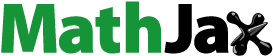
ABSTRACT
The paper uses firm-level data to examine gender wage and productivity gaps in the Ethiopian manufacturing sector for the period 1996–2010. It investigates gender wage differentials between skilled and unskilled workers after controlling for factors affecting average wages. Our findings show significant gender wage and productivity gaps and the segregation of female workers into low-paying firms. Controlling for average productivity reduces the magnitude of the wage gap but does not eliminate it. However, results using a simultaneaous estimation of wage equation and production function at a firm-level indicate no significant difference between the gender wage and productivity gaps. As such, further investigation into the sources of the dual gaps using matched employer-employee data is necessary. The Ethiopian government should focus on skill development to improve the productivity of female workers and address the segregation of female workers into low-paying firms.
1. Introduction
The unequal treatment of men and women in the workplace, mainly in terms of wages and employment has attracted the attention of policymakers, politicians, and economists worldwide. The employment and earnings gap between men and women in the labor market have been found to be a result of discrimination of female workers by employers or differences in the level of human capital among other factors (Blau & Khan, Citation2000). Klasen and Lamanna (Citation2009) showed that gender education and employment gaps have a negative effect on economic growth. Leopold, Ratcheva, and Tyson (Citation2016) argued that the current global economic gender gap will not be closed for another 170 years if the current rate of change is maintained. Without doubt, the elimination of gender gap may improve economic efficiency and economic growth (Appleton, Hoddinott, & Krishnan, Citation1999). To move towards gender equality worldwide, the United Nations oversaw the adoption of the 2030 Agenda for Sustainable Development (ASD) in 2015. Gender equality was included among the 17 sustainable development goals adopted by the 193 United Nations General Assembly countries. Among the main reasons for the adoption of the 2030 ASD is that gender inequality continues to be a major issue in developing countries, particularly in Africa, despite earlier efforts to narrow the problem by the year 2015 under the Millennium Development Goals.
This study aims to contribute to the existing literature by investigating gender wage and productivity gaps in the Ethiopian manufacturing sector. In addition, the study investigates gender and skill wage differentials after controlling for the factors affecting average wages in the sector. Existing studies using Ethiopian data (Appleton et al., Citation1999; Fafchamps, Söderbom, & Benhassine, Citation2009; Kolev & Robles, Citation2010; Temesgen, Citation2006) and other sub-Saharan Africa data (except for the work by Agesa, Citation1999 for Kenya; Abegaz & Nene, Citation2018 for Ghana) did not consider the interplay between productivity and wage gaps in one framework and the contribution of the former into the latter. We employ two approaches to address the issue of gender wage and productivity gaps: first, we adopt a reduced-form wage-setting equation at the firm-level with a control for productivity proposed by Bartolucci (Citation2014). We estimate the wage equation with and without firm fixed effects to investigate issues of labor market segregation and to identify the within firm wage gaps. This approach does not assume perfect competition in the labor market. Second, we employ the Hellerstein, Neumark, and Troske (Citation1999) approach in which firm level wage and production equations are estimated simultaneously. Statistical tests are conducted to evaluate the equality of estimated coefficients across the two equations and determine whether wage gaps can be explained by productivity gaps.
There are two reasons behind our choice to focus on the manufacturing industry in the current study. The first reason is that the Ethiopia’s manufacturing sector has been growing significantly and it is becoming one of the country’s most important sectors. The manufacturing sector, which accounts for about 4.3% of the national output, grew at a rate of about 12% per year for the period 2006–2015. The total employment in this sector grew by more than 400% from less than 40,000 workers to a little over 200,000 workers between 2002 and 2014. The second reason for focusing on this sector has to do with the availability of data which makes the capture of pay gaps due to gender and skill differentials possible.
Our empirical results confirm the presence of significant gender wage and productivity differentials. Estimates of the average wage equation using OLS show higher wage gaps than the estimates using the fixed effects model, suggesting segregation of female workers into low-wage firms. The inclusion of labor productivity and other firm characteristics accounts for a small portion of the gender wage gap. Although the firm-level wage gap unexplained by average productivity, firm characteristics, and firm fixed effects imply presence of within firm wage discrimination, further investigation employing matched employer-employee data is required to make such determination. In contrast, statistical tests addressing equality of female to male (female–male) wage and productivity ratios using the Hellerstein et al. (Citation1999) approach show no statistically significant difference on the two gaps after controlling for sector and region fixed effects. Such mixed results were also reported in Bartolucci (Citation2014) where the reduced-form wage-setting equation model showed evidence of wage discrimination against immigrant workers whereas results using the Hellerstein et al. (Citation1999) approach indicated no wage discrimination against immigrants. Lastly, we observe that firms that are globally connected through exporting and foreign ownership offer wage premiums for their workers but play little or no role to narrow the gender wage gap.
