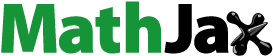
ABSTRACT
A reliable means of assessing the accuracy of a creep model’s predictions is fundamental to safe power plant operation. This paper introduces a method of decomposing the mean absolute prediction error for such a purpose to overcome the limitations that are inherent in the traditional approach of squaring prediction errors to prevent over and underestimates of life offsetting each other. When this method is applied to 2.25Cr-1Mo steel and 316 H stainless steel, it was found that squared errors leads to overestimates of the average prediction error associated with a particular creep model, and it also dramatically underestimates the proportion of this error that is systematic in nature. These differences were more noticeable for 316 H stainless steel.
Introduction
It is important to be able to predict the creep life and other creep properties of materials used in power plants and aero engines. When this can be done with a high degree of confidence, the results can potentially be used to justify the continued use of ageing power plants beyond their original design lives – as a short-term solution to potential energy gaps, for example – or increase operating temperatures to raise efficiency levels. 2.25Cr-1Mo is a main stay steel used for structural components operating at high temperature within such ageing power plants – where the usual service conditions for heater tubes are around 840 K and 35 MPa. 316H stainless steel is used when higher operating temperatures are required. Yet it has proved very challenging to predict the service life of these materials at such conditions using just the results from accelerated tests (tests done at much higher stresses and temperatures).
Consequently, one of the aims of the European Creep Collaborative Committee (ECCC), established in 1992, was to develop techniques for assessing creep data in Europe. In particular, the Technical Working Group (WG1) of the ECCC developed methods and guidelines for the assessment of creep data [1b – e]. The first creep data assessment exercise undertaken by this working group examined a single-cast of 2.25Cr-1Mo steel [Citation1,Citation2]. This exercise involved the application of 16 creep models by nine analysts and this resulted in the development of the Z parameter (among other statistics) to provide a measure of how well a creep model performs in predicting creep properties such as strain, time to failure and time to various strains. To construct this Z parameter, Holdsworth et al. [Citation2] first defined the residual log time as
where Ai = ln is the natural log of the time at which the ith test specimen fails (of which there are n such failure times in a data set) and
is a creep model’s prediction of Ai. Note that in what follows Ai could equally stand for any other creep property such as the minimum creep rate, time to various strains or even strain itself. The reason for working with natural logs is that this residual log time is then approximately equal to the percentage difference between the actual time to failure and a model’s prediction of it. This approximation is very good for percentages of around 30% or less (the smaller the better). Consequently, in this paper ei will be referred to as the percentage prediction error. This percentage scaling enables comparisons to be made of different creep properties which have different units of measurement. Next, the authors compute the standard deviation in residual log time (labelled SA-RLT by Holdsworth et. al.)
where is the mean percentage error in predicting the log failure time associated with all tested specimens. Note the denominator in Eq. (1b) is n, rather than the more usual n-1, and so if applied to a small sample of data would produce a biased estimate of the true or population standard deviation. The Z parameter is then defined as
Ideally, for single-cast assessments, Z should be less than or equal to 2, whereas Z ≥ 4 is unacceptable [Citation1,Citation2]. This was determined by looking at the Z values associated with the best performing creep models. For multi-cast assessments, even the best performing creep models studied by Holdsworth et al. [Citation2] could not achieve Z values below 6–7. These values can therefore be used for benchmarking purposes. If a creep model is assumed to predict the median time to failure at a given test condition, and that failure times at a given test condition follow a normal distribution, and if se is also independent of test conditions, then 99% of all failure times will be within the range
no matter what the test condition is. Thus, a Z value of 2 means the predictive accuracy of the creep model is such that there is only a 0.5% chance that failure times will be more than two times the model’s prediction and there is only a 0.5% chance of failure times being lower than half the model’s prediction. This is the recommended level of predictive accuracy according to Holdsworth under these assumptions (for single batches of material). However, this simple rule can be misleading as it is possible for a creep model to produce a Z value of 2 or less but produce predictions that are on average incorrect – i.e. produces systematic and biased percentage prediction errors, rather than random percentage prediction errors.
