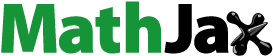
Abstract
Measurement invariance (MI) is required for validly comparing latent constructs measured by multiple ordinal self-report items. Non-invariances may occur when disregarding (group differences in) an acquiescence response style (ARS; an agreeing tendency regardless of item content). If non-invariance results solely from neglecting ARS, one should not worry about scale inequivalences but model the ARS instead. In a simulation study, we investigated the effect of ARS on MI testing, both when including ARS as a factor in the measurement model or not. For (semi-) balanced scales, disregarding a large ARS resulted in non-invariance already at the configural level. This was resolved by including an ARS factor for all groups. For unbalanced scales, disregarding ARS did not affect MI testing, and including an ARS factor often resulted in non-convergence. Implications and recommendations for applied research are discussed.
1. Introduction
Social and behavioral scientists are often interested in assessing whether groups of individuals differ regarding latent constructs (e.g., extraversion). These unobservable constructs are often measured by self-report scales. Commonly, these scales consist of questionnaire items, where, for each item, respondents rate their level of agreement by selecting one of a few ordered response options on a Likert scale (e.g., “disagree”, “neutral”, “agree”).
To validly draw conclusions about group differences on latent constructs, scales must function equivalently across groups. Measurement invariance (MI) testing evaluates the tenability of this hypothesis by assessing whether the measurement model (MM) of the psychological construct is equivalent across groups. As an example of an inequivalence, one may think of differences in item interpretations that may lead one group to systematically pick lower/higher response options for some items, which can result in under/overestimation of sum-scores (Jeong & Lee, Citation2019), item means (Jones & Gallo, Citation2002), and regression parameters in structural equation models (Guenole & Brown, Citation2014). Thus, testing for MI is an essential precursor to investigating group differences (Borsboom, Citation2006; Meredith & Teresi, Citation2006) to avoid building on latent construct differences that are purely due to measurement discrepancies and thus invalid or “biased”.
Measurement invariance is often tested with a latent variable approach, which models the relationship between unobserved psychological constructs (i.e., latent variables) and observable behaviors (i.e., items). Within the latent variable modeling framework, multiple group categorical confirmatory factor analysis (MG-CCFA) and multiple group item response theory (MG-IRT) are the most popular approaches to evaluate MI for models with ordinal data (i.e., items with too few response categories to treat them as continuous). Note that while equivalences can be drawn between MG-CCFA and MG-IRT models (Chang et al., Citation2017), some differences remain in the way MI is tested within each of these two approaches (D'Urso et al., Citation2022). For instance, MG-CCFA primarily focuses on assessing MI at the scale level (i.e., for the complete set of items measuring a construct), whereas MG-IRT traditionally tests MI for each item separately. In this paper, we focus on a MG-CCFA-based MI testing approach (i.e., scale level) since it is more commonly used in practice (Putnick & Bornstein, Citation2016). Specifically, in a MG-CCFA-based MI testing approach, different levels of MI are assessed in a step-wise procedure. For each step, increasingly restrictive models are estimated by imposing equality constraints on specific MM parameters. Then, the fit of the more constrained model to the data is compared to that of the less constrained one to evaluate whether the equality constraints worsen the model fit significantly, thus indicating non-invariance of (at least some of) the constrained MM parameters.
Apart from measurement model differences or “non-invariances” such as differential item interpretations, bias in latent variable comparisons may also arise when responses to self-report item rating scales are affected by response tendencies or response styles in some groups but not in others (Cheung & Rensvold, Citation2000). Acquiescence, or agreeing, response style (ARS) is a well-known one, which represents a tendency to agree with items regardless of their content (Paulhus, Citation1991). Interestingly, various studies have indicated that different groups of individuals may have a more or less pronounced ARS depending on their education (Meisenberg & Williams, Citation2008), age (Weijters et al., Citation2010), gender (Austin et al., Citation2006), length of employment (Johnson et al., Citation2005) or culture (Bachman & O'Malley, Citation1984; Marin et al., Citation1992). ARS can inflate observed means (Van Vaerenbergh & Thomas, Citation2013) and affect the measurement model by introducing an additional factor (Billiet & McClendon, Citation2000; D'Urso et al., Citation2023) or changing the strength of the relationships between items and factors (i.e., factor loadings; Ferrando & Lorenzo-Seva, Citation2010). To control for ARS, previous research indicated that including ARS as an additional factor in the MM (Billiet & McClendon, Citation2000) proved to effectively reduce bias in MM parameters recovery as well as estimated factor scores (Savalei & Falk, Citation2014).
Though it is confirmed that not taking ARS into account affects the MM in single group studies, extensive investigations about the impact of disregarding ARS on MI testing are currently lacking. Existing studies in the literature have either focused on assessing the effect of other RSs on MI, such as extreme response style (ERS; Liu et al., Citation2017) and non-effortful responding (NER; Arias et al., Citation2020; Rios, Citation2021), or on evaluating the impact of including an additional ARS factor when assessing MI using empirical data (Aichholzer, Citation2015; Welkenhuysen-Gybels et al., Citation2003). In case of empirical data, the” true” MM is unknown, however. Thus, in this paper, we thoroughly assess the effects of ARS on MI testing in a simulation study. Identifying in which conditions and to what extent ARS distorts measurement invariance conclusions may give us clues on correcting for bias in latent mean differences due to differential response tendencies across groups. For instance, when the influence of ARS is disregarded, researchers may wrongly conclude that there is non-invariance. Furthermore, this may introduce bias in the latent means of the groups and thus mislead conclusions about between-group differences in latent means. Taking ARS into account when testing for MI likely facilitates distinguishing non-invariance of the scale itself from (amendable) non-invariance due to disregarding ARS. Indeed, if non-invariance results from not taking ARS into account, one needs to correct for the ARS instead of worrying about the scale being inequivalent across groups. In addition to evaluating the effect of ARS on multigroup factor models rather than single-group ones, we expand the existing literature (e.g., Savalei & Falk, Citation2014) by (i) evaluating models for ordinal data, (ii) including multi-dimensional factor models (iii) for balanced, semi-balanced and unbalanced scales. The remainder of this paper proceeds as follows: In Section 2, we elaborate on MG-CCFA, MI testing, and how it may be affected by ARS. Then, in Section 3, we present a simulation study that evaluates the effect of ARS on MI both when (i) ARS is disregarded, and (ii) ARS is taken into account by including ARS as an additional factor in the MM. Finally, in Section 4, we discuss recommendations based on the simulation study results, limitations of our investigation, and potential future research directions.
2. Measurement Invariance Testing and the Potential Effects of ARS
In this section, we introduce MG-CCFA, describe the standard MI testing framework including the identification constraints proposed by Wu and Estabrook (Citation2016) to assess MI with ordinal data, and discuss the potential effects of ARS on MI testing.
