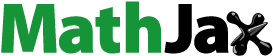
ABSTRACT
The notion of the “complex dual hesitant fuzzy set (CDHFS)” is the combination of the “dual hesitant fuzzy set (DHFS)” and the “complex fuzzy set (CFS).” It is characterized by two degrees, namely the membership and nonmembership, in the form of a finite subset on a unit disc in the complex plane. CDHFS is useful for dealing with real-world problems involving uncertain or hard-to-predict information. Also, to approximate smoothly, the Einstein operators are well-known aggregation operators, while prioritized operators are effective tools for prioritization among criteria. Therefore, the goal of this study is to develop some prioritized aggregation operators under the CDHFS environment; namely the complex dual hesitant fuzzy prioritized averaging (CDHFPA) operator, the complex dual hesitant fuzzy prioritized geometric (CDHFPG) operator, the complex dual hesitant fuzzy Einstein prioritized averaging (CDHFEPA) operator, and complex dual hesitant fuzzy Einstein prioritized geometric (CDHFEPG) operator. Some properties of the proposed operators are investigated in detail. In addition, a multi-criteria decision-making (MCDM) method based on the proposed operators with the complex dual hesitant fuzzy setting is developed. Moreover, a numerical example is given for the application and effectiveness of the developed MCDM approach. A comparison study is also done with existing methods to show that the proposed MCDM method is better and more reliable. The study finds that if the expert’s preference is used to choose the right aggregation operators, the decision maker will have access to a wide range of compromise solutions.
Introduction
The fuzzy set (FS) theory was first initiated by Zadeh (Citation1965). It has been used widely and successfully in all areas of real-life decision-making problems (Yager Citation1977), and it is a good way to show data that needs to be clarified. Only the degree of membership function mapping to the close interval [0, 1] is defined by an FS. But because the membership function only has one value, it cannot show proof of endorsement or rejection in many cases. So, more than the information we can get from the FS is needed for many practical situations. To overcome this situation, Atanassov (Citation1986) was the first to develop the concept of an intuitionistic fuzzy set (IFS), which is an extension of FS. The IFS is characterized by membership and non-membership functions such that their sum . People think that IFS is better than traditional FS theory at dealing with ambiguity and uncertainty. The notion of IFS is widely applied to deal with decision-making (DM) problems (Beg and Rashid Citation2014; Boran et al. Citation2009; De, Biswas, and Roy Citation2001; Li Citation2005). Torra and Narukawa (Citation2009) and Torra (Citation2010) introduced the theory of hesitant fuzzy sets (HFS), which presented a new generalization form of FS. It is characterized by a membership function having a set of possible values from the close interval [0,1]. Based on the relationship between the hesitant fuzzy elements (HFEs) and the IF values (IFVs), Xia and Xu (Citation2011) developed some hesitant fuzzy (HF) operational laws. Zhu, Xu, and Xia (Citation2012) developed dual hesitant fuzzy set (DHFS) to generalize IFS with HFS. DHFSs comprise the membership hesitancy function and the nonmembership hesitancy function. These functions make it easier and more flexible to give each element in the domain a value. In this circumstance, we must deal with two different types of hesitancy. We do not face an interval of possibilities; rather, the current sets, including FSs, IFSs, and HFSs, can be regarded as special cases of DHFSs or some possibility distributions on the possible values or multiple occurrences of an element, but several different possible values indicate the epistemic degrees, whether there is certainty or uncertainty. For instance, in a multicriteria decision-making situation, some decision makers take into account 0.2, 0.3, and 0.4 as possible values for the membership degree of
into the set
, and 0.5, 0.6, and 0.7 as possible values for the nonmembership degrees instead of simply one integer or a tuple. Therefore, there is some restriction on the confidence and uncertainty of the potential values, which can more accurately reflect the initial information provided by the decision makers. Moreover, Limboo and Dutta (Citation2022) developed a q-rung orthopair basic probability assignment method and applied the concept to medical diagnosis. Furthermore, Das and Granados (Citation2022) initiated the notion of the FP-intuitionistic multi-fuzzy N-soft set and its induced FP-Hesitant N soft set, which are the extension of IFS and HFS, respectively. Riaz and Farid (Citation2022) developed some picture fuzzy aggregation operators and proposed an MCDM method for the selection of third-party logistic providers.
Some scholars have questioned what will happen if we convert the fuzzy set’s range into a unit disc in the complex plane. Due to this reason, Ramot et al. (Citation2002) developed the concept of complex fuzzy set (CFS), which contains the degree of membership function (amplitude and phase terms) as a complex number of a unit disc in the complex plane. CFS deals with two dimensions of information in a single set. CFS is an effective method for demonstrating how people believe that grades are formed. The complex fuzzy set considers only the degree of membership; the non-membership component of the data entities, which also assumes an equal role in evaluating the object in the decision-making process, is not given any weight. Although it is frequently challenging to describe the assessment of the membership degree by an accurate number in a fuzzy collection in the real world. After the appearance of CFS, many researchers have started working on it and applied the concept to MCDM problems (Xiao and Ding Citation2019; Munir et al. Citation2020; Ullah, Mahmood, and Garg Citation2020; Mahmood et al. Citation2019; Ali and Mahmood Citation2020a, Citation2020b; Liu, Mahmood, and Ali Citation2019).