Therefore, an approach taken by Evans [Citation3] was to assess creep model adequacy using the approximate mean percentage squared error (MPSE)
The advantage of this approach is that the MPSE can be decomposed along the lines suggested by Granger and Newbold [Citation4] into a part that is systematic and another part that is random in nature – so overcoming the above problem with using Z. However, issues remain. First, an absolute value-based measure such as the mean percentage absolute error (MPAE) is much more interpretable. Whilst taking the square root of the MPSE (to give the RMPSE) helps with interpretation by converting the MPSE into the same units as e (i.e. to an average percentage error), this can still give very misleading assessments of a creep models adequacy. This is because the percentage prediction errors are squared and this makes the MPSE, and thus the RMPSE, very sensitive to the presence of a few outliers or poor predictions. Thus, there is the potential for the RMPSE to underestimate the predictive performance of a given creep model. This is not true of absolute percentage errors which are more robust to such outliers.
The aim of this paper is therefore to assess whether the use of the MPAE (and its decomposition into random and systematic error components) along with Z gives a better and more meaningful measure of creep model adequacy compared to using RMPSE and Z in predicting failure times using data on 2.25Cr-1Mo steel and 316 H stainless steel as test beds. To this end the paper is structured as follows. The next section summarises the data sets on these two steels. There then follows a section showing how to decompose the MPSE and the MPAE. In the results section these statistics are calculated using a parametric creep model to illustrate the misleading conclusions that can be drawn from the RMPSE. The paper concludes with recommendations for future work.
The data
This paper makes use of the information in Creep Data Sheets 3B, 50A and 14B, published by the Japanese National Institute for Materials Science (NIMS) [Citation5–7]. These have extensive data on 12 batches of 2.25Cr-1Mo (according to ASTM A 387, Grade 22) steel where each batch has a different chemical composition that underwent one of four different heat treatments – details of which are given in [Citation5]. This paper makes use of just one of these batches, the MAF batch, which was in tube form that had an outside diameter of 50.8 mm, a wall thickness of 8 mm and a length of 5000 mm with a chemical composition of: Fe − 2.46 Cr − 0.94 Mo − 0.1 C – 0.23 Si − 0.43 Mn − 0.011 P – 0.009 S – 0.008 Ni − 0.07 Cu − 0.005 Al. Specimens for creep testing were taken longitudinally from this material. Each test specimen had a diameter of 6 mm with a gauge length of 30 mm. The creep tests were obtained over a wide range of conditions: 400 MPa − 22 MPa and 723 K − 923 K. For the MAF batch (and only this batch) both minimum creep rates and time to failure measurements were recorded, together with the times to attain various strains −0.005, 0.01, 0.02 and 0.05. plots the creep failure times obtained for this MAF batch at the different stresses and temperatures used. The relationship between time to failure and test conditions is quite complicated for this batch – which has made it very difficult to model and predict such failure times using parametric creep models. In particular, the slope of a visually drawn curve changes dramatically at different test temperatures, so that some of the isothermal lines have more than one inflection point.
Figure 1. Relationship between stress, temperature, and time to failure for the MAF batch of 2.25Cr-1Mo steel contained within NIMS creep data sheets 3B &50A [Citation5,Citation6].
![Figure 1. Relationship between stress, temperature, and time to failure for the MAF batch of 2.25Cr-1Mo steel contained within NIMS creep data sheets 3B &50A [Citation5,Citation6].](/cms/asset/bc77193e-5d9c-47f4-8cf9-9236145bbd4a/ymht_a_2268332_f0001_b.gif)
The second set of data used in this paper is on type 316 H stainless steel (18Cr-12Ni-Mo with up to 0.08% C). This data set produced by NIMS [Citation7] reported failure times for two batches of plate at 873 K through to 1123 K and are shown in . These batches were labelled by NIMS as AaA to AaB. These plate specimens were hot rolled and held at 1323 K for either 40 mins or 80 mins before water quenching. More details of the chemical composition and heat treatments for these two batches are contained in Reference [Citation7].
Figure 2. Relationship between stress, temperature, and time to failure for 316H stainless steel plate contained within NIMS creep data sheets 14B [Citation7].