2.1. Multiple Group Categorical Confirmatory Factor Analysis
Consider having data composed of J items for a group of N subjects, and that a grouping variable (e.g., nationality) exists to divide the N subjects into G groups. Then, let xj be the polytomously scored response on item j that can take on C possible values with c = {0,1,2,…,C-1}. MG-CCFA assumes that each of the C possible observed responses is obtained from a discretization of a continuous unobserved response variable through a set of threshold parameters
which indicate the cut-off point for the response categories (e.g., division between scoring a 1 or a 2) for group g. Note that the first and last thresholds are defined as
= −
and
= +
respectively. Then, formally
(1)
(1)
A factor-analytical model for a vector of latent response variables = (
…,
) is obtained as:
(2)
(2)
where
is a J-dimensional vector of latent intercepts (i.e., intercepts of the unobserved response variables in
),
is a
matrix of factor loadings,
is a Q-dimensional vector of scores on the Q factors,
is a J-dimensional vector of residuals. Note that the latent intercepts
thresholds
and loadings
in
are group specific, and that, within each group g, both the factors
and the item-specific residual components
are mutually independent and normally distributed, with:
(3)
(3)
where
are the group-specific factor means,
the group-specific factors variance-covariance matrix, and
is a diagonal matrix containing the group-specific unique variances of the items. Further, within each group, the model-implied mean vector
and covariance matrix
is obtained as:
(4)
(4)
2.2. Measurement Invariance Testing Procedure
In MG-CCFA, MI is commonly evaluated by testing, for all items, equality of a set of MM parameters (e.g., loadings) across groups in a step-wise fashion. The starting point is to identify the MG-CCFA model, which requires: (i) setting the scale for the latent variable η, (ii) setting the scale for the unobserved response variable and (iii) aligning the scale of the latent variable η across groups. Note that the latter is necessary to make the groups comparable. Then, in addition to the identification constraints, additional equivalence constraints are imposed on MM parameters (e.g., thresholds) in a step-wise fashion to evaluate their invariance. Therefore, a new, more constrained model is estimated for each step, and its fit to the data is evaluated to conclude whether these new constraints significantly worsen the fit. Below, we first discuss the main steps and identification constraints to test MI for ordinal data following the recommendations by Wu and Estabrook (Citation2016), summarized in , and then elaborate on standard goodness-of-fit criteria that are used to draw MI conclusions.
Table 1. Identification and MI constraints Wu and Estabrook (Citation2016) for MI testing with MG-CCFA.
2.2.1. Configural Invariance
Configural invariance is usually the first invariance level tested, where the goal is to test the equivalence of the number of factors and of the loadings pattern (i.e., which factors are measured by which items) across groups. In this step, following Wu and Estabrook (Citation2016), the baseline model is identified by fixing, for all groups, the latent intercepts to 0 and variances (i.e., diagonal elements of
) to 1, which is commonly known as the delta parameterization (Muthén & Muthén, Citation2009). Similarly, the latent factor means
and variances
(i.e., diagonal elements of
) are also fixed to 0 and 1, respectively.
After specifying and estimating this factor model for all groups, conclusions on configural invariance are drawn following the examination of goodness-of-fit measures. If supported, configural equivalence indicates that the shape of the model (i.e., number of factors and pattern of zero and non-zero loadings) is the same across groups.
2.2.2. Thresholds Invariance
If configural invariance holds, the invariance of thresholds is tested next. Here, the baseline model is identified by setting, for all groups, the latent content factor means and variances
to 0 and 1, respectively. Additionally, for the reference group r, the vector of latent intercepts
and latent response variable variances in
are set to 0 and 1, respectively. On top of these identification constraints, thresholds
are equated across groups and, after model estimation, the hypothesis of thresholds invariance is evaluated by evaluating the change in model fit between the configural model and the thresholds invariant model.
2.2.3. Loadings Invariance
If thresholds invariance holds, invariance of loadings is assessed. To identify the baseline model, for all groups, the latent content factor means are set to 0, while, for the reference group r, the factor variances
are set to 1, the latent intercepts
to 0 and variances in
to 1. In addition to these identification constraints, both thresholds
and loadings
are constrained to be equal across groups. Again, the model is estimated and the hypothesis of loadings invariance is evaluated by assessing the change in model fit between the thresholds invariant model and the loadings invariant model. Note that, if the hypothesis of thresholds and loadings invariance holds, factor variances can be validly compared across groups.
2.2.4. Intercepts Invariance
Finally, if loadings invariance holds, invariance of latent intercepts is assessed. To identify the baseline model, for the reference group, the latent content factor means and variances
are set to 0 and 1, respectively. Additionally, building on the previous equality constraints on thresholds and loadings, the latent intercepts
are set to 0 and equated across groups. To assess the hypothesis of latent intercepts invariance, the model is estimated and its fit is compared to the loadings invariant model. Following non-rejection of latent intercepts invariance, the factor means can be validly compared across groups.
2.2.5. Criteria to Assess Model Fit
Goodness-of-fit indices are commonly used as criteria to assess the tenability of MI hypotheses. This commonly entails evaluating the fit of the baseline model (i.e., configural model) and then the change in fit for the more restrictive models. To aid conclusions on whether the (change in) fit allows to conclude that a certain level of invariance (e.g., thresholds) holds, various criteria are inspected, each with its own proposed cut-off value determined via extensive simulation studies. Classically, only the chi-squared test was used as a criterion to assess the significance of change for two nested models (Putnick & Bornstein, Citation2016) but multiple studies have shown that relying solely on this statistic is sub-optimal due to it being extremely sensitive to negligible MM differences in large samples (Bentler, Citation1990; French & Finch, Citation2006; Citation2008). Therefore, in practice, MI decisions are based on multiple criteria (Putnick & Bornstein, Citation2016), and, among them, two of the most commonly used are the root mean square error of approximation (RMSEA; Browne & Cudeck, Citation1993) and the comparative fit index (CFI; Bentler, Citation1990). Configural invariance is concluded if RMSEA
0.06 and/or CFI is
0.95 (Brown, Citation2015). For the more restrictive models, the change in fit (e.g., ΔRMSEA) is assessed to conclude whether the additional constraints worsen the fit significantly. Cheung and Rensvold (Citation2002) suggested to conclude non-invariance when ΔCFI
−0.01, while Chen (Citation2007) recommended ΔRMSEA
0.01. Note that various criteria have been suggested for different fit measures, and we refer the reader to Svetina, Rutkowski, and Rutkowski (Citation2020) for an overview. Also, while recent research has indicated that model-specific cut-off values may be generally preferred to evaluate model fit (e.g., see McNeish & Wolf, Citation2023; or Finch & French, Citation2018) there are no available guidelines for calculating these cut-offs for MI testing in ordered-categorical data.
