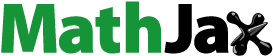
Abstract
Large-scale molecular datasets have generally outperformed morphological data for inferring phylogeny, and sources of error in the latter are poorly understood. The morphologically and ecologically diverse marsupial order Diprotodontia (kangaroos and their relatives, the koala, wombats and possums) is well suited to considering these issues. Recent molecular results provide a phylogenetic benchmark for comparing previous molecular and morphological studies, encompassing all of the major phylogenetic data sources and methods that have been employed over the past 50 years. We show here that most molecular methodologies and ‘informal-comparative’ morphological studies have inferred diprotodontian relationships that closely resemble the recent molecular consensus. However, and perhaps surprisingly, algorithmic morphology, such as maximum parsimony analysis of morphological matrices, has inferred markedly inaccurate phylogenies, and is not improved by re-analysis with more recently developed, model-based (e.g., likelihood and Bayesian) methods. This is particularly concerning because algorithmic morphology is the primary approach for integrating fossils into the tree of life, and hence, for both calibrating molecular timescales and extending phylogenetic inferences of evolutionary processes beyond the snapshot provided by modern species. A novel simulation study presented here suggests that the inaccuracies in the marsupial algorithmic morphology studies partly stem from functional and body-size correlations among taxa that over-ride phylogenetic signals. We use the results to trial a reverse engineered phylogeny approach to correcting for such functional and developmental correlations among morphological data. In addition, we interrogated a newly published, densely taxon-sampled morphological matrix. Deeper level phylogeny reconstruction was improved by including fossils alongside extant taxa and counterintuitively, by increased effort to resolve relationships among shallow taxa.
Matthew J. Phillips [[email protected]]; Mélina A. Celik [[email protected]] School of Biology and Environmental Science, Queensland University of Technology, 2 George Street, Brisbane, Qld, 4000, Australia; Robin M.D Beck [[email protected]] Ecosystems and Environment Research Centre, School of Science, Engineering and Environment, University of Salford, Manchester, UK.
THE TREE OF LIFE is rapidly being resolved, primarily due to advances in molecular phylogenetics. This has ignited intense debate on the future relevance of morphological phylogenetics (Scotland et al. Citation2003, Wiens Citation2004, Springer et al. Citation2007, Citation2013, Lee & Palci Citation2015). Although the composition of most members of mammalian orders, families and genera was robustly inferred from morphological data (see McKenna & Bell Citation1997), the recent dominance of molecular studies is highlighted by examples such as the resolution of relationships among the mammal orders, which morphological studies had failed to untangle (Springer et al. Citation2004, Duchéne et al. Citation2018). Ironically, such successes have also served as notice that the future of phylogenetics above the species level will increasingly be concerned with integrating extinct species into the tree of life, which in turn, can improve macroevolutionary inference (Slater et al. Citation2012, Marshall Citation2017). The success of this task depends on advances in extracting phylogenetic signal from morphological data.
Morphological phylogenetics is broadly divisible into ‘algorithmic morphology’, which uses data matrices comprising explicitly defined characters and character states that are then analysed using specific optimality criteria, such as maximum parsimony (MP) or maximum likelihood (ML), and ‘informal-comparative morphology’, which informally determines support for competing hypotheses based on subjective weighting of morphological evidence, often without the use of clearly defined characters or character states. Both approaches seek to tease phylogenetic signal apart from non-phylogenetic signals and from variation that is more or less stochastic. However, relative to each other, algorithmic morphology focuses more statistical rigour (Kitching et al. Citation1998, Wiens Citation2000), and informal-comparative morphology emphasizes untangling shared ancestry from functional and developmental influences (Szalay Citation2000).
The Australasian marsupial order Diprotodontia is ideal for examining the accuracy of alternative approaches for inferring relationships. The ∼150 extant species (Mammal Diversity Database v1.9.1) comprise arguably the most ecologically diverse terrestrial assemblage of any mammalian order (Aplin & Archer Citation1987). Moreover, publication from 2008 onwards of closely congruent inter-family relationships from multiple nuclear and mitochondrial genes (Beck Citation2008, Phillips & Pratt Citation2008, Meredith et al. Citation2009, Citation2011, Duchéne et al. Citation2018) and genomic retroposons or ‘jumping genes’ (Nilsson et al. Citation2010, Gallus et al. Citation2015) provide a benchmark for testing the relative phylogenetic performance of alternative data sources and analytical methods. The molecular consensus (then and now) strongly favours reciprocal monophyly of Vombatiformes (wombats and koalas) and Phalangerida (possums and macropods), which in turn includes three suborders/superfamilies, Petauroidea (Acrobatidae, (Tarsipedidae, (Petauridae, Pseudocheiridae)))), Macropodiformes or Macropodoidea (Hypsiprymnodontidae, (Potoroidae, Macropodidae))), and Phalangeroidea (Burramyidae, Phalangeridae) sensu Kirsch et al. (Citation1997).
The only remaining inter-family uncertainty for the extant members of Diprotodontia is the branching order of the three phalangeridan superfamilies. The 2008–2009 nuclear and mitochondrial studies cited above all favoured grouping Phalangeroidea and Macropodiformes. Phylogenomic analyses (Duchéne et al. Citation2018, Doronina et al. Citation2022) have tended to favour grouping Petauroidea and Macropodiformes, whereas genome-wide retroposons favoured grouping Phalangeroidea and Petauroidea (Doronina et al. Citation2022). There is also conflict between DNA sequence analyses and retroposons for placing the root of marsupials (e.g., Meredith et al. Citation2011, Gallus et al. Citation2015). For such short internal branches, it is possible that misspecified models are misleading phylogenetic analyses of DNA sequences, or that retroposon counts are biased by lineage sorting probabilities differing among incipiently speciating populations of different size and structure. Nevertheless, it is a remarkable achievement that recent molecular phylogenetic studies have collectively whittled down 654,729,075 possible rooted topologies among the 11 extant diprotodontian families to just three plausible alternative topologies.
The absence of agreement among molecular phylogenetic studies prior to 2008 on the relationships among diprotodontian families (except for a wombat-koala grouping) is also important; earlier morphological inferences of diprotodontian phylogeny are likely to be largely independent of molecular results. This contrasts with the Springer et al. (Citation2007) suggestion of molecular influence on morphological phylogenies of placental mammals. Over the past decade and a half there have been influential morphological studies of clades within Diprotodontia, such as Macropodiformes (e.g., Kear et al. Citation2007, Prideaux & Warburton Citation2010) and Vombatiformes (e.g., Gillespie et al. Citation2016, Beck et al. Citation2020). However, Diprotodontia-wide morphological studies over this timeframe have mostly been modest extensions of older matrices. For instance, Luo et al. (Citation2003) is an earlier iteration of the character set used by Wang et al. (Citation2021) and includes the same diprotodontian taxa. Conversely, the Beck et al. (Citation2022) craniodental morphological data matrix does deserve particular attention, because it employs many new characters and selects (and in many cases revises) earlier-published characters in light of whether discrete states can be consistently recognised across marsupials. Moreover, Beck et al. (Citation2022) include all extant diprotodontian genera and many of the most complete Oligocene–Pleistocene fossil taxa, which in turn allows us to explore the long-anticipated benefit of including fossil taxa for resolving deeper-level phylogeny (see Gauthier et al. Citation1988, Beck & Baillie Citation2018, Mongiardino et al. Citation2021).
Close phylogenetic agreement between analyses of retroposons and both nuclear and mitochondrial DNA sequences for the relationships among diprotodontian families provides a benchmark for testing the relative phylogenetic performance of alternative data sources and analytical methods. Here we consider current and legacy molecular inference methods, including DNA–DNA hybridization and micro complement fixation (MC’F), as well as informal-comparative and algorithmic morphology. Asher (Citation2018) compared historical and recent methods for resolving placental mammal relationships. Here we want to extend our analysis to better understand the sources of conflict from among morphological characters. Several frameworks have been developed to identify sets of characters that are saturated (noisy) or with correlated homoplasy (e.g., Dávalos et al. Citation2014, Celik & Phillips Citation2020). A number of different methods have also been used to attempt to minimize the negative impact of homoplasy on phylogenetic analysis of discrete morphological character matrices. These include implied weights parsimony (Goloboff et al. Citation2018, Asher et al. Citation2019), model-based analysis with homoplasy-based partitioning (Rosa et al. Citation2019), and identification and removal of morphological characters that strongly conflict with molecular data (Dávalos et al. Citation2014). Here, we propose an alternative approach to explore the contribution of ecological covariation to (apparent) morphological phylogenetic signal and, in turn, endeavour to correct for this covariation and improve the accuracy of phylogenetic inference.