![Figure 2. Relationship between stress, temperature, and time to failure for 316H stainless steel plate contained within NIMS creep data sheets 14B [Citation7].](/cms/asset/6b56fbb6-54cc-469e-8ce5-c06c5dd0bc3c/ymht_a_2268332_f0002_b.gif)
Evaluation statistics
Limitations of the Z parameter
As mentioned in the introduction section, Z should be less than or equal to 2 for an acceptable level of predictive accuracy to have been achieved for a single batch of material. However, this simple rule can be misleading as it is possible for a creep model to produce a Z value of 2 or less but produce predictions that are on average incorrect – i.e. produce systematic and biased percentage prediction errors, rather than random percentage prediction errors. This can be seen by applying the rules of expected values through EquationEquation (1a)(1a)
(1a) to give
where E stands for the expected value. An expected value is simply the population mean. There is no reason to suppose a creep model will produce values for that yield an average or expected value for e that is zero. To see the conditions under which a creep model will produce E[e] = 0, i.e. will not produce systematic errors when Z ≤ 2, rewrite EquationEquation (1a)
(1a)
(1a) as
where εi is a random disturbance term. If α and β are estimated using linear least squares, then
where
and where is the sample average of all the log failure time predictions and
is the sample average of all the log failure times. The hat above α and β denotes that EquationEquation (3c)
(3c)
(3c) gives a sample estimate of the population values for these parameters.
Then if = 0 and
= 1,
, and so
must equal ei for EquationEquations (1a)
(1a)
(1a) and (Equation3b
(3b)
(3b) ) to be consistent with each other. Further, by least squares construction (i.e. given EquationEquations (3c
(3c)
(3c) -Equation3d
(3d)
(3d) )), the average value for εi will be zero and so E[ε] = 0 = E[e]. So only when α = 0 and β = 1 will a creep model produce predictions that are on average equal to the actual values and so have no systematic errors. Ideally then, an acceptable creep model should have Z ≤ 2 and also α = 0and β = 1.
The MPSE and its decomposition
Evans [Citation3] has decomposed the percentage prediction error e along the lines suggested by Granger and Newbold [Citation4]
The first bracketed term on the right-hand side in EquationEquation (4a)(4a)
(4a) is that part of the MPSE that is due to the average percentage prediction error and so is often termed the bias error. This is a systematic error present in the predictions as a positive value implies that the creep model predicts incorrectly on the average. This follows from EquationEquations 2
(2)
(2) that implies
. The second bracketed term on the right-hand side in EquationEquation (4a)
(4a)
(4a) is due to the regression coefficient
differing from one, or the regression error for short. This follows from the fact that
). This is also a type of systematic error that is present in the predictions, because β < 1 indicates that the creep model systematically underestimates the failure time below
and overestimates above
(the opposite is true when β > 1). For this reason, it is also referred to as the proportionality error. The last term on the right-hand side of EquationEquation (4a)
(4a)
(4a) is due to random prediction errors because
=
and ε is pure random disturbance term. That is, the last term is just the variance in the random disturbance term (given the mean for ε is by construction zero). Dividing EquationEquation (4a)
(4a)
(4a) throughout by the MPSE gives
Consequently, UM+UR equals the proportion of the MPSE that is systematic in nature and UD is the proportion of the MPSE that is random in nature. An acceptable creep model therefore needs to have Z ≤ 2, with UD making up most of the mean squared percentage error.
Note that in this decomposition, the percentage errors are squared in the construction of the MPSE. Whilst this has the advantage of being easily modified into a test of statistical significance for any differences between competing creep model predictions using well-known distributions, it does create two major problems. The first is that an absolute-value-based measure such as the mean percentage absolute error (MPAE) is much more interpretable. Whilst taking the square root of the MPSE (or RMPSE) helps with interpretation by converting the MPSE into the same units as e, this can still give very misleading assessments of a creep model’s adequacy. This is because the percentage prediction errors are squared, and this makes the MPSE very sensitive to the presence of a few outliers or poor predictions. Thus, there is the potential for the MPSE to underestimate the predictive performance of a given creep model. This is not true of absolute percentage errors which are more robust to such outliers.