2.3. From Single Group to Multiple Groups: The Potential Effects of (Not) Correcting for ARS
In the literature, the bias resulting from disregarding ARS for single-group analyses is well-known but it is not yet clear to what extent it may generalize to multiple-group analyses, such as MG-CCFA. Response tendencies, such as ARS, represent sources of systematic response bias that may or may not appear as violations of measurement invariance (i.e., measurement non-invariance). In fact, ARS is often viewed as a factor with weak to moderate loadings (Danner et al., Citation2015; Ferrandoet al., Citation2004), which may be insufficient to result in significant violations of MI (i.e., rejection of MI). When ARS affects individuals’ responses in one of the groups, not taking into account this tendency towards acquiescence likely results in systematic differences in the responses across groups that are not purely due to the intended-to-be-measured (i.e., content) factors and, eventually, may lead to the rejection of measurement invariance. For instance, in single group studies, one well-known consequence of not accounting for ARS is that it may result in an additional factor (Billiet & McClendon, Citation2000; D'Urso et al., Citation2023). Therefore, it is reasonable to expect that researchers unaware of the (potential) influence of ARS would disregard this and reject configural invariance, which would lead them to conclude that the content factor(s) cannot be validly compared across groups since they (seem to) qualitatively differ. Additionally, single group studies showed that ARS can bias item (latent) intercepts (Cheung & Rensvold, Citation2000), and factor loadings (D'Urso et al., Citation2023). Ferrando & Lorenzo-Seva, Citation2010; Savalei & Falk, Citation2014; Again, neglecting this agreeing response tendency may result in non-equivalence (i.e., non-invariance) of intercepts and/or loadings, and lead researchers to conclude that the MM is non-invariant and, potentially, to allow some parameters to freely vary across groups (i.e., partial invariance) to reach an acceptable level of invariance before investigating differences in the content factor(s). Finally, even if invariance is tenable, ARS may still bias latent mean differences and thus lead researchers to conclude that the mean of the targeted latent variable differs across groups, while this may be a byproduct of neglecting an agreeing tendency in one of the groups.
Another important aspect to consider is that the performance of psychometric approaches developed to correct for ARS has not been thoroughly investigated in the context of MI testing. Savalei and Falk (Citation2014) have discussed some of the main factor-analytical approaches to correct for ARS, their underlying assumptions and compared their performance for single group analyses through a simulation study. The results have shown that the classical CFA-based approach (Billiet & McClendon, Citation2000), where ARS is specified as an additional factor orthogonal to the content factor(s) with all loadings set to 1 (i.e., the influence of ARS does not vary across items) outperformed the remaining onesFootnote1, even when some of its main assumptions (e.g., equal ARS loadings) are violated. Thus, based on the authors’ results, recommendations, and its straightforward implementation to MG-CCFA, we will mainly focus on this CFA-based approach in the remainder of this paper. Specifically, following this CFA-based approach, an additional ARS factor is added to the MM in all groups and all factor loadings on this additional factor are fixed to 1, which allows to freely estimate the ARS factor variance for all groups. Then, between-group differences in the amount of ARS are captured by differences in the ARS factor means, and within-group differences in the strength of ARS are captured by the ARS factor variances.
3. Simulation Study
To assess the effect of ARS on MI testing, both when including ARS as an additional factor in the measurement model (MM) and when not including it, we conducted a simulation study where individual responses in one group were affected by an ARS. Our goal is to solely focus on whether the bias introduced by disregarding ARS results in measurement non-invariance, and whether this is rectified by including an additional ARS factor in the MM for all groups. Therefore, we did not simulate other sources of non-invariance (e.g., differences in factor loadings). Furthermore, a null scenario was simulated, where invariance holds and ARS is not at play for both groups, which only served as a comparison for evaluating the performance of MG-CCFA approaches. Note that we report these latter results in the Online Supplementary (Tables A1–A3).
The following 5 factors were manipulated:
The number of subjects N within each group at 2 levels: 250, 1000;
The type of scale at 3 levels: balanced, semi-balanced and unbalanced;
The number of content factors Q at 2 levels: 1, 2;
The scale length (i.e., total number of items) at 2 levels: 12, 24;
The overall strength of the ARS factor at 2 levels: medium and large.
The difference in strength of the ARS across item at 2 levels: equal, unequal.
For the minimum sample size within each group, we followed the recommendations from previous research, which indicated that for obtaining precise factor loading estimates a sample size of 250 is sufficient when item communalities are moderate (Fabrigar et al., Citation1999; MacCallum et al., Citation1999). Furthermore, we varied (a) the number of factors to simulate both unidimensional and multidimensional scales, (b) the total number of items to simulate scales that measure the psychological construct to a varying degree of accuracy, and (c) the type of scale (e.g., semi-balanced) to emulate scales that allow disentangling the content factor(s) from the ARS factor to a different extent (de la Fuente & Abad, Citation2020; Savalei & Falk, Citation2014). Negatively keyed items may be more difficult to understand for some groups and thus elicit agreeing responses more than positively keyed items. To simulate this, we include conditions where the ARS loading size was equal across all items (i.e., “equal ARS”) as well as conditions where, for the semi(-) balanced scales, negatively keyed items had larger loadings on the ARS factor compared to positively keyed ones (i.e., “unequal ARS”) For unbalanced scales, half of the items had larger loadings on the ARS factor in the “unequal ARS” conditions.
In terms of the performance of MI testing, we hypothesize the following: violations of MI (i.e., non-invariance) will likely be detected when ARS is large and ignored (i.e., not included as an additional factor for both groups). Specifically, we expect that, for balanced and semi-balanced scales, disregarding a large ARS will result in non-invariance at all levels. For unbalanced scales, violations of MI may not be detected since ARS will likely affect structural rather than measurement parameters, like the covariance among content factors in case of a multidimensional scale (D'Urso et al., Citation2023), or factor variances, especially in the conditions with unidimensional scales (Ferrando & Lorenzo-Seva, Citation2010). In addition, we expect that including the additional ARS factor for both groups will allow for MI to be established in the case of balanced and semi-balanced scales. Finally, in the conditions with unbalanced scales, we hypothesize that including an additional ARS may result in model estimation issues since ARS cannot be easily disentangled from the content factor(s).
A full-factorial design was used with 2 (number of subjects) × 3 (type of scale) × 2 (number of content factors) × 2 (number of items) × 2 (strength of ARS) = 48 conditions. For each condition, 100 replications were generated, resulting in 4,800 data sets.
3.1. Methods
3.1.1. Data Generation
Data were generated from a factor model with one or two factors and two groups, and the model parameters are displayed in . To simulate balanced scales, for the content factor(s), half of the loadings were positive (i.e., indicative items) and the other half were negative (i.e., contra-indicative items), whereas 33% and none of the loadings were negative for semi-balanced scales and unbalanced scales, respectively. Note that, for both groups, 0 and 1 were used as generating values for the content factor(s) means and variances, respectively. As displayed in , we simulated ordinal items with 5 categories and the distance between the first threshold of the easiest and the most difficult item was 2 standard deviations. To avoid estimation issues (e.g., non-convergence), we only retained data sets where each category for each item contains at least a single observation. In the rare cases where, for a specific item, a category was not observed among the generated scores, we repeated the data generation process until all response categories were observed.
