer for the conduct of the current study.
Peer reviewers on this manuscript have no relevant financial or other relationships to disclose.
Data availability statement
The data that support the findings of this study are available from COTA, Inc, but restrictions apply to the availability of these data, which were used under a license agreement for the current study and, accordingly, are not publicly available. Access to the data can be requested by contacting COTA, Inc.
References
- Madduri D, Hagiwara M, Parikh K, et al. Real-world treatment patterns, healthcare use and costs in triple-class exposed relapsed and refractory multiple myeloma patients in the USA. Future Oncol. 2021;17(5):503–515. doi: 10.2217/fon-2020-1003.
- Bal S, Malek E, Kansagra A, et al. Treatment outcomes of triple class refractory multiple myeloma: a benchmark for new therapies. Leukemia. 2022;36(3):877–880. doi: 10.1038/s41375-021-01471-3.
- Nooka AK, Kastritis E, Dimopoulos MA, et al. Treatment options for relapsed and refractory multiple myeloma. Blood. 2015;125(20):3085–3099. doi: 10.1182/blood-2014-11-568923.
- Mikhael J. Treatment options for triple-class refractory multiple myeloma. Clin Lymphoma Myeloma Leuk. 2020;20(1):1–7. doi: 10.1016/j.clml.2019.09.621.
- Gandhi UH, Cornell RF, Lakshman A, et al. Outcomes of patients with multiple myeloma refractory to CD38-targeted monoclonal antibody therapy. Leukemia. 2019;33(9):2266–2275. doi: 10.1038/s41375-019-0435-7.
- Lee HC, Ramasamy K, Weisel K, et al. Treatment patterns, survival, quality of life, and healthcare resource use among patients with triple-class refractory multiple myeloma in US clinical practice: findings from the Connect MM Disease Registry. Clin Lymphoma Myeloma Leuk. 2023;23(2):112–122. doi: 10.1016/j.clml.2022.11.008.
- Kumar S, Baizer L, Callander NS, et al. Gaps and opportunities in the treatment of relapsed-refractory multiple myeloma: consensus recommendations of the NCI Multiple Myeloma Steering Committee. Blood Cancer J. 2022;12(6):98. doi: 10.1038/s41408-022-00695-5.
- Kumar S, Paiva B, Anderson KC, et al. International Myeloma Working Group consensus criteria for response and minimal residual disease assessment in multiple myeloma. Lancet Oncol. 2016;17(8):e328–e346. doi: 10.1016/S1470-2045(16)30206-6.
- Foster RB, Tromanhauser M, Gayer C, et al. Approximating International Myeloma Working Group uniform response criteria to derive response for multiple myeloma (MM) patients using data from electronic health records (EHR). Blood. 2019;134(Supplement_1):4727–4727. Abstract 4727, doi: 10.1182/blood-2019-127181.
- Lane D, Norden AD, Belli AJ, et al. Assessing real-world clinical response in patients with multiple myeloma (MM): a survey of the literature. J Clin Oncol. 2020;38(15_suppl):e19260–e19260. doi: 10.1200/JCO.2020.38.15_suppl.e19260.
- COTA Healthcare [Internet]. Life sciences. [cited 2023 Aug 28] Available from: https://cotahealthcare.com/life-sciences/.
- COTA Healthcare. Multiple myeloma real-world evidence. New York, NY: COTA Healthcare; 2022.
- Goldman-Mazur S, Visram A, Kapoor P, et al. Outcomes after biochemical or clinical progression in patients with multiple myeloma. Blood Adv. 2023;7(6):909–917. doi: 10.1182/bloodadvances.2022007082.
- Chakraborty R, Liu HD, Rybicki L, et al. Progression with clinical features is associated with worse subsequent survival in multiple myeloma. Am J Hematol. 2019;94(4):439–445. doi: 10.1002/ajh.25415.
- Soyer N, Patır P, Uysal A, et al. Efficacy and safety of lenalidomide and dexamethasone in patients with relapsed/refractory multiple myeloma: a real-life experience. Turk J Med Sci. 2018;48(4):777–785. doi: 10.3906/sag-1712-160.
- Kobayashi T, Kuroda J, Fuchida S, et al. Impact of early use of lenalidomide and low-dose dexamethasone on clinical outcomes in patients with relapsed/refractory multiple myeloma. Int J Hematol. 2015;101(1):37–45. doi: 10.1007/s12185-014-1696-6.
- Jethava YS, Mitchell A, Epstein J, et al. Adverse metaphase cytogenetics can be overcome by adding bortezomib and thalidomide to fractionated melphalan transplants. Clin Cancer Res. 2017;23(11):2665–2672. doi: 10.1158/1078-0432.CCR-15-2620.
- Moreau P, Stewart KA, Dimopoulos M, et al. Once-weekly (70 mg/m2) vs twice-weekly (56 mg/m2) dosing of carfilzomib in patients with relapsed or refractory multiple myeloma: a post hoc analysis of the ENDEAVOR, A.R.R.O.W., and CHAMPION-1 trials. Cancer Med. 2020;9(9):2989–2996. doi: 10.1002/cam4.2945.