The MPAE and its decomposition
This paper therefore proposes assessing a creep model’s performance using Z and a decomposition of the MPAE into systematic and random components along the lines proposed by Robeson and Cort [Citation8]. The MPAE is formally defined as
Robeson and Cort have recently put forward a method for decomposing this MPAE based on the same three components as those proposed by Granger and Newbold (although they use slightly different terminology). To demonstrate this decomposition, consider the hypothetical data shown in , where the black solid line is the regression line through the raw data (shown by the black filled circles). For these data, the MPSE = 5 and the RMPSE = 2.24. However, the MPAE = 2 and so the RMPSE tends to downplay the accuracy of the predictions, even when there are no obvious outliers as is the case for these hypothetical data.
Unlike the decomposition of the MPSE, if a prediction has a zero error associated with it, that data point will not contribute to the MPAE, which is a strong advantage over the MPSE. Because of this, it is also possible to decompose the absolute error for each data point individually and then add them up to get the decomposition of the MPAE. This is illustrated in for the first data point corresponding to an actual value of −2. The absolute prediction error associated with this data point is |e1| = |-2 - −4| = 2 and is given by the vertical length of the longest arrowed line. This is then decomposed into three separate parts. The first component of |ei| is the absolute bias error = 1. Removing this component by adding it onto the prediction of −4 takes us to the open circled data point alongside, Pu1 = P1 + BE = −4 + 1 = −3. The dashed line is the regression line through the open circled data where the other predictions have had the bias error removed in the same way. The remaining absolute prediction error is then equal to the vertical distance from the open circled data point to the 45° line and equals a value of 1 (0.62 + 0.38). This vertical distance is made up of two components. The first component is random in nature and is made up of the vertical distance between the open circled data point and the dashed regression line, v1 = 0.62. The second part is a systematic error and is made of the vertical distance between the dashed regression line and the 45° line, f1 = 0.38. This vertical distance only exists because the slope of the dashed regression line is different from 1, i.e. the dashed regression line does not correspond to the 45° line. It is thus analogous to UR above. Robeson and Willmott call this component the proportionality error. Thus, adding up the absolute value for the bias error, the random error and the proportionality error gives the absolute error for this data point, |e1| = |BE|+|f1|+|v1| = 1 + 0.38 + 0.62 = 2. For this data point, |BE| make up 50% of |ei|, the proportionality error a further 31% and the random error a further 19%.
This equivalence only works for data points above the dashed regression line and when the dashed regression line lies above the 45° line. For example, take the one but last data point corresponding to (Ai,Pi) = [Citation1,Citation4] which does not meet this condition. Here |ei| = 3, with |fi| = 0.127 and |vi| = 2.127 and so |BE|+|fi|+|vi| = 3.25 (which exceeds |ei|). The decomposition still makes sense, however, because fi = −0.127 and so |BE|+fi+|vi| = |ei| = 3. So, the equivalence in absolute terms breaks down sometimes, but negative values cannot be allowed to offset positive values in the calculation of the average error. The solution is to divide each component by |BE|+|fi|+|vi|, and then say this ratio represents the proportion of |ei| attributable to that component. Thus, for this data point the bias equals |ei||BE|/|BE|+|fi|+|vi| = 3 [Citation9]/3.25 = 0.92. The proportionality error equals |ei||fi|/|BE|+|fi|+|vi| = 3(0.127)/3.25 = 0.12 and the random error, |ei||vi|/|BE|+|fi|+|vi| = 3(2.127)/3.25 = 1.96. These now add up to 3, i.e. to the absolute error. On this basis, the decomposition formulas are
where MPAEM is the part of MPAE due to the bias – the bias error. MPAER is the part of MPAE due to the regression line – the proportionality error. MPAED is the part of MPAE due to the random error. The first two sum to the systematic error. One note of caution when using EquationEquations (5(2)
(2) ,Equation6
(3a)
(3a) ) is that the denominator can very rarely equal zero. This can only occur when a creep model has no bias and the regression line passes through a predicted value that has no error (i.e. when BE = 0 and Pi = Ai =
, where
is the value given by the regression line for Ai). This is likely to be a rare event, but in the context of creep failure time data, it is almost impossible for a model’s predictions to exactly equal the recorded failure times – that are usually quoted to one decimal place. So, this issue can be avoided by ensuring the model predictions are always expressed to more significant figures than the actual data. These equations of course give the same answer as straight addition when data points are above the dashed regression line and when the dashed regression line lies above the 45° line. So going back to the first data point
which is the same as the result derived above. Like with the squared decomposition, these can be expressed as proportions of MPAE
So whilst the RMPSE was only slightly inflated relative to the MPAE, when it comes to decompositions a big difference is observed using this hypothetical data set. The decomposition of the MPSE suggests the model produces prediction errors that are almost 80% random in nature. This seems at odds with the adjusted trend line (shown as the dashed line) that differs from the 45° line. This is better reflected in the decomposition of the MPAE that suggests the proportion of the model’s prediction errors that is random in nature is much lower at 58%. Thus, the decomposition based on squaring prediction errors can lead to various misleading conclusions about the systematic/random nature of the model’s errors in prediction. Hence, the advice is to use the MPAE, its decomposition and Z to decide which of a range of competing creep models produces the best predicted failure times.