Material and methods
The DNA sequence matrix
A matrix of 20,654 nucleotides (MtNuc20654) was compiled for 16 diprotodontians and seven outgroup marsupials. This consisted of mitochondrial genome protein and RNA coding sequences (14,704 nucleotides), and five nuclear genes (5950 nucleotides from ApoB, BRCA1, IRBP, RAG1 and vWF). The 23 taxa sampled and associated GenBank accessions are listed in Supplemental data file 1 (Table S1). Mitogenomes and the five nuclear loci were chosen for two reasons: (1) they reflect gene sampling availability at the time the molecular consensus on diprotodontian phylogeny initially crystallized and that we used as a cut-off for our initial survey of molecular and morphological phylogenies, and (2) all diprotodontian families are sampled for each locus, whereas even the best sampled genome-scale study (Duchéne et al. Citation2018) does not cover all diprotodontian families. The mitochondrial and nuclear sequences were each manually aligned in Se-Al 2.0a (Rambaut Citation1996) and then concatenated into a single data matrix.
We reconstructed diprotodontian phylogeny from MtNuc20654 using maximum likelihood (IQ-TREE 2.13: Nguyen et al. Citation2015) and Bayesian inference (MrBayes 3.2.7: Ronquist et al. Citation2012). The nuclear genes were concatenated, but with codon positions modelled separately, following Phillips et al. (Citation2022), resulting in three partitions. The mtDNA RNA-coding sites were partitioned into stem and loop positions for both transfer RNA and ribosomal RNA coding positions. The 12S and 16S rRNA structures are based on Gutell et al. (Citation1993) and Springer & Douzery (Citation1996) respectively, while the tRNA structures are based on models designed in Phillips et al. (Citation2001). These structures and the assignment of stem and loop positions have been progressively updated by one of us (MJP) as additional mitogenomes have become available. The updates involve a combination of manual base-paring/structural correspondence and stem-loop assignment, and automated RNA folding (minimum free energy with variable-length sequences, to help search for global optima) in RNAfold (Gruber et al. Citation2008). The mtDNA protein-coding sites were partitioned into codon positions for NADH2 and the other 12 genes together, following Phillips & Pratt (Citation2008). Thus, there are four RNA-coding and six protein-coding partitions. Models for each partition were selected by ModelFinder within IQ-TREE. Maximum likelihood (ML) analyses were performed with proportional branch lengths among partitions (-spp option). Ultrafast bootstrap approximation (2000 replicates) was employed for assessing confidence in the resulting topology.
For Bayesian inference in MrBayes, two independent analyses were each run with two Markov chain Monte Carlo (MCMC) chains for five million generations. The same nuclear and mitochondrial partitions were used as described above for ML. Models were unlinked across all partitions for the substitution matrix, state frequencies (empirical), invariant sites and the shape parameter of the rates-across-sites gamma distribution. This is consistent with the partitioning default in IQ-TREE. MrBayes differs from IQ-TREE by allowing branch lengths to be variously proportional and fully independent between partitions. Our MrBayes analyses followed Phillips et al. (Citation2013), with branch lengths fully unlinked between the nuclear and mtDNA data (which have different DNA polymerase proof-reading mechanisms). However, branch lengths were proportionally scaled across partitions within each of these genomes. Trees were sampled every 2500 generations, with the first 25% discarded as burn-in. Clade frequencies across the four independent runs reached convergence (clade frequency standard deviations <0.01) and estimated sample sizes for likelihood, prior and substitution parameter estimates were all above 200 (Tracer v.1.7.1: Rambaut et al. Citation2018).
Summary trees for alternative phylogenetic inference data sources and methods
We employed summary consensus trees to represent each of the major methodological efforts to infer relationships between the diprotodontian families. Only studies published before 2008 were included, so as to predate the molecular consensus. Family-level summary trees were available for MC’F (Baverstock et al. Citation1990) and DNA–DNA hybridization (Kirsch et al. Citation1997). Such singular summaries are not justifiable for other data sources/methods, for which multiple, largely independent research programs had interrogated marsupial phylogeny. To summarize multiple studies for each data source (e.g., mitochondrial genes), we inferred matrix representation with parsimony trees (MRP: Baum Citation1992) in PAUP* 4.0b10 (Swofford Citation2002). To select source trees for the MRP analyses, only the most definitive study from each research program was included (see below). This avoids selective inclusion of published trees and facilitates data/analysis/researcher non-independence as far as possible. For example, it would be inappropriate for the matrix representation parsimony summary for algorithmic morphological phylogeny to be swamped by the numerous cladistic analyses of Luo and colleagues, which are based on largely identical characters and character scores. Instead, only the most recent (prior to the molecular consensus) and taxonomically complete study by these researchers that was aimed at resolving marsupial relationships (Luo et al. Citation2003) was selected for inclusion in the MRP analysis alongside algorithmic morphology studies from other research groups.
When constructing the MRP summary trees, only individual studies that included members of at least six diprotodontian families were included as source trees. MRP summary trees for each of the methods cover all extant diprotodontian families, except that only one taxon, ‘Macropodidae’ (Macropodiformes/Macropodoidea), is employed to represent Macropodidae, Potoroidae and Hypsiprymnodontidae. This decision was made to ensure equivalence between summary trees (and their maximum likelihood scores) across different data sources/methods, among which some earlier studies had not distinguished these three taxa or had used a Potoroidae–Hypsiprymnodon chimaera. All summary trees and source tree references are provided in Supplemental data file 1, and cover nuclear genes, mitochondrial genes, albumin MC’F, DNA–DNA hybridization, informal-comparative morphology and algorithmic morphology.
The pre-molecular-consensus morphological matrix (morph352)
Minimally overlapping characters derived from four pre-2008 algorithmic morphology studies (see below) were combined to provide a 352-character morphological matrix (morph352) for diprotodontian marsupials. This covers 330 cranial, mandibular, dental and postcranial osteological characters, and 22 soft-tissue characters. Taxon sampling reflects availability among the source studies. Each of the nine diprotodontian families (kangaroos and allies = Macropodidae) are represented, with five additional marsupial genera included as outgroups (Dasyurus, Notoryctes, Perameles, Macrotis and Dromiciops).
Horovitz & Sánchez-Villagra (Citation2003) provides the most comprehensive coverage of morphological characters that are variable among marsupials, and their matrix is the foundation for morph352. Many of the 229 characters derived from this source are also covered by one or more of the other source matrices, allowing Acrobatidae and Tarsipedidae (which Horovitz & Sánchez-Villagra [Citation2003] did not include) to be represented in morph352. Additional characters not included by Horovitz & Sánchez-Villagra (Citation2003) are drawn from Springer et al. (Citation1997) (chs. 230–286), with minor updates from Sánchez-Villagra (Citation2001), and also from Luckett (Citation1994) (chs. 287–290) and Luo et al. (Citation2003) (chs. 291–352). The source derivation for the 352 characters (56 ordered, 296 unordered) included in morph352 is shown in Supplemental data file 1 (Table S2). Trees were inferred from morph352 under MP in PAUP, ML (Mk model) in Garli 0.97 (Zwickl Citation2006), and Bayesian inference (Mk + G model) in MrBayes 3.1.6 (Huelsenbeck & Ronquist Citation2001). These programs reflect the phylogenetic applications available in 2008–2009 when the molecular consensus on diprotodontian phylogeny crystallized (and when we set the cut-off for the inclusion of studies in the MRP trees). Morphological matrices for phylogenetic inference often only include variable characters (or indeed, parsimony informative characters). However, after the deletion of Mesozoic mammal taxa from the source data matrices, morph352 includes a substantial proportion (12%) of constant characters. Hence, we used the Lewis (Citation2001) Mk model, which does not assume that only variable characters were sampled, unlike his Mkv model, which assumes no variable characters.