CFS only deal with complex-valued membership degree but does not weigh the non-membership degree, which plays an equal role in the decision-making process for system evaluation. However, it is frequently challenging to represent membership degree estimation by a fuzzy set’s precise value in the real world. In these circumstances, using two dimensions of information rather than one may make it easier to portray the ambiguity and vagueness that exist in the real world. Due to uncertainties’ hesitant assessment in the intricate decision-making problem, an expansion of the existing theories may be very helpful for explaining uncertainties. Due to this, Liu, Mahmood, and Ali (Citation2019) expanded the definition of complex fuzzy sets to include complex intuitionistic fuzzy sets (CIFS) by integrating complex degrees of non-membership functions, and their fundamental characteristics were investigated. Garg and Rani (Citation2021, Citation2019) developed some aggregation operators to aggregate the CIF information.
Moreover, Garg et al. (Citation2021) extended the CFS notion and developed the complex hesitant fuzzy set (CHFS) concept. CHFS is the result of combining the HFS with CFS. CHFS have only the degree of membership function in which all the possible values belong from the close interval [0,1]. CHFS deals with the two-phase information precisely and is a better tool for tackling this information. Recently, Mahmood et al. (Citation2020) generalized the concept of CHFS and introduced the complex dual hesitant fuzzy set (CDHFS), characterized by complex valued hesitant fuzzy membership degree and complex valued hesitant fuzzy non-membership degree. The complex dual hesitant fuzzy set is characterized by complex-valued membership and nonmembership degrees and is represented in polar coordinates membership and nonmembership degrees give the extent of belongingness and does not belongingness of an object in a CDHFS, and the phase term associated with membership and nonmembership degree gives the additional information, generally related with periodicity. The phase terms are novel parameters of the membership and nonmembership degrees and these are the parameters which distinguish the CDHFS and traditional DHFS theories. The DHFS theory only considers one dimension at a time, which occasionally causes information loss.
Literature Review
The idea of aggregation operators plays an important role in aggregating overall preference data in making decisions where the experts provide the preference values for certain information. For this reason, numerous aggregation operators have been established. Xu (Citation2007) developed some IF weighted operators and discussed some properties of the developed operators. The aggregation operators for IFNs discussed above made the assumption that the attributes and the decision makers had the same priority level, which is something else that should be mentioned. However, in multiple attribute group decision-making issues, decision makers and attributes have various priority levels. Therefore, to overcome this shortcoming, Yu (Citation2013) developed IF prioritized operators. Moreover, Kefeng and Bin (Citation2017) utilized the Einstein t-norm and t-conorm Klement, Mesiar, and Pap (Citation2004) and developed some IF Einstein prioritized operators. Yu (Citation2014) developed some Einstein weighted aggregation operators such as hesitant fuzzy Einstein weighted averaging (HFEWA) operator and hesitant fuzzy Einstein weighted geometric (HFEWG) operator. Wei (Citation2012) established certain prioritized aggregation operators for collecting hesitant fuzzy data and afterward utilized them to develop models for hesitant fuzzy MADM problems with different priority levels because of the possibility of prioritized aggregation operators. Yu et al. (Citation2016) developed some hesitant fuzzy Einstein aggregation operators, such as the hesitant fuzzy prioritized Einstein averaging (HFPEA) operator and hesitant fuzzy prioritized Einstein geometric (HFPEG) operator. Zhou and Li (Citation2014) developed some generalized hesitant fuzzy prioritized Einstein averaging in order to deal with MCDM problems under HFS environment. Further, Yu et al. (Citation2016), developed dual hesitant fuzzy (DHF) aggregation operators, namely the DHF weighted average (DHFWA) operator and the DHF weighted geometric (DHFWG) operator and discussed some of their properties. Moreover, Zhao, Xu, and Liu (Citation2017), developed the concept of DHF t-norm and t-conorm based operators for aggregating DHF elements. Extending the notion of DHF aggregation operators, Zhao and Xu (Citation2018) developed prioritized dual hesitant fuzzy aggregation operators and applied the concept to multiple criteria decision making (MCDM) problems. Based on Einstein t-norm and t-conorm, Biswas and Sarkar Biswas and Sarkar (Citation2018) proposed the idea of DHF prioritized operators to deal with MCDM problems. Garg and Rani (Citation2021, Citation2019) developed some aggregation operators to aggregate the CIF information. Furthermore, Talafha et al. (Citation2021) developed some aggregation operators under CHFS environment and applied the concept to MCDM problems. Ali et al. (Citation2021) developed some Einstein aggregaton operators to deal with Green Supplier Chain Management under complex interval valued Pythagorean fuzzy set environment. The weighted aggregation operators play a crucial role in order to aggregate the preference information. But cannot deal with the prioritization among the experts or decision criteria.
Gap, Motivation, and Contribution
Although many decision-making methods such as TOPSIS, VIKOR, COPRAS, WASPAS, EDAS, and MULTIMOORA have been proposed and applied to real-world problems. However, the aggregation operators not only aggregate the opinion of the experts but also deal with the prioritized relation among the decision makers and decision criteria. In aggregating the experts’ information, aggregation operators play an important role. The advantage of the prioritized operators is that they deal with the prioritized relationship among the experts or criteria, while all other operators assume the criteria are of the same priority level. For example, if and
are two attributes with the weight
and
, respectively. Based on the above aggregation operator, we can compensate for a decrease in
satisfaction to attribute
by gaining
in satisfaction to attribute
. But in many real decision-making problems, this kind of compensation between attributes is impossible.
