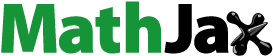
ABSTRACT
Operational performance of fully mechanized cut-to-length (CTL) harvesting varies greatly due to the human factor i.e. the machine operator. This study investigated how CTL machine operators’ workability index (WAI), personal lifestyle choices, seasons, and shift work affected operational performance. Research evaluated 14 volunteer CTL machine operators for a longitudinal study with continuous data collection of productivity, activity level, sleep, and follow-up on a workability index questionnaire and fitness test every three months over a year. The study analyzed the production of 152 745.5 m3 of timber combined with self-tracking data. Operators’ relative productivity (Pr) had an increasing trend whilst WAI increased, thus WAI seems to work well also for forestry applications. Physical fitness (VO2max) didn’t seem to connect with Pr and WAI had only a slightly increasing trend when VO2max increased. The participants slept longer in the evening shift than in the morning shift (p < 0.000) consequently catching up on their sleep deficit from the morning shift period. Furthermore, operators’ higher sleep value (SV) in the evening shift increased Pr in the final fellings. The results should be of interest to both practitioners and researchers interested in the productivity of harvesting operations.
Introduction
Fully mechanized cut-to-length (CTL) harvesting varies greatly in productivity due to the machine operator, i.e. the human factor. Productivity varies as much as 59% between operators (e.g. Sirén Citation1998; Ovaskainen et al. Citation2004; Purfürst and Erler Citation2011; Olivera et al. Citation2016; Liski et al. Citation2020). Operators carry out intensive decision-making processes and complex visual-motor tasks at a high pace (Asikainen et al. Citation2009; Gellerstedt Citation2013) but their overall work description varies globally. In the Nordic forest environment, operating CTL machines is a strongly independent and demanding work requiring expertise with heavy machinery, forest management, raw material, IT and communications to succeed. Operator’s productivity and expertise develop over several years. Purfürst (Citation2010) suggests that the first learning phase lasts approximately 8 months but others have identified that productivity and expertise can continuously increase for 15–20 years (Malinen et al. Citation2018; Spinelli et al. Citation2020). In the study of Spinelli et al. (Citation2020), productivity increased linearly by ca. 1.5% points per year on the job. Subjects with 20 years of experience were 27% more productive than beginners. Work experience and expertise are not the only factors influencing operational performance (Häggström and Lindroos Citation2016). Whilst stem size is the most recognized factor influencing productivity (Jiroušek et al. Citation2008; Eriksson and Lindroos Citation2014; Apǎfǎian et al. Citation2017; Visser and Spinelli Citation2017), level of productivity varies with environmental and organizational changes e.g. forest structure (Spinelli et al. Citation2020), and terrain characteristics (Ottaviani Aalmo et al. Citation2020), length and timing of work shifts, and work schedule design (e.g. Nicholls et al. Citation2004; Pasicott and Murphy Citation2013; Murphy et al. Citation2014).
Close tracking and supervision of CTL productivity have been enabled by modern information systems (Arlinger et al. Citation2010; Eriksson and Lindroos Citation2014; Liski et al. Citation2020). CTL machines’ have onboard computers with an internet connection, digital work instructions, maps with real-time positioning (GPS), decision support features, and monitoring and reporting applications (Kemmerer and Labelle Citation2021). These technologies continuously capture massive volumes of “big data,” featuring the machine functions, productivity, and processed raw material. Furthermore, many CTL machine companies provide browser based “fleet management” applications for real-time office use to ease supervising work. Outside of the fleet management systems, the CTL machines’ software can also produce some data that are readable with standard PC programs.
Both work descriptions, and forest machine technology (machinery and IT) affect operators’ well-being, work fluency, and flow, and it’s generally recognized that employees’ individual workability influences their work productivity (e.g. Kuoppala et al. Citation2008; Vänni et al. Citation2015; Ahola et al. Citation2018). Workability can be described as the balance between the employee’s personal resources and the work demands (Ilmarinen Citation2009), and it constantly changes throughout their life and different life phases. Workability can be evaluated with the workability index questionnaire, developed by the Finnish Institute of Occupational Health (Ilmarinen et al. Citation1991; Tuomi Citation1997) and it is by far the most well-accepted instrument to measure workability (van der Berg et al. Citation2008). In addition to productivity, high workability is associated with a high quality of work, work satisfaction, and staying in one’s job (Tuomi et al. Citation2001), and poor workability has been linked with increased risk of early retirement, sick leaves, long-term disability and long-term unemployment (Burdorf et al. Citation2005; Sell et al. Citation2009; Lundin et al. Citation2016). Employee lifestyle habits, personal characteristics, and attitudes play a vital role in the employee’s workability. Obesity, low physical activity, smoking, and unhealthy diet are associated with low workability (e.g. Tuomi et al. Citation2001; Arvidson et al. Citation2013; Oellingrath et al. Citation2019) whereas duration and quality of sleep, and physical activity are related to higher levels of workability (e.g. Camerino et al. Citation2008; Lian et al. Citation2014; Kettunen Citation2015; Bergman et al. Citation2020).
Forest machine operators tend to work in shifts (Kymäläinen et al. Citation2021) which vary in length with the market situation and the seasons/weather in the Nordic countries. Shift work exposes the worker to circadian misalignment and sleep restriction, and it’s generally associated with disturbed life rhythms (Declercq et al. Citation2022). Shift workers suffer excessive fatigue and insomnia far more often than day workers, and it can lead to undesirable effects, such as reduced work performance, increased processing errors, and accidents at work (Drake et al. Citation2004; Eldevik et al. Citation2013; Katz et al. Citation2014; Gingerich et al. Citation2018; Declercq et al. Citation2022). The rising and setting of the sun synchronizes human circadian rhythm through a process known as entrainment (Gibson and Shrader Citation2018), and the human circadian clock is predominantly and powerfully entrained by the sun rather than by social time (Roenneberg et al. Citation2007). Furthermore, daylight hours in Nordic countries vary to a great extent between summer and winter seasons, and intra-annual changes in sunlight also influence human sleep patterns through a similar process of entrainment (Hubert et al. Citation1998; Gibson and Shrader Citation2018). Nicholls et al. (Citation2004) discovered the timber harvesting operators’ shortest sleep was after the third rostered shift implying operator fatigue would be highest toward the end of the working week. In addition, studies show that insufficient sleep induces a decline in cognitive capacity (Rosekind et al. Citation2010; Bartrim et al. Citation2020) which is essential in CTL harvesting work.