Maximum likelihood advantage
Data partitions and maximum-likelihood (ML) model categories were specified as described above (for MtNuc20654) for the first, second and third protein-coding positions and the RNA-coding stem and loop sites (separately for rRNA and tRNA). Likelihood modelling is described further in Supplemental data file 1. Partitioned ML analysis of the molecular matrix (MtNuc20654) in PAUP allowed likelihood disadvantage (the difference in likelihood, ΔlnL) relative to the molecular consensus (, −lnL = 121,316.34) to be calculated for summary trees derived from each of the alternative data types and analytical methods, including for the trees inferred from our morph352 data matrix. Where two topologies are equally favoured for a data source/analytical method, the likelihood disadvantage (ΔlnL) is given as the average of the two scores.
Figure 1. Family-level phylogeny of diprotodontian marsupials. A, The favoured molecular tree in phylogenetic analyses of MtNuc20654. The consensus of recent molecular studies collapses the branch indicated by the asterisk. Maximum likelihood bootstrap support is shown at nodes when less than 100%. All nodes received 100% Bayesian posterior probability. B, Algorithmic morphology tree favoured under MP and ML analyses of morph352. C, Tree inferred under the optimal mixture (see ) of molecular phylogenetic (sim352) and ecological (size/diet) signals. Ancestral diet was reconstructed under ordered MP (herbivorous; dark green/dark tone, plant-dominated omnivorous; light green/light tone, broadly omnivorous; orange/intermediate tone, nectivorous; dashed). Circles indicate average adult mass (open; 0–50 g, 1 closed; 50–200 g, 2 closed; 200–800 g, 3 closed; 800 g–3 kg, 4 closed; 3–12 kg, 5 closed; >12 kg). Half circles indicate variation between species (e.g., 3, 4 for Phalangeridae). Diet and size states are assigned according to the attributes of the species included in the studies from which the morph352 dataset is derived.

Calculating the likelihood disadvantage (on MtNuc20654) of alternative trees relative to the recent molecular consensus provides a direct and quantitative comparison between the various molecular and morphological phylogeny approaches. The inverse strategy of determining the fit of the recent molecular consensus to each data source/method is not comparable between distance (e.g., MC’F) and cladistic (e.g., algorithmic morphology) selection criteria and is not objectively quantifiable for informal morphology.
The simulated morphological phylogenetic signal dataset (sim352)
Simulated ‘morphological’ data were evolved in Seq-Gen 1.3.2 (Rambaut & Grassly Citation1997). The resulting Sim352 dataset provides the expected phylogenetic signal based on the study that cemented the molecular consensus (Meredith et al. Citation2009). More specifically, we take Meredith et al.’s (Citation2009) multi-gene molecular chronogram, which covers all Australasian marsupial families, and we scaled the branch lengths to sum to the per-character tree-length estimated for the morphological dataset (morph352). The molecular chronogram was trimmed to the same 14 taxa employed in morph352. The tree-length of the chronogram is 671.7 million years. The tree-length of the morph352 data on the same (molecular consensus) topology and under the Mk model in Garli 0.97 (Zwickl Citation2006) is 4.3866. Hence, the scaling factor applied to the chronogram in order to provide phylogenetic signal consistent with morphological tree-length is 4.3866/671.7 = 0.0065306, giving the phylogenetic tree on which 60,000 characters were evolved in Seq-gen 1.3.2 (Rambaut & Grassly Citation1997) under an F81 model (Supplemental data file 2). The F81 model is equivalent to the ‘morphological’ Mk4 model in which the condition of producing variable characters is relaxed. Both this relaxation and the 4-state maximum are properties of the original morphological data (morph352).
Beck et al. (2022) craniodental data: phylogenetic performance with fossils included or excluded
The Beck et al. (Citation2022) morphological data matrix includes 180 craniodental characters that are scored across 144 marsupials, including 47 fossil taxa; of these characters, 30 representing plausible morphoclines were specified as ordered by Beck et al. (Citation2022), and this was maintained here. The matrix has comparatively high completeness (see Beck et al. Citation2022, table 4), but to further reduce missing data, we excluded the four fossil taxa with <50% completeness, namely the fossil vombatid diprotodontian Warendja wakefieldi and three fossil paucituberculatans from South America (Evolestes spp., Pichipilus spp. and Stilotherium dissimile). Bayesian inference was implemented in MrBayes 3.2.7. All 180 characters are variable, with 17 (=9.4%) autapomorphic, and hence the Mkv model, which assumes that only variable (both parsimony informative and autapomophic) characters are scored (Lewis, Citation2001), was specified. Following Beck et al. (Citation2022), a single 8-category rates-across-sites lognormal distribution was specified. We note that only a gamma rates-across-sites distribution was available for Mk models when the (molecular) consensus on diprotodontian phylogeny arose, and hence, for comparison with those earlier studies, we employed the Mkv + G model with the morph352 dataset. Four independent runs each included three MCMC chains for 15,000,000 generations, with the first 25% discarded as burn-in. Each chain was sampled every 5000th generation. Clade frequencies across the four independent runs reached convergence (clade frequency standard deviations <0.01) and estimated sample sizes for likelihood, prior, tree length and substitution parameter estimates were all above 200 (Tracer v.1.7.1: Rambaut et al. Citation2018). The analysis was repeated with a backbone scaffold (Supplemental data file 3) in which monophyly was enforced for modern members of each family (as confirmed by independent molecular data, e.g., Mitchell et al. Citation2014). The macropod family unit employed as a backbone constraint is in fact Macropodiformes (=Macropodoidea), consistent with our usage for the summary trees (see that section above, for the rationale).
Maximum parsimony analyses of the Bmorph180 dataset were implemented in TNT v.1.5. For each analysis, non-parsimony informative characters were ignored using the ‘XINACT’ command. For analyses with the molecular scaffold, the scaffold topology was enforced as a backbone constraint using the ‘force’ command. All parsimony analyses used the same two-stage search strategy, comprising a ‘New Technology’ search (using Sectorial Search, Ratchet, Drift, and Tree Fusing with default parameters) that was run until the same minimum tree length was hit 100 times, followed by a ‘Traditional’ search with tree bisection reconnection (TBR) branch swapping among the trees saved from the first stage, run until a maximum of 100,000 trees were saved. Multiple most parsimonious trees were summarized using strict (=Nelsen) consensus.
Results
Comparing the phylogenetic performance of alternative data sources and methods
The combined mitochondrial-nuclear (MtNuc20654) diprotodontian phylogeny conforms to the recent molecular consensus (). The only uncertainty in that consensus is a basal trichotomy among Phalangerida, for which our ML tree groups macropods and phalangeroids (with 75% bootstrap support) to the exclusion of petauroids. Bayesian inference recovered each clade with 100% posterior probability. We compared this topology with alternatives derived from each of the major phylogenetic data sources employed over the past 50 years. Comparing alternative trees in terms of shared groupings/subtrees ignores the reality that some conflicting clades are more strongly supported than others. As such, we use likelihood disadvantage to compare alternative trees. This is the difference in log likelihood (ΔlnL) on MtNuc20654 between the maximum-likelihood (ML) tree () and trees favoured in the alternative studies.
Among molecular data sources, the best result (least likelihood disadvantage) was 81.3 ΔlnL for the summary tree from mitochondrial studies, and the worst, 182.4 ΔlnL for albumin micro-complement fixation (). Informal-comparative morphology compares well with these approaches (71.7 ΔlnL); indeed, this is a better result than we obtained for individual nuclear loci and for mitogenome phylogenies prior to 2008. In contrast, the algorithmic morphology summary tree fits MtNuc20654 very poorly (690.1 ΔlnL). Lumping together the 352 non-overlapping characters from the four algorithmic morphology studies (morph352) resulted in an MP tree that provided only minor improvement (594.6 ΔlnL). ML and Bayesian inference reanalyses of morph352 using the ‘morphological’ Mk model (Lewis Citation2001) respectively inferred identical and worse trees relative to MP ().