Table 2. Population values for the simulation study.
We sampled the ARS factor scores from a right-censored normal distribution to match an agreeing tendency closely. Employing this distribution, we only simulated subjects who did or did not show an ARS (i.e., have a positive or zero factor score on the ARS dimension) without allowing for scores to represent a disagreeing tendency (i.e., a negative factor score). For simulating the effect of ARS on the item responses, we used loading values of 0.3 and 0.6 for the medium and large ARS scenario, respectivelyFootnote2. Note that, for the reference group, the ARS factor scores were simulated to be 0 for all subjects (i.e., ARS did not affect the item responses). To simulate between-item-type differences in ARS loadings, in the “unequal ARS” conditions, we decreased the size of the loadings on positively keyed items compared and increased those on the negatively keyed ones, so that the average ARS loadings remained the same across groups (). Similarly, for unbalanced scales, the loadings were decreased for half of the items and increased for the other half.
3.1.2. Data Analysis
To simulate the effect of ignoring or including ARS when testing for MI, we considered two different MMs—and, thus, performed two different MG-CCFA analyses—for each replication, that is, with or without an additional ARS factor. For the latter, we used the standard CFA-based approach proposed by Billiet and McClendon (Citation2000), where an additional ARS factor is specified with all loading on this factor fixed to 1. Note that no additional constraints are imposed on the content factor loadings nor on the variance of the ARS factor under this model. To identify the MG-CCFA models, we followed the Wu and Estabrook (Citation2016) identification constraints for MI testing described in Section 2 for both the model with and without the ARS factor.
All MG-CCFA models were estimated using diagonally weighted least squares (DWLS), but the full weight matrix was used to compute the mean-and-variance-adjusted test statistics (default in lavaan; Rosseel, Citation2012). DWLS is a two-step estimation procedure, where the thresholds and polychoric correlation matrices for the groups are estimated in the first step, and, in the second step, the remaining parameters are estimated using the polychoric correlation matrices from the previous step.
3.1.3. Outcome Measures
After fitting the models, we evaluated both the convergence rate (CR) and the performance of different model fit criteria. For the latter, we recorded the results obtained from the test, the root mean square error of approximation (RMSEA; Browne et al., Citation1993) and the comparative fit index (CFI; Bentler, Citation1990) and we averaged across replications in a cell of the factorial design. In empirical practice, decisions about MI results are often dichotomous (i.e., invariant or not). Thus, we also calculated the false positive rate (FPR) for the different goodness-of-fit criteria, which is here defined as flagging the scale as non-invariantFootnote3. Specifically, configural non-invariance was concluded if:
test was significant (α = 0.05), RMSEA >0.06, CFI <0.95. In addition, since common guidelines suggest to base invariance decisions on different goodness-of-fit indices, we created a combined criterion and concluded configural invariance if both a significant
-difference test and at least one between RMSEA <0.06 and CFI >0.95 was observed (Putnick & Bornstein, Citation2016). We compared the fit between the configural and the thresholds invariant models for thresholds invariance, between the threshold and loadings invariant models for loadings invariance, and between loadings and intercepts invariant models for intercepts invariance. For all these comparisons, non-invariance was concluded if:
-difference test was significant (α = 0.05), ΔRMSEA >0.01, ΔCFI < −0.01. Finally, for the combined criterion, non-invariance was concluded if we observed both a significant
-difference test and at least one between ΔRMSEA >0.01 and ΔCFI < −0.01. Since we deem the results on the values of or differences in the fit indices to be more informative than these dichotomized results, we only display the latter in the Online Supplementary (Tables A10–A21). In addition, we examined the potential bias in latent mean differences when acquiescence is not accounted for in the measurement model. To achieve this goal, for each factor, we averaged the estimated latent variable mean for the focalFootnote4 (i.e., nonreference) group κf across replications in the intercepts invariance model (see 2.2.4). Note that, this average latent mean is a direct indication of bias, since we simulated it to be zero in the data generating model.
3.1.4. Data Simulation, Softwares and Packages
The data were simulated and analyzed using R (R Core Team, Citation2013). Specifically, for estimating MG-CCFA models and obtaining fit measures, we used the R package lavaan (Rosseel, Citation2012), while for specifying the MG-CCFA models we used the semTools package (Jorgensen et al., Citation2022).
3.2. Results
3.2.1. Without ARS Factor
3.2.1.1. Convergence
In the Online Supplementary, Tables A4 and A5 displays the convergence results when ARS is not included as an additional factor (i.e., disregarded) for equal and unequal ARS conditions, respectively. The convergence rate was always 100% across conditions for all MG-CCFA models and for both unidimensional and multidimensional scales. Therefore, disregarding the influence of ARS when assessing different levels of MI does not seem to affect model convergence.
3.2.1.2. MI Testing
The average fit measures results obtained when evaluating MI for unidimensional and multidimensional scales are displayed in , respectively, and we display the results for the “unequal” ARS conditions in the Online Supplementary in Tables A6–A9 since they largely overlap with those for the “equal” ARS ones. The results indicate that the ARS strength and the type of scale were the most relevant design factors affecting the MI testing results. In fact, for both unidimensional and multidimensional scales, ignoring the influence of ARS deteriorated models fit at all MI levels, and especially in the conditions with large ARS and balanced or semi-balanced scales. In these conditions, the RMSEA was often >0.10 and the CFI <0.90, which, in empirical practice, are commonly interpreted as “unacceptable” fit values. Note that, when the influence of ARS was small (i.e., λARS = 0.175), model fit was often good (i.e., RMSEA <0.06 and CFI >0.95), which is line with previous research indicating that when loadings on the ARS factor are small (i.e., 0.1) ignoring ARS does not seem to strongly affect the MM parameters recovery (Savalei & Falk, Citation2014). The fit measure values were good (i.e., RMSEA <0.06 and CFI >0.95) in the conditions with unbalanced scales regardless of the strength of the ARS factor and the MI level tested. Again, these results partially overlap with previous studies, indicating that the ARS factor gets absorbed by the content factors for unbalanced scales. Therefore, for unbalanced scales, one may conclude that MI holds even when one group has a strong agreeing tendency. Bear in mind that, for these scales, the bias introduced by an ARS does not seem to affect MI testing results but it may affect factor scores or factor covariances (e.g., see Savalei & Falk, Citation2014) for some groups, and thus (potentially) substantive conclusions. Concerning the dichotomized results (Tables A10–A12 in the Online Supplementary), almost all the considered criteria resulted in a close-to-one FPR when testing configural and intercepts invariance for balanced and semi-balanced scale, whereas for unbalanced scales the FPR was often close to 0.
Table 3. Average fit value for MI testing when the ARS factor is not included for unidimensional scales in function of the simulated conditions with equal ARS.
Table 4. Average fit value for MI testing when the ARS factor is not included for unidimensional scales in function of the simulated conditions with equal ARS.