Tracking one’s well-being and quality of life is common outside of working life as the use of consumer-based wearable smart technology (wearables), such as smartwatches, pedometers, and mobile applications is becoming ubiquitous in modern society (Depner et al. Citation2019). With a promise to improve the quality of life of consumers, digital technologies rely greatly on gamification to sustain users’ motivation to continued monitoring and tracking (Whitson Citation2013; Maturo and Setiffi Citation2016), and such phenomenon enables the use of collected data for research purposes, in particular those connected to productivity and well-being. Tracking and monitoring in everyday authentic settings carries the potential to grasp phenomena that cannot be observed in laboratory settings or short-term follow-ups (Henriksen et al. Citation2018). Light-weighted and accessible smartwatches (Larrichia Citation2022) use accelerometers, optical heart rate monitoring, and algorithms combined with the user’s characteristics, such as age, gender, weight, and height to measure and estimate heart rate, heart rate variability, physical activity, energy expenditure, maximal oxygen uptake (VO2nax), and sleep quality.
Albeit digital technologies enable a wide range of long-term data collection of CTL machine productivity and personal self-tracking, the research is limited to the theme. Some studies in the area of forest ergonomics have integrated consumer-based wearables in research settings, mainly for measuring heart rate (e.g. Leszczyński and Stańczykiewicz Citation2015; Aalmo et al. Citation2016; Grzywiński et al. Citation2017; Bowen et al. Citation2019; Borz et al. Citation2019; Spinelli et al. Citation2020), thus monitoring time was limited to short periods during (physical) work. Moreover, previous research has a limited focus on job demands. The importance of personnel’s workability is recognized for successful work performance, not only for the individual but for the thriving business altogether. Alongside work characteristics and organizational factors, one’s attitudes and lifestyle choices have a great impact on workability and overall well-being.
The present case study aimed for long-term follow-up on how personal lifestyle habits influence CTL machine operational productivity and operators’ workability by conjoining CTL harvester machines’ productivity data with machine operators’ personal “self-tracking” data on and off work. The study estimated how productivity was influenced by changes in sleep, activity, maximal oxygen uptake, and workability index, to discover if there were connections between lifestyle habits, maximal oxygen uptake, and workability. Also, organizational and environmental job demands, such as shift work and the changes of seasons were taken into consideration.
Materials and methods
The study group participants
Research evaluated a group of volunteer CTL machine operators in Finland for a longitudinal study with continuous data collection of productivity, activity level, sleep, and follow-up on a workability index questionnaire and fitness test every three months over a year. The data were collected remotely from harvester machines and smartwatches between November 2019 and November 2020. The volunteers for the research project were identified via social media through a channel that is popular among CTL machine operators. From 60 applicants there were selected 14 operators. All the participants were male aged between 25 and 52, and they had 6–35 years of work experience. 13 operated mainly harvesters and one operated mainly a forwarder. Two participants were harvester contractors e.g. employers who participated closely in the daily operations, and the other 12 were employees from different companies. The attendees in the study group were interviewed, familiar with wearing a watch or a smartwatch, and they were interested in their overall health and workability, or they showed interest to improve their overall health and/or workability. Wearing smartwatches was completely optional, and it was acknowledged that participants could remove their watches any time they wanted, or even drop out from the follow-up completely. At the end of the follow-up, there were comprehensive productivity and smartwatch data from nine participants (operators A-I). The other five participants’ data were incomplete due to lack of using the smartwatch, change of work description or career path, sick leaves, and/or failures in the data delivery.
The smartwatch data
Participants were given smartwatches (Polar Ignite) which they wore for 12 months. During the monitoring study, participants’ daily activity (time), daily passivity (marks), daily steps (the number of steps), sleep duration (time), and physical exercise (the sport and time) data were collected. Daily activity time included all the awake time, and it was “active” if one did physical movements (e.g. walking or standing up) and inactive if one was sedentary (e.g., sitting). However, practice showed daily steps were heavily impacted by the trembles and vibrations of machines leading to an exaggeration of steps and underestimation of passivity marks, hence both had to be excluded from the final analysis. Polar Ignite recognized sleep according to the user’s physical movements when sleeping started and ended, and according to nightly movements, it divided sleep into a deep sleep, light sleep, rem-sleep, and interruptions. Due to the variety of personal sleeping habits and smartwatches’ uncertainty to collect different sleep stages successfully, the study included only total sleep time per night in the data.
Every 3 months participants performed the smartwatch’s fitness test that estimated the maximal oxygen uptake (VO2max) from heart rate at rest. The test was safe and uncomplicated as it demanded 5 min of rest/lying position to perform. The Polar Ignite smartwatch algorithm categorized the VO2max outcome into seven categories: 1) weak, 2) low, 3) satisfying, 4) average, 5) good, 6) very good, 7) top performance, and the outcome took into consideration users’ background information (weight, height, age, workout history, and gender). VO2max is a widely accepted measure of cardiorespiratory fitness, which has been shown to result in short-term and long-term benefits, both physiologically and psychologically. Small improvements in VO2max have been linked to significant improvements in cardiovascular disease (CVD) risk profile, and according to World Health Organization (WHO), CVDs are the leading cause of death globally. Many laboratory tests are used to determine VO2max, but wearables/smartwatches also have VO2max estimation algorithms that are user-friendly for consumers to evaluate their physical fitness.
The workability index
As workability is the balance between personal resources (sleep, physical fitness, and mental well-being) and work demands (productivity, shift work), alongside with fitness test participants completed modified workability index (WAI) questionnaires. WAI helps to answer the question of how well or healthy the employees are currently but also expected to be in the near future (Kettunen Citation2015) and it reiably predicts work disability, retirement, and mortality (Ilmarinen and Lehtinen Citation2004). A WAI questionnaire includes seven sections concerning employee’s 1) workability compared to the lifetime best, 2) workability in relation to work demands, 3) current medical diagnosed conditions, 4) disadvantages of the medical conditions related to work, 5) amount of sick days during the past 12 months, 6) employee’s personal prognosis of future workability, and 7) psychological resources (Rautio and Michelsen Citation2013). The outcome of the index varies between 7–49 points and the result of WAI is divided into four groups according to the final score: poor (7–27 points), moderate (28–36 points), good (37–43 points) and excellent (44–49 points). The WAI questionnaire used in this study was modified from the original questionnaire. The specific definitions of the possible medical diagnosed conditions (section 3) the machine operators suffered were left out since there was no need to know them and they didn’t affect the final score. Furthermore, working in relatively static work postures in the CTL machines, a question was added concerning pain, aches, or numbness in hands or any part(s) of the spine.