Modelling time to failure
A selected creep model
The Orr-Sherby-Dorn (OSD) [Citation10] parametric creep model is given by
where T is the absolute temperature, R the universal gas constant, σ is stress and tF time to failure. Qc is the activation energy for creep, and B and n are further model parameters. The model implies that there is a linear relationship between log failure time and log stress at a given temperature, and the role of temperature is then to shift this linear relationship in a parallel fashion.
When it comes to applying this model to different materials, some modifications of this equation are often required. A study of 316 H by Whittaker et al. [Citation11] revealed changes in creep mechanism with respect to stress. They found that dislocation processes are rate controlling, with no transition to diffusional mechanisms in low stress tests. However, they found that a change in the dominant dislocation process occurs when σ falls from above to below the yield stress. Thus, when stress exceeded the yield stress, these authors stated that creep is controlled by the movement of dislocations newly generated during the plastic component of the initial strain, whereas the creep rate is determined only by grain boundary zone deformation when the stress falls below the yield stress – because no new dislocations are created during the elastic initial loading strain. It is to be expected that the activation energy for creep would be lower between grain boundaries compared to within the grains themselves. This suggested that EquationEquation (8)(8)
(8) requires modifying to
where σy is the yield stress, with the expectation being that <
and where n0 ≠ n1 and Bo ≠ B1.
A study of the MAF batch of 2.25Cr1-1Mo by Brear [Citation12] observed that for this material the activation energy for creep was constant with respect to test conditions, and that below some critical stress (σ*) the resulting prolonged length of the creep tests lead to high amounts of oxidised material on the failed creep specimens that considerably weakened the creep strength of this material. This suggests that EquationEquation (8)(8)
(8) requires modifying to
and where again n0 ≠ n1 and Bo ≠ B1.
Parameter estimation
As the yield stress is not published by NIMS, it must be estimated from EquationEquations (9)(9a)
(9a) and this can be achieved using a dummy variable D1
where D1 equals zero when σ > σy and unity otherwise. Thus when σ > σy, D1 = 0 and EquationEquation (11a)(11a)
(11a) collapsed to EquationEquation (9a)
(9a)
(9a) and when σ <σy, D1 = 1 and EquationEquation (11a)
(11a)
(11a) collapsed to EquationEquation (9b)
(9b)
(9b) with B1 = B0 + λ1, n1 = n0 +λ2 and Qc,1 = Qc,o + λ3.
Similarly, σ* can be estimated using
with B1 = B0 + λ1, and n1 = n0 +λ2
To estimate values for all the parameters in EquationEquations (11)(11a)
(11a) , the following procedure can be used. First σy or σ* is set equal to some starting value allowing D1 to be quantified. Then all variables on the right-hand side of EquationEquations (11)
(11a)
(11a) are fully defined so that ln(tF) can be regressed on these right-hand side variables to obtain least squares estimates for B0, n0, λ1 to λ3 and Qc,0. These regression equations have a residual sum of squares (RSS) associated with them – that of course is minimised by the linear least squares procedure. Then a generalised reduced gradient non-linear search technique that uses centralised numerical derivatives is used to search for values of σy and/or σ* that minimise the RSS associated with the regression lines given by EquationEquations (11)
(11a)
(11a) . This non-linear search is carried out using Excel’s Solver [Citation13] subroutine.