Consider a scenario in which a mother decides based on the cost and safety of powdered milk for her child. She should not permit a benefit related to the price of powdered milk to compensate for a safety hazard. This is a typical form of attribute prioritization, in which safety has a greater priority than cost. As previously explained, the DHFS theory only evaluates one dimension simultaneously, resulting in occasional information loss. To define membership and non-membership grades, a second dimension must be included because, in the actual world, we encounter natural occurrences with several dimensions. By adding this second dimension of information, the entire data collection can be projected in one set. We present an illustration to illustrate the importance of phase phrases. Suppose an organization installs biometric-based attendance devices (BBADs) at each regional office. To accomplish this, the company consults an expert for information regarding (a) BBAD models and (b) BBAD production dates. The organization desires to concurrently select the optimal BBAD model and manufacturing date. The two aspects of this dilemma are the BBADs’ model and date of manufacture. This problem cannot be adequately modeled using standard DHFS theory, as DHFS theory cannot simultaneously address two dimensions. The CDHFS hypothesis is the most effective method for communicating the expert’s knowledge. The phase terms in CDHFS can represent the company’s view on the production date of BBADs, while the amplitude terms can be used to express the company’s view on the model of BBADs. In this research, we design the prioritized aggregation technique for CDHFVs, prompted by the CDHFS and bearing in mind the benefits of the priority relationship among the criteria. Our efforts can be summed up as follows:
To define the score function for the comparison of CDHFVs.
Propose operational laws and Einstein operational laws for the CDHFVs.
To develop complex dual hesitant fuzzy prioritized average (CDHFPA) operator and complex dual hesitant fuzzy prioritized geometric (CDHFPG), complex dual hesitant fuzzy prioritized Einstein averaging (CDHFPEA) operator, and complex dual hesitant fuzzy prioritized Einstein geometric (CDHFPEG) operator.
To investigate properties of the developed operators.
Develop an MCDM method based on the developed operators.
A numerical illustration of the hotel selection problem is provided to illustrate the applicability and utility of the established method.
To do this, the remainder of the paper is organized as follows. In Section 2, some basic concepts are presented and preliminary operations are discussed. Section 3 dealt with some algebraic and Einstein operational laws and investigated some of the properties of the proposed operational properties. In Section 4, using the operational laws, we develop complex dual hesitant fuzzy prioritized operators such as complex dual hesitant fuzzy prioritized averaging (CDHFPA) operator, complex dual hesitant fuzzy prioritized geometric (CDHFPG) operator, and complex dual hesitant fuzzy prioritized Einstein averaging (CDHFPEA) operator, complex dual hesitant fuzzy prioritized Einstein geometric (CDHFPEG) operator. Some properties of the developed operators are also discussed. Section 5 develops an MCDM approach based on the proposed operators. Section 6 deals with a numerical example related to the faculty selection problem for applying the proposed method. In Section 7, a comparative study is also conducted for the effectiveness and legitimacy of the proposed method. Concluding remark is in section 8.
Preliminaries
In this section, we review some basic definitions and operational laws of complex hesitant fuzzy set and complex dual hesitant fuzzy set
, which can be applied in our next sections.
Complex Hesitant Fuzzy Set
The theory of CHFS was first initiated by Garg et al. (Citation2021) as an extension of a complex fuzzy set (Ramot et al. Citation2002). CHFS is characterized by a membership degree of some possible values that contains amplitude and phase term belonging to and
, respectively. It can be defined as:
Definition 1.
(Garg and Rani Citation2021) Let be a universe of discourse. Than CHFS
is of the form:
where represent the complex-valued of membership which the subset of the unit disc in complex plane with a condition
Furthermore,
is called a complex hesitant fuzzy number (CHFN).
Definition 2.
(Garg and Rani Citation2021) Let be two CHFNs.Then their complement,union and intersection are define as follows:
(1)
(2)
(3)
Complex Dual Hesitant Fuzzy Set
The theory of complex dual hesitant fuzzy set was first initiated by Mahmood et al. [34] as an extension of complex hesitant fuzzy set.
Definition 3.
(Talafha et al. Citation2021) Let U be a universe of discourse. A CDHFS is of the form
where and
represented the complex-valued membership grade and non-membership grade,which are subsets of a unit disc in the complex plane with conditions
and
where
for and
. The pair
is called complex dual hesitant fuzzy element (CDHFE).
Definition 4.
(Talafha et al. Citation2021) Let be two CDHFEs. Then their complement,union and intersection are define as follows:
Complex Dual Hesitant Fuzzy Operational Laws
In this section, first we define score and accuracy functions in order to compare CDHFEs. We also develop operational laws for CDHEs.
Definition 5.
Let be two CDHFEs. Then
is said to be the score function of and
shows the accuracy function of . Here
and
shows the number of elements in
and
In the following, we define operational laws for CDHFEs.
Definition 6.
Let be two CDHFEs. Then their addition,multiplication and complement are define as follows:
In what follows, we review the complex t-conorm and t-norm respectively (Mahmood et al. Citation2020):
Based on the above Einstein t-conorm and t-norm, we can define the Einstein sum and Einstein product of CDHFEs as below:
Definition 7.
Complex Dual Hesitant Fuzzy Prioritized Operators
In this section, we define some aggregation operators based on Definition 6 and Definition 7. By using the operational laws presented in Definition 6, in the following we define some prioritized operators under CDHFS environment.
Definition 8.
Let , be CDHFEs, and
, where
and is the score of CDHFE
. Then a mapping
defined by:
is called a complex dual hesitant fuzzy prioritized averaging (CDHFPA) operator.
Theorem 1.
Let
be a collection of CDHFEs and
where
and is the score of CDHFE
. Then, the aggregated value by using CDHFPA operator is also a CDHFE and
Proof:
To prove the theorem, we shall use mathematical induction. First, we prove that the statement is true for . Therefore,
Then,
We suppose that the theorem is true for . We have to show that the theorem is true for
. To do this, we have
Now, when ,
So the Theorem is true for and thus the statement is true for all
.