Productivity data and work characteristics
Most of the participants worked mainly in two shifts: morning (commonly from 6 a.m. to 2 p.m.) and evening (commonly from 2 p.m. to 10 p.m.). However, shift work and work tasks fluctuated as organizational work arrangements, (inter)national market situation, weather and seasons changed. Especially in smaller contractor businesses, it was common for harvester operators to operate forwarders from time to time for short periods and incorporate large maintenance work and/or machine transportation into their work time, thus their entire work time included tasks other than only productive machine work.
Participants operated machines from John Deere (3 harvesters), Ponsse (7 harvesters and 1 forwarder), and Komatsu (3 harvesters), and the machines were under 5 years old. Productivity data were in StanForD- or StanForD2010 formats, which are global standards for CTL machines used by all major machine manufacturers (Skogforsk Citation2013). The productivity data from John Deere and Komatsu harvesters was collected in .pdf and .xml formats and further converted/processed in excel-spreadsheets. Ponsse data were collected by using Ponsse’s fleet management application (OptiOffice) to read the harvesters’ files for time (drf-files) and production (prd-files) before further processing in excel-spreadsheets. Productivities were calculated as gross effective time productivity (E15), which includes delays shorter than 15 min. For longer interruptions, or e.g. tasks related to maintenance, the operator was required to manually register information to obtain accurate records of all scheduled work hours. However, this was not always correctly done resulting in some unproductive times that may have leaked into productive machine hours. To eliminate errors in the data and unusual harvesting sites, productivity data under 5 m3/h, and/or stem size smaller than 0.060 m3, was removed. Furthermore, the study accepted thinning stands and clear-cut stands but excluded some sites e.g. power line-, road line-, and plot sites from the final data. The total timber volume that was used in calculations and combined with smartwatch data were 152 745.5 m3 of which 60.3% was harvested from clear-cuttings and 39.7% from thinnings (). Felling methods e.g. thinnings and clear-cuttings weren’t divided evenly between operators as some operators had more thinnings and some more clear-cuttings due to their geographical positioning, machine type, or customer demands.
Table 1. The total amount of harvested timber in the study and its distribution between thinnings and clear-cuttings.
Data analysis
As the exact machine and employee productivity are sensitive data in Finnish business culture, and it was agreed with the participated contractors and participants that the actual productivity numbers are confidential, this study used relative calculations to evaluate and analyze productivity data. The level of productivity is presented as relative volumes compared to an arbitrarily selected volume, which was given a value of 100 (Malinen et al. Citation2018). The arbitrarily selected volume was 0.186 m3, which was the average stem size. The use of scaled values does not compromise the results of the study since the aim of the study was to investigate the changes in the productivity, not the actual level of productivity. For the comparisons of the operator’s productivity against modeled average productivity, the operator’s relative productivity (Pr) was calculated (equation 1) by dividing the stand and operator-specific actual productivity ratio (P0) by modeled productivity ratio (Pm, Malinen et al. Citation2018).
Where:
Pr relative productivity
P0 operators’ actual productivity ratio, m3%/E15
Pm modeled productivity ratio, m3%/E15
A nightly sleep value (SV) was estimated for every operator from the average sleep time on workdays the operator had. The SV was 1 if sleeping time was the same as the average sleeping time on workdays, below 1 if it was less, and above 1 if it was more.
Basic characteristics of the data were analyzed by the standard statistic parameters (mean, median, mode, standard deviation), and correlation were used to clarify content and possible relationship of dependences. Pr, WAI, VO2max, SV, and daily activity were evaluated through different background variables. Since the values did not show a normal distribution, the non-parametric Kruskal–Wallis test was used to evaluate variables. Furthermore, data were modeled by regression analysis though there weren’t statistical differences or relevance for regression models.
Results
Relative productivity (Pr)
The relative productivity (Pr) varied both for operators over time and between the operators from 0.75 to 1.47 ().
Overall, the Pearson’s correlation coefficient showed negligible or weak relationships between relative productivity (Pr), maximal oxygen uptake (VO2max), workability index (WAI), daily activity, sleep value (SV), and work shift ().
Table 2. Correlations (Pearson’s correlation coefficient) between relative productivity (Pr), workability index (WAI), sleep value (SV), maximal oxygen uptake (VO2max), and shift work.
Workability index (WAI)
At the beginning of the study and every 3 months, operators filled up the workability index (WAI) questionnaire (). In most cases, the WAI increased or decreased one unit or stayed stable during the study. Operator F had a visible drop in WAI due to multiple difficulties in health and work arrangements/organization. Furthermore, operator H’s working environment stayed stable but after the covid pandemic began his leisure time activities changed dramatically.
Figure 2. Workability indexes (WAI) of operators during the study. WAI questionnaires were filled at the beginning of the study (NOV), and every 3 months (FEB-NOV).

In the total data CTL machine operators’ relative productivity (Pr) had an increasing trend whilst the workability index (WAI) increased. Whilst the correlation didn’t show a strong relationship between Pr and WAI () but there was a statistical difference in Pr between WAI groups excellent and good (p < 0.000), and between WAI groups excellent and poor to moderate (p < 0.001). When the relative productivity was above average (Pr >1.1), the WAI values were higher compared to the relative productivity level under average (, p < 0.004).
Table 3. The workability index (WAI) when relative productivity (Pr) was above and under average.
Maximal oxygen uptake (VO2max)
At the beginning of the study and every 3 months operators performed the smartwatch’s fitness test that estimated the maximal oxygen uptake (VO2max) (). In most cases, the WAI and the VO2max increased or decreased one unit or stayed stable during the study. Participants’ G and H leisure time suffered dramatic change due to the covid pandemic beginning in February 2020.
Figure 3. Operators’ calculated maximal oxygen uptake (VO2max) during the study. Test was done in the beginning of the study (I) and every 3 months (II-V). VO2max categories 1) weak, 2) low, 3) satisfying, 4) average, 5) good, 6) very good, and 7) top performance.

As the correlation coefficient between VO2max and Pr was negative (−0.13) VO2max had irregular values whilst relative productivity increased. WAI had a slightly increasing trend for increasing VO2max groups (). Furthermore, there were statistical differences between VO2max groups in both Pr and WAI ().