Results
2.25Cr-1Mo steel
The results of estimating EquationEquation (11b)(11b)
(11b) are shown in . The model’s predictions are shown by the solid segmented line, and the fit to the short-term data (failure times less than 10,000 h) is good – with an R2 value of 99.57%. The model yields an activation energy of 382 kJmol−1, which is again quite close to that for lattice self-diffusion in this material. A break in the relationship seen in appears to occur at a stress of 62 MPa and the stress exponent n is much higher in the high-stress range (~-8.13), compared to ~ −2.22 in the low-stress regime. Brear [Citation12] suggested that this difference may be due to the high amounts of oxidised material seen on the failed creep specimens at the very lowest stresses – the result of prolonged testing. Such oxidation would make the life of the material much more sensitive to a change in stress, such that a heavily oxidised specimen would not see such a big increase in life at a lower stress compared to a non-oxidised specimen.
Figure 4. Showing (a) the OSD representation of failure times for 2.25Cr-1Mo steel, where the model is estimated using tF < 10,000 h and, (b) actual v predicted tF values beyond 10,000 h.

The extrapolative performance is summarised in and in the first half of . The best fit line deviates only marginally from the ideal 1:1 line – the power term on predicted tF is below unity (~0.86). The Z parameter equals 2.72, so that there is 99% certainty that the actual failure time at any test condition will be within 1/2.72 (= 0.37) and 2.72 times the models predicted time. So, whilst the dashed lines in are inside the solid lines, the model meets the intent of the ECCC recommendations.
Table 1. Extrapolative performance of the OSD models over all temperatures for each steel.
Table 2. Extrapolative performance of the OSD models broken down by temperature.
The results in correspond to those in the first half of . Given the trend line has an exponent below 1 we would expect |UR| to be quite large, and reveals that 17.27% of the MPAE is due to the best fit line in being slightly flatter than the 1:1 line. As the absolute bias error = 0.056 we would expect |UM| to be relatively small, and the first half of reveals that 11.65% of the MPAE is due to the average predicted and average actual failure times being different. Thus, over all temperatures, this OSD model has a systematic prediction error of nearly 29% that is in the form of under predictions at low failure times and over predictions at higher failure times – which means the model produces slightly conservative life estimates closer to the operating conditions for this material. Consequently, most of the prediction errors made by this model are random in nature – some 71.08%. The rest of the first half of shows the distortion in these conclusions that stem from using squared percentage prediction errors (rather than absolute percentage errors). The RMPSE of 38.48% overestimates the inaccuracy of the model’s predictions as its nearly 6 percentage points above the average absolute error. More misleading is the decomposition of the MPSE. This decomposition suggested that nearly 89% of the percentage prediction errors are random in nature, which is a substantial overestimate compared to the 71% value for |UD|.
The above comments relate to the model’s performance over all stresses and temperatures that induce a failure time beyond 10,000 h. The first half of breaks this analysis down by temperature. The MPAE is smallest at 748 K (13.13%) and largest at 723 K (52.33%). On average the model overestimates at 773 K and 873 K but on average underestimates at the other temperatures (as revealed by the sign on BE). The RMPSE is slightly pessimistic about the model’s extrapolative capability at all temperatures (as shown by RMPSE > MPAE and especially so at the highest two temperatures). Apart from at 748 K, the random component of the model’s absolute prediction errors is always about 65% or higher. However, at 748 K the model has a large systematic prediction error component, but thankfully the MPAE is the smallest of all the temperatures at 13.13%. Again, the decomposition of the MPSE is highly misleading. It suggests that the random component of the squared prediction errors is between 6% and 7%. But the values for |UD| are between 65% and 77% and so UM and UR vastly overestimate the size of the systematic error made by this model. For the lowest two temperatures, the value for UD cannot be quantified due to too few data points (which in turn leads to the over estimation of the systematic error using squared prediction errors).