Theorem 2. (Idempotency) Let be a groups of CDHFEs and
where
and
is the score of CDHFE
. If all
are equal, i.e.,
for all
, wherever
then
Proof: We have
Theorem 3.
(Additivity) Let be a collections of CDHFEs, and
be another CDHFE, and
where
and
is the score of CDHFE
. Then
Proof:
By using operational laws of CDHFEs, we have
Thus, by using Theorem 1, we have
By using operational laws of CDHFEs, we have
Hence,
Definition 9.
Let be the CDHFEs, and
where
and
is the score of CDHFE
. Then a mapping
defined by:
is called a complex dual hesitant fuzzy prioritized geometric (CDHFPG) operator.
Theorem 4.
Let be a collection of CDHFEs, and
where
and
is the score of CDHFE
. Then the aggregated value by using CDHFPG operator is also a CDHFE and
Proof:
Proof of the Theorem is same as Theorem 1.
Theorem 5.
(Idempotency) Let be a collections of CDHFEs and
where
and
is the score of CDHFE
. If all
are equal, i.e.,
for all
, where
, then
Proof:
Proof of the Theorem is same as Theorem 2.
Theorem 6.
(Additivity) Let be a collections of CDHFEs, and
be another CDHFE, and
where
and
is the score of CDHFE
. Then
Proof:
Proof of the Theorem is same as Theorem 3
The above aggregation operators are based on the operational laws defined in Definition 6. In the following we develop complex dual hesitant fuzzy Einstein Prioritized (CDHFWP) operators based on operational laws defined in Definition 7.
Definition 10.
Let be a collection of CDHFEs and
where
,
and
is the score of CDHFE
. Then, a mapping
defined by:
is called a complex dual hesitant fuzzy prioritized Einstein averaging (CDHFPEA) operator.
Theorem 7.
Let be a collection of CDHFEs and
where
and
is the score of CDHFE
. Then the aggregated value by using CDHFPEA operator is also a CDHFE and
Proof:
Using the mathematical induction method,the theorem will be proved. The theorem is obvious for . We suppose that the theorem is valid for
. We will to show that the theorem is valid for
. We have
Now when ,
Hence, the Theorem is true for and thus is true for all
.
Theorem 8.
Let be a collection of CDHFEs and
where
and
is the score of CDHFE
. Then the aggregated value by using CDHFPEG operator is also a CDHFE and
Proof:
The proof of this Theorem is similar to the proof of Theorem 7.
Theorem 9.
(Idempotency) Let be a collection of CDHFEs and
where
and
is the score of CDHFE
. If all
are equal, i.e.,
for all
,where
, then
Proof: We have
Hence, the theorem is proved.
Theorem 10.
be a collection of CDHFEs and
where
and
is the score of CDHFE
. If all
are equal, i.e.,
for all i, where
then
Proof:
The proof of this theorem is similar to the proof of Theorem 9.
Theorem 11. (Boundary)
Let be a collection of CDHFEs and
where
and
is the score of CDHFE
. Let
where,
. Then
Proof: We have,
From definition of , for all k, we get
for all . That is
i.e.,
i.e.,
i.e.,
i.e.,
Similarly, since
and
then
i.e.,
i.e.,
Then, from inequities (19), (20), and Definition 5, we obtained
Therefore, from the comparative laws of CDHFE, it is obvious that
Theorem 12. (Boundary)
Let be a collection of CDHFEs and
where
and
is the score of CDHFE
. Let
,
,
,
,
Then
Proof:
The proof of the theorem is obvious.
Theorem 13. (Additivity)
Let be a collection of CDHFEs and
where
and
is the score of CDHFE
. If
is another CDHFE, then
Proof:
Based on the operational laws of CDHFEs, we have
According to theorem 1, we have,
Again, from the operational laws of CDHFE.
Thus,
Theorem 14. (Additivity)
Let be a collection of CDHFEs and
where
and
is the score of CDHFE
. If
be another CDHFE, then
Proof:
The proof of this theorem is similar to the proof of theorem 11.
Decision Making Method Based on CDHFP Operators
Suppose be the set of alternative and let
be the collection of criteria and there priority is given as
in such a manner that criteria
has a higher priority than
if
Now
represents a set of decision makers and the linear ordering
represent prioritization between the decision makers in such a manner that decision maker
has a higher priority than decision maker
if
. Suppose that the decision matrix
is in the format of CDHF matrix. The element of this matrix are represented by CDHFEs as
which designates the value of the alternative
on the criteria
provided by the decision maker
,where the
assigns the degree of membership functions of the alternative
satisfies the criteria
shown by the decision maker
while
shows the degree of non-membership function of the same alternative relating to the same criteria.
By using the CDHFPEA and CDHFPEG operators to propose an approach in multi-criteria group decision making under CDHF enviroment, the main steps are described as follows:
Step 1. Construct the complex dual hesitant fuzzy decision matrix.
Step 2. Calculate the value of as follows:
where,
is the score of CDHFEs
.
Step 3: Calculate the CDHFEs for each alternative
using the CDHFPEA or CDHFPEG operators as follows:
Step 4. Ranking of all the alternatives by the developed score function explained in Definition 5, the highest value of
the larger the overall
and thus the best alternative
is determined.
Step 5. Ranking the alternatives based on score values.