Figure 4. Kruskal-Wallis -test showed difference in relative productivity (Pr) and workability index (WAI) among VO2max groups. VO2max groups 1) weak, 2) low, 3) satisfying, 4) average, and 5) good.

Table 4. Statistical differences between VO2max groups (1) weak, 2) low, 3) satisfying, 4) average, and 5) good) in relative productivity (Pr), and workability index (WAI).
Sleep value (SV) and activity time
The participants’ (A-I) average sleep time on workdays varied from 5 h 56 min to 7 h 6 min, and their average time of daily activity on workdays varied from 7 h 45 min to 8 h 33 min (). It was not possible to gather reliable activity data from operators A and I since their harvester machine vibration caused significant additional activity time to the smartwatch affecting the activity level.
Figure 5. The study participants’(A-I) average activity time, average non-active waking hours, and average sleep time on workdays. Operators A and I activity data had significant additional activity from the harvester vibration and weren’t reliable.

Since the need for sufficient sleep varies, individual personal sleep values (SV) were calculated according to one’s average sleep time during workdays. When the operators’ SV was under 1, the average Pr was lower than when SV was 1 or above (), but there wasn’t a statistical difference (p = 0.073).
Table 5. Relative productivity (Pr) when sleep value (SV) is under or above 1.
Participants with poor or moderate workability index slept more often irregularly while participants with excellent WAI had more stable sleep rhythms closer to their average sleep time. Consequently, SV among participants with poor or moderate WAI was lower (mode = 0.79, SD = 0.20) than among participants with excellent WAI (mode = 0.88, SD = 0.16, ). In the overall data sleep variation between WAI, groups didn’t have statistical significance (p > 0.05).
Table 6. Sleep values between workability groups excellent, and poor and moderate.
Every 3 months participants performed the fitness test resulting in VO2max values categorized from 1 to 5: 1) weak, 2) low, 3) satisfying, 4) average, and 5) good. In most cases, the participant’s VO2max category remained the same for the whole 12 months or shifted one category up or down. However, there was a statistical difference in SV between the weak VO2max group (1) and all the other groups (p < 0.000, ). Between groups 2, 3, 4, and 5 there were no statistical differences.
Shift work and the seasons
There was no difference in the SV, WAI, or VO2max between summer and winter. However, there was a difference in the Pr between the summer and winter seasons (). Consequently, the average Pr was greater in the summer (1.09) than in the winter season (0.98) (p < 0.000). Naturally, several environmental variables affect operational performance between seasons.
Figure 7. Relative productivity ratio (m3%/E15) during the light (summer) and the dark (winter) season.

Of the 14 participants 11 worked in two shifts on a regular basis (morning shift from 6 a.m. to 2 p.m. and evening shift from 2 p.m. to 10 p.m.). In the evening shift, participants slept longer than in the morning shift, sleep values were 1.09 and 0.94, respectively () with the statistical difference (p < 0.000).
Figure 8. Participants observed sleep values (SV) in the morning and evening work shift. In the morning and evening shift average SV was 0.94 and 1.09, respectively.

Sleep value correlation with Pr was 0.04 (), but SV had a slightly increasing impact in the winter season. However, compared to the felling method, an increase in sleep increased productivity on clear cuttings, especially in the evening shift ().
Discussion
Participants’ relative productivity (Pr) increased when the workability index (WAI) was excellent. The workability can be described as the balance between the employee’s personal resources and the work demands (Ilmarinen Citation2009), and in this study sleep and physical fitness can be classified as personal resources and shift work as one of the work demands. Operators’ connection between excellent WAI and higher Pr was in line with earlier studies where high WAI results in higher work productivity (e.g. Kuoppala et al. Citation2008; Vänni et al. Citation2015; Ahola et al. Citation2018) thus WAI seems to work well also for forestry work applications. Good WAI results in higher quality of work and the enjoyment of staying in one’s job (Tuomi et al. Citation2001). As the nature of harvesting work involves alternating nature environments leading to minor daily operational problems and decision-making, high WAI among operators is implying they are more likely to solve daily operational problems in order to improve work quality and fluency, and thus indirectly productivity.
Improvements in maximum oxygen uptake (VO2max) are generally associated with better physical and mental health (Warburton et al. Citation2006; Ströhle Citation2009; Miller et al. Citation2016; Korge and Nunan Citation2018), and further physical health seems to correlate with work performance and productivity (Burton et al. Citation2005; Mills et al. Citation2007; Kumar et al. Citation2009), hence there are controversial results to productivity as well (Bernaards et al. Citation2007). In this study, VO2max was estimated with the smartwatch’s fitness test. Accuracy of wearables has shown variation between different devices demonstrating a 9–30 % variation when evaluating accuracy on physical activity, heart rate, and energy consumption (Lee et al. Citation2014; Yetisen et al. Citation2018). More promising results showed concerning estimating the maximal oxygen uptake (VO2max) and heart rate variability (Esco et al. Citation2011; Hernando et al. Citation2018; Cooper and Shafer Citation2019) whereas Molina-Garcia et al. (Citation2022) concluded that wearables using resting condition information overestimate VO2max (bias 2.17 ml∙kg−1∙min−1) compared to exercise-based algorithms accuracy (bias −0.09 ml∙kg−1∙min−1ml). Also, forest work’s robust and rugged environment and methods set limitations and challenges for the technology that is used in measuring worker’s health (Bowen et al. Citation2015; Hinze et al. Citation2022). While manual forest work is a risk for the actual wearable device, the fully mechanized harvesting aggravates e.g. the activity estimation, which occurred in this study as well.
In the current study, VO2max didn’t seem to have a connection with the Pr, and WAI had only a slightly increasing trend when VO2max increased. Fully mechanized CTL harvesting operational work includes prolonged periods of sitting and operational performance doesn’t require immediate physical exertion thus temporary operational performance could be excellent regardless of VO2max. On the other hand, there is more or less constant vibration from the machine that the body is forced to adapt, and occasionally physical efforts may be needed if there are technical problems in the machine. Reduced VO2max and its influence on operational performance would probably demand several years or even decades of follow-up studies to demonstrate the possible connection. However, the operators’ VO2max had a decreasing trend toward the end of the study and simultaneously the two first waves of covid rushed through society, and various degrees of lockdowns restrained people during the year 2020.