Table 5. Average fit value for MI testing when the ARS factor is not included for multidimensional scales in function of the simulated conditions with equal ARS.
Table 6. Average fit value for MI testing when the ARS factor is not included for multidimensional scales in function of the simulated conditions with equal ARS.
3.2.1.3. Latent mean differences
The average bias in estimated latent mean difference in function of the different conditions when ARS is ignored are displayed in . Overall, the bias is especially large in the conditions with unbalanced scales (0.19 and 0.35 for small and large ARS, respectively). This is likely due to the fact that, in these conditions, all items introduce bias in the same direction (i.e., positive) since all items were positively-keyed. In contrast, in the conditions with balanced scales and equal ARS, positively and negatively keyed items bias the latent mean in opposite directions, thus canceling out one another and resulting in nearly unbiased estimates of the latent means. In the unequal ARS conditions, with larger ARS loadings for negatively-keyed items, the biases of the positively- and negatively-keyed items do not completely cancel out, resulting in negatively biased latent means for the balanced scales. This also explains why, for semi-balanced scales (with only one-third of negatively-keyed items), the bias is positive in the equal ARS conditions and nearly zero in the unequal ARS conditions, since the bias result from the positively-keyed items is only canceled out completely when the (fewer) negatively-keyed items get larger loadings. Finally, note that, given that latent variables are standardized, the latent mean for the focal group can be interpreted as Cohen’s d, thus indicating that disregarding ARS erroneously leads to small to moderate standardized mean differences across groups.
Table 7. Latent mean bias in the intercept invariance model in function of the simulated conditions when the ARS factor is not included.
3.2.2. With ARS Factor
3.2.2.1. Convergence
Footnote5 displays the model convergence results when including an additional ARS factor in the MM. Convergence was strongly affected by the type of scale. In fact, for unbalanced scales, the convergence rate was lower than in the conditions with (semi-) balanced scales and especially low when testing for configural invariance. Therefore, one may often fail to evaluate configural invariance when including ARS for an unbalanced scale in empirical practice. Note that this is likely caused by the fact that the ARS factor cannot be distinguished from the content factor(s), which is corroborated by previous research indicating that, in EFA, the additional ARS factor for unbalanced scales is not captured when selecting the number of factors (D'Urso et al., Citation2023); Ferrando & Lorenzo-Seva, Citation2010; The convergence rate is a lot higher for the higher levels of invariance, however, which leaves possibilities to scrutinize measurement (non-) invariance at these levels.
Table 8. Convergence rate in function of the simulated conditions when the ARS factor is included.
3.2.2.2. MI Testing
display the MI testing results when an additional ARS factor is included in the MM for unidimensional and multidimensional scales, respectively. We display the results for the unequal ARS conditions in the Online Supplementary in Tables A15–A18 as they largely overlap with those for the equal ARS conditions. The average fit measures results indicate that, for both unidimensional and multidimensional scales and for all MI levels tested, including the additional ARS factor yields good to perfect fit according to all fit measures regardless of the other design factors. For the dichotomized results (Tables A19–A22 in the Online Supplementary), almost all the considered criteria resulted in a close-to-zero FPR when testing MI at all levels.
Table 9. Average fit value for MI testing when the ARS factor is included for unidimensional scales in function of the simulated conditions with equal ARS.
Table 10. Average fit value for MI testing when the ARS factor is included for unidimensional scales in function of the simulated conditions with equal ARS.
Table 11. Average fit value for MI testing when the ARS factor is included for multidimensional scales in function of the simulated conditions with equal ARS.
Table 12. Average fit value for MI testing when the ARS factor is included for multidimensional scales in function of the simulated conditions with equal ARS.
3.2.2.3. Latent Mean Differences
displays the bias in the estimated latent mean difference when ARS is included as an additional factor in function of the simulated conditions. The results show that the bias is negligible for (semi-) balanced scales, which adds to the benefits of working with (semi-) balanced scales. For the unbalanced scales, the latent mean difference appeared to be highly distorted. These distortions were mostly due to inadmissible solutions with negative factor variances, signaling model identification issues. Thus, we calculated the bias only for those models that did not result in improper solutions. The results showed not only that many models resulted in improper solutions, but also that, for the ones with admissible solutions the latent mean difference were not negligible. Hence, for unbalanced scales, ARS still distorts conclusions for latent mean differences even when it is explicitly modeled, and (semi-) balanced scales should be preferred to accurately recover latent mean differences in content factors across groups.
Table 13. Latent mean bias in the intercept invariance model in function of the simulated conditions when the ARS factor is included.
4. Conclusions
The simulation study assessed the effect of disregarding and including an additional ARS factor on MI testing when responses in one group are affected by an ARS. The results showed that not taking a strong (unequal) ARS into account resulted not only in wrongly concluding there is measurement non-invariance for balanced and semi-balanced scales but also in biased estimated latent means. In fact, for these scales, model fit heavily deteriorated for all MI levels, and thus one may conclude that the content factor(s) MM differs across groups while this is purely due to a strong agreeing tendency in one group. Note that this result is significant for empirical practice, where researchers that follow the standard CFA-based MI testing approach may conclude that configural invariance does not hold and try to modify the MM to be able to compare the groups or, in the most extreme case, refrain from further analyses. In the balanced and semi-balanced scales conditions, this issue was solved by including, for all groups, an additional ARS factor with all its loadings fixed to 1, which resulted in concluding that MI held at all levels, and in an accurate recovery of latent mean differences. For unbalanced scales, disregarding ARS (i.e., not including ARS as an additional factor) did not affect the MI testing results, which indicated that MI held at all levels. This latter result partially overlaps with previous research, showing that ARS gets absorbed by the content factor(s) in unbalanced scales (D'Urso et al., Citation2023). Ferrando & Lorenzo-Seva, Citation2010; However, for these scales, the disregarded ARS resulted in considerable bias in estimated latent mean differences. Hence, even though ARS does not influence MI testing in case of unbalanced scales, it may still lead to wrongly concluding that latent means differ across groups, while this is purely due to a disregarded ARS. Thus, for unbalanced scales, this is a possibility that one should take into account. Including an additional factor to capture ARS is not a solution for unbalanced scales since this often led to model non-convergence, especially when testing for configural invariance, likely due to the indistinguishability of the ARS factor from the content factor(s). Further, it either resulted in improper solutions when testing for intercepts invariance or it did not allow to accurately recover latent means, thus indicating that, when ARS is at play, group differences may be heavily misjudged.