316 H stainless steel
The results of estimating EquationEquation (11a)(11a)
(11a) are shown in . The model’s predictions are shown by the solid segmented line, and the fit to the short-term data (failure times less than 10,000 h) is good – with an R2 value of 99.98%. The estimated value for the yield strength σY is 142 MPa. Above this yield strength, the model yields an activation energy of 549 kJmol−1, whilst below this stress the activation energy is much lower at 376 kJmol−1. Whilst it is expected that the activation energy would be lower below the yield stress, the values are a lot higher than those quoted by Wilshire et al. [Citation11]. – probably the result of not using the Wilshire model that normalises the stress using the high temperature tensile strengths. The stress exponent n is much higher above the estimated yield stress (~ −12.29), compared to ~ −8.29 below this stress.
Figure 5. Showing (a) the OSD representation of failure times for 316H stainless steel, where the model is estimated using tF < 10,000 h and, (b) actual v predicted tF values beyond 10,000 h.

The extrapolative performance is summarised in and in the second half of . The best fit line deviates from the ideal 1:1 line – the power term on predicted tF is below unity (~0.77). The Z parameter equals 2.74, so that there is 99% certainty that the actual failure time at any test condition will be within 1/2.74 (= 0.36) and 2.74 times the model’s predicted time. So, whilst the dashed lines in are inside the solid lines, the model meets the intent of the ECCC recommendations.
The results in correspond to those in the second half of . Given the trend line has an exponent below 1 we would expect |UR| to be quite large, and reveals that 43.14% of the MPAE is due to the best fit line in being flatter than the 1:1 line. As the absolute bias error = 0.006 is very small, we would expect |UM| to be small as well, and the second half of reveals that 1.43 of the MPAE is due to the average predicted and average actual failure times being different. Thus, over all temperatures, this OSD model has a systematic prediction error of nearly 45% that is in the form of under predictions at low failure times and over predictions at higher failure times – which means the model produces slightly conservative life estimates closer to the operating conditions for this material. Consequently, just over half of the prediction errors made by this model are random in nature – some 55.43%. The rest of the second half of shows the distortion in these conclusions that stem from using squared prediction errors (rather than absolute errors). The RMPSE of 38.3% overestimates the inaccuracy of the model’s predictions as its nearly 7 percentage points above the average absolute error. More misleading is the decomposition of the MPSE. This decomposition suggested that nearly 71% of the prediction errors are random in nature, which is a substantial overestimate compared to the 55.43% value for |UD|.
The above comments relate to the model’s performance over all stresses and temperatures that induce a failure time beyond 10,000 h. The second half of breaks this analysis down by temperature. The MPAE is smallest at 923 K (19.00%) and largest at 898 K (44.32%). On average the model underestimates at all temperatures (as revealed by the sign on BE). The RMPSE is slightly pessimistic about the model’s extrapolative capability at all temperatures (as shown by RMPSE > MPAE and especially at the highest temperatures). Depending on the temperature, the random component of the model’s prediction errors varies between 25% and 75%. Again, the decomposition of the MPSE is highly misleading. For example, at 1023 K the random component of the squared prediction errors is 84.41%, but the value for |UD| is only 62.79%. So, UM and UR vastly overestimate the size of the systematic error made by this model. For the lower temperatures and the highest temperature, the values for UR and UD cannot be quantified due to too few data points (which in turn leads to the over estimation of the systematic error using squared prediction errors).
Material comparison
Whilst the OSD model produced very similar values for Z and the MPAE over all temperatures when applied to each material, the model clearly works better when applied to 2.25Cr-1Mo – because it has a much higher |UD| value. For 2.25Cr-1Mo steel, some 71.08% of the MPAE is random in nature compared to just 55.43% for 316 H steel. Irrespective of the temperature, the value for |UM| is between 9% and 15% for 2.25Cr-1Mo steel (except at 748 K where it is 33%). But for 316 H, |UM| never exceed 3% no matter what the temperature is, and so for this material the difference between the average failure time and the average prediction is much smaller. But for 316 H, a much bigger proportion of the MPAE is due to |UR| and so for this material there is a stronger tendency to underpredict with increasing failure times. These trends are seen in that show the predictions made by the OSD model in the more familiar stress-time space.