The human circadian clock is predominantly entrained by sun time rather than by social time (Roenneberg et al. Citation2007; Gibson and Shrader Citation2018). However, due to work and school scheduling workers have to deal with morning coordinator constraints (Hamermesh et al. Citation2008; Jagnani Citation2022) resulting in decreased sleep duration, both intra-annually and in the long run (Hubert et al. Citation1998; Gibson and Shrader Citation2018). In this study, there were no signs of increased or decreased sleep time during the longitudinal follow-up. However, there were differences in sleep time between morning and evening shifts. Work shifts cycled on alternate weeks and participants tended to sleep longer in the evening shift than in the morning shift indicating early morning shifts interrupt and shorten natural sleep duration. On weekends participants slept longer than during workdays, but as it takes even several days to recover from sleep deficit (Kitamura et al. Citation2016) part of the debt may pass on to the following (evening shift) work week.
Operators’ relative productivity was higher in the light summer than in the dark winter. Nicholls et al. (Citation2004) reported that poor light in forest operations reduced visibility for night work and affected log making and sorting decisions, and operators found shadowing and glare inhibited visibility and precision of machine positioning reducing productivity. Lighting technology has improved since early 2000 (e.g. LED lights) resulting in fairly good working visibility. However, work planning in dark conditions (e.g. outlining strip roads) is still challenging, and rainfall, fog, or snowfall hinder the luminosity and visibility, especially in the dark. Light conditions are a vital factor in harvesting work but as the working environment changes a great deal in Nordic countries between summer and winter, there are many factors in addition to lighting influencing productivity between seasons (snow, ground frost, temperature). Strictly from the productivity point of view, the light summer season is more productive than the dark season, which implies harvesting work is more profitable when carried out during the summer season. However, the balance between work and leisure time is significant for overall health and workability, and the whole forest industry’s longer periods of holidays and service breaks tend to take place in the light season let alone many harvesting sites in the Nordics require ground frost for successful operations. Also, it is expected that climate change is increasing precipitation in Nordic countries impeding summer loggings by the decreased load-carrying capacity.
In this study operators in the evening shift with higher sleep value (SV) increased relative productivity (Pr) in clear cuttings. Häggström et al. (Citation2015) found out through an eye-tracking study that harvester operators looked more frequently at the canopy, the falling trees, and the monitor during second thinnings and final fellings than during first thinnings. Thus, being fresh and well rested would ease and enhance performing demanding work as final felling demands higher concentration and accuracy than first thinnings (in this study first and second thinnings weren’t separated), and the evening shift is more likely hindered by the darkness that requires more concentration to observe this environment.
The data used in this study were unique, and its longitudinal nature provided additional value and knowledge to the timber harvesting sector from the operational ground level. The amount of data collected decreased during the longitudinal follow-up due to unpredicted career changes and failure to keep up with some of the participants in the study.Significant differences were found in the data between Pr and studied factors (WAI, VO2max, SV, seasons, and shift work), thus beginning to reveal relationships between lifestyle habits, environmental surroundings, and Pr, although the study didn’t create general productivity models. Over the period of data collection, the timber logging sector in Finland suffered several exceptional circumstances including a delayed and extremely poor winter season, as well as the forest industry’s industrial actions that impeded logging. The worldwide covid pandemic also reached Finland February in 2020 which didn’t have a direct affect on logging but undisputedly caused changes and uncertainty at the organizational level, lockdown in society, and affected mentally. Finally, the forest as a varying working environment combined with the diverse issue of workability and well-being creates a context of a complex research area that could lead easily to oversimplifications and misinterpretations. By carefully understanding the nature of harvesting work, operational performance, workability, well-being, and providing long-term monitoring, the study comprehended risks that complex issues undoubtedly contain. For future studies it would be useful to succeed in larger data gathering during more stable circumstances in society. Also, new applications of decision support for CTL machines have been introduced, and it would be important to consider these when evaluating workability and the work demands in the future.
Conclusions
This study contributes to the understanding of the productivity of forest harvesting by acknowledging workability and well-being as key factors. Overall, it’s important to understand the human factor and its contribution to operational performance as well as the operational work demands on the human being. Workability supports productivity, and workability relies greatly on recovery, sleep, and sufficient physical fitness. Supporting employees’ workability ensures good operational performance, which is reliant on motivated and healthy operators.
Employees’ sleep time, sleep timing, and recovery are often defined by the work shifts, hence careful shift planning secures decent sleep time and recovery. After busy peak periods (such as the ground frost period) there should be room for recovery. This provides sustainability in long-term productivity and continuity in the localand globally competitive business environment. Furthermore, recognizing and understanding sleep patterns and recovery needs support adjustment of the behavior to the work demands.
Further research is needed to create productivity models under varying circumstances and clarify the different individual elements behind the human factor. As new decision support systems are introduced and developed for harvester machines, these should be included when evaluating workability and future work demands. Also, research is needed to assess what kind of support systems would benefit the operators the most without decreasing workability and operational performance, and without increasing work’s complexity and the unnecessary overflow of information.
Acknowledgements
We are grateful for the Academy of Finland Flagship Programme for financial support via Forest-Human-Machine Interplay – Building Resilience, Redefining Value Networks and Enabling Meaningful Experiences (UNITE) with decision number 337127. The authors thank Metsämiesten Säätiö Foundation for financial support. Furthermore, the authors are grateful for all the volunteers and their priceless commitment to the study group, and for their employers for providing the productivity data.
Disclosure statement
No potential conflict of interest was reported by the authors.
Additional information
Funding
References
- Aalmo GO, Magagnotti N, Spinelli R. Forest workers and steep terrain winching: the impact of environmental and anthropometric parameters on performance. Croat J For Eng. 2016;37(1):97.
- Ahola S, Eskelinen J, Heikkilä-Tammi K, Kuula M, Larjovuori R-L, Nuutinen S. 2018. Digisti työn imuun? - Tutkimus työhyvinvoinnin ja tuottavuuden yhteydestä finanssialan palveluyrityksessä; Digital path to work engagement? Study of the linkage between employee well-being and productivity in a financial service company. Aalto University; Aalto-yliopisto. urn: http://urn.fi/URN:ISBN:978-952-60-8262-2.
- Apǎfǎian AI, Proto AR, Borz SA. 2017. Performance of a mid-sized harvester-forwarder system in integrated harvesting of sawmill, pulpwood and firewood. Ann For Res. 60(2):227–241. doi:10.15287/afr.2017.909.
- Arlinger J, Möller JJ, Sorsa J. 2010. StanForD 2010 –. Uppsala, Sweden: Skogforsk. pp. 1–16.