5. Discussion
In psychological science, self-report scales are widely used to compare targeted latent constructs (e.g., depression) across groups. To draw valid and unbiased conclusions concerning latent construct differences, one must ensure that the self-report scales used to measure these constructs function equivalently across groups. The latter is often assessed through measurement invariance (MI) testing, which evaluates the tenability of the hypothesis of measurement model (MM) equivalence. For scales composed of ordinal items, MI is often tested through multiple group categorical confirmatory factor analysis (MG-CCFA), which allows evaluating MM parameters’ equivalence across groups in a step-wise fashion. In addition to the scale itself being inequivalent, non-invariances may emerge when disregarding the influence of an agreeing response style (ARS), which represents a tendency to agree with items regardless of their content (Paulhus, Citation1991). Though it is known that certain groups may be particularly prone to ARS (i.e., see Van Vaerenbergh & Thomas, Citation2013 for a review), and that such response tendency can bias MM parameters in single group studies (Ferrando & Lorenzo-Seva, Citation2010), this is the first paper thoroughly evaluating the effects of ARS on MI testing. Determining if disregarding ARS can appear as measurement non-invariance may help to ascertain how to correct for bias in latent construct differences due to differential response tendencies across groups. In fact, it is superfluous to look for scale-specific causes of non-invariance if these are entirely due to ARS. Instead, including an extra factor to model the ARS corrects for the bias. In this paper, we conducted a simulation study to evaluate in what conditions and to what extent an ARS affecting the individual responses in one of the groups is detected as measurement non-invariance, both when disregarding ARS and when including it as an additional factor in the measurement model (MM) with all its loadings fixed to 1.
One of the more significant findings from this study is that ignoring a large ARS resulted in measurement non-invariance at all levels and biased latent mean differences for balanced and semi-balanced scales, which was solved by including an additional factor capturing ARS. Therefore, when using (semi-) balanced scales, researchers should bear in mind that configural non-invariance and artificial differences in latent means may result from disregarding a large ARS, and that including an additional ARS factor in the MM for all groups is an effective way to correct for this. In this way, researchers can ascertain that there is no need to look for or remedy inequivalences that pertain to the scale. For unbalanced scales, disregarding an ARS did not affect MI testing results, and including an additional ARS factor was not advantageous since it often led to model non-convergence. This is likely due to the fact that, for unbalanced scales, the intended-to-be-measured (i.e., content) factors cannot be easily distinguished from the ARS factor. Nevertheless, for these scales, one should not conclude that ignoring an ARS is harmless because estimated latent mean differences are heavily biased (potentially in addition to bias in the factor correlations; Savalei & Falk, Citation2014; de la Fuente & Abad, Citation2020; D'Urso et al., Citation2023), and thus affect substantive conclusions. Sadly, in practice, the bias due to neglecting an ARS (e.g., in factor correlations and factor scores) may not be detected when testing for MI using unbalanced scales, and the correction proposed by Billiet and McClendon (Citation2000) is not a solution. Therefore, in settings where ARS could be present, using unbalanced scales is inherently problematic as they do not allow one to correct for this response tendency.
Taken all together, these results indicate that ARS is a serious threat to MI testing results and that (semi-)balanced scales should be preferred when suspecting that an ARS may be at play for specific groups. Using balanced or semi-balanced scales is not always straightforward, however. For instance, negatively worded items often require higher reading levels or intellectual capacity that cannot be assumed for certain (e.g., clinical) populations (Chyung et al., Citation2018). In these cases, one may consider using specific “marker” items or scales tailored to measure ARS, but further research is needed to evaluate the feasibility of this approach in the context of multidimensional scales and multiple group models (Ferrando et al., Citation2016). Alternatively, model non-convergence and improper solutions may be solved by bounded estimation (De Jonckere & Rosseel, Citation2022), where data-driven upper and lower bounds for model parameters can be specified prior to estimating the model (e.g., setting the lower bound of the ARS factor variance to a non-negative number), is a promising solution that has not yet been evaluated for MG-CCFA.
Our simulation study is subject to a few limitations that are worth noting. First, ARS was the only considered source of bias that, when disregarded, yielded us to conclude that there is non-invariance. However, in practice, it is reasonable to expect that other, scale-specific sources of non-invariance, such as differential item interpretation, may affect individual responses in some (or all) groups. In the future, it would be interesting to extend the current simulation study to evaluate whether non-invariance due to disregarding an ARS may be disentangled from other non-invariances such as specific factor loading differences for the content factors. However, this CFA-based approach can also fall short (i) when items load on more than one factor at the same time (i.e., cross-loadings) and (ii) when researchers are interested in assessing (between-group differences in) the ARS factor loadings. One may consider using multiple group exploratory factor analysis (MG-EFA; Jöreskog, Citation1970) to overcome these limitations. MG-EFA does not impose an assumed structure on the factor loadings and thus can easily capture cross-loadings. Furthermore, in MG-EFA, one does not need to assume that the influence of ARS is equal across items (i.e., the loadings on the ARS factor do not need to be constrained to be 1). In fact, by using a (semi-)specified target rotation, one may estimate the loadings of the additional ARS factor—that is, by specifying (part of) the rotation target according to a priori expectations on the MM while leaving the ARS factor loadings unspecified (D'Urso et al., Citation2023). Second, ARS was assumed to affect the responses in only one of the groups, while, in practice, the responses in all groups may be influenced by ARS but to a different extent (e.g., ARS loadings may be higher in one group). In those cases, the CFA-based approach discussed can also be applied to test for MI, and its outcome (i.e., rejecting MI or not) will depend on the differences in ARS across groups.
Third, we considered simulation scenarios with only two groups. MI testing has become increasingly relevant for cross-cultural and cross-national research, where large data sets with many groups are the norm (Rutkowski & Svetina, Citation2017). Hence, future research should evaluate the extent to which disregarding ARS or not affects MI testing when this agreeing bias influences responses only for a subset of groups or when it gradually differs across all groups.
Fourth, we followed a scale-based MI testing framework, but alternative approaches, such as item-based analyses (e.g., multiple group item response theory; D'Urso et al., Citation2022), may also be of interest, especially when the bias caused by an ARS affects some items more than others or when trying to distinguish ARS from specific differences in the content factor loadings.
Fifth, we use standard cut-off values for describing our results based on known guidelines (e.g., Cheung & Rensvold, Citation2002). However, these cut-off values have been criticized due to their lack of generalizability beyond the models used to determine these values in the first place. Therefore, alternative approaches to determine model-specific cut-off values have been proposed, such as: (1) Dynamic fit indices (McNeish & Wolf, Citation2023) and (2) equivalence testing procedures (Finch & French, Citation2018; Marcoulides & Yuan, Citation2017; Yuan et al., Citation2016). However, these alternatives are not yet readibly applicable to the conditions evaluated in this paper, since the former is still limited in its generalization to measurement invariance (MI) testing, while the latter is limited to continuous, normally distributed items. In the future, once these limitations are mitigated, it may be interesting to re-assess our conclusions’ generalizability to alternative cut-off values.
Nevertheless, the present study is the only thorough investigation of the effect of ARS on MI testing. We showed that correcting for agreeing bias when testing for MI allows to determine that the scale is invariant otherwise. We expect this outcome to be tremendously valuable in empirical practice as this avoids unnecessary worries about and investigations of scale non-equivalence (e.g., looking for non-invariant items).