Table 1. Likelihood disadvantage of diprotodontian phylogenies from alternative data sources.
The extreme likelihood disadvantages of the algorithmic morphology trees result from support for clades that are strongly contradicted by the molecular data. This could be explained in part if strong molecular signals are associated with relatively long shared clade histories during which little morphological change occurred. However, the success of the informal morphological analyses belies this explanation. Furthermore, a simulated morphological dataset was constructed by evolving 60,000 characters on a molecular-dated diprotodontian tree (Meredith et al. Citation2009) consistent with , but with per-site tree-length scaled to the ML tree-length inferred in Garli (Zwickl Citation2006) for the morphological data (morph352). This provides an arbitrarily long dataset from which to bootstrap largely independent sub-samples of 352 characters. MP bootstrapping (1000 replicates, resampling 352 characters) for this ‘sim352’ dataset suggests that morph352 sampled sufficient characters to resolve the basic structure of the tree, given the temporal pattern of branching. All groupings () receive 73–100% bootstrap support, except for two, which receive 53–61% support. Hence, the morph352 results in cannot easily be explained by stochastic error alone. We considered two potential solutions for improving the accuracy of algorithmic phylogeny for these marsupials: correcting for functional/developmental correlations, and including fossils to help tease apart homoplasy and synapomorphy.
Diet and body-size correlations among characters mislead phylogeny
Several informal-comparative morphological studies of marsupials (e.g., Aplin & Archer Citation1987, Szalay Citation1994) emphasize the importance of untangling phylogenetic signals from correlations with size (allometric scaling) and diet, which for brevity we refer to collectively as ecological characters. Comparison with the molecular consensus () further suggests influence from functional-developmental correlations on the algorithmic morphology tree (). Ecologies are widely distributed across the molecular tree, with large size (>4 circles) and stricter herbivory (dark green lineages) respectively evolving at least two and three times independently. Algorithmic morphology instead lumps all of the larger and more herbivorous taxa together, relative to the smaller, omnivorous (and nectivorous) taxa.
Simulations were employed to explore the influence of ecological correlations on phylogenetic signal among diprotodontian families. The sim352 data (see above) provided phylogenetic signal, which was combined with ecological characters, coded as ordered states for diet (animalivorous, generalized omnivorous, plant-specialized omnivorous, herbivorous) and body-size (<50 g, 50–200 g, 200–800 g, 800 g–3 kg, 3–12 kg, >12 kg). As expected, the simulated ‘phylogenetic’ characters alone favour the molecular tree (, 0% ecological contribution). Other trees were preferred as the two ecological characters were included and scaled (multiplied out) to fixed contributions, with corresponding numbers of the 60,000 sim352 phylogenetic characters excluded. Once the ecological contribution is increased to 2% of characters, the algorithmic morphology tree () is favoured over the molecular tree ().
Figure 2. Maximum parsimony treelength disadvantage. Percentage additional treelength required for the diprotodontian molecular consensus (open circles) and for the algorithmic morphology tree (closed circles), relative to the treelength of the MP topology, for each mixture of phylogenetic (sim352) and ecological (size/diet) characters. Optimal fit to the algorithmic morphology tree occurs with the ecological characters multiplied out to contribute 2.9% of the data. Fit to the molecular consensus tree continues to deteriorate (25.1% treelength disadvantage at 16% ecological contribution).

As shown in , the optimum fit to the algorithmic morphology tree occurs with a 97.1% to 2.9% mix of phylogenetic to ecological characters – the latter contributing disproportionately to overall signal (7.8% of MP tree-length). The favoured tree under this mixture () is identical to the morph352 tree (), besides local swaps among the small, arboreal possums at the base of Diprotodontia. It is remarkable that a tree so similar to the algorithmic morphology tree (phylogenetic randomization test, p = 0.00063; see Supplemental data file 2) is reconstructed simply from the interaction of molecular phylogenetic signal and correlations with two ecological characters.
Reverse engineered phylogeny
Success in modelling phylogenetic and ecological contributions to morph352 () points to a new direction for morphological phylogenetics, which we introduce as ‘reverse engineered phylogeny’ (REP). The aim is to correct phylogenetic inference for the influence of non-phylogenetic signals; in the present case, these are from allometric and diet-related covariation. Algebraically then, if the signal in the morphological phylogeny is phylogenetic signal plus ecological signal, the ‘true’ phylogeny may be recovered as the morphological signal minus the ecological signal.
(1)
(1)
Given EquationEquation 1(1)
(1) , reverse engineered phylogeny follows a five step process.
Infer a phylogeny from the morphological data matrix (morph352 in this case).
Simulate pseudomorphological ‘phylogenetic’ characters on a molecular chronogram, scaled to morphological tree-length. This provides characters with the rate characteristics of the morphological data, but with the underlying ‘true’ phylogenetic signal (sim352 in this case).
Compile an ecological character matrix (body mass and diet characters in this case).
Infer the proportional contributions of ‘phylogenetic’ and ‘ecological’ signals to the morphological dataset (the optimum fit to the morphological phylogeny; see ).
Apply EquationEquation 1
(1)
(1) to alternative hypotheses with the intention to correct the signal in the morphological data (morph352) for the inferred contribution of ecological signal (see 4).
As shown in , MP on the morphological data (morph352) favoured the algorithmic morphology tree (723 steps) over the molecular tree (773 steps). The size and diet characters require 26 steps (13 steps per character) for the molecular tree and 14 steps (seven steps per character) for the morphological tree. To apply EquationEquation 1(1)
(1) and correct for non-phylogenetic signals, we note that the optimization in allows us to infer that the size and diet signal contribution to morph352 is equivalent to 2.9% of the matrix (), or 10.2 characters. For the morphological tree, subtracting the ecological contribution (10.2 × 7) from the morph352 MP score (723) gives a REP score of 651.6. For the favoured molecular tree, the morph352 MP score is greater (773), but so too is the ecological contribution (10.2 × 13), such that applying this correction results in morph352 instead favouring our molecular tree () by 11.2 steps, with an REP score of 640.4. However, another topology consistent with the molecular consensus (which includes a trichotomy at the base of Phalangerida) has an even better REP score (630.4). This REP tree (see ) supports possum monophyly, in contrast to our molecular tree (from MTNuc20654), in which macropods are sister to the phalangeroid possums, to the exclusion of the petauroid possums.
Table 2. Reverse engineered phylogeny (REP) scores for alternative diprotodontian phylogeny hypotheses, (a) the favoured tree on the morphological character matrix, morph352 (), (b) the favoured tree on the molecular matrix, MtNuc20654 (Fig. 1A), and the two other topologies that are consistent with the molecular consensus, which includes a basal trichotomy among phalangeroids, (c) grouping phalangeroids + petauroids (possum monophyly) and (d) grouping macropods and petauroids. The REP score is the morph352 MP score minus the size and diet signal correction (size & diet MP score) × 10.2, where 10.2 is the optimal character contribution to apparent phylogenetic signal (2.9% of the 352 characters; see ).
Including fossils alongside extant marsupials for inferring phylogeny from craniodental data
The Beck et al. (Citation2022) craniodental morphology data matrix (Bmorph180) is more densely sampled right across the diversity of modern marsupials than previous morphological matrices and includes 43 modern diprotodontian taxa, and as used here, another 23 fossil diprotodontian taxa (after exclusion of the fossil vombatid, Warendja, due to its character incompleteness; see Methods). We inferred phylogeny using both methods initially employed in Beck et al. (Citation2022), maximum parsimony (MP) and Bayesian inference (BI). We provide the MP and BI trees in Supplemental data file 3.