- Arvidson E, Börjesson M, Ahlborg G, Lindegard A, Jonsdottir IH. 2013. The level of leisure time physical activity is associated with work ability-a cross sectional and prospective study of health care workers. BMC Public Health. 13(855): https://gup.ub.gu.se/publication/185448
- Asikainen A, Leskinen LA, Pasanen K, Väätäinen K, Anttila P, Tahvanainen T. 2009. Metsäkonesektorin nykytila ja tulevaisuus. Vantaa: Working papers of the Finnish Forest Research Institute. 125. http://www.metla.fi/julkaisut/workingpapers/
- Bartrim K, McCarthy B, McCartney D, Grant G, Desbrow B, Irwin C. 2020. Three consecutive nights of sleep loss: effects of morning caffeine consumption on subjective sleepiness/alertness, reaction time and simulated driving performance. Transp Res Part F Traffic Psychol Behav. 70:124–134. doi:10.1016/j.trf.2020.02.017.
- Bergman E, Löyttyniemi E, Myllyntausta S, Rautava P, Korhonen PE. 2020. Factors associated with quality of life and work ability among Finnish municipal employees: a cross-sectional study. BMJ Open. 10(9):e035544. doi:10.1136/bmjopen-2019-035544.
- Bernaards CM, Proper KI, Hildebrandt VH. 2007. Physical activity, cardiorespiratory fitness, and body mass index in relationship to work productivity and sickness absence in computer workers with preexisting neck and upper limb symptoms. J Occup Environ Med. 49(6):633–640. doi:10.1097/JOM.0b013e318058202c.
- Borz SA, Talagai N, Cheţa M, Chiriloiu D, Gavilanes Montoya AV, Castillo Vizuete DD, Marcu MV. 2019. Physical strain, exposure to noise and postural assessment in motor-manual felling of willow short rotation coppice. Croat J For Eng. 40(2):377–388. https://hrcak.srce.hr/228256
- Bowen J, Hinze A, Cunningham SJ, Parker R. 2015. Evaluating low-cost activity trackers for use in large-scale data gathering of forestry workers. OzChi 2015 Being Hum - Conf Proc. 474–482. doi:10.1145/2838739.2838741.
- Bowen J, Hinze A, Griffiths C. 2019. Investigating real-time monitoring of fatigue indicators of New Zealand forestry workers. Accid Anal Prev. 126(August 2017):122–141. doi:10.1016/j.aap.2017.12.010.
- Burdorf A, Frings-Dresen MHW, van DC, Elders LAM. 2005. Development of a decision model to identify workers at risk of long-term disability in the construction industry. Scand J Work Environ Health. 31(2):31–36. https://www.airitilibrary.com/Publication/alDetailedMesh?DocID=03553140-200512-201011100061-201011100061-31-36
- Burton WN, McCalister KT, Chen CY, Edington DW. 2005. The association of health status, worksite fitness center participation, and two measures of productivity. J Occup Environ Med. 47(4):343–351. doi:10.1097/01.jom.0000158719.57957.c6.
- Camerino D, Conway PM, Sartori S, Campanini P, Estryn-Béhar M, van der Heijden BIJM, Costa G. 2008. Factors affecting work ability in day and shift-working nurses. Chronobiol Int. 25(2–3):425–442. http://www.tandfonline.com/doi/abs/10.1080/07420520802118236
- Cooper KD, Shafer AB. 2019. Validity and reliability of the polar A300’s fitness test feature to predict VO2max. Int J Exerc Sci. 12(4):393–401. https://www.ncbi.nlm.nih.gov/pubmed/30899351
- Declercq I, Van Den Eede F, Roelant E, Verbraecken J. 2022. SHIFTPLAN: a randomized controlled trial investigating the effects of a multimodal shift-work intervention on drivers’ fatigue, sleep, health, and performance parameters. Trials. 23(1):1–18. doi:10.1186/s13063-022-06573-6.
- Depner CM, Cheng PC, Devine JK, Khosla S, de Zambotti M, Robillard R, Vakulin A, Drummond SPA. 2019. Wearable technologies for developing sleep and circadian biomarkers: a summary of workshop discussionsSleep (New York, NY)432. https://search.datacite.org/works/10.1093/sleep/zsz254
- Drake CL, Roehrs T, Richardson G, Walsh JK, Roth T. 2004. Shift work sleep disorder: prevalence and consequences beyond that of symptomatic day workers. Sleep. 27(8):1453–1462. doi:10.1093/sleep/27.8.1453.
- Eldevik MF, Flo E, Moen BE, Pallesen S, Bjorvatn B, Gamble KL. 2013. Insomnia, excessive sleepiness, excessive fatigue, anxiety, depression and shift work disorder in nurses having less than 11 hours in-between shifts. PLos One. 8(8):e70882. doi:10.1371/journal.pone.0070882.
- Eriksson M, Lindroos O. 2014. Productivity of harvesters and forwarders in CTL operations in northern Sweden based on large follow-up datasets. Int J For Eng. 25(3):179–200. https://search.datacite.org/works/10.1080/14942119.2014.974309
- Esco MR, Mugu EM, Williford HN, McHugh NA, Bloomquist EB. Cross-validation of the polar fitness test via the polar F11 heart rate monitor in predicting VO2Max. J Ecercise Physiol. 2011;14(5):31–37.
- Gellerstedt S. 2013. Operation of the single-grip harvester: motor-sensory and cognitive work. Int J For Eng. 13(2):35–47.
- Gibson M, Shrader J. 2018. Time use and labor productivity: the returns to sleep. Rev Econ Stat. 100(5):783–798. doi:10.1162/rest_a_00746.
- Gingerich SB, Seaverson ELD, Anderson DR. 2018. Association between sleep and productivity loss among 598 676 employees from multiple industries. Am J Heal Promot. 32(4):1091–1094.
- Grzywiński W, Jelonek T, Tomczak A, Jakubowski M, Bembenek M. 2017. Does body posture during tree felling influence the physiological load of a chainsaw operator? Ann Agric Environ Med. 24(3):401–405. https://www.ncbi.nlm.nih.gov/pubmed/28954479
- Häggström C, Englund M, Lindroos O. 2015. Examining the gaze behaviors of harvester operators: an eye-tracking study. Int J For Eng. 26(2):96–113. doi:10.1080/14942119.2015.1075793.
- Häggström C, Lindroos O. 2016. Human, technology, organization and environment – a human factors perspective on performance in forest harvesting. Int J For Eng. 27(2):1–12. doi:10.1080/14942119.2016.117049510.1080/14942119.2016.1170495.