- Sharkey MM, McKavanagh D, Walpole E, et al. Using pharmacy management systems for research: survival outcomes for lenalidomide in multiple myeloma in the clinical setting. Int J Clin Pharm. 2017;39(4):836–843. doi: 10.1007/s11096-017-0480-0.
- Jelínek T, Maisnar V, Pour L, et al. Adjusted comparison of daratumumab monotherapy versus real-world historical control data from the Czech Republic in heavily pretreated and highly refractory multiple myeloma patients. Curr Med Res Opin. 2018;34(5):775–783. doi: 10.1080/03007995.2017.1410121.
- Sun C, Ye JN, Mao JJ, et al. Impact of extended treatment interval on the prognosis of multiple myeloma patients: a retrospective study. Oncol Res Treat. 2020;43(11):592–604. doi: 10.1159/000508451.
- Smetana J, Berankova K, Zaoralova R, et al. Gain(1)(q21) is an unfavorable genetic prognostic factor for patients with relapsed multiple myeloma treated with thalidomide but not for those treated with bortezomib. Clin Lymphoma Myeloma Leuk. 2013;13(2):123–130. doi: 10.1016/j.clml.2012.11.012.
- Rocchi S, Tacchetti P, Pantani L, et al. A real-world efficacy and safety analysis of combined carfilzomib, lenalidomide, and dexamethasone (KRd) in relapsed/refractory multiple myeloma. Hematol Oncol. 2021;39(1):41–50. doi: 10.1002/hon.2820.
- Wang H, Hu WM, Xia ZJ, et al. High numbers of CD163+ tumor-associated macrophages correlate with poor prognosis in multiple myeloma patients receiving bortezomib-based regimens. J Cancer. 2019;10(14):3239–3245. doi: 10.7150/jca.30102.
- Varga G, Dávid Tóth A, Réka Szita V, et al. Beneficial effect of lenalidomide-dexamethason treatment in relapsed/refractory multiple myeloma patients: results of real-life data from 11 Hungarian centers. Pathol Oncol Res. 2021;27:613264. doi: 10.3389/pore.2021.613264.
- Oka S, Ono K, Nohgawa M. Clinical effects of CD33 and MPC-1 on the prognosis of multiple myeloma treated with bortezomib. Leuk Lymphoma. 2019;60(9):2152–2157. doi: 10.1080/10428194.2019.1574003.
- Matsumura-Kimoto Y, Kuroda J, Kaneko H, et al. Pomalidomide with or without dexamethasone for relapsed/refractory multiple myeloma in Japan: a retrospective analysis by the Kansai Myeloma Forum. Int J Hematol. 2018;107(5):541–550. doi: 10.1007/s12185-018-2416-4.
- Goel U, Charalampous C, Kapoor P, et al. Defining drug/drug class refractoriness vs lines of therapy in relapsed/refractory multiple myeloma. Blood Cancer J. 2023;13(1):11. doi: 10.1038/s41408-023-00785-y.
- Usmani SZ, Diels J, Ito T, et al. Daratumumab monotherapy compared with historical control data in heavily pretreated and highly refractory patients with multiple myeloma: an adjusted treatment comparison. Am J Hematol. 2017;92(8):E146–E152. doi: 10.1002/ajh.24781.
- Usnarska-Zubkiewicz L, Debski J, Butrym A, et al. Efficacy and safety of lenalidomide treatment in multiple myeloma (MM) patients—report of the Polish Myeloma Group. Leuk Res. 2016;40:90–99. doi: 10.1016/j.leukres.2015.11.005.
- Kumar SK, Dimopoulos MA, Kastritis E, et al. Natural history of relapsed myeloma, refractory to immunomodulatory drugs and proteasome inhibitors: a multicenter IMWG study. Leukemia. 2017;31(11):2443–2448. doi: 10.1038/leu.2017.138.
- Jagannath S, Lin Y, Goldschmidt H, et al. KarMMa-RW: comparison of idecabtagene vicleucel with real-world outcomes in relapsed and refractory multiple myeloma. Blood Cancer J. 2021;11(6):116. doi: 10.1038/s41408-021-00507-2.
- Dhanasiri S, Hollier-Hann G, Stothard C, et al. Treatment patterns and outcomes in triple-class exposed patients with relapsed and refractory multiple myeloma: findings from the multinational ITEMISE study. Clin Ther. 2021;43(11):1983–1996.e3. doi: 10.1016/j.clinthera.2021.09.013.
- Mohty M, Terpos E, Mateos MV, et al. Multiple myeloma treatment in real-world clinical practice: results of a prospective, multinational, noninterventional study. Clin Lymphoma Myeloma Leuk. 2018;18(10):e401–e419. doi: 10.1016/j.clml.2018.06.018.
- Rajkumar SV, Richardson P, San Miguel JF. Guidelines for determination of the number of prior lines of therapy in multiple myeloma. Blood [Internet]. 2015; Aug 13 [cited 2023 Sep 15];126(7):921–922. https://pubmed.ncbi.nlm.nih.gov/26272048/ doi: 10.1182/blood-2015-05-647636.