Before including the fossil taxa, the Bmorph180 trees have even greater likelihood disadvantage (BI: 774.4 ΔlnL, MP 1042.5 ΔlnL) relative to the favoured molecular tree on molecular data (MtNuc20654) than did the best morph352 tree (594.6 ΔlnL). However, this is not a fair comparison, because we assumed or forced family-level monophyly for the morph352 tree and for other studies included in , for comparability with those studies that only include a single representative of each family. With family-level monophyly similarly enforced, likelihood advantage for Bmorph180 improves (BI; 540.2 ΔlnL, MP; 573.2 ΔlnL). This also improves slightly upon morph352, but the differences from the molecular tree are similar to the apparently functionally/developmentally based relationships reconstructed with morph352 (see ). This includes the small, omnivorous/nectivorous Tarsipes and Burramyidae being drawn to the base of Diprotodontia, and recovery of a clade that groups the macropods and Vombatiformes (BI), both of which include many larger, specialized herbivores. Vombatiformes and macropods were drawn together relative to their consensus placements with MP, but with Phalangeridae as sister to the macropods.
Figure 3. Comparison of A, the morph352 tree and C, the MtNuc20654 molecular tree, respectively, with the diprotodontian phylogenies reconstructed from Bayesian inference of the Bmorph180 dataset B, without including fossil taxa and D, with fossil taxa included. Node support values on the Bmorph180 trees are Bayesian posterior probabilities.

Upon restoring the fossil taxa, the trees inferred from Bmorph180 show dramatically increased congruence with the molecular tree (BI; 51.7 ΔlnL, MP; 255.4 ΔlnL), albeit with family-level monophyly enforced among the modern taxa. These trees recover the accepted basal split among diprotodontians, which is between Vombatiformes and Phalangerida. Indeed, for the Bayesian inference tree, the only interfamily differences from the molecular consensus are the grouping of Tarsipes with acrobatids (a clade that was proposed by Aplin and Archer (Citation1987), and who named it Tarsipedoidea), although this clade is still within a monophyletic Petauroidea, and burramyids being placed at the base of Phalangerida (). Some of the molecular consensus clades that are recovered with Bmorph180 (upon the inclusion of fossils) have relatively strong statistical support (e.g., Phalangerida, 0.93 Bayesian posterior probability, BPP), while others are only weakly supported (e.g., Petauroidea, 0.45 BPP). Other algorithmic morphology studies have not recovered these clades without the addition of molecular data in ‘total evidence’ analyses (e.g., Beck et al. Citation2022). Thus, our results lend encouragement for more confidently resolving relationships among many branches of the tree of life for which molecular data is not accessible.
Discussion
Diprotodontian phylogeny
‘Traditional morphological phylogeny is often performed by anatomists in ignorance of statistics and without clear repeatability. But it concerns me more that cladistics is often performed by geologists who treat fossils as rocks, in ignorance of evolution.’ ̶ ̶ Tim Hamley, 1999.
On morphological phylogenetics: ‘The results are often characters described in an uninterpretive framework of anatomy, akin to static, process-purged, descriptions of land forms.’ ̶ ̶ Frederick Szalay (Citation1993).
Molecular phylogenetics using genome-scale sequencing (e.g., Duchéne et al. Citation2018) and retroposons (e.g., Doronina et al. Citation2022) provides a robust and stable family-level phylogeny for Diprotodontia, with the only uncertainty being the local arrangement of macropods and the two possum clades, Petauroidea and Phalangeroidea. Phylogenetic analysis of our combined nuclear and mitochondrial dataset (MtNuc20654) reflects both the overall diprotodontian molecular consensus and the uncertainty of that single contentious relationship. Using this molecular data to gauge the performance (likelihood disadvantage) of trees derived from alternative data sources and methods found that informal-comparative morphology (e.g., Aplin & Archer Citation1987, Marshall et al. Citation1990, Szalay Citation1994) performed as well (), if not better, than legacy molecular methods, such as DNA–DNA hybridization and single gene sequences. In contrast, algorithmic morphology based on character matrices for modern taxa performed poorly (). These results bring to mind Tim Hamley’s partly facetious concern (see above quote in conversation with MJP) that apparent statistical rigour and scientific repeatability count for little if hitched to methods that are ignorant of evolutionary processes.
The contention that cladistics ignores evolutionary processes is unfair, especially over recent years. Many advocates of algorithmic morphology (Jenner Citation2004; Wiens Citation2004) argue for more thorough character analysis; for example, including morphometric approaches or developmental studies to promote scoring objectivity. Moreover, only the informal-comparative morphology studies had the benefit of fossils for tracing character evolution (). Below, we will see the benefit of including fossils with algorithmic morphology. Nevertheless, evolutionary non-independence is rife among mammalian traits (Kangas et al. Citation2004, Goswami et al. Citation2009, Billet & Bardin Citation2019), allowing ecological similarity to masquerade as phylogenetic signal. Hence, it is likely to be important that the informal-comparative studies are fundamentally attempts to discriminate between functional-developmental covariance and phylogenetic signal. None of the algorithmic morphology studies for marsupials provided explicit criteria for considering such covariance either in character inclusion, state delimitation, or evolutionary modelling, although such methods are being proposed (e.g., Dávalos et al. Citation2014).
If algorithmic morphology can examine functional/ecological influences on apparent phylogenetic signal, as has been done with informal-comparative studies, algorithmic morphology will add valuable statistical rigour to this process. In this regard, our simulations for inferring the contribution of body size and diet correlations to apparent phylogenetic signal in morph352 (see ) are encouraging in their simplicity, and closely model the original morphological signal (cf. ). Future studies might also consider other ecological traits with which numerous morphological characters covary (e.g., foraging height and locomotion, Szalay & Sargis Citation2001). Given our success in using size and diet correlates to characterize phylogenetic biases, we introduced a novel (reverse engineered phylogeny) correction for functional/developmental correlations.
Reverse engineered phylogeny
Previous studies have identified suits of homoplastic morphological characters that are associated with convergent ecological strategies and that contribute to erroneous clades being reconstructed, such as for burrowing squamates (Lee Citation1998) and nectar feeding phylostomid bats (Dávalos et al. Citation2012, Citation2014). Simply excluding these characters introduces circularity and removes any phylogenetic signal they contribute to inferring relationships among other taxa. We propose a method that does not exclude characters. Reverse engineered phylogeny (REP) is so named because it employs information on relationships from independent molecular data, to infer and correct for ecological signal contributions (not pre-specified characters) to morphological matrices. By varying the contribution of the size and diet characters alongside the simulated phylogenetic characters (), we found the best fit for the algorithmic morphology tree () to be 97.1% molecular ‘phylogenetic’ signal and 2.9% ‘ecological’ (size and diet) signal. Correcting for this inferred contribution of ecological covariation to the morphological data overturned the morphological tree in favour of the recent molecular consensus, providing a simple proof of concept for reverse engineered phylogeny. More specifically, REP favoured possum monophyly in agreement with the genomic retroposon tree of Doronina et al. (Citation2022), over the macropod–phalangeroid grouping reconstructed in our MtNuc20654 tree ().
Whereas molecular data has largely resolved relationships among modern taxa, reverse engineered phylogeny may be of most value for inferring the affinities of fossil taxa for which functional morphology is broadly similar to modern counterparts, such that covariation between ecological and morphological states is similar across the tree. Inferred corrections for ecological correlations among the modern taxa can then be applied to the phylogenetic placement of fossils. To infer the proportional contribution of ‘phylogenetic’ and ‘ecological’ signals to the morphological dataset, steps 1–4 from Methods, ‘reverse engineered phylogeny’ would be applied to the taxa for which molecular data are available (as for the present study). The ecological traits would be scored for the fossil taxa (incorporating any uncertainty) and employed in step 5, to correct the phylogenetic placement of the fossils for ecological covariation. It is particularly notable that the leading hypotheses for Mesozoic mammal relationships derive from some of the same matrices (e.g., Luo et al. Citation2003, Horovitz & Sánchez-Villagra 2003) for which we successfully applied REP to correct diprotodontian phylogeny. As such, good candidates for REP might include some key fossils for understanding the origins of modern mammal clades and for calibrating molecular timescales.