Hotel Selection in Murree (Case Study)
In this part, a case study of the hotel selection problem in Murree, Pakistan, is done to show how the proposed method can be used. This case study’s evaluation values are based on what past visitors said about the criteria considered. Thus, hotel selection resembles decision making based on ratings (reviews) provided by visitors (experts). There are 137 hotels in Murree, Pakistan, of which 10 are five-star. Given the computation required, we selected the top 5 hotels out of all the five-star hotels in Murree as our research objective to illustrate the applicability of the suggested approach. The names of five hotels are : Himala Guest House,
: Arcadian Blue Pines,
: Shangrila Resort Hotel Murree,
: Khattak Lodge Murree, and
: Hotel One Mall Road Murree. Visitors (experts) rate the five hotels based on four criteria:
: cleanliness,
: value,
: sleeping quality,
: location. The criteria take over the prioritization as
. The four decision makers figure out the candidates
concerning the criteria
, and the evaluation values are presented in the form of CDHFEs. The complex dual hesitant fuzzy decision-making matrix
is given in .
Table 1. Complex dual hesitant fuzzy decision matrix.
Step 2.To fined the value of use the EquationEquations (25)
(25)
(25) and (Equation29
(29)
(29) )
Utilizing CDHFPEA Operators
Step 3. Utilize CDHFPEA operator to aggregate all CDHFEs (k = 1,2,3,4,5; j = 1,2,3,4) for each alternative
to reduce it in CDHFE
(k = 1,2,3,4,5). We have added calculation of only five values for amplitude term of complex valued membership degree.
For convenience we write here the value of
Step 4. By Definition 5, calculate the score values of the alternative
. The values are as follows:
Step 5. The ranking of alternatives is . Our best alternatives is
.
Utilizing CDHFPEG Operator
Step 3. Utilize CDHFPEG operator to aggregate all CDHFEs
for each alternative
to reduce in CDFE
. For the convenience, we write here only the value of
Step 4. By using to calculate the score values
of the alternative
. The score value are found as
Step 5. The ranking of alternatives is
. Here the best alternative is
.
Comparative Analysis with Existing Approaches
In this section the proposed method is compared with (Zhu, Xu, and Xia Citation2012), CHFEs (Garg and Rani Citation2019), HFEs (Torra and Narukawa Citation2009), CIFEs (Liu, Mahmood, and Ali Citation2019), and IFEs (Atanassov Citation1986).
Comparison with DHFNs
This section comprises a comparative study with DHFEs (Zhu, Xu, and Xia Citation2012). We compare the proposed method with DHFPEA operator (Zhao and Xu Citation2018), DHFPEG operator (Zhao and Xu Citation2018), DHFEWA operator (Biswas and Sarkar Citation2018), DHFEWG operator (Biswas and Sarkar Citation2018), DHFPA operators (Yu et al. Citation2016), DHFPG operator (Yu et al. Citation2016), DHFWA operator (Zhao, Xu, and Liu Citation2017), and DHFWG operator (Zhao, Xu, and Liu Citation2017). The comparative result of the proposed approach and existing methods is presented in .
Table 2. Ranking of alternatives of the proposed method and existing dual hesitant fuzzy approaches.
From , we see that the ranking of alternatives by using the proposed operator and
operator are
. The best alternatives by using both the operators are the same. Also, by utilizing
operator,the ranking of alternatives is
and the best alternative is
. By using
operator, the ranking of alternatives is
. For this operator the best alternative is
. The best alternative by using both the operators are not same, while in our approach, the best alternative is same. Also, in our approach
and
. While using
operator it is
and
. The proposed operators are most suitable as compared to
operator and
operator (because it involves
operator and
operator t-norm and t-conorm) as it involves Einstein t-norm and t-conorm which is the generalization of the existing operators. Also to compare the proposed operators with
operator and
operator. The ranking of alternatives by using the
operator is
and for
operator is
. The discussed operators have two different best alternatives which are
and
. However, in ranking order
and
are different from each other. Also, in our approach
and
. While using
operator it is
and
. Due to this reason our proposed operators is the most suitable then
operator and
operator. Furthermore we are going to compare our proposed operators with
operator and
operator. The ranking of alternatives for
operator is
and for
operator is
. The discussed operators have not the same best alternatives. Since there are two best alternatives but we want only one. Also, in first ranking order, the first alternative is
, and in second ranking order, first alternative is
which are different from each other. Also, in our approach
and
. While using
operator, it is
and
. That’s why our proposed operators are more authentic then
operator and
operator. Now the last comparison is between our proposed operators and
operators,
operator. The ranking of alternatives for
operator is
and for
operator is
The discussed operators have two different best alternatives which are
and
. The best alternative by using both the operators are not same, while in our approach, the best alternative is same. However, in ranking order
and
are different from each other. Due to this reason our proposed operators are more suitable then
operator, and
operator. Concluding that our proposed environment
is more informative than
. So, our proposed environment is the best.
Comparison with CHFSs
This section composed a comparative study with CHFEs (Garg and Rani Citation2019). We compare the proposed method with CHFWA operator CHFWG operator developed by (Garg and Rani Citation2019).
From , we see that the ranking of alternatives by using the operators developed in this paper is different from the operators defined by (Talafha et al. Citation2021). The operators developed by (Talafha et al. Citation2021) only deals with CHFNs and cannot deal with the CDHFVs. Also, the MCDM method proposed by (Talafha et al. Citation2021) cannot deal with the prioritized relationship among the criteria, while the developed operators capture the prioritized relationship among criteria. Therefore, the method developed in this paper is more effective than the existing ones.
Table 3. Ranking of alternatives of the proposed method and existing complex hesitant fuzzy approaches.
Comparison with HFNs
This section composed a comparative study with HFEs (Torra and Narukawa Citation2009). We compare the proposed method with HFPEA operator (Yu et al. Citation2016), HFPEG operator (Yu et al. Citation2016), HFEWA operator (Zhou and Li Citation2014), HFEWG operator (Zhou and Li Citation2014), HFPA operator (Xia and Xu Citation2011), HFPG operator (Xia and Xu Citation2011), HFWA operator (Torra Citation2010), and HFWG operator (Torra Citation2010).