- Hamermesh DS, Myers CK, Pocock ML. 2008. Cues for timing and coordination: latitude, letterman, and longitude. J Labor Econ. 26(2):223–246. doi:10.1086/525027.
- Henriksen A, Haugen Mikalsen M, Woldaregay AZ, Muzny M, Hartvigsen G, Hopstock LA, Grimsgaard S. 2018. Using fitness trackers and smartwatches to measure physical activity in research: analysis of consumer wrist-worn wearables. J Med Internet Res. 20(3):e110. https://search.datacite.org/works/10.2196/jmir.9157
- Hernando D, Roca S, Sancho J, Á A, Bailón R. 2018. Validation of the apple watch for heart rate variability measurements during relax and mental stress in healthy subjects. Sensors (Basel). 18(8):2619. https://search.datacite.org/works/10.3390/s18082619
- Hinze A, Bowen J, König JL. 2022. Wearable technology for hazardous remote environments: smart shirt and Rugged IoT network for forestry worker health. Smart Heal. 23(July 2021):100225. doi:10.1016/j.smhl.2021.10022510.1016/j.smhl.2021.100225.
- Hubert M, Dumont M, Paquet J. 1998. Seasonal and diurnal patterns of human illumination under natural conditions. Chronobiol Int. 15(1):59–70. doi:10.3109/07420529808998670.
- Ilmarinen J, Joyner KH, Fleming AH. 1991. Implanted medical devices in workers exposed to radio-frequency radiation. Scand J Work Environ Health. 17(1):1–141. doi:10.5271/sjweh.1740.
- Ilmarinen J. 2009. Work ability-a comprehensive concept for occupational health research and prevention. Scand J Work Environ Health. 35(1):1–5. https://www.airitilibrary.com/Publication/alDetailedMesh?DocID=03553140-200901-201011040123-201011040123-1-5
- Ilmarinen J, Lehtinen S. 2004. Past, present and future of work ability: proceedings of the 1st international symposium on work ability: 5-6 september 2001 Tampere, Finland. Ilmarinen J, Lehtinen S, editors. Helsinki: Finnish Institute of Occupational Health (People and work, Research reports, 65).
- Jagnani M. 2022. Children‘s Sleep and Human Capital Production The Review of Economics and Statistics. doi:10.1162/rest_a_01201.
- Jiroušek R, Klvač R, Skoupý A. 2008. Productivity and costs of the mechanised cut-to-length wood harvesting system in clear-felling operations. J For Sci. 53(No. 10):476–482.
- Katz AS, Pronk NP, Lowry M. 2014. The association between optimal lifestyle-related health behaviors and employee productivity. J Occup Environ Med. 56(7):708–713. doi:10.1097/JOM.0000000000000191.
- Kemmerer J, Labelle ER. 2021. Using harvester data from on-board computers: a review of key findings, opportunities and challenges. Eur J For Res. 140(1):1–17. doi:10.1007/s10342-020-01313-410.1007/s10342-020-01313-4.
- Kettunen O. 2015. Effects of physical acitivity and fitness on the psychological wellbeing of young men and working adults: associations with stress, mental resources, overweight and workability. University of Turku.
- Kitamura S, Katayose Y, Nakazaki K, Motomura Y, Oba K, Katsunuma R, Terasawa Y, Enomoto M, Moriguchi Y, Hida A, et al. 2016. Estimating individual optimal sleep duration and potential sleep debt. null. 6(1):1–10. doi:10.1038/srep35812.
- Korge J, Nunan D. 2018. Higher participation in physical activity is associated with less use of inpatient mental health services: a cross-sectional study. Psychiatry Res. 259(August 2017):550–553.
- Kumar S, McCalla M, Lybeck E. 2009. Operational impact of employee wellness programs: a business case study. Int J Product Perform Manag. 58(6):581–597. doi:10.1108/17410400910977109.
- Kuoppala J, Lamminpää A, Husman P. 2008. Work health promotion, job well-being, and sickness absences—a systematic review and meta-analysis. J Occup Environ Med. 50(11):1216–1227. https://search.datacite.org/works/10.1097/jom.0b013e31818dbf92
- Kymäläinen H, Laitila J, Väätäinen K, Malinen J. 2021. Workability and well-being at work among cut-to-length forest machine operators. Croat J For Eng. 42(3):405–417. doi:10.5552/crojfe.2021.874.
- Larrichia F. 2022. Wearables - statistics & facts. Statista. https://www.statista.com/topics/1556/wearable-tech.https://www.statista.com/topics/1556/wearable-technology/#dossierKeyfigures.
- Lee J-M, Kim Y, Welk GJ. 2014. Validity of consumer-based physical activity monitors. Vol. 46. doi:10.1249/MSS.0000000000000287.
- Leszczyński K, Stańczykiewicz A. 2015. Workload analysis in logging technology employing a processor aggregated with a farm tractor. For Syst. 24(2):e024. https://explore.openaire.eu/search/publication?articleId=dedup_wf_001:ce89465895e64754d30359624db6f784
- Lian Y, Xiao J, Liu Y, Ning L, Guan S, Ge H, Li F, Liu J. 2014. Associations between insomnia, sleep duration and poor work ability. J Psychosom Res. 78(1):45–51. https://www.clinicalkey.es/playcontent/1-s2.0-S0022399914003365
- Liski E, Jounela P, Korpunen H, Sosa A, Lindroos O, Jylhä P. 2020. Modeling the productivity of mechanized CTL harvesting with statistical machine learning methods. Int J For Eng. 31(3):253–262.
- Lundin A, Lundin A, Kjellberg K, Kjellberg K, Leijon O, Leijon O, Punnett L, Punnett L, Hemmingsson T, Hemmingsson T. 2016. The association between self-assessed future work ability and long-term sickness absence, disability pension and unemployment in a general working population: a 7-year follow-up study. J Occup Rehabil. 26(2):195–203. https://www.ncbi.nlm.nih.gov/pubmed/26319413
- Malinen J, Taskinen J, Tolppa T. 2018. Productivity of cut-to-length harvesting by operators’ age and experience. Šumarski fakultet Sveučilišta u Zagrebu. https://hrcak.srce.hr/193546
- Maturo A, Setiffi F. 2016. The gamification of risk: how health apps foster self-confidence and why this is not enough. Health Risk Soc. 17(7–8):477–494. https://search.datacite.org/works/10.1080/13698575.2015.1136599
- Miller KR, McClave SA, Jampolis MB, Hurt RT, Krueger K, Landes S, Collier B. 2016. The health benefits of exercise and physical activity. Curr Nutr Rep. 5(3):204–212. doi:10.1007/s13668-016-0175-5.