Searching tree space efficiently for REP with large Mesozoic mammal data matrices or indeed, for the Bmorph180 marsupial matrix employed here, will require a simple modification to phylogenetic inference software—an option for scores from individual data partitions (e.g., ecological) to be subtracted instead of added. Furthermore, size and diet are given equal weight as ecological correlates in the present study. Future studies could optimize relative weightings for the individual ecological correlates simultaneously with the inference of the overall ecological contribution to apparent phylogenetic signal (see ). Another refinement for this inference of the ecological contribution would be to randomly assign the uncertainty state (?) to a proportion of the simulated phylogenetic (e.g., sim352) and ecological characters for taxa that are missing data in the original morphological data matrix.
Geometric morphometrics (MacLeod & Forey Citation2002) may directly facilitate the goal of reverse engineered phylogeny—to exclude non-phylogenetic signals. These shape-based methods are increasingly being considered for phylogenetic inference (e.g., Parins-Fukuchi Citation2018, Ascarrunz et al. Citation2019), because the subjectivity of character choice and state assignments are obviated, although landmarking is still required in most instances. A nice property of geometric morphometrics is that correlates of size (or allometry) can be readily excluded, regressing shape against centroid size, as Celik et al. (Citation2019) did for inferring macropod phylogeny. In principle, regressions against shape could also be used to remove covariation with ecological or other life history traits, with the expectation that the residuals contain higher fidelity phylogenetic signals. In practice, however, geometric morphometric phylogeny has often struggled with low signal to noise, and limited implementation of explicit evolutionary models within ML or Bayesian frameworks (Klingenberg & Gidaszewski Citation2010, Parins-Fukuchi Citation2018). Overcoming these challenges and others that are more prominent for fossils, such as fragmentary/deformed samples, would greatly advance the value of geometric morphometrics for inferring phylogeny.
Integrating fossils into diprotodontian phylogeny
It is well established that merging fossils into the tree of life can improve phylogenetic inference from morphological data for relationships among modern taxa (Gauthier et al. Citation1988, Beck et al. Citation2022). Adding fossils can better discriminate homoplasy and synapomorphy, both by breaking up long branches in the phylogeny and by sampling temporally closer to nodes (Mongiardino & Parry Citation2020#x00029;. With our likelihood disadvantage analyses we find that agreement between the Bmorph180 phylogeny and the molecular tree improved moderately from 774.4 ΔlnL to 642.2 ΔlnL when including the fossils, but then dramatically improved by using a backbone scaffold to force family-level monophyly for the modern taxa (51.7 ΔlnL). This latter improvement includes recovering the clades Petauroidea and ‘core-Petauroidea’ (Petauridae and Pseudocheiridae) as well as shifting the affinities of Vombatiformes (previously sister to macropods) to being sister to all other diprotodontians ().
Our finding that accurately recovering relationships at the targeted phylogenetic depths is assisted by correctly recovering shallower relationships is not novel (Lin et al. Citation2002) but appears to be underappreciated, and should encourage inclusion in matrices of morphological variation that is phylogenetically informative below focal depths. Indeed, ‘hidden phylogenetic support’ (Gatesy et al. Citation1999, Lee & Camens Citation2009) and phylogenetic accuracy gains from total evidence studies (e.g., O’Leary & Gatesy Citation2008, Asher & Smith Citation2022) may derive in part from employing molecular data that also informs shallow relationships, and which in turn, allow morphological apomorphies to be more accurately traced across the tree. Another factor is that the combination of fossils and correct shallow relationships allow more accurate modelling of evolutionary rate variation among characters. This may help explain why the ΔlnL improvement upon including fossils (Supplemental data file 3, Table S3) was smaller with maximum parsimony but more substantial with Bayesian inference, which models evolution and distributes characters into different evolutionary rate bins. Improved congruence with molecular phylogeny is also encouraging for inferring the relationships of fossil taxa. A notable example is that with the family-level scaffold in the Bayesian trees, the marsupial lions (Thylacoleonidae) shift from outside crown Diprotodontia to be a sister to other Vombatifomes, with admittedly weak support (0.44 BPP). Again, this result is closer to informal-comparative morphology studies, which typically place thylacoleonids within Vombatiformes, often close to Vombatidae (e.g., Aplin & Archer Citation1987, Marshall et al. Citation1990).
Conclusions
Molecular phylogenetics has been highly successful in resolving the relationships of modern organisms. However, the power of molecular data for inferring evolutionary patterns, processes and character histories diminishes looking further back in time, as modern lineages coalesce, and recent trends obscure earlier evolutionary history (Rabosky & Alfaro Citation2010). In addition to combining morphological and molecular data, the solution is to accurately integrate fossils into the tree of life. The summary tree from the informal-comparative morphology studies shows remarkably good agreement with diprotodontian molecular phylogeny (), but the source trees lack statistical rigour and are not easily repeatable. The present study provides a simple proof of concept for a new direction for combining biological realism and scientific rigour – reverse engineered phylogeny (REP), which uses molecular phylogeny and ecological/life-history characters to characterize and correct for functional/developmental correlations across characters. Moreover, we show that the dense sampling of fossil and modern taxa by Beck et al. (Citation2022) also provides good agreement with molecular phylogeny, particularly when family-level monophyly is enforced (as the intended total-evidence treatment would provide); this confirms predictions that improvements in our knowledge of fossil diversity – which in the case of Diprotodontia is evidently far from adequate (Archer et al. Citation2018, Beck et al., Citation2018) – will result in increased phylogenetic accuracy of algorithmic analyses of morphological data. Simple modifications of tree-search algorithms to implement REP, in combination with improved morphological matrices that have denser sampling of modern and (in particular) fossil taxa, should lead to more accurate merging of fossils into the tree of life.
TALC-2022-0053-File009.pdf
Download PDF (163 KB)TALC-2022-0053-File008.pdf
Download PDF (137.7 KB)TALC-2022-0053-File007.pdf
Download PDF (302.8 KB)Acknowledgements
We thank Michael Lee and David Penny for valuable discussions respectively, on morphological phylogenetics and historical innovations in molecular phylogenetics. Comments from Robert Asher and one anonymous reviewer improved the manuscript. Funding was provided to MJP by The Australian Research Council (DP220103234).
Disclosure statement
No potential conflict of interest was reported by the author(s).
Supplementary material
Supplementary research materials for this article can be accessed online at https://doi.org/10.1080/03115518.2023.2184492
References
- Aplin, K.P. & Archer, M., 1987. Recent advances in marsupial systematics with a new syncretic classification. In Possums and Opossums: Studies in Evolution. Archer, M., ed., Surrey Beatty & Sons, Sydney, xv–lxxi.
- Archer, M., Binfield, P., Hand, S.J., Black, K.H., Creaser, P., Myers, T.J., Gillespie, A.K., Arena, D.A., Scanlon, J., Pledge, N. & Thurmer, J., 2018. Miminipossum notioplanetes, a Miocene forest-dwelling phalangeridan (Marsupialia; Diprotodontia) from northern and central Australia. Palaeontologia Electronica 21, 1–11.
- Ascarrunz, E., Claude, J. & Joyce, W.G., 2019. Estimating the phylogeny of geoemydid turtles (Cryptodira) from landmark data: an assessment of different methods. PeerJ 7, e7476.
- Asher, R., 2018. Taxonomy, trees, and truth in historical mammalogy. In Mammalian Evolution, Diversity and Systematics. Zachos, F.E. & Asher, R.J., eds, DE Gruyter, Berlin, Germany.
- Asher, R.J. & Smith, M.R., 2022. Phylogenetic signal and bias in palaeontology. Systematic Biology 71, 986–1008.
- Asher, R.J., Smith, M.R., Rankin, A. & Emry, R.J., 2019. Congruence, fossils and the evolutionary tree of rodents and lagomorphs. Royal Society Open Science 6, 190387.
- Baum, B.R., 1992. Combining trees as a way of combining datasets for phylogenetic inference and the desirability of combining gene trees. Taxon 41, 3–10.
- Baverstock, P.R., Krieg, M. & Birrell, J., 1990. Evolutionary relationships of Australian marsupials as assessed by albumin immunology. Australian Journal of Zoology 37, 273–287.
- Beck, R., 2008. A dated phylogeny of marsupials using a molecular supermatrix and multiple fossil constraints. Journal of Mammalogy 89, 175–189.