Notes
1 The other approaches discussed by Savalei and Falk (Citation2014) are the Chan and Bentler (Citation1993) approach and the EFA-based approach (Ferrando et al., Citation2004). In the former, data must be first mean-centered within person (i.e., ipsatized). Then, a residual structure must be specified by adding a linear combination of the original residual components for each of the ipsatized variables. In the latter, an additional factor is first extracted, and then a rotation is performed to a partially specified target, which allows to estimate both content and ARS factor loadings.
2 The variance of a right-censored normal distribution is smaller than the identification restrictions imposed to set a scale for the variance of the ARS factor (i.e., fixing all its loadings to 1). In Table 2 we report the value of the original loadings on the ARS factor multiplied by the standard deviation of a right-censored normal distribution, which is 0.583. This results in loadings on the ARS factor of 0.175 and 0.350 for medium and large ARS conditions, respectively. Note that these values match those used in previous studies to simulate ARS factor loadings that can be realistically expected in well-designed measures (Danner et al., Citation2015; Ferrando & Lorenzo-Seva, Citation2010).
3 Note that, outside of the null condition, this is not formally a FPR. In fact, only when considering the ARS factor can we really say that the scale is invariant, whereas disregarding it results in between the scales.
4 Note that we only considered the latent mean estimates for the focal group since the reference group latent means are constrained to 0 for model identification.
5 The results for the unequal ARS conditions largely overlap with those displayed in this table, thus we report them in the Online Supplementary on Table A14.
References
- Aichholzer, J. (2015). Controlling acquiescence bias in measurement invariance tests. Psihologija, 48, 409–429. https://doi.org/10.2298/PSI1504409A
- Arias, V. B., Garrido, L., Jenaro, C., Martínez-Molina, A., & Arias, B. (2020). A little garbage in, lots of garbage out: Assessing the impact of careless responding in personality survey data. Behavior Research Methods, 52, 2489–2505. https://doi.org/10.3758/s13428-020-01401-8
- Austin, E. J., Deary, I. J., & Egan, V. (2006). Individual differences in response scale use: Mixed Rasch modelling of responses to neo-FFI items. Personality and Individual Differences, 40, 1235–1245. https://doi.org/10.1016/j.paid.2005.10.018
- Bachman, J. G., & O'Malley, P. M. (1984). Yea-saying, nay-saying, and going to extremes: Black-white differences in response styles. Public Opinion Quarterly, 48, 491–509. https://doi.org/10.1086/268845
- Bentler, P. M. (1990). Comparative fit indexes in structural models. Psychological Bulletin, 107, 238–246. https://doi.org/10.1037/0033-2909.107.2.238
- Billiet, J. B., & McClendon, M. J. (2000). Modeling acquiescence in measurement models for two balanced sets of items. Structural Equation Modeling, 7, 608–628. https://doi.org/10.1207/S15328007SEM0704_5
- Borsboom, D. (2006). When does measurement invariance matter? Medical Care, 44, S176–S181. https://doi.org/10.1097/01.mlr.0000245143.08679.cc
- Brown, T. A. (2015). Confirmatory factor analysis for applied research. Guilford publications.
- Browne, M. W., & Cudeck, R. (1993). Alternative ways of assessing model fit. Sage Focus Editions, 154, 136–136.
- Chan, W., & Bentler, P. M. (1993). The covariance structure analysis of Ipsative data. Sociological Methods & Research, 22, 214–247. https://doi.org/10.1177/0049124193022002003
- Chang, Y.-W., Hsu, N.-J., & Tsai, R.-C. (2017). Unifying differential item functioning in factor analysis for categorical data under a discretization of a normal variant. Psychometrika, 82, 382–406. https://doi.org/10.1007/s11336-017-9562-0
- Chen, F. F. (2007). Sensitivity of goodness of fit indexes to lack of measurement invariance. Structural Equation Modeling, 14, 464–504. https://doi.org/10.1080/10705510701301834
- Cheung, G. W., & Rensvold, R. B. (2000). Assessing extreme and acquiescence response sets in cross-cultural research using structural equations modeling. Journal of Cross-Cultural Psychology, 31, 187–212. https://doi.org/10.1177/0022022100031002003
- Cheung, G. W., & Rensvold, R. B. (2002). Evaluating goodness-of-fit indexes for testing measurement invariance. Structural Equation Modeling, 9, 233–255. https://doi.org/10.1207/S15328007SEM0902_5
- Chyung, S. Y., Barkin, J. R., & Shamsy, J. A. (2018). Evidence-based survey design: The use of negatively worded items in surveys. Performance Improvement, 57, 16–25. https://doi.org/10.1002/pfi.21749
- Danner, D., Aichholzer, J., & Rammstedt, B. (2015). Acquiescence in personality questionnaires: Relevance, domain specificity, and stability. Journal of Research in Personality, 57, 119–130. https://doi.org/10.1016/j.jrp.2015.05.004
- De Jonckere, J., & Rosseel, Y. (2022). Using bounded estimation to avoid nonconvergence in small sample structural equation modeling. Structural Equation Modeling, 29, 412–427. https://doi.org/10.1080/10705511.2021.1982716
- de la Fuente, J., & Abad, F. J. (2020). Comparing methods for modeling acquiescence in multidimensional partially balanced scales. Psicothema, 32, 590–597. https://doi.org/10.7334/psicothema2020.96
- D'Urso, E. D., De Roover, K., Vermunt, J. K., & Tijmstra, J. (2022). Scale length does matter: recommendations for measurement invariance testing with categorical factor analysis and item response theory approaches. Behavior Research Methods, 54, 2114–2145. https://doi.org/10.3758/s13428-021-01690-7
- D'Urso, E. D., Tijmstra, J., Vermunt, J. K., & De Roover, K. (2023). Awareness is bliss: How acquiescence affects exploratory factor analysis. Educational and Psychological Measurement, 83, 433–472. https://doi.org/10.1177/00131644221089857
- Fabrigar, L. R., Wegener, D. T., MacCallum, R. C., & Strahan, E. J. (1999). Evaluating the use of exploratory factor analysis in psychological research. Psychological Methods, 4, 272–299. https://doi.org/10.1037/1082-989X.4.3.272
- Ferrando, P. J., Condon, L., & Chico, E. (2004). The convergent validity of acquiescence: An empirical study relating balanced scales and separate acquiescence scales. Personality and Individual Differences, 37, 1331–1340. https://doi.org/10.1016/j.paid.2004.01.003
- Ferrando, P. J., & Lorenzo-Seva, U. (2010). Acquiescence as a source of bias and model and person misfit: A theoretical and empirical analysis. The British Journal of Mathematical and Statistical Psychology, 63, 427–448. https://doi.org/10.1348/000711009X470740