Conclusions
This article has proposed that the assessment of a creep model's predictive capability should be based on absolute rather than squared errors to avoid distortions arising from the squaring of prediction errors associated with outlying data points. It also presented a way of decomposing the average absolute error into random and systematic components to further quantify a model’s performance – a procedure not used so far in creep model evaluation. When applied to 2.25Cr-1Mo and 316 H failure time data, it was found that the MPSE tended to underestimate the predictive capability of the OSD creep model. It also tended to dramatically overestimate the component of the prediction error that was random in nature in these two materials. Areas for future work include the application of these assessment statistics to other materials – especially data sets that have more heat-treated batches (so as to study the role of batch-to-batch variation) and to develop statistical tests to see whether one creep model has a better MPAE compared to another.
Disclosure statement
No potential conflict of interest was reported by the author.
References
- Holdsworth SR, Merckling G, ECCC developments in the assessment of creep-rupture data. In: Holdsworth SR, Orr J, Granacher J, et al., editors. Proceedings of sixth international Charles Parsons Conference on engineering issues in turbine machinery, power plant and renewables; Trinity College, 16–18 September,; Dublin; 2003.
- Holdsworth SR. Developments in the assessment of creep strain and ductility data. Mater High Temp. 2004;21(1):125–132. doi: 10.1179/mht.2004.004
- Evans M. Estimating threshold stresses using parametric equations for creep: application to low-alloy steels. Mater Sci Technol. 2023;1–16. doi: 10.1080/02670836.2023.2198395
- Granger C, Newbold P. Some comments on the evaluation of economic forecasts. Appl Econ. 1973;5(1):35–47. doi: 10.1080/00036847300000003
- NIMS Creep Data Sheet No. 3B. Data sheets on the elevated-temperature properties of 2.25Cr-1Mo steel for Boiler and heat exchanger seamless tubes (STBA 24). Tokyo, Japan: National Research Institute for Metals; 1986.
- NIMS Creep Data Sheet No.50A. Long-term creep Rupture data obtained after publishing the final edition of the creep data sheets. Tokyo, Japan: National Research Institute for Metals; 2015.
- NIMS Creep Data Sheet No.14B. Data sheets on the Elevated-temperature properties of 18Cr-12Ni-mo stainless steel Plates for reactor vessels (316HP). Tokyo, Japan: National Research Institute for Metals; 1988.
- Robeson SM, Cort JW. Decomposition of the mean absolute error (MAE) into systematic and unsystematic components. PLoS One. 2023;18(2):e0279774. doi: 10.1371/journal.pone.0279774
- ECCC Recommendations. Creep data validation and assessment procedures. In: Holdsworth SR, eds., ECCC. publ., (a) vol. 1: overview, (b) vol. 2: terms and terminology, (c) vol. 3: data acceptability criteria, data generation, (d) vol. 4: data exchange and collation, (e) vol. 5: data assessment, (f) vol. 6: characterisation of microstructure and physical damage for remaining life assessment, (g) vol. 7: data assessment—creep crack initiation, (h) vol. 8: data assessment—multi-axial, (i) vol. 9: component assessment.
- Dorn JE, Shepherd LA, What we need to know about creep. In Proceedings of the STP 165 Symposium on The Effect of Cyclical Heating and Stressing on Metals at Elevated Temperatures, Chicago, IL, USA, 17 June 1954.
- Whittaker MT, Evans M, Wilshire B B. Long-term creep data prediction for type 316H stainless steel. Mater Sci Eng A. 2016;552:145–150. doi: 10.1016/j.msea.2012.05.023
- Brear JM. A perspective on the Wilshire creep equations. Strength, Fract Complex. 2022;15(1):79–98. doi: 10.3233/SFC-228006
- Microsoft Corporation. Microsoft Excel [Internet]. 2018. Available from: https://office.microsoft.com/excel.