- Beck, R.M.D. & Baillie, C., 2018. Improvements in the fossil record may largely resolve current conflicts between morphological and molecular estimates of mammal phylogeny. Proceedings of the Royal Society B: Biological Sciences 285, 20181632.
- Beck, R.M.D., Louys, J., Brewer, P., Archer, M., Black, K.H. & Tedford, R.H., 2020. A new family of diprotodontian marsupials from the latest Oligocene of Australia and the evolution of wombats, koalas, and their relatives (Vombatiformes). Scientific Reports 10, 9741.
- Beck, R.M., Voss, R.S. & Jansa, S.A., 2022. Craniodental morphology and phylogeny of marsupials. Bulletin of the American Museum of Natural History 457, 1–352.
- Billet, G. & Bardin, J., 2019. Serial homology and correlated characters in morphological phylogenetics: Modeling the evolution of dental crests in placentals. Systematic Biology 68, 267–280.
- Celik, M., Cascini, M., Haouchar, D., VAN Ded Burg, C., Dodt, W., Evans, A.R., Prentis, P., Bunce, M., Fruciano, C. & Phillips, M.J., 2019. A molecular and morphometric assessment of the systematics of the Macropus complex clarifies the tempo and mode of kangaroo evolution. Zoological Journal of the Linnean Society 186, 793–812.
- Celik, M.A. & Phillips, M.J., 2020. Conflict resolution for Mesozoic mammals: reconciling phylogenetic incongruence among anatomical regions. Frontiers in Genetics 11, 0651.
- Dávalos, L.M., Cirranello, A.L., Geisler, J.H. & Simmons, N.B., 2012. Understanding phylogenetic incongruence: lessons from phyllostomid bats. Biological Reviews 87, 991–1024.
- Dávalos, L.M., Velazco, P.M., Warsi, O.M., Smits, P.D. & Simmons, N.B., 2014. Integrating incomplete fossils by isolating conflicting signal in saturated and non-independent morphological characters. Systematic Biology 63, 582–600.
- Doronina, L., Feigin, C.Y. & Schmitz, J., 2022. Reunion of Australasian possums by shared SINE insertions. Systematic Biology 71, 1045–1053.
- Duchéne, D.A., Bragg, J.G., Duchéne, S., Neaves, L.E., Potter, S., Moritz, C., Johnson, R.N., Ho, S.Y. & Eldridge, M.D., 2018. Analysis of phylogenomic tree space resolves relationships among marsupial families. Systematic Biology 67, 400–412.
- Gallus, S., Janke, A., Kumar, V. & Nilsson, M.A., 2015. Disentangling the relationship of the Australian marsupial orders using retrotransposon and evolutionary network analyses. Genome Biology and Evolution 7, 985–992.
- Gatesy, J., O’Grady, P. & Baker, R.H., 1999. Corroboration among data sets in simultaneous analysis: hidden support for phylogenetic relationships among higher level artiodactyl taxa. Cladistics 15, 271–313.
- Gauthier, J., Kluge, A.G. & Rowe, T., 1988. Amniote phylogeny and the importance of fossils. Cladistics 4, 105–209.
- Gillespie, A.K., Archer, M. & Hand, S.J., 2016. A tiny new marsupial lion (Marsupialia, Thylacoleonidae) from the early Miocene of Australia. Palaeontologia Electronica 19, 1–25.
- Goloboff, P.A., Torres, A. & Arias, J.S., 2018. Weighted parsimony outperforms other methods of phylogenetic inference under models appropriate for morphology. Cladistics 34, 407–437.
- Goswami, A., Weisbecker, V. & Sanchez-Villagra, M.R., 2009. Developmental Modularity and the Marsupial-Placental Dichotomy. Journal of Experimental Zoology. Part B, Molecular and Developmental Evolution 312B, 186–195.
- Gruber, A.R., Lorenz, R., Bernhart, S.H., Neuböck, R. & Hofacker, I.L., 2008. The Vienna RNA websuite. Nucleic Acids Research 36, W70–W74.
- Gutell, R., Grey, M. & Schnare, M., 1993. A compilation of large subunit (23S and 23S-like) ribosomal RNA structures. Nucleic Acids Research 21, 3055–3074.
- Horovitz, I. & Sánchez-Villagra, M.R., 2003. A morphological analysis of marsupial mammal higher-level phylogenetic relationships. Cladistics 19, 181–212.
- Huelsenbeck, J.P. & Ronquist, F., 2001. MrBayes: Bayesian inference of phylogenetic trees. Bioinformatics 17, 754–755.
- Jenner, R.A., 2004. Accepting Partnership by Submission? Morphological Phylogenetics in a Molecular Millennium. Systematic Biology 53, 333–342.
- Kangas, A.T., Evans, A.R., Thesleff, I. & Jernvall, J., 2004. Nonindependence of mammalian dental characters. Nature 432, 211–214.
- Kear, B.P., Cooke, B.N., Archer, M. & Flannery, T.F., 2007. Implications of a new species of the Oligo-Miocene kangaroo (Marsupialia: Macropodoidea) Nambaroo, from the Riversleigh World Heratige Area, Queensland, Australia. Journal of Paleontolgy 81, 1147–1167.
- Kirsch, J.A.W., Lapointe, F.J. & Springer, M.S., 1997. DNA-hybridisation studies of marsupials and their implications for metatherian classification. Australian Journal of Zoology 45, 211–280.
- Kitching, I.J., Forey, P.L., Humphries, C.J. & Williams, D., 1998. Cladistics: The Theory and Practice of Parsimony Analysis. Oxford University Press, Oxford; New York.
- Klingenberg, C.P. & Gidaszewski, N.A., 2010. Testing and quantifying phylogenetic signals and homoplasy in morphometric data. Systematic Biology 59, 245–261.
- Lee, M.S.Y., 1998. Convergent evolution and character correlation in burrowing reptiles: towards a resolution of squamate relationships. Biological Journal of the Linnean Society 65, 369–453.
- Lee, M.S.Y. & Camens, A.B., 2009. Strong morphological support for the molecular evolutionary tree of placental mammals. Journal Evolutionary Biology 22, 2243–2257.
- Lee, M.S. & Palci, A., 2015. Morphological phylogenetics in the genomic age. Current Biology 25, R922–R929.
- Lewis, P.O., 2001. A likelihood approach to estimating phylogeny from discrete morphological character data. Systematic Biology 50, 913–925.
- Lin, Y.H., Mclenachan, P.H., Gore, A.R., Phillips, M.J., Ota, R., Hendy, M. & Penny, D., 2002. Four new mitochondrial genomes, and the increased stability of evolutionary trees of mammals from improved taxon sampling. Molecular Biology Evolution 19, 2060–2070.
- Luckett, W.P., 1994. Suprafamilial relationships within Marsupialia: resolution and discordance from multidisciplinary data. Journal of Mammalian Evolution 2, 255–288.
- Luo, Z.X., Ji, Q., Wible, J.R. & Yuan, C.X., 2003. An early Cretaceous tribosphenic mammal and metatherian evolution. Science 302, 1934–1940.
- Macleod, N. & Forey, P.L., 2002. Morphology, Shape and Phylogeny. CRC Press, London.
- Mammal Diversity Database, 2022. Mammal Diversity Database. v1.9.1. Zenodo, Arizona State University, Tempe, AZ, USA.
- Marshall, L.G., Case, J.A. & Woodburne, M.O., 1990. Phylogenetic relationships of the families of marsupials. In Current Mammalogy Volume 2, Genoways, H.H., ed., Plenum Press, New York, 433–502.
- Marshall, C.R., 2017. Five palaeobiological laws needed to understand the evolution of the living biota. Nature Ecology & Evolution 1, 0165.
- Mckenna, M.C. & Bell, S.K., 1997. Classification of Mammals above the Species Level. Columbia University Press, New York.
- Meredith, R.W., Janecka, J.E., Gatesy, J., Ryder, O.A., Fisher, C.A., Teeling, E.C., Goodbla, A., Eizirik, E., Simao, T.L., Stadler, T. & Rabosky, D.L., 2011. Impacts of the Cretaceous Terrestrial Revolution and KPg extinction on mammal diversification. Science 334, 521–524.