- Mills PR, Kessler RC, Cooper J, Sullivan S. 2007. Impact of a health promotion program on employee health risks and work productivity. Am J Heal Promot. 22(1):45–53. doi:10.4278/0890-1171-22.1.45.
- Molina-Garcia P, Notbohm HL, Schumann M, Argent R, Hetherington-Rauth M, Stang J, Bloch W, Cheng S, Ekelund U, Sardinha LB, et al. 2022. Validity of estimating the maximal oxygen consumption by consumer wearables: a systematic review with meta-analysis and expert statement of the INTERLIVE network. null. 52(7):1577–1597. doi:10.1007/s40279-021-01639-y10.1007/s40279-021-01639-y.
- Murphy G, Marshall H, Dick A. 2014. Time of day impacts on machine productivity and value recovery in an off-forest central processing yard. New Zeal J For Sci. 44(1):1–19. https://search.datacite.org/works/10.1186/s40490-014-0019-2
- Nicholls A, Bren L, Humphreys N. 2004. Harvester productivity and operator fatigue: working extended hours. Int J For Eng. 15(2):57–65.
- Oellingrath IM, De Bortoli MM, Svendsen MV, Fell AKM. 2019. Lifestyle and work ability in a general working population in Norway: a cross-sectional study. BMJ Open. 9(4):e026215. doi:10.1136/bmjopen-2018-026215.
- Olivera A, Visser R, Acuna M, Morgenroth J. 2016. Automatic GNSS-enabled harvester data collection as a tool to evaluate factors affecting harvester productivity in a Eucalyptus spp. harvesting operation in Uruguay. Int J For Eng. 27(1):15–28. http://www.tandfonline.com/doi/abs/10.1080/14942119.2015.1099775
- Ottaviani Aalmo G, Kerstens PJ, Belbo H, Bogetoft P, Talbot B, Strange N. 2020. Efficiency drivers in harvesting operations in mixed Boreal stands: a Norwegian case study. Int J For Eng Ahead-Of-Print(ahead-Of-Print). 1–13.
- Ovaskainen H, Uusitalo J, Väätäinen K. 2004. Characteristics and significance of a harvester operators’ working technique in thinnings. Int J For Eng. 15(2):67–77.
- Pasicott P, Murphy G. 2013. Effect of work schedule design on productivity of mechanised harvesting operations in Chile. New Zeal J For Sci. 43(1):1–10. https://natlib-primo.hosted.exlibrisgroup.com/primo-explore/search?query=any,contains,997751693602837&tab=innz&search_scope=INNZ&vid=NLNZ&offset=0
- Purfürst FT. 2010. Learning curves of harvester operators. Šumarski fakultet Sveučilišta u Zagrebu. https://hrcak.srce.hr/63720.
- Purfürst T, Erler J. 2011. The human influence on productivity in harvester operations. Int J For Eng. 22(2):15–22. https://www.tandfonline.com/doi/citedby/10.1080/14942119.2011.10702606
- Rautio M, Michelsen T. 2013. WAI: how to use the work ability index questionnaire. 2nd ed. Helsinki, Finland: TTL (Finnish Institute of Occupational Health).
- Roenneberg T, Kumar CJ, Merrow M. 2007. The human circadian clock entrains to sun time. Curr Biol. 17(2):R44–45. doi:10.1016/j.cub.2006.12.011.
- Rosekind MR, Gregory KB, Mallis MM, Brandt SL, Seal B, Lerner D. 2010. The cost of poor sleep: workplace productivity loss and associated costs. J Occup Environ Med. 52(1):91–98. doi:10.1097/JOM.0b013e3181c78c30.
- Sell L, Bultmann U, Rugulies R, Villadsen E, Faber A, Sogaard K. 2009. Predicting long-term sickness absence and early retirement pension from self-reported work ability. Int Arch Occup Environ Health. 82(9):1133–1138. https://www.narcis.nl/publication/RecordID/oai:pure.rug.nl:publications%2F11bd1c60-c128-4c90-945a-1791ab58aee8
- Sirén M. 1998. One-grip harvester operation, it‘s silvicultural result and possibilities to predict tree damage. Metsantutkimuslaitoksen tiedonantoja 694. Metsantutkimuslaitos.
- Skogforsk. 2013. StanForD 2010 modern communication with forest machines.
- Spinelli R, Magagnotti N, Labelle ER. 2020. The effect of new silvicultural trends on mental workload of harvester operators. Croat J For Eng. 41(2):177–190.
- Ströhle A. 2009. Physical activity, exercise, depression and anxiety disorders. J Neural Transm. 116(6):777–784. doi:10.1007/s00702-008-0092-x.
- Tuomi K. 1997. Eleven-year follow-up of aging workers. Scand J Work Environ Health. 23(suppl 1):7–11.
- Tuomi K, Huuhtanen P, Nykyri E, Ilmarinen J. 2001. Promotion of work ability, the quality of work and retirement. Occup Med. 51(5):318–324.
- Vänni K, Virtanen P, Luukkaala T, Nygård C-H. 2015. Relationship between perceived work ability and productivity loss. Int J Occup Saf Ergon. 18(3):299–309. https://search.datacite.org/works/10.1080/10803548.2012.11076946
- van der Berg T, Elders L, de Zwart B, Burdorf A. 2008. The effects of work-related and individual factors on the work ability index: a systematic review. 66(4):211–220. doi:10.1136/oem.2008.039883.
- Visser R, Spinelli R. 2017. Determining the shape of the productivity function for mechanized felling and felling-processing. J For Res. 17(5):397–402. https://search.datacite.org/works/10.1007/s10310-011-0313-2
- Warburton DER, Nicol CW, Bredin SSD. 2006. Health benefits of physical activity: the evidence. Cmaj. 174(6):801–809. doi:10.1503/cmaj.051351.
- Whitson JR. 2013. Gaming the quantified self. Surveill Soc. 11(1/2):163–176. https://search.datacite.org/works/10.24908/ss.v11i1/2.4454
- Yetisen AK, Martinez-Hurtado JL, Ünal B, Khademhosseini A, Butt H. 2018. Wearables in medicine. Adv Mater. 30(33):1706910–n/a.