- Ferrando, P. J., Morales-Vives, F., & Lorenzo-Seva, U. (2016). Assessing and controlling acquiescent responding when acquiescence and content are related: A comprehensive factor-analytic approach. Structural Equation Modeling, 23, 713–725. https://doi.org/10.1080/10705511.2016.1185723
- Finch, W. H., & French, B. F. (2018). A simulation investigation of the performance of invariance assessment using equivalence testing procedures. Structural Equation Modeling, 25, 673–686. https://doi.org/10.1080/10705511.2018.1431781
- French, B. F., & Finch, W. H. (2006). Confirmatory factor analytic procedures for the determination of measurement invariance. Structural Equation Modeling, 13, 378–402. https://doi.org/10.1207/s15328007sem1303_3
- French, B. F., & Finch, W. H. (2008). Multigroup confirmatory factor analysis: Locating the invariant referent sets. Structural Equation Modeling, 15, 96–113. https://doi.org/10.1080/10705510701758349
- Guenole, N., & Brown, A. (2014). The consequences of ignoring measurement invariance for path coefficients in structural equation models. Frontiers in Psychology, 5, 980. https://doi.org/10.3389/fpsyg.2014.00980
- Jeong, S., & Lee, Y. (2019). Consequences of not conducting measurement invariance tests in cross-cultural studies: A review of current research practices and recommendations. Advances in Developing Human Resources, 21, 466–483. https://doi.org/10.1177/1523422319870726
- Johnson, T., Kulesa, P., Cho, Y. I., & Shavitt, S. (2005). The relation between culture and response styles: Evidence from 19 countries. Journal of Cross-Cultural Psychology, 36, 264–277. https://doi.org/10.1177/0022022104272905
- Jones, R. N., & Gallo, J. J. (2002). Education and sex differences in the mini-mental state examination: effects of differential item functioning. The Journals of Gerontology. Series B, Psychological Sciences and Social Sciences, 57, P548–P558. https://doi.org/10.1093/geronb/57.6.p548
- Jöreskog, K. (1970). Simultaneous factor analysis in several populations. ETS Research Bulletin Series, 1970, i–31. https://doi.org/10.1002/j.2333-8504.1970.tb00790.x
- Jorgensen, T. D., Pornprasertmanit, S., Schoemann, A. M., & Rosseel, Y. (2022). semTools: Useful tools for structural equation modeling. R package version 0.5-6. Retrieved from https://CRAN.R-project.org/package=semTools
- Liu, M., Harbaugh, A. G., Harring, J. R., & Hancock, G. R. (2017). The effect of extreme response and non-extreme response styles on testing measurement invariance. Frontiers in Psychology, 8, 726. https://doi.org/10.3389/fpsyg.2017.00726
- MacCallum, R. C., Widaman, K. F., Zhang, S., & Hong, S. (1999). Sample size in factor analysis. Psychological Methods, 4, 84–99. https://doi.org/10.1037/1082-989X.4.1.84
- Marcoulides, K. M., & Yuan, K.-H. (2017). New ways to evaluate goodness of fit: A note on using equivalence testing to assess structural equation models. Structural Equation Modeling, 24, 148–153. https://doi.org/10.1080/10705511.2016.1225260
- Marin, G., Gamba, R. J., & Marin, B. V. (1992). Extreme response style and acquiescence among hispanics: The role of acculturation and education. Journal of Cross-Cultural Psychology, 23, 498–509. https://doi.org/10.1177/0022022192234006
- McNeish, D., & Wolf, M. G. (2023). Dynamic fit index cutoffs for confirmatory factor analysis models. Psychological Methods, 28, 61–88. https://doi.org/10.1037/met0000425
- Meisenberg, G., & Williams, A. (2008). Are acquiescent and extreme response styles related to low intelligence and education? Personality and Individual Differences, 44, 1539–1550. https://doi.org/10.1016/j.paid.2008.01.010
- Meredith, W., & Teresi, J. A. (2006). An essay on measurement and factorial invariance. Medical Care, 44, S69–S77. https://doi.org/10.1097/01.mlr.0000245438.73837.89
- Muthén, B., & Muthén, B. O. (2009). Statistical analysis with latent variables (vol. 123). Wiley.
- Paulhus, D. L. (1991). Measurement and control of response bias. In J. P. Robinson, P. R. Shaver, & L. S. Wrightsman (Eds.), Measures of personality and social psychological attitudes (pp. 17–59). Academic Press.
- Putnick, D. L., & Bornstein, M. H. (2016). Measurement invariance conventions and reporting: The state of the art and future directions for psychological research. Developmental Review, 41, 71–90. https://doi.org/10.1016/j.dr.2016.06.004
- R Core Team. (2013). R: A language and environment for statistical computing [Computer software manual]. Vienna, Austria. Retrieved from http://www.R-project.org/
- Rios, J. A. (2021). Is differential non-effortful responding associated with type I error in measurement invariance testing? Educational and Psychological Measurement, 81, 957–979. https://doi.org/10.1177/0013164421990429
- Rosseel, Y. (2012). Lavaan: An R package for structural equation modeling. Journal of Statistical Software, 48, 1–36. https://doi.org/10.18637/jss.v048.i02
- Rutkowski, L., & Svetina, D. (2017). Measurement invariance in international surveys: Categorical indicators and fit measure performance. Applied Measurement in Education, 30, 39–51. https://doi.org/10.1080/08957347.2016.1243540
- Savalei, V., & Falk, C. F. (2014). Recovering substantive factor loadings in the presence of acquiescence bias: A comparison of three approaches. Multivariate Behavioral Research, 49, 407–424. https://doi.org/10.1080/00273171.2014.931800
- Svetina, D., Rutkowski, L., & Rutkowski, D. (2020). Multiple-group invariance with categorical outcomes using updated guidelines: An illustration using M plus and the Lavaan/SEMtools packages. Structural Equation Modeling, 27, 111–130. https://doi.org/10.1080/10705511.2019.1602776
- Van Vaerenbergh, Y., & Thomas, T. D. (2013). Response styles in survey research: A literature review of antecedents, consequences, and remedies. International Journal of Public Opinion Research, 25, 195–217. https://doi.org/10.1093/ijpor/eds021
- Weijters, B., Geuens, M., & Schillewaert, N. (2010). The stability of individual response styles. Psychological Methods, 15, 96–110. https://doi.org/10.1037/a0018721
- Welkenhuysen-Gybels, J., Billiet, J., & Cambré, B. (2003). Adjustment for acquiescence in the assessment of the construct equivalence of Likert-type score items. Journal of Cross-Cultural Psychology, 34, 702–722. https://doi.org/10.1177/0022022103257070
- Wu, H., & Estabrook, R. (2016). Identification of confirmatory factor analysis models of different levels of invariance for ordered categorical outcomes. Psychometrika, 81, 1014–1045. https://doi.org/10.1007/s11336-016-9506-0
- Yuan, K.-H., Chan, W., Marcoulides, G. A., & Bentler, P. M. (2016). Assessing structural equation models by equivalence testing with adjusted fit indexes. Structural Equation Modeling, 23, 319–330. https://doi.org/10.1080/10705511.2015.1065414