- Meredith, R.W., Westerman, M. & Springer, M.S., 2009. A phylogeny of Diprotodontia (Marsupialia) based on sequences for five nuclear genes. Molecular Phylogenetics and Evolution 51, 554–571.
- Mitchell, K.J., Pratt, R.C., Watson, L.N., Gibb, G.C., Llamas, B., Kasper, M., Edson, J., Hopwood, B., Male, D., Armstrong, K.N., Meyer, M., Hofreiter, M., Austin, J., Donnellan, S.C., Lee, M.S.Y., Phillips, M.J. & Cooper, A., 2014. Molecular phylogeny, biogeography, and habitat preference evolution of marsupials. Molecular Biology and Evolution 31, 2322–2330.
- Mongiardino Koch, N., Garwood, R.J. & Parry, L.A., 2021. Fossils improve phylogenetic analyses of morphological characters. Proceedings of the Royal Society B: Biological Sciences 288, 20210044.
- Mongiardino Koch, N. & Parry, L.A., 2020. Death is on our side: Paleontological data drastically modify phylogenetic hypotheses. Systematic Biology 69, 1052–1067.
- Nguyen, L.-T., Schmidt, H.A., Von Haeseler, A. & Minh, B.Q., 2015. IQ-TREE: A fast and effective stochastic algorithm for estimating maximum-likelihood phylogenies. Molecular Biology and Evolution 32, 268–274.
- Nilsson, M.A., Churakov, G., Sommer, M., VAN Tran, N., Brosius, J. & Schmitz, J., 2010. Tracking marsupial evolution using archaic genomic retroposon insertions. PLoS Biology 8, e1000436.
- O’Leary, M.A. & Gatesy, J., 2008. Impact of increased character sampling on the phylogeny of Cetartiodactyla (Mammalia): combined analysis including fossils. Cladistics 24, 397–442.
- Parins-Fukuchi, C., 2018. Use of continuous traits can improve morphological phylogenetics. Systematic Biology 67, 328–339.
- Phillips, M.J., Haouchar, D., Pratt, R.C., Gibb, G.C. & Bunce, M., 2013. Inferring kangaroo phylogeny from incongruent nuclear and mitochondrial genes. PLoS One 8, e57745.
- Phillips, M.J., Lin, Y.-H., Harrison, G.L. & Penny, D., 2001. Mitochondrial genomes of a bandicoot and a Brushtail possum confirm the monophyly of Australiadelphian marsupials. Proceedings of the Royal Society B 268, 1533–1538.
- Phillips, M.J. & Pratt, R.C., 2008. Family-level relationships among the Australasian marsupial "herbivores" (Diprotodontia: Koala, wombats, kangaroos and possums). Molecular Phylogenetics and Evolution 46, 594–605.
- Phillips, M.J., Cascini, M. & Celik, M., 2022. Identifying complex DNA contamination in pig-footed bandicoots helps to clarify an anomalous ecological transition. Diversity 14, 352.
- Prideaux, G.J. & Warburton, N.M., 2010. An osteology-based appraisal of the phylogeny and evolution of kangaroos and wallabies (Macropodidae: Marsupialia). Zoological Journal of the Linnean Society 159, 954–987.
- Rabosky, D.L. & Alfaro, M.E., 2010. Evolutionary bangs and whimpers: methodological advances and conceptual frameworks. Systematic Biology 59, 615–618.
- Rambaut, A. & Grassly, N.C., 1997. Seq-Gen: an application for the Monte Carlo simulation of DNA sequence evolution along phylogenetic trees. Bioinformatics 13, 235–238.
- Rambaut, A., 1996. Se-Al: Sequence Alignment Editor (online). http://evolve.zoo.ox.ac.uk/.
- Rambaut, A., Drummond, A.J., Xie, D., Baele, G. & Suchard, M.A., 2018. Posterior summarisation in Bayesian phylogenetics using Tracer 1.7. Systematic Biology 67, 901–904.
- Ronquist, F., Teslenko, M., VAN DER Mark, P., Ayres, D.L., Darling, A., Hohna, S., Larget, B., Liu, L., Suchard, M.A. & Huelsenbeck, J.P., 2012. MrBayes 3.2: Efficient Bayesian phylogenetic inference and model choice across a large model space. Systematic Biology 61, 539–542.
- Rosa, B.B., Melo, G.A.R. & Barbeitos, M.S., 2019. Homoplasy-based partitioning outperforms alternatives in Bayesian analysis of discrete morphological data. Systematic Biology 68, 657–671.
- Sánchez-Villagra, M.R., 2001. The phylogenetic relationships of argyrolagid marsupials. Zoological Journal of the Linnean Society 131, 481–496.
- Scotland, R.W., Olmstead, R.G. & Benett, J.R., 2003. Phylogeny reconstruction: The role of morphology. Systematic Biology 52, 539–548.
- Slater, G.J., Harmon, L.J. & Alfaro, M.E., 2012. Integrating fossils with molecular phylogenies improves inference of trait evolution. Evolution; International Journal of Organic Evolution 66, 3931–3944.
- Springer, M.S., Burk-Herrick, A., Meredith, R., Eizirik, E., Teeling, E., O'Brien, S.J. & Murphy, W.J., 2007. The adequacy of morphology for reconstructing the early history of placental mammals. Systematic Biology 56, 673–684.
- Springer, M.S. & Douzery, E., 1996. Secondary structure and patterns of evolution among mammalian 12S rRNA molecules. Journal of Molecular Evolution 43, 357–373.
- Springer, M.S., Kirsch, J.A.W. & Case, J.A., 1997. The chronicle of marsupial evolution. In Molecular Evolution and Adaptive Radiations. Givnish, T. & Systma, K., eds, Cambridge University Press, Cambridge, 129–157.
- Springer, M.S., Stanhope, M.J., Madsen, O. & DE Jong, W.W., 2004. Molecules consolidate the placental mammal tree. Trends in Ecology & Evolution 19, 430–438.
- Springer, M.S., Meredith, R.W., Teeling, E.C. & Murphy, W.J., 2013. Technical comment on “The placental mammal ancestor and the post-K-Pg radiation of placentals.” Science 341, 613.
- Swofford, D.L., 2002. PAUP*: Phylogenetic Analysis Using Parsimony (*and Other Methods). Version 4.0b10. Sinauer Associates, Sunderland.
- Szalay, F.S., 1993. Metatherian taxon phylogeny: evidence and interpretation from the cranioskeletal system. In Mammal Phylogeny: Mesozoic Differentiation, Multituberculates, Monotremes, Early Therians, and Marsupials. Szalay, F.S., Novacek, M.J. & Mckenna, M.C., eds, Springer-Verlag, New York, 216–242.
- Szalay, F.S., 1994. Evolutionary History of the Marsupials and an Analysis of Osteological Characters. Cambridge University Press, Cambridge.
- Szalay, F.S., 2000. Function and adaptation in paleontology and phylogenetics: why do we omit Darwin? Palaeontologia Electronica 3, 1–25.
- Szalay, F.S. & Sargis, E.J., 2001. Model-based analysis of postcranial osteology of marsupials from the Palaeocene of Itaborai (Brazil) and the phylogenetics and biogeography of Metatheria. Geodiversitas 23, 139–302.
- Wang, J., Wible, J.R., Guo, B., Shelley, S.L., Hu, H. & Bi, S., 2021. A monotreme-like auditory apparatus in a Middle Jurassic haramiyidan. Nature 590, 279–283.
- Wiens, J.J., 2004. The role of morphological data in phylogeny reconstruction. Systematic Biology 53, 653–661.
- Wiens, J.J., 2000. Phylogenetic Analysis of Morphological Data. Smithsonian Institution Press, Washington, DC.
- Zwickl, D.J., 2006. Genetic Algorithm Approaches for the Phylogenetic Analysis of Large Biological Sequence Datasets Under the Maximum-likelihood Criterion. PhD thesis, University of Texas at Austin.