In , we see that the ranking of alternatives by using the proposed operator is
and
operator is
The best alternatives by using both the operators are the same. However, the ranking order of
and
are different from each other. Also, by utilizing
operator, the ranking of alternatives is
For
operator, the ranking of alternatives is
The best alternatives by using both the operators are the same but the ranking order of alternatives is different from our proposed operators. since we use
So, our proposed operators result is more accurate than
operator and
operator. Also to compare the proposed operators with
operator and
operator. The ranking of alternatives by using the
operator, is
and for
operator, is
For both the operators the best alternative is same but the ranking order is different from our developed operators. So, our proposed operators result is best than
operator and
operator. Also to compare the proposed operators with
operator and
operator. The ranking of alternatives by using the
operator, is
For
operator, the ranking of alternatives is
The discussed operators have two different best alternatives which are
and
. The best alternative by using both the operators are not same, while in our approach, the best alternative is same. However, in ranking order
and
are different from each other. Due to this reason our proposed operators are more suitable then
operator, and
operator. Now the last comparison is between our proposed operators and
operators,
operator. For
operator, the ranking of alternatives is
. For
operator, the ranking of alternatives is
For both the operators the best alternative is same but the ranking order is different from our developed operators. Our developed operators is more suitable than
operator and
operator because our developed environment
is more informative than
Table 4. Ranking of alternatives of the proposed method and existing hesitant fuzzy approaches.
Comparison with CIFNs
In this section we compare the developed approach to CIFEs (Alkouri and Salleh Citation2012). we compare the proposed method with CIFEWA operator (Garg and Rani Citation2019), CIFEWG operator (Garg and Rani Citation2019), CIFPA operator (Garg and Rani Citation2021), CIFPG operator (Garg and Rani Citation2021), CIFWA operator (Alkouri and Salleh Citation2012), CIFWG operator (Alkouri and Salleh Citation2012).
In , we see that the ranking of alternatives by using the proposed operator is
and for
operator is
The best alternatives by using both the operators are the same. However, the ranking order of
and
are different from each other. Also, by utilizing
operator, the ranking of alternatives is
For
operator, the ranking of alternatives is
The ranking order is differ from our proposed operators. As we use
environment which is more informative than
So, our proposed operators is more accurate then
operator and
operator. Also to compare the proposed operators with
operator and
operator. The ranking of alternatives by using the
operator, is
and for
operator, is
. However, the ranking order of
and
are different from each other.For both the operators the best alternative is differ from each other. So, our proposed operators result is more accurate than
operator and
operator. Furthermore, to compare
operator and
operator with our proposed operators. The ranking of alternatives by using the
operator, is
and for
operator, is
For both the operators, the best alternative is same but the ranking order is different from our proposed operators. So, our proposed operators result is best than
operator and
operator. Now the last comparison is between our proposed operators and
operators,
operator. For
operator, the ranking of alternatives is
. For
operator, the ranking of alternatives is
For both the operators the best alternative is different from each other. Also the ranking order of
operator and
operator is different from our developed operators. Our developed operators is more suitable than
operator and
operator.
Table 5. Ranking of alternatives of the proposed method and existing complex intuitionistic fuzzy approaches.
Comparison with IFNs
In this section we compare the developed approach to IFEs (Atanassov Citation1986). We compare the proposed method with IFPEA operator (Xu Citation2007), IFPEG operator (Xu Citation2007), IFEWA operator (Xu Citation2007), IFEWG operator (Xu Citation2007), IFPA operator (Yu Citation2013), IFPG operator (Yu Citation2013), IFWA operator (Kefeng and Bin Citation2017), IFWG operator (Kefeng and Bin Citation2017).
In , we see that the ranking of alternatives by using the proposed operator is
and for
operator is
The best alternatives
by using both the operators are the same. However, the ranking order of
and
are different from each other. Also, by utilizing
operator,the ranking of alternatives is
For
operator, the ranking of alternatives is
The best alternative and ranking order is different from our proposed operators. As we use
environment which is more informative than
So our proposed operators result is more suitable then
operator and
operator. Also to compare the proposed operators with
operator and
operator. The ranking of alternatives by using the
operator, is
and for
operator, is
. In this two rankings to find the score values of the alternatives
and
, we use accuracy function. For both the operators the best alternative is same but the ranking order is different from our proposed operators. As we use
environment which have more infomation then
. Due to this reasons our proposed operators result is more accurate than
operator and
operator. Furthermore to compare the proposed operators with
operator and
operator. The ranking of alternatives by using the
operator, is
and for
operator, is
. For both the operators the best alternative is same but the ranking order is different from our proposed operators. So our proposed operators result is more suitable then
operator and
operator. Now the last comparison is between our proposed operators and
operators,
operator. For
operator, the ranking of alternatives is
. For
operator, the ranking of alternatives is
In this ranking to find the score values of the alternatives
and
we use accuracy function. For both the operators the best alternative is different from each other. Also the ranking order of
operator and
operator is different from our developed operators. Due to this reason our proposed operators result is more suitable than
operator and
operator.
Table 6. Ranking of alternatives of the proposed method and existing intuitionistic fuzzy approaches.