The rest of the study is organized as follows. In the next section, we outline the empirical evidence on wage and productivity gaps in Ethiopia and sub-Saharan Africa and identify the gap in the literature that the current study contributes to. Section 3 documents the data and empirical strategy. Section 4 discusses the results and section 5 provides concluding remarks.
2. Empirical evidence on sub-Saharan Africa and Ethiopia
Many developing countries have adopted policies to help reduce gender inequality. A case in point is Ethiopia, an East African state that has made important improvements toward universal primary education, gender equality and women empowerment (Kolev & Robles, Citation2010; Ogato, Citation2013). In addition, several higher education reforms were passed with the goal of reducing education disparities across gender lines (Molla, Citation2013). Reforms in family law and land registration were also implemented to improve the position of women in marriage, divorce, land ownership, paid employment, and related legal and socio-economic aspects (Hallward-Driemeier & Gajigo, Citation2015; Kumar & Quisumbing, Citation2015).
Despite the efforts to reduce the gender gap in education, employment, income, and political and economic participations, large inequalities continue to exist in Ethiopia. According to the World Economic Forum (WEF), Ethiopia ranked 100 (out of 115 countries) and 109 (out of 144 countries) with a gender gap index of 0.60 and 0.66 in 2006 and 2016, respectively. In the 2016 WEF report, the country’s overall global gender gap index was heavily pulled down by the large gaps in two main areas: education (ranked 132); and economic participation and opportunity (ranked 106). These rankings suggest that there may be a close link between the gender gaps in educational attainment and economic participation in this country. A breakdown of the three components of the gender gap in the economic participation and opportunity category is in order. Within the economic participation and opportunity category, Ethiopia’s progress in reducing gaps in the labor force participation rate (ranked 42) was undercut by lower achievements in other components, most notably wage equality among men and women for similar work (ranked 105) and the gap in professional and technical work opportunities (ranked 113). Clearly, Ethiopia has a long way to go before gender equality can be achieved in the working environment.
From here on, we will focus our attention on the wage equality for similar work component. We begin by reviewing existing studies on gender wage gap in Ethiopia. To date, only a handful of studies attempted to quantify and understand the prevailing gender pay gap in the Ethiopian economy.Footnote1 Fafchamps et al. (Citation2009), who examined the link between educational attainment and gender wage differentials based on matched employer-employee manufacturing data in eleven African countries, showed the existence of significant gender wage gaps in seven countries including Ethiopia. In addition, the same study found that education narrowed the gender wage gap in three of the seven countries with Ethiopia being one of the four countries in which education did not appear to narrow the gender wage gap. The study by Kolev and Robles (Citation2010) also linked education to the gender wage gap in Ethiopia. Kolev and Robles (Citation2010), using the 2005 Ethiopian Labor Force Survey data, showed that female workers earned 66% of male hourly wages and that at least 14% of the gap can be explained by education gap between men and women. Similarly, Temesgen (Citation2006) estimated wage differentials for a sample of workers from Ethiopia’s urban manufacturing sector. The study argued that the existence of gender wage gap was mainly driven by the discriminatory component, 60%, with the treatment component arising from human capital differentials being responsible for the other 40%.
Studies using data from other Sub-Saharan Africa countries also sought to understand the reasons behind wage inequality issues in the region. Glick and Sahn (Citation1997) examined earnings of men and women in self-employment, private sector employment and public sector employment in Guinea. The authors found that differences in characteristics such as education and experience were responsible for about 45% and 25% of the male-female earnings gap in self-employment and public sector employment, respectively. Their results also showed that women earned more than their male counterparts in the private sector. Agesa (Citation1999) who estimated the gender earnings gap for Kenyan urban workers showed evidence of a considerable pay gap. The author also found that the pay gap is mainly due to discrimination by urban employers. Similarly, Siphambe and Thokweng-Bakwena (Citation2001) showed that most of the gender wage gap in Botswana’s public sector was mainly due to differences in male and female characteristics and that the wage gap in the private sector was driven by unequal treatment between men and women.
Amongst studies focusing on manufacturing industries, Nordman and Wolf (Citation2009) used matched employer-employee data to examine wage gaps in seven African countries. The authors found significant gender wage gaps in Mauritius and Morocco, while no evidence was found in Benin, Kenya, Madagascar, Senegal and Uganda. Jones (Citation2001) concluded that the wage gaps among workers of different educational backgrounds in the Ghanaian manufacturing industry were due to productivity differentials. Further, Van Biesebroeck (Citation2011) compared wage and productivity gaps arising from disparities in human capital in the manufacturing industries of each of the following countries: Kenya; Zimbabwe; and Tanzania. Findings showed that productivity premiums associated with worker characteristics were equal to the corresponding wage premiums in Zimbabwe (the most developed country in the sample), but not in Tanzania (the least developed country), while results were intermediate for Kenya. Abegaz and Nene (Citation2018) showed evidence of significant gender wage and productivity gaps in the Ghanaian manufacturing industry.
The foregoing studies showed that gender wage gap continues to be an issue in several sub-Saharan Africa countries including Ethiopia. Despite the large consensus on the existence of significant gender wage inequalities in the region, empirical evidence is scarce. Among the key areas lacking empirical investigation include the dual presence of wage and productivity gaps and evaluating the residual wage differentials accounting for worker selection (market segregation) and productivity. Thus, the current study aims to contribute to the existing literature in filling this gap by examining the gender wage and productivity gaps in the Ethiopian manufacturing sector.
3. Data and empirical strategy
3.1. Data
This study uses a census-based firm-level manufacturing data collected by the Ethiopian Central Statistics Agency (CSA) for the period 1996–2010. The data consist of about 12,800 firm/year observations. The data covers all medium and large size, power-driven manufacturing establishments employing at least ten workers. It contains detailed plant-level characteristics regarding production, wages and salaries, employment, investment, export sales, and other related factors. A few other studies used the same dataset to examine problems relating to production, export, and the expansion of the Ethiopian economy and its manufacturing sector.Footnote2
The Ethiopian manufacturing industry is largely dominated by labor-intensive production technologies and firms mainly producing light manufacturing goods. According to the 2010 manufacturing survey report, the sectoral distribution of firms shows that food and beverages, non-metallic minerals, furniture, and fabricated metal (except machinery and equipment) account for 26%, 22%, 13%, and 7% of all the manufacturing plants in the country, respectively. In terms of employment share, the food and beverages industry take the lead, followed by textile, non-metallic minerals, and rubber and plastic industrial groups. reports the summary statistics of variables in the analysis. It shows that about 4.4% of the establishments are exporting and that a similar percentage of firms are fully or partially owned by foreign entities. This shows that the Ethiopian manufacturing sector is characterized by limited interactions with the rest of the world in terms of exporting and foreign ownership.
Table 1. Descriptive statistics of firm characteristics from the large and medium manufacturing surveys, 1996–2010.
The female employment share among exporting firms is 3% higher than the overall average of 30%. Besides, the share of unskilled male workers is higher than that of unskilled female workers for all firms and foreign-owned firms. This observation is different for exporting firms where there is no significant gender difference in the share of unskilled workers. However, on average, exporting firms have a higher share of unskilled workers suggesting that they create more production-level jobs that require less skill and educational attainment. With respect to wages, the average real wage of workers is higher among exporting and foreign-owned firms.
In terms of size, capital intensity, and production levels, exporting and foreign-owned firms have clear advantages. Exporting firms are large (hire more workers), capital-intensive, and more productive than others. The average number of workers among the exporting firms is 457 workers, whereas among foreign-owned firms is 114 workers and the aggregate average for the sector is 105 workers. The descriptive statistics also show that older firms are more likely to be exporters and owned partially or fully by foreign companies. This could be due to the privatization of previously state-owned companies following the regime change in 1991. The share of imported materials is higher among foreign-owned firms, while it is lower among exporting firms compared to the sector average. This may suggest that exporting firms use more domestic raw materials than the foreign-owned ones.
The data have some issues and limitations. A few of those issues are discussed next. First, the data lacks detailed employee characteristics that goes beyond skill and gender composition. It is not matched with employee survey data to allow estimations of worker-level wage regression equation and to aggregate the human capital characteristics of workers such as education, experience, occupation, and age for estimations at the firm-level. Second, inconsistencies regarding year of establishment were observed for some firms across different survey years. We took the mode of the establishment year for such firms to compute their age. Third, data regarding temporary employees are incomplete or inconsistent. As such, we were not able to determine the full-time equivalence of seasonal workers. Therefore, the number of employees and the gender and skill composition of workers are computed based on the number of permanent employees.
3.2. Estimating wage and productivity differentials
As highlighted earlier, our study examines the factors affecting firm-level wage gaps. We address the effects of productivity, selection of workers (firm heterogeneity), and other firm characteristics. We employ two approaches: a single firm-level wage cost regression equation proposed by Bartolucci (Citation2014) and a simultaneous regression of wage and production equations using the Hellerstein et al. (Citation1999) approach.
3.2.1. Linear wage regrssion
In the first approach, we examine the wage gap using a linear wage regression equation including the share of female workers as a regressor to capture the gender wage gap. The linear firm-level per-worker wage regression equation is defined as:
where is firm
’s per-worker average wage bill at time
,
is the employment share of female workers, and the vector Z controls for firm-level characteristics including globalization factors and sector and location fixed effects. The main variable of interest is
which captures the effects of an increase in the employment share of female workers on the firm-level average wage bill. A negative coefficient shows the existence of gender wage differentials in favor of male workers. Such an outcome shows that the average wage deteriorates as more women join the labor force of a firm and reflect a situation where women are paid less than the average.
Initially, we estimate this equation using the Ordinary Least Squares (OLS) method without control for firm fixed effects. The estimated coefficient of the female employment share captures the overall gender wage differential. Next, we estimate the regression equation using the panel fixed effects model to control for firm heterogeneity and determine the within firm wage gap. This model exploits the within-firm variation of the female–male composition of workers across time to identify wage gap within a firm. The difference between the OLS estimate (overall gap) and the fixed effects estimate (within firm gap) is informative about market segregation leading to sorting of workers in the manufacturing sector (Bartolucci, Citation2014; Kampelmann & Rycx, Citation2016). A positive difference suggests selection of female workers into high-wage firms and vice versa.
In this approach, we also control for productivity using the log of output per worker as a regressor. Bartolucci (Citation2014) proposed the inclusion of average productivity in the wage cost equation to overcome three notable assumptions of the Hellerstein et al. (Citation1999) approach: perfectly competitive labor market, linear relationship between productivity and wages, and assumption regarding the functional form of the production function. The Bartolucci (Citation2014) approach neither assumes a perfectly competitive labor market nor that relative wages are equal to relative productivity (allows wage-productivity elasticity to be different from one). This approach has been used in recent studies including Garnero, Rycx, and Terraz (Citation2020) to study the effect of firm-level collective agreements on wages and Kampelmann and Rycx (Citation2016) to study wage discrimination against immigrants. It provides a more flexible strategy to examine the extent of wage gaps after controlling for productivity, labor market segregation, and other firm characteristics.
The above linear wage regression equation assumes perfect substitution between female and male workers in the production function. However, substitutability between the two groups may depend on the skill levels. Accordingly, we can implement a detailed classification of the workforce by skill levels and modify the wage cost equation as follows:
where is the share of skilled female workers in the total employment of firm i at time t,
is the share of unskilled female workers, and
is the share of unskilled male workers. Skilled male workers are the reference group. We include log of output per worker as a control for labor productivity. Additional controls for firm-level characteristics include firm size, capital intensity, and firm age. These are captured by the logarithm of number of workers, logarithm of capital per worker, and the number of years a firm has been operating in the industry, respectively.
A firm’s involvement in the global village (globalization) has been found to be an important factor affecting wages of female workers. Following the studies by Chen, Ge, Lai, and Wan (Citation2013) and Abegaz and Nene (Citation2018), globalization is captured using indicators for exporting status (Exporting) and foreign ownership (Foreign). The indicator variable for exporting status takes a value of 1 if a firm exported that year and 0 otherwise. Similarly, the indicator variable for foreign ownership takes a value of 1 if a firm is partially or fully foreign-owned (at least 10% equity share by foreign investors) and 0 otherwise. We also control for the use of imported inputs using the share of imported materials in the production process. Globalization increases competition among firms and the cost of discrimination, which helps reduce the pay gap between men and women (Becker, Citation1957). Several studies have shown that globalization is associated with more female employment, higher female wages and lower gender pay gap (Becker, Citation1957; Juhn, Ujhelyi, & Villegas-Sanchez, Citation2013; Oostendorp, Citation2009). A few other studies have also found that exporting firms are associated with larger wage gap when compared to their non-exporting counterparts (Bøler, Smarzynska Javorcik, & Ulltveit-Moe, Citation2015; Chen et al., Citation2013). We, therefore, expect the coefficients of the globalization variables to be either positive or negative.
3.2.2. Simultaneous wage and production regression equations
The second part of our analysis focuses on estimating productivity differentials and wage differentials simultaneously using the Hellerstein et al. (Citation1999) approach. We estimate productivity differentials using the following production function in the same manner as in Hellerstein et al. (Citation1999) and Dong and Zhang (Citation2009)Footnote3
where Y is the value of output, A is technical coefficient, K is capital, Material is the value of materials used in the production process, QL is quality weighted aggregate labor, and the vector Z represents firm characteristics including foreign ownership, exporting status, and location and sector fixed effects. The quality weighted aggregate labor (QL) using marginal productivities as weight assumes perfect substitution of workers across groups. QL is defined as follows:
where ,
,
and
represent the number of unskilled male workers, skilled male workers, unskilled female workers, and skilled female workers, respectively. The weights,
’s, are the corresponding marginal productivities of each group of workers. Assuming the female-male relative marginal productivities of unskilled and skilled workers are equal (
) and the unskilled-skilled relative marginal productivities for the two genders are equal (
, we can simplify and rewrite Equationequation (3)
(3)
(3) by taking the natural logarithm as follows:
where ,
, and
represent the number of total, male, and female workers, and
and
are female-male and unskilled-skilled ratios of marginal productivities, respectively. Finally, the production regression equation with quality weighted labor can now be re-written as:
The non-linear production function in Equationequation (6)(6)
(6) allows for the estimation of the relative marginal productivities of female-male (
) and unskilled-skilled (
) workers which are then compared to the corresponding relative wages. To achieve this objective, we need to have a firm-level wage equation analogous to the production function in (6). We follow similar steps as used in the production function, moving from Equationequation (3)
(3)
(3) through Equationequation (6)
(6)
(6) , to come up with an equation that reflects a weighted average of wage bills paid to unskilled men, skilled men, unskilled female, and skilled female workers using employment shares as weights.Footnote4 The final firm-level average wage regression equation, which is similar to the production function in Equationequation (6)
(6)
(6) , is defined in a same manner as in Hellerstein et al. (Citation1999) and Dong and Zhang (Citation2009) as follows:
where is the firm-level average wage bill,
and
are relative wages of unskilled-skilled and female-male workers, respectively, and the vector
represents firm characteristics such as size, capital intensity, export status, foreign ownership, and others.
EquationEquations (6)(6)
(6) and (Equation7
(7)
(7) ) are estimated simultaneously using Zellner’s seemingly unrelated regression (SUR). We perform linear parameter tests to verify the existence of wage and productivity gaps between female and male as well as unskilled and skilled workers. This is done by testing the null hypotheses that the coefficients representing productivity ratios (
and
) and wage ratios (
and
) are each equal to one. Under these hypotheses, relative wages and productivities are considered to be equal between female and male workers and between unskilled and skilled workers. Rejecting the null hypotheses reflect existence of productivity and wage differentials based on gender and skill levels.
Finally, we perform a statistical test to determine whether the female-male productivity ratio () and the female-male wage ratio (
are equal or not. This assumes that workers in a competitive market are paid based on their marginal productivities. Rejecting the null hypothesis signals a presence of wage gap unexplained by productivity differentials. Similar steps are taken to compare the unskilled-skilled productivity (
) and wage (ratios).
4. Results and discussion
presents estimation results of the panel wage regression Equationequation (1)(1)
(1) . We used the pooled OLS technique without control for firm effects in columns 1–3. We control for individual firm heterogeneity using the panel fixed-effects method in columns 4–9.
Table 2. OLS and panel fixed effects estimation results of the linear wage regression Equationequation (1)(1)
(1) without grouping workers by skill levels.
The coefficient of the female employment share is negative and statistically significant. This reflects the presence of a significant gender wage gap. Specifically, firms with higher female employment share pay lower average wages than firms with higher male employment share. Column (1) presents the unconditional gender wage gap after controlling for time effects without the rest of the control variables. The result indicates that the gross gender wage gap is −0.49 (49%).Footnote5 A comparable estimate to the one in column (1), presented in column (4) where we control for unobserved firm fixed effects presents the unconditional within firm wage gap. The within firm estimate shows that female workers earn 39% lower than male workers. Zhang and Dong (Citation2008) and Chen et al. (Citation2013) also found a negative association between female employment share and firm-level average wages in the Chinese manufacturing industry. The negative difference between the OLS estimate (−0.49) and the fixed effects estimate (−0.39) indicates a sorting/segregation of female workers into low-wage firms. In this scenario, the segregation of female workers into low-wage firms accounts for about 10% of the gender wage gap.
In the OLS regressions (columns 2 and 3), controls for firm characteristic such as exporting status, foreign ownership, capital per worker, firm size (measured by the logarithm of number of employees), and firm age are significant determinants of wages. However, their contribution in terms of reducing the gender gap remain insignificant. The only exception is labor productivity which appears to be a significant determinant of wages and the gender wage gap. The coefficient of the female employment share is lower in column (3) when we control for productivity measured by the logarithm of output per worker. In the fixed effects model without labor productivity (column 6), the firms’ globalization indicators, such as exporting status, foreign ownership, and the use of imported materials in production (the variable Import Ratio) are associated with higher average wages. When a control for labor productivity is included, only the ratio of imported materials remains significant. The null results for such indicators as exporting and foreign-ownership may be due to lack of sufficient within firm variation overtime. Interaction terms in column (9) suggest that the observed gender wage gap is lower among foreign-owned firms, while exporting activity plays no role to narrow or widen the gap. Unlike Chen et al. (Citation2013) who finds larger wage gaps among exporting and foreign-owned than non-exporting and domestic firms in the Chinese manufacturing, we find no such effects in the Ethiopian manufacturing.
With respect to firm characteristics, capital intensity (capital per worker) is positive and significant in the firm-level wage regression equation with or without control for productivity. This indicates that capital intensity is an important determinant of wages across firms. However, the coefficient of capital–labor ratio becomes insignificant when the firm fixed effects are controlled for. This may be due to a lack of variation of capital intensity within a firm across time or that it is not a significant determinant of the within firm wage-setting process. Further, firm age and size are significant positive determinants of wages in the OLS regressions, but they become insignificant or even negative (for size) in the fixed effects model. With control for firm heterogeneity, results show that the firm-level average wages decrease with firm size. This may reflect a slow wage growth or that firms hire more production-level workers as they grow bigger in size. The in-kind benefits such as education, health care, job security, transportation, and other incentives may also grow faster accompanied with slow wage growth. On the other hand, productivity remains a significant positive determinant of wages in the linear wage-setting equation with or without control for firm heterogeneity. Although controlling for productivity shrinks the gender wage gap in all estimations, the gender penalty remains significant and high. The overall results show that females suffer from wage gaps that are robust to firm characteristics, sorting, and productivity. The estimated within firm pay gap could be a result of the unobserved worker characteristics not captured by the firm productivity effects and other firm characteristics. Besides, it may reflect firm-level gender biases in hiring and wage setting processes that could be deemed as discrimination and may lead to gender-based wage differentials. However, matched employee-employer data that provides detailed worker characteristics are necessary to examine the existence of within firm gender discrimination and the role it plays in explaining the observed wage gap.
Next, we present estimation results of Equationequation (2)(2)
(2) accounting for a detailed classification of workers by skill levels in . Compared to the reference group, the share of skilled male workers, employment share of the three groups (skilled female, unskilled male, and unskilled female) are associated with lower average wages. For instance, the unconditional wage gap between skilled male and skilled female workers with control for time effects is about 41% as shown in column (1). Similarly, the wage difference between unskilled female (−0.45) and unskilled male (−0.35) appears to be significant.Footnote6 The fixed effects model (column 4) indicates a lower within firm wage gap for unskilled male and skilled female workers. This suggests that unskilled male and skilled female workers are sorted into low-wage firms, but they face lower within firm gaps. On the other hand, unskilled female workers are segregated into high-wage firms and face higher within firm wage gaps compared to unskilled male workers.
Table 3. OLS and panel fixed effects estimation results of the linear wage regression Equationequation (2)(2)
(2) grouping male and female workers by skill levels.
Accounting for productivity (columns 5–8) eliminates the wage gap between skilled and unskilled male workers. Besides, it closes half of the gap between skilled male and skilled female workers. However, controlling for productivity does not lead to a reduction in the pay gap between unskilled female and unskilled male workers. The gap between the two groups remains high and significant after controlling for productivity, other firm characteristics, and firm fixed effects. This may reflect occupational segregation between male and female unskilled workers and that there is premium to the occupations of male workers. These occupations may tend to be physically demanding, riskier, and pay higher wages.
Following the presentation and discussion regarding estimation results of the average wage Equationequations (1(1)
(1) and Equation2
(2)
(2) ), presents the SUