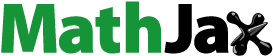
ABSTRACT
This paper evaluates the effects of the government's COVID-19 economic stimulus and relief package (emergency/one-off income grant of ND750) on household food security in Namibia during the period of the lockdown. The analysis reveals that a household that received the income grant ($42 equivalent) experienced about 11%−17% reduction in food insecurity compared to their non-recipient counterparts. We also found that the effect was relatively higher in female-headed households than in male-headed households. The positive effect is supported by a higher proportion (53%) of the beneficiary households who were satisfied with the policy. These findings underscore the need for the government of Namibia to institutionalise and sustain the income grant policy as a safety net and extend it to cover other vulnerable households in the post-pandemic. Such a programme should be gender-responsive and targeted at household heads who make decision over food consumption and other household arrangements for a bigger impact.
JEL CLASSIFICATION:
1. Introduction
In the heat of the COVID-19 outbreak, governments around the world imposed restrictions (lockdowns) on human movement in order to contain the spread of the disease. This policy measure affected households’ sources of livelihood and other socioeconomic welfare. The extent of the impact of the restrictions on households, firms and the economy has been extensively documented (Arndt et al., Citation2020; Breisinger et al., Citation2020; Abdeen et al., Citation2021; Almeida et al., Citation2021). For instance, evidence in Kenya and Uganda suggests that more than two-thirds of households experienced income shocks and worsened food security due to the pandemic. The impact was particularly higher among the income poor and households that depended on labour income (Kansiime et al., Citation2021). Evidence from a Social Accounting Matrix (SAM) Multiplier analysis of the distancing measures in South Africa revealed that although government transfer payment helped to significantly insulate the total incomes of low-income households, the restriction imposed large reductions in wage income particularly for low-skilled workers (Arndt et al., Citation2020).
As part of efforts to ease the socioeconomic burdens associated with such restrictions, governments introduced different economic policy measures including stimulus and relief packages for both households and firms. In Africa, some of the interventions included but were not limited to the provision of free electricity, waiver/suspension of bill payments, free distribution of meals, and VAT exemptions on electricity bills (Akrofi & Antwi, Citation2020). While there has been extensive literature on how the disease impacted households, firms and the economy and the various measures taken by governments (Arndt et al., Citation2020; Breisinger et al., Citation2020; Abadie & Cattaneo, Citation2018; Abdeen et al., Citation2021), the effectiveness of those interventions in alleviating the effect of the disease on households’ welfare in developing countries remains under-researched. This paper contributes to knowledge on this policy issue by assessing the effect of one of the government’s COVID-19 economic stimulus and relief packages on households in Namibia.
Namibia is one of the countries in Africa which was worse hit by the pandemic. As of 13 January 2022, the situation reports from the government revealed that 154 664 cases (6% of 2 550 226 population) had been recorded. The country reported its first two confirmed cases of the virus on 13th March 2020. By December 2020, the number of confirmed cases had exceeded 18000 with more than 170 deaths (Shangula, Citation2020). Five days after the first confirmed cases, the government declared a State of Emergency (SOE) on 17th March 2020 and subsequently announced a country-wide lockdown 10 days later. The lockdownFootnote1 which was implemented in five stages from March to December 2020 necessitated the closing of businesses and services, except essential services such as banks and medical services (Amesho et al., Citation2020).
This policy measure affected virtually every facet of the Namibian economy. Available statistics from the Bank of Namibia revealed that the economy contracted by 8.0% in 2020 before recovering with positive GDP growth rates of 2.7% in 2021 and 3.9% in 2022 (Bank of Namibia, Citation2022). Prior to the pandemic, about 447,000 Namibians were already living under the international poverty line of US$1.90 per day (United Nations, Citation2020). UNECA estimates showed that the COVID-19 pandemic was expected to contribute to a surge in these poverty levels. The best-case scenario of a 3.4 percentage point drop in GDP growth would increase poverty from 17.2% to 19.5%. Namibia’s Human Development Index was also revised downward from 0.645 to 0.417, with education, health, and income contributing 25%, 22% and 53.6% to the loss, respectively (United Nations, Citation2020).
The daily economic activities of households and their food security were equally affected. The impact was particularly dire for low-income households whose main sources of livelihood were their participation in the labour market (Devereux et al., Citation2020). There were instances of retrenchment and layoffs by employers due to disruption in production and supply chain activities. It was estimated that unemployment would increase between 0.75 (best-case) and 1.4 (worst-case) percentage points, bringing it up from 33.4% to 34.2% and 34.5%, respectively.
As part of efforts to mitigate some of the undesirable impacts of the COVID-related restrictions on households and firms, the government rolled out an Economic Stimulus and Relief Package to support these two economic agents. The key interventions to support businesses towards job retention continued productive economic activities and their cash flow were wage subsidies for hardest hit sectors, accelerated repayment of overdue and undisputed VAT refunds, accelerated payment of overdue and undisputed invoices for goods and services provided to the government, non-agricultural small business loan scheme, agricultural business loan scheme, tax-back loan scheme for non-mining corporates, and relaxation of labour regulations to protect jobs. The government also granted policy relief to borrowers by the Development Bank of Namibia (DBN) and AgriBank in the form of a capital repayment moratorium with provisions for a holiday on the principal amount for a period between 6 and 24 months based on assessment, recapitalisation of interest, lengthening of the repayment periods and waiving of penalty provisions (Ministry of Finance, Citation2020).
The interventions for households were meant to support them to cope with reduced income, increased health-related spending and other hardships. The packages were water subsidies, a tax-back loan scheme for tax-registered and tax-paying (PAYE) employees and self-employed persons who lost income or part thereof or experienced difficulties. The main package which is the focus of this study is a once-off N$750 Emergency Income Grant intended to support those employees who had lost their jobs in either the formal or informal sectors due to the pandemic and its associated restrictions on human movement (Ministry of Finance, Citation2020). The grant (amounting to N$ 562 million in total) aimed to benefit Namibians between the ages of 18–60 years who lost their jobs. It, however, excluded students, those who experienced wages-cut in the formal sector and those who were recipients of government social grants (Deloitte, Citation2020).
While some studies have assessed the socioeconomic effect of Covid-19 in Namibia and other developing countries (Evelina et al., Citation2020; Kaisara & Bwalya, Citation2020; Lendelvo et al., Citation2020; Tirivangani et al., Citation2021), there is limited literature on the effectiveness of the various government interventions on household’s welfare and other living conditions. A review of the literature revealed that only Bahta & Musara (Citation2022) have attempted to quantify the impact of the COVID-19 Relief Vouchers Schemes on food security in South Africa. In the Namibian context, there is no study on how the government’s Emergency Income Grant impacted the livelihood of the beneficiary households.
We fill this gap in the literature by assessing the effect of the government’s Emergency Income Grant policy on the food security condition of households in the country.Footnote2 The overarching objective of the paper is to assess the extent to which this policy could be used as a safety net for poor and vulnerable households in the post-pandemic as part of the government’s overall poverty alleviation strategy. This study focuses on the grant because of the rationale for its disbursement, and its direct implication for households’ food consumption. Earlier studies suggest that the impact of COVID on households’ food security has gender implications (Bukari et al., Citation2022) There is, therefore, the need to understand how gender interacts with the implemented policy measures to ensure a gender-sensitive response that promotes women’s ability to safely perform crucial roles in the food value chain (Doss et al., Citation2020). Chiwona-Karltun et al. (Citation2021) advocate for a feminist economics approach to predict the likely impacts of the regulatory relief responses during the recovery process and post-COVID-19. Given these pieces of evidence, we explore the potential gender element of the impact of the income grant policy by disaggregating the analysis based on the gender of the household head.
We further argue that the extent to which households were affected by the lockdown and the effectiveness of the income grant policy in improving their living condition was largely dependent on their socioeconomic conditions before the pandemic. We, therefore, explore how households’ consumption expenditure per capita within 6 months preceding the pandemic, their sources of income for food, and other characteristic of the household head influenced their satisfaction with the income grant policy during the lockdown. This study is timely in the sense that Namibia is one of the countries in Africa with a high rate of food insecurity. Recent estimates by IPC (Citation2021) revealed that approximately 659000 people (26% of the population) who faced high levels of acute food insecurity (IPC Phase 3 or above) required urgent humanitarian assistance. A further breakdown of the distribution showed that 557000 people (22% of the analysed population) were classified to be in Crisis (IPC Phase 3), 102000 (4%) of them were in Emergency (IPC Phase 4) while 896000 people (35%) of them were found to be Stressed (IPC Phase 2).
In the subsequent section, we discuss the methodology used in addressing the objective of the paper. This is followed by a presentation and discussion of the results of the empirical analysis while the final section concludes the paper with some policy recommendations.
2. Materials and methods
The analysis and the interpretation of the results of this paper are based on cross-sectional data collected from households during the period of the lockdown in Namibia. The survey targeted household heads or household members who were responsible for making decisions about food consumption. The rationale for this decision was to ensure that adequate and reliable information was obtained from respondents who were both direct beneficiaries of the interventions and had an influence on decision-making in the household. It also targeted respondents who received only the income grant to avoid any potential confounding effect of external sources of income or assistance. We used some screening questions (screeners) to determine the respondents’ selection to participate in the study.
The survey design followed a mixed model research approach which involved a combination of both qualitative and quantitative methods of data collection. This allowed us to use a questionnaire composed of multiple closed-ended or quantitative-type items as well as several open-ended or qualitative-type items (Neuman, Citation2014; Creswell, Citation2015). We used the total number (10368) of households included in the 2015/2016 Namibia Household Income and Expenditure Survey (NHIES) as our sample frame because that was the most current survey at the time of our field exercise. Following earlier studies such as Charan & Biswas (Citation2013) and Daniel & Cross (Citation2018), the formula that we used to determine the sample size can be expressed as equations 1 and 2:
(1)
(1)
(2)
(2) In equation 2,
is the critical value of the normal distribution at
(for the confidence level of 95%, α is 0.05 for a two-tailed test or 0.025 for a one-tailed test and the critical value is 1.96). MOE is the margin of error (5% or 0.05 for this study),
is the sample proportion (50% or 0.5), N is the total number of households that were included in the NHIES, and n is the final sample used for the analysis.
Based on this formula, the initial strategy was to sample 374 (3.6%) of the 10368 households that participated in the 2015/2016 NHIES. As presented in , we arrived at this sample size by taking into consideration the potential saturation (due to homogeneity) of the responses that the households were likely to provide to our questions on food security. We also considered the regional distribution of the number of active cases of COVID-19 at the time of the survey. However, the implementation of the COVID-19 lockdown and the associated social distancing made it difficult to achieve this objective as envisaged.
Table 1. Confirmed COVID-19 cases (as of 26 August 2020) and sampled households.
In all, we were able to contact 271 (72.5%) of the expected sample of 374 households due to challenges relating to the availability of respondents and the need to observe the protocols of the ongoing social distancing policy. However, 259 of the 271 respondents completed the questionnaires with 9 incomplete responses which were dropped from the analysis. This led to a final sample of 250 households, representing 96.5% of the questionnaires completed together by both the respondents and the three interviewers or 92.3% of the total questionnaires (271) administered. This sample also represents 66.9% of the estimated sample of 374 households. Although we could not achieve the target sample size, the potential homogeneity of the responses elicited provides enough basis to consider our findings valid and reliable.
The data collection exercise took place within two weeks (11-25 May 2020). Due to the social distancing policy in place at the time of the field exercise, respondents of the identified households were contacted either on the telephone or in-person to complete the questionnaire. Unique identification numbers were generated for the respondents using their physical addresses together with their telephone numbers during the data cleaning process to identify any potential duplicates. We used SurveyMonkey (an electronic data collection application) which enabled us to populate our questionnaire in an online template. This facilitated the generation and management(cleaning) of the data. We used Stata software (version 16) to analyse the data gathered. The initial stage of the analysis involved a cross-tabulation of relevant variables and their frequencies to explore the response rate and identify any incomplete information on the variables of interest and the characteristics of the respondents.
2.1. Computation of the food security indicators
The food security indicators were computed from 6 variables which are presented in . This short form of the household food security scale has the potential to maximise the probability of correct classification of households concerning their financially based food insecurity and hunger. It is estimated that compared to the 18-item household food security, the short form classifies 97.7% of households correctly and underestimates the prevalence of overall food insecurity and hunger by only 0.3 percentage points. Although it is brief, the short form scale is a potentially useful tool for national and state/local surveys (Blumberg et al., Citation1999).
Table 2. Test of internal reliability of the food security indices.
The 6 variables were based on six questions that household heads were asked during the field exercise: (1) During the lockdown, did any adult (18 years and above) in your household go hungry because there was not enough food?; (2) During the lockdown did any child (17 years or younger) in your household go hungry because there was not enough food?; (3) During the lockdown did your household run out of money to buy food?; (4) Did you or your household cut the size of meals during the lockdown because there was not enough food in the house?; (5) Did you or any member of the household skip any meals during the lockdown because there was not enough food in the house?; (6) Did you or any member of the household eat a smaller variety of foods during the lockdown than you or that person would have liked to because there was not enough food in the house?
We computed 2 indices-an additive indexes and Principal Component Analysis (PCA) index. The additive index ranges between 0 and 1 where 0 indicates that the household was food secure. Following earlier studies (Asmah & Orkoh, Citation2017; Orkoh & Efobi, Citation2023), this index is multiplied by 100 for ease of interpretation. The second index is based on the Principal Component (PCA) approach to assess the reliability and robustness of the estimates. This index also ranges between −1.137 (more food secure) and 0.875+ (less food secure).
We use Cronbach's alpha to assess the internal consistency of the indices. The alpha value shows how closely related the items (food security variables) are and it is considered as a measure of scale reliability (Tavakol & Dennick, Citation2011). The recommended minimum alpha coefficient is between 0.65 and 0.80 (or higher) while a value less than 0.50 is usually considered unacceptable. In our case, we find an alpha value of 0.879 (approximately 88%) and an interitem correlation coefficient of 0.55. These parameters indicate that our indices are reliable, and the variables used to compute the indices are internally consistent and well correlated. Thus, all the variables in this test measure the same concept or construct. The calculation and use of alpha value to assess the reliability and internal consistency of a set of items is common in various fields including medical and education research (Tavakol & Dennick, Citation2011).
2.2. Empirical model specification
This study combines the matching estimator approach with inverse probability weighting method in assessing the effect of the income grant on household food security. Ideally, an assessment of this nature requires a pure Randomized Control Trial (RCT) which is mostly designed to test a hypothesis under optimal setting in the absence of confounding factors (Saturni et al., Citation2014). However, the same objective can be achieved using the quasi-experimental approach for observational data from surveys where perfect randomised assignment is impossible. The quasi-experimental approach involves the identification of a comparison group that is as similar as possible to the treatment group in terms of baseline (pre-intervention) characteristics (White & Sabarwal, Citation2008). The comparison group captures what would have been the outcomes if the programme or policy had not been implemented and this is what is known as the counterfactual. The difference in outcome between the treatment and control groups can be attributed to the intervention.
The estimation process requires the use of a treatment effect model where a dummy variable indicating the treatment condition (1 if respondent/household head received the income grant and 0 otherwise) is directly entered into the regression equation. The outcome variables (index of list of food security indicators) of the regression equation are observed for both observations (0, 1) of the dummy (policy) variable. In this analysis, we use the matching estimators to identify assisted household heads who had similar observable characteristics (such as age, education, and gender) as those who were not assisted. Following Abadie & Cattaneo (Citation2018), we used the propensity score matching (PSM), matching on covariate (near neighbour matching) method.Footnote3 The first step in the computation of the propensity score (PSM) involves the estimation of the predicted probability that a household head would be selected for assistance. We begin the estimation of the propensity scores [P()], with a logistic regression of the probability that a household would receive the income grant (1 if the household head received the income grant and 0 otherwise) on the observable covariates. The equation for the logistic regression can be specified as:
(3)
(3) We apply the selected algorithms to obtain robust matching estimates. The statistical significance of the average treatment effects on the quantities treated was tested using bootstrapped standard errors, which accounts for the variation caused by the matching process. The vector
captures covariates such as gender, age, level of education, employment, and marital status of the household head. It also contains the households’ source of income, expenditure per capita in the last 6 months before the lockdown and the regional location of the household.
The choice of the covariates was informed by two main conditions as discussed in the literature (Heckman et al., Citation1997; Becker & Ichino, Citation2002; Rosenbaum, Citation2002; Caliendo & Kopeinig, Citation2008). The first condition is that only variables that influence simultaneously the treatment status (receipt of the income grant) and the outcome variables (food security index) must be included in the model. The second requirement is that the variables included in the model should not be confounded with the outcome variable. The outcome variable must be independent of treatment conditional on the propensity score. In other words, a variable should only be excluded from the analysis if it is either unrelated to the outcome variable or not a proper covariate. The variables included in the analysis for the estimation of the propensity scores were selected based on these two conditions as recommended by Rubin & Thomas (Citation1996).
We estimate the average treatment effect by specifying food security as a function of the treatment (receipt of the income grant) in EquationEquation 4(4)
(4) .
(4)
(4)
(5)
(5)
(6)
(6)
The subscript represents the household head,
is the average treatment effect while
is the binary variable which takes the value 1 if the household head received the income grant, and 0 otherwise. The variable
represents the outcome variable (food security index). The propensity score,
captures the probability that a household would receive the income grant (X). To validate the consistency of the results, we use three matching techniques, namely, nearest neighbour, kernel, and radius matching. The subscript
represents a household,
denotes receipt of the income grant while
represents the food security indices (additive index and Principal Component Analysis index).
is a binary variable which takes the 1 if a household head received the income grant, and 0 otherwise.
The second objective of this study is to assess the socioeconomic determinants of households’ satisfaction with the income grant policy with particular attention to the households’ food expenditure per capita in the last 6 months preceding the lockdown. This objective is addressed using the logistic estimation technique. Household satisfaction is specified as functions of the individual and household characteristics.
(7)
(7) The variable
represents the probability that a household head
would be satisfied with the policy. Satisfaction with the policy is measured as a binary variable which takes the value 1 if the household head indicated that he/he was satisfied with the income grant policy and 0, otherwise. The variables included in this analysis are the food expenditure of the household during 6 months before the lockdown, and the households’ source of income for food. The other explanatory variables are the gender, age, education, employment status and marital status of the household head. provides a detailed description of each of the variables included in the models.
Table 3. Summary statistics of the main variables included in the analysis.
2.2.1. Results and discussion
As indicated in the introduction, the first objective of this paper is to analyse the effect of the emergency income grant on households’ food security while the second objective is to access households’ satisfaction with the policy with particular attention to their average expenditure per capita for food within the 6 months preceding the lockdown and the source of their income for food. This section presents a brief description of how households’ consumption expenditure before the pandemic and other characteristics of the household head influenced how they might differ in their views of the extent to which the lockdown affected them. As presented in , only 15% of the sampled households indicated that they were not affected by the lockdown.
Table 4. Extent of the effect the lockdown by selected household characteristics.
Considering the combined responses from moderately affected to a great deal, it appears that relatively more male-headed (69.8%) households than female-headed (61.9%) households were affected by the lockdown. This observation can be interpreted from the perspective of households’ income and expenditure patterns. Evidence shows that women spend a greater proportion of their income on food consumption although they earn less compared to male (Orkoh, Citation2018). This means that any shocks to households’ income for food should ideally affect female-headed households more than their male counterparts. It is therefore surprising that in this analysis, male-headed households were more affected compared to their female-headed counterparts.
The effect was higher among household heads who had low levels of education and those who were unemployed. The effect was also higher in households that relied on other sources of transfers such as government, NGOs, and churches, and those that relied on remittances from other family members compared to those that relied on monthly earnings from their economic activities for food. Figure A1 in the appendix shows that the lockdown policy affected a higher proportion of households that had low levels of food expenditure per capita before the COVID outbreak compared to those whose expenditures were already high. These results show the extent to which the pandemic affected economically vulnerable households and the importance of interventions such as the income grant in helping to alleviate the food and nutrition-related challenges that those households face.
Across the 14 regions of the country, the lockdown affected sources of income for food of a higher proportion of households in Omusati, Omaheke, Erongo, Ohangwena and Kavango East compared to households in Oshana, Oshikoto Otjozondjupa and Zambezi. The extent to which the lockdown affected a particular household was dependent on the socioeconomic characteristics of the region in which it was located. Households located in regions where there were higher numbers of COVID cases and those in regions where poverty was higher were the hardest hit. For instance, Kavango East was one of the regions that had the lowest number of active COVID cases and deaths (see ) as of the time of the survey. However, it has the highest monetary poverty rate (41.5%) and the second highest non-monetary poverty rate (70.0%). Similarly, Omaheke has the third highest rate of monetary poverty (34.2%) and the fifth highest rate (51.4%) of non-monetary poverty. Although the monetary poverty rate is relatively lower in Ohangwena (14.6%) it has the fourth higher rate (56.6%) of non-monetary poverty (Namibian Statistical Agency, Citation2021). Furthermore, Erongo has the least monetary (3.7%) and non-monetary (16.6%) rates of poverty, but it had the highest number of active COVID cases (3,373) and deaths (27 people) as of the time of the survey.
Considering the distribution of households’ receipt of the income grant, the analysis of the data shows that household heads who benefited constituted 39% of the sample. In the proportion of female-headed households (44%) that benefitted from the policy was higher than the proportion of male-headed households (33%). These distributions do not necessarily imply that there are more female household heads than male household heads in Namibia because the 2013 Demographic and Health Survey 2013 estimate the share of female-headed households to be 44% (Legal Assistance Center, Citation2017). It, however, reflects the priorities of the government in implementing the policy by focusing on vulnerable households. Receipt of the income grant was also higher among household heads who had low levels of education, were unemployed, and those who relied on remittances from family members and other sources of income such as government, NGOs, and churches.
Table 5. Households’ receipt of and satisfaction with the income grant by their characteristics.
Concerning the regional location of households, receipt of the grant was higher among household heads in Kavango West, Kavango East, Khomas, Omusati, Omaheke, Oshikoto, Ohangwena, and Oshana but very low among those in Otjozondjupa, Hardap, Kunene and Erongo. Receipt of the grant was determined by a combination of factors such as the regional distribution of households’ main sources of income for food, type of employment, poverty levels and the rate of COVID-19 cases and deaths at the time of the disbursement of the grant. For instance, 2015/2016 Namibia Household Income and Expenditure Survey Report revealed that Kavango East and Omaheke were among the poorest regions in the country having poverty levels higher than the national average of 10.7%. Similarly, Kavango East (42%), Kavango West (57%), Omaheke (46%), Ohangwena (56%) and Omusati (63.5%) are among the regions in which households spent a higher proportion of their income on food and beverages (Nabia Statistical Agency, Citation2017). Considering the potential vulnerability of these households to the impact of the lockdown, it is intuitive that they were among the regions in which more households received the grant.
We further validate the results by looking at the receipt of the grant based on households’ food expenditure per capita in the previous 6 months preceding the lockdown, source of income for food and other socioeconomic characteristics. The results (see Figure A2 in the appendix) show that receipt of the income grant was higher among households that had low food expenditure per capita than their counterparts who had higher food expenditure per capita. This observation is consistent with the criteria used by the government as the core rationale for the distribution of the grant was to reduce the vulnerability of low-income households to food insecurity and other livelihood-related shocks of the lockdown.
Observe from Figure A3 in the appendix that satisfaction with the income grant policy was generally higher among households in the middle of the distribution of food expenditure per capita but low among households in the upper and lower ends of the distribution. These dynamics of the distribution can be interpreted from two perspectives. Households at the upper end of the food expenditure per capita distribution might have enough income to meet their needs during the lockdown. As a result, they might not necessarily rely on the income grant. Households in the lower end of the distribution might also have relatively higher food needs possibly due to their households’ sizes.
2.3. Effect of the income grant on households’ food security
We precede the discussion of the results with the post-estimation analysis of the quality of the matching. The overlap assumption, which is a major requirement for the use of the treatment effect estimators requires that everyone has a positive probability of receiving each treatment level. The assumption is satisfied when there is a chance of seeing observations in both the control and the treatment groups at each combination of covariate values (Busso et al., Citation2014). shows that all the covariates in the treatment group of the model for receipt of the income grant were balanced after the matching.
Consistent with , Table A1 in the appendix shows that the observable individual and household characteristics used for the matching between the two groups have insignificant biases as far as households’ receipt of the grant is concerned. These results confirm that the observable individual and household characteristics used for calculating the propensities are sufficient for matching the treated (receipt of the grant) and control (non-receipt of the grant). The results also provide enough bases for the use of the matching estimation technique and the interpretation of the associated average treatment effects.
Having satisfied the preconditions for using the matching estimators, we present in the estimates of the average treatment effect of the income grant on households’ food security. The results generally suggest that on average, receipt of the income grant contributed to a reduction in food insecurity by 11% to 17% for the additive index and 0.36–0.47 for the principal component analysis index. The results are sensitive to the different matching techniques used in the analysis. Nonetheless, there is an element of consistency in the potential impact of the policy in helping to ameliorate households’ food insecurity. The results corroborate the findings of Arndt et al. (Citation2020) whose study emphasised the importance of the government’s income transfer for low-income households in times of crisis.
Table 6. Effect of the income grant on food security–full sample.
As indicated in the introduction, hunger and malnutrition have disproportionate impacts on women and girls. It is therefore imperative for efforts towards the reduction of food insecurity to treat it as an equality, rights, and social justice issue (Longo, Citation2016). The results of our analysis of this gender element (see ) generally indicate that receipt of the income grant had a relatively higher effect on the food security of male-headed households than female-headed households. The results consistently show that the grant contributed to easing the food security challenges of the beneficiary households during the lockdown. This observation supports the propositions of earlier studies that policy interventions like the income grant must be gender sensitive (Doss et al., Citation2020; Bukari et al., Citation2022). This is evident by the consistency of the results regardless of the food security indicators that we used in the analysis.
Table 8. Correlates of households’ satisfaction with the income grant policy.
2.4. Robustness check
We further use the inverse probability weightingFootnote4 (IPW) methods to assess the robustness of the estimates in Tables and . Like the matching estimators, the IPW methods are also based on the propensity score. The approach involves estimation of the propensity score values, and then using those estimates to weight the outcome values (Abadie & Cattaneo, Citation2018). As reported in Table A2 in the appendix the IPW estimates are consistent with those of the matching estimators as far as the direction of effects are concerned. Although the estimates are relatively lower than those of the matching estimates, on average the income grant contributed to about an 8% to 10% reduction in households’ food insecurity.
Table 7. Effect of the income grant on food security by gender of the household head.
Across the gender of the household head (see Table A3) the same consistency of the direction of the effect is observed although the estimates appear to be weakly significant possibly due to the weights imposed on them. The results support earlier advocacy by Altman et al. (Citation2009) for an improved system of social protection such as income grants that stabilises food consumption in the South African context. The results also support the findings of similar studies conducted in Malawi (Miller et al., Citation2011), and South Africa (Waidler & Devereux, Citation2019) that found evidence of similar income grants in improving households’ food security.
2.5. Households’ satisfaction with the emergency income grant policy
As a validation of the treatment effect estimates, we further analyse households’ satisfaction with the emergency income grant. We focus on households’ consumption expenditure 6 months before the lockdown and their main source of income for food. The results (see Table ) show that households that had higher food consumption expenditure 6 months before the lockdown were less likely to find the income grant useful. A percentage increase in a household's expenditure before the lockdown is associated with approximately a 12–15% reduction in the likelihood that it would be satisfied with the emergency income grant policy. It can be inferred from these results that vulnerable households might find the policy more useful than non-vulnerable households. While households that relied on family support for income for food did not find the policy useful, those who relied on other sources such as government, charity and benevolence were satisfied with the policy.
In relation to the characteristics of the household head, we find that age and level of education were positively associated with the likelihood that the household would find the policy useful. At a 1% level of statistical significance, a household head who had completed basic education was about 32%-% to 34% more likely to be satisfied with the policy than his/her counterpart who has no education. The corresponding likelihood for a household head who had completed at least secondary school was about 47% to 50%. Compared to the unemployed, employed household heads were less likely to be satisfied with the policy, although the results are statistically insignificant. Married household heads were also less likely to be satisfied with the policy than their counterparts who were never married.
An important inference that can be drawn from this analysis is that the policy might benefit vulnerable households more than those who had relatively better livelihoods and stable sources of income for food before the outbreak of the pandemic and the subsequent lockdown policy. As many developing countries explore different ways to rebuild their economies from the impact of the pandemic, these results underscore the essence of well-targeted income transfer policies to complement the effort towards closing the existing income gap which has a lot of implications for human capital development and sustained growth.
3. Conclusion
As COVID-19 has ravaged the economies of countries around the world, tried and tested policies with potentially higher impact are required for a quick and robust recovery in the post-pandemic. Such policies must complement existing efforts intended to reduce inequality, promote inclusion, improve wellbeing and spur sustained growth in particularly, developing countries. This study has assessed the potential of one such policy implemented by the government of Namibia during the height of the pandemic. The study combines matching estimators with inverse probability weighting method to assess the effect of the government’s one-off income grant on households’ food security. It further used logistic regression to analyse households’ satisfaction with the income grant policy.
The findings suggest that although the policy was intended to be a temporary measure to help households to cope with the financial distress associated with the COVID-19-related restrictions on human movement, it has the potential to be institutionalised as a major social intervention to improve the welfare of economically vulnerable households in the country. The results across all the models reveal that if the policy is well-targeted and efficiently implemented, it could improve households’ food security and nutrition. Female-headed households stand to gain more from the policy than their male-headed counterparts. Based on these findings, this study recommends that the government of Namibia and other developing countries with similar food security and nutritional challenges must implement and sustain this type of policy in their post-pandemic recovery efforts. Such a programme should be gender-responsive and targeted at household heads who make decision over food consumption and other household arrangement for a bigger impact.
Disclosure statement
No potential conflict of interest was reported by the author(s).
Notes
1 Stage 1 which took place from 28 March to 4 May 2020 was classified as full lockdown while stage 2 (strict precaution) took place from 5 May to 1 June. Stage 3 (moderate precaution) and stage 4 (relaxed precaution) took place from 2 June to 29 June, and 30 June to 17 September. Stage 5 (a new normal) took place from 18 September to 30 December 2020.
2 It is important to emphasize that this research is purely an academic exercise that is neither motivated nor supported in kind/cash by any national or international institutions.
3 Detailed explanation and functional specification of the various matching estimators used in this analysis can be found in the work of Abadie & Cattaneo (Citation2018).
4 See Abadie & Cattaneo (Citation2018) for detail explanation of the IPW methods
References
- Abadie, A & Cattaneo, MD, 2018. Econometric methods for program evaluation. Annual Review of Economics 10, 465–503.
- Abdeen, A, Kharvari, F, O'Brien, W & Gunay, B, 2021. The impact of the COVID-19 on households’ hourly electricity consumption in Canada. Energy and Buildings 250, 111280.
- Akrofi, MM & Antwi, SH, 2020. COVID-19 energy sector responses in Africa: A review of preliminary government interventions. Energy Research & Social Science 68, 101681.
- Almeida, V, Barrios, S, Christl, M, De Poli, S, Tumino, A & van der Wielen, W, 2021. The impact of COVID-19 on households´ income in the EU. The Journal of Economic Inequality, 1–19.
- Altman, M, Hart, T & Jacobs, P, 2009. Food security in South Africa. tech. Rep. HSRC, Cape Town.
- Amesho, JN, Ahmadi, A & Lucero-Prisno III, DE, 2020. The calculated responses against COVID-19 in Namibia. The Pan African Medical Journal 37(Suppl 1), 25.
- Arndt, C, Davies, R, Gabriel, S, Harris, L, Makrelov, K, Robinson, S, … Anderson, L, 2020. COVID-19 lockdowns, income distribution, and food security: An analysis for South Africa. Global Food Security 26, 100410.
- Asmah, EE & Orkoh, E, 2017. Self-care knowledge of hypertension prevention and control among women in Contemporary Ghana. American Journal of Health Education 48(6), 374–81.
- Bahta, YT & Musara, JP, 2022. Quantifying the impact of COVID-19 relief vouchers schemes on food security: Empirical evidence insights from South Africa. Land 11(9), 1431.
- Bank of Namibia, 2022. Annual report, PART B: State of the economy - Macroeconomic Review. Retrieved from https://www.bon.com.na/Publications/Annual-Report/PART-B.aspx#mainContentWrapper.
- Becker, SO & Ichino, A, 2002. Estimation of average treatment effects based on propensity scores. The Stata Journal: Promoting Communications on Statistics and Stata 2(4), 358–77.
- Blumberg, SJ, Bialostosky, K, Hamilton, WL & Briefel, RR, 1999. The effectiveness of a short form of the household food security scale. American Journal of Public Health 89(8), 1231–4.
- Breisinger, C, Raouf, M, Wiebelt, M, Kamaly, A & Karara, M, 2020. Impact of COVID-19 on the Egyptian economy: Economic sectors, jobs, and households (Vol. 6). Intl Food Policy Res Inst.
- Bukari, C, Aning-Agyei, MA, Kyeremeh, C, Essilfie, G, Amuquandoh, KF, Owusu, AA, … Bukari, KI, 2022. Effect of COVID-19 on household food insecurity and poverty: Evidence from Ghana. Social Indicators Research 159(3), 991–1015.
- Busso, M, DiNardo, J & McCrary, J, 2014. New evidence on the finite sample properties of propensity score reweighting and matching estimators. Review of Economics and Statistics 96(5), 885–97.
- Caliendo, M & Kopeinig, S, 2008. Some practical guidance for the implementation of propensity score matching. Journal of Economic Surveys 22(1), 31–72.
- Charan, J & Biswas, T, 2013. How to calculate sample size for different study designs in medical research? Indian Journal of Psychological Medicine 35(2), 121.
- Chiwona-Karltun, L, Amuakwa-Mensah, F, Wamala-Larsson, C, Amuakwa-Mensah, S, Abu Hatab, A, Made, N, … Bizoza, AR, 2021. COVID-19: From health crises to food security anxiety and policy implications. Ambio 50(4), 794–811.
- Creswell, JW, 2015. Revisiting mixed methods and advancing scientific practices. In S Hesse-Biber & J RB (Eds.), The Oxford handbook of multimethod and mixed methods research inquiry. (pp. 61–71) Oxford University Press, New York.
- Daniel, WW & Cross, CL, 2018. Biostatistics: A foundation for analysis in the health sciences. Wiley.
- Deloitte, 2020. COVID-19 Pandemic: Announcement of economic stimulus and relief package by the Minister of Finance: Tax Alert 3/2020, Namibia.
- Devereux, S, Béné, C & Hoddinott, J, 2020. Conceptualising COVID-19’s impacts on household food security. Food Security 12(4), 769–72.
- Doss, C, Njuki, J & Mika, H, 2020. The potential intersections of COVID-19, gender and food security in Africa. Journal of Gender, Agriculture and Food Security 5(1).
- Evelina, J, Nuugulu, S & Lukas Homateni, J, 2020. Estimating the economic impact of COVID-19: A case study of Namibia. University Library of Munich, Germany.
- Heckman, JJ, Ichimura, H & Todd, PE, 1997. Matching as an econometric evaluation estimator: Evidence from evaluating a job training programme. The Review of Economic Studies 64(4), 605–54.
- IPC, 2021. Acute Food Insecurity Analysis October 2021 - March 2022. Access on 6 March 2022 from https://www.ipcinfo.org/ipc-country-analysis/details-map/en/c/1155353/.
- Kaisara, G & Bwalya, KJ, 2020. Investigating the E-learning challenges faced by students during COVID-19 in Namibia. International Journal of Higher Education 10(1), 308–18.
- Kansiime, MK, Tambo, JA, Mugambi, I, Bundi, M, Kara, A & Owuor, C, 2021. COVID-19 implications on household income and food security in Kenya and Uganda: Findings from a rapid assessment. World Development 137, 105199.
- Legal Assistance Center, 2017. Namibia Gender Analysis 2017. http://www.lac.org.na/projects/grap/Pdf/Namibia_Gender_Analysis_2017.pdf.
- Lendelvo, SM, Pinto, M & Sullivan, S, 2020. A perfect storm? The impact of COVID-19 on community-based conservation in Namibia. Namibian Journal of the Environment 4, 1–15.
- Longo, P, 2016. Food justice and sustainability: A new revolution. Agriculture and Agricultural Science Procedia 8, 31–6.
- Miller, CM, Tsoka, M & Reichert, K, 2011. The impact of the social cash transfer scheme on food security in Malawi. Food Policy 36(2), 230–8.
- Ministry of Finance, 2020. Economic stimulus and relief package: Impact of COVID-19 on the economy and households, media statement. Ministry of Finance, Namibia.
- Namibian Statistical Agency, 2021. Namibia Multidimensional Poverty Index (MPI) Report 2021. Retrieved from https://ophi.org.uk/wp-content/uploads/Namibia_MPI_report_2021.pdf.
- Namibia Statistics Agency, 2017. Namibia household income and expenditure survey (NHIES) 2015/2016 report. Windhoek. Namibia.
- Neuman, WL, 2014. Pearson new international edition social research methods: Qualitative and quantitative approaches. Pearson Education Limited, England.
- Orkoh, E, 2018. Gender welfare effects of regional trade integration on households in Ghana. In Trade and poverty reduction: New evidence of impacts in developing countries. (pp. 36–57). World Trade Organization, Geneva.
- Orkoh, E & Efobi, U, 2023. Effects of behaviour change communication on knowledge and prevention of malaria Among women in Ghana. Evaluation Review, doi:10.1177/0193841X231194565
- Rosenbaum, PR, 2002. Covariance adjustment in randomized experiments and observational studies. Statistical Science 17(3), 286–327.
- Rubin, DB & Thomas, N, 1996. Matching using estimated propensity scores: Relating theory to practice. Biometrics, 249–64.
- Saturni, S, Bellini, F, Braido, F, Paggiaro, P, Sanduzzi, A, Scichilone, N, … Papi, A, 2014. Randomized controlled trials and real life studies. Approaches and methodologies: A clinical point of view. Pulmonary Pharmacology & Therapeutics 27(2), 129–38.
- Shangula, K, 2020. Update by Dr Kalumbi Shangula, minister of health and social services on COVID-19 in Namibia. Ministry of Health and Social Services.
- Tavakol, M & Dennick, R, 2011. Making sense of Cronbach's alpha. International Journal of Medical Education 2, 53.
- Tirivangani, T, Alpo, B, Kibuule, D, Gaeseb, J & Adenuga, BA, 2021. Impact of COVID-19 pandemic on pharmaceutical systems and supply chain – a phenomenological study. Exploratory Research in Clinical and Social Pharmacy 2, 100037.
- United Nations, 2020. Socioeconomic impact assessment of COVID-19 in Namibia. The United Nations System (UNS) in Namibia.
- Waidler, J & Devereux, S, 2019. Social grants, remittances, and food security: Does the source of income matter? Food Security 11(3), 679–702.
- White, H & Sabarwal, S, 2008. Quasi-experimental design and methods, methodological briefs: Impact evaluation 8. UNICEF Office of Research, Florence, Italy.
Appendix
Figure A1. Effect of the lockdown based on households’ food expenditure per capita. Source: Authors’ computation based on field survey 2020

Figure A2. Households’ food expenditure per capita and receipt of the grant. Source: Authors’ computation based on field survey 2020

Figure A3. Households’ satisfaction with the policy based on their food expenditure per capita. Source: Authors’ computation based on field survey 2020

Table A1. Checking the balancing quality
Table A2. Inverse probability weighting estimates-full sample
Table A3. Inverse probability weighting estimates-Gender of the household head