Conclusion
CDHFS is a useful tool for dealing with complex two-phase information. It is made up of complex hesitant fuzzy membership degrees and complex hesitant fuzzy non-membership degrees. Also, to deal with the relationship between prioritization and criteria, the notion of prioritized operators plays a key role in aggregating the evaluation values. Because no such operators for aggregating CDHF information are defined in the literature, in this paper, we propose some complex dual hesitant fuzzy prioritized operators: the CDHFPA operator, the CDHFPG operator, the CDHFEPA operator, and the CDHFEPG operator. Some desirable properties of the proposed operators are also investigated. Further, an MCDM method is developed based on the proposed operators. Also, a numerical example of a hotel selection problem is given to show how the developed method can be used and how well it works. Finally, we compared the proposed method with the existing method. The comparison study showed that the new method is more reliable than the old one. The main benefit of the operators made in this paper is that the criteria are ranked in order of importance, and the information from the CDHF can be put together. We will extend the proposed method to complex Pythagorean fuzzy sets, complex q-rung orthopair fuzzy sets, and complex interval-valued Pythagorean fuzzy sets, moreover, integrating the proposed method with recent improved MCDM models (Moslem Citation2024; Kakati et al. Citation2024).
Disclosure statement
No potential conflict of interest was reported by the author(s).
Additional information
Funding
References
- Ali, Z., and T. Mahmood. 2020a. Complex neutrosophic generalised dice similarity measures and their application to decision making. CAAI Transactions on Intelligence Technology 5 (2):78–39. doi:10.1049/trit.2019.0084.
- Ali, Z., and T. Mahmood. 2020b. Maclaurin symmetric mean operators and their applications in the environment of complex q-rung orthopair fuzzy sets. Computational and Applied Mathematics 39 (3):1–27. doi:10.1007/s40314-020-01145-3.
- Ali, Z., T. Mahmood, K. Ullah, and Q. Khan. 2021. Einstein geometric aggregation operators using a novel complex interval-valued pythagorean fuzzy setting with application in green supplier chain management. Reports in Mechanical Engineering 2 (1):105–134. doi:10.31181/rme2001020105t.
- Alkouri, A. M. D. J. S., and A. R. Salleh, 2012. Complex intuitionistic fuzzy sets. In AIP conference proceedings, September, American Institute of Physics, 1482, 464–70. 10.1063/1.4757515.
- Atanassov, K. T. 1986. Intuitionistic fuzzy set. Fuzzy Sets and Systems 20 (1):87–96. doi:10.1016/S0165-0114(86)80034-3.
- Beg, I., and T. Rashid. 2014. Multi-criteria trapezoidal valued intuitionistic fuzzy decision making with Choquet integral based TOPSIS. Opsearch 51 (1):98–129. doi:10.1007/s12597-013-0134-5.
- Biswas, A., and A. Sarkar. 2018. Development of dual hesitant fuzzy prioritized operators based on Einstein operations with their application to multi-criteria group decision making. Archives of Control Sciences 28 (4):527–49.
- Boran, F. E., S. Genç, M. Kurt, and D. Akay. 2009. A multi-criteria intuitionistic fuzzy group decision making for supplier selection with TOPSIS method. Expert Systems with Applications 36 (8):11363–11368. doi:10.1016/j.eswa.2009.03.039.
- Das, A. K., and C. Granados. 2022. FP-intuitionistic multi fuzzy N-soft set and its induced FP-Hesitant N soft set in decision-making. Decision making. Decision Making: Applications in Management and Engineering 5 (1):67–89. doi:10.31181/dmame181221045d.
- De, S. K., R. Biswas, and A. R. Roy. 2001. An application of intuitionistic fuzzy sets in medical diagnosis. Fuzzy Sets and Systems 117 (2):209–213. doi:10.1016/S0165-0114(98)00235-8.
- Garg, H., T. Mahmood, U. U. Rehman, and Z. Ali. 2021. CHFS: Complex hesitant fuzzy sets-their applications to decision making with different and innovative distance measures. CAAI Transactions on Intelligence Technology 6 (1):93–122. doi:10.1049/cit2.12016.
- Garg, H., and D. Rani. 2019. Some generalized complex intuitionistic fuzzy aggregation operators and their application to multicriteria decision-making process. Arabian Journal for Science and Engineering 44 (3):2679–2698. doi:10.1007/s13369-018-3413-x.
- Garg, H., and D. Rani. 2021. New prioritized aggregation operators with priority degrees among priority orders for complex intuitionistic fuzzy information. Journal of Ambient Intelligence and Humanized Computing 14 (3):1373–99. doi:10.1007/s12652-021-03164-2.
- Kakati, P., T. Senapati, S. Moslem, and F. Pilla. 2024. Fermatean fuzzy Archimedean Heronian Mean-Based Model for estimating sustainable urban transport solutions. Engineering Applications of Artificial Intelligence 127:107349. doi:10.1016/j.engappai.2023.107349.
- Kefeng, L., and C. Bin, 2017, July. Intuitionistic fuzzy prioritized information aggregation operators based on einstein operations and their applications to MCDM. In 2017 13th International Conference on Natural Computation, Fuzzy Systems and Knowledge Discovery (ICNC-FSKD) (pp. 1479–84). IEEE. doi:10.1109/FSKD.2017.8392983.
- Klement, E. P., R. Mesiar, and E. Pap. 2004. Triangular norms. Position paper I: Basic analytical and algebraic properties. Fuzzy Sets and Systems 143 (1):5–26. doi:10.1016/j.fss.2003.06.007.
- Li, D. F. 2005. Multiattribute decision making models and methods using intuitionistic fuzzy sets. Journal of Computer and System Sciences 70 (1):73–85. doi:10.1016/j.jcss.2004.06.002.
- Limboo, B., and P. Dutta. 2022. A q-rung orthopair basic probability assignment and its application in medical diagnosis. Decision Making: Applications in Management and Engineering 5 (1):290–308. doi:10.31181/dmame191221060l.
- Liu, P., T. Mahmood, and Z. Ali. 2019. Complex q-rung orthopair fuzzy aggregation operators and their applications in multi-attribute group decision making. Information 11 (1):5. doi:10.3390/info11010005.
- Mahmood, T., K. Ullah, Q. Khan, and N. Jan. 2019. An approach toward decision-making and medical diagnosis problems using the concept of spherical fuzzy sets. Neural Computing and Applications 31 (11):7041–7053. doi:10.1007/s00521-018-3521-2.
- Mahmood, T., U. Ur Rehman, Z. Ali, R. Chinram, and C. Zhang. 2020. Jaccard and dice similarity measures based on novel complex dual hesitant fuzzy sets and their applications. Mathematical Problems in Engineering 2020:1–25. doi:10.1155/2020/5920432.
- Moslem, S. 2024. A novel parsimonious spherical fuzzy analytic hierarchy process for sustainable urban transport solutions. Engineering Applications of Artificial Intelligence 128:107447. doi:10.1016/j.engappai.2023.107447.
- Munir, M., H. Kalsoom, K. Ullah, T. Mahmood, and Y. M. Chu. 2020. T-spherical fuzzy Einstein hybrid aggregation operators and their applications in multi-attribute decision making problems. Symmetry 12 (3):365. doi:10.3390/sym12030365.
- Ramot, D., R. Milo, M. Friedman, and A. Kandel. 2002. Complex fuzzy sets. IEEE Transactions on Fuzzy Systems 10 (2):171–186. doi:10.1109/91.995119.
- Riaz, M., and H. A. Farid. 2022. Picture fuzzy aggregation approach with application to third-party logistic provider selection process. Reports in Mechanical Engineering 3 (1):318–27. doi:10.31181/rme20023062022r.
- Talafha, M., A. U. Alkouri, S. Alqaraleh, H. Zureigat, and A. Aljarrah. 2021. Complex hesitant fuzzy sets and its applications in multiple attributes decision-making problems. Journal of Intelligent & Fuzzy Systems 41 (6):7299–7327. doi:10.3233/JIFS-211156.
- Torra, V. 2010. Hesitant fuzzy sets. International Journal of Intelligent Systems 25 (6):529–539. doi:10.1002/int.20418.
- Torra, V., and Y. Narukawa, 2009. On hesitant fuzzy sets and decision. InThe 18th IEEE International Conference on Fuzzy Systems (pp. 1378–82). Jeju Island, Korea. doi:10.1109/FUZZY.2009.5276884.
- Ullah, K., T. Mahmood, and H. Garg. 2020. Evaluation of the performance of search and rescue robots using T-spherical fuzzy Hamacher aggregation operators. International Journal of Fuzzy Systems 22 (2):570–582. doi:10.1007/s40815-020-00803-2.
- Wei, G. 2012. Hesitant fuzzy prioritized operators and their application to multiple attribute decision making. Knowledge-Based Systems 31:176–182. doi:10.1016/j.knosys.2012.03.011.
- Xiao, F., and W. Ding. 2019. Divergence measure of Pythagorean fuzzy sets and its application in medical diagnosis. Applied Soft Computing 79:254–267. doi:10.1016/j.asoc.2019.03.043.
- Xia, M., and Z. Xu. 2011. Hesitant fuzzy information aggregation in decision making. International Journal of Approximate Reasoning 52 (3):395–407. doi:10.1016/j.ijar.2010.09.002.
- Xu, Z. 2007. Intuitionistic fuzzy aggregation operators. IEEE Transactions on Fuzzy Systems 15 (6):1179–1187. doi:10.1109/TFUZZ.2006.890678.
- Yager, R. R. 1977. Multiple objective decision-making using fuzzy sets. International Journal of Man-Machine Studies 9 (4):375–382. doi:10.1016/S0020-7373(77)80008-4.
- Yu, D. 2013. Intuitionistic fuzzy prioritized operators and their application in multi-criteria group decision making. Technological and Economic Development of Economy 19 (1):1–21. doi:10.3846/20294913.2012.762951.
- Yu, D. 2014. Some hesitant fuzzy information aggregation operators based on Einstein operational laws. International Journal of Intelligent Systems 29 (4):320–340. doi:10.1002/int.21636.
- Yu, Q., F. Hou, Y. Zhai, and Y. Du. 2016. Some hesitant fuzzy Einstein aggregation operators and their application to multiple attribute group decision making. International Journal of Intelligent Systems 31 (7):722–746. doi:10.1002/int.21803.
- Zadeh, L. A. 1965. Fuzzy sets. Information & Control 8 (3):338–353. doi:10.1016/S0019-9958(65)90241-X.
- Zhao, N., and Z. Xu. 2018. Prioritized dual hesitant fuzzy aggregation operators based on t-norms and t-conorms with their applications in decision making. Informatica 29 (3):581–607. doi:10.15388/Informatica.2018.183.
- Zhao, H., Z. Xu, and S. Liu. 2017. Dual hesitant fuzzy information aggregation with Einstein t-conorm and t-norm. Journal of Systems Science and Systems Engineering 26 (2):240–264. doi:10.1007/s11518-015-5289-6.
- Zhou, X., and Q. Li. 2014. Generalized hesitant fuzzy prioritized Einstein aggregation operators and their application in group decision making. International Journal of Fuzzy Systems 16 (3):303–316.
- Zhu, B., Z. Xu, and M. Xia. 2012. Dual hesitant fuzzy sets. Journal of Applied Mathematics 2012:1–13. Article ID 879629. doi:10.1155/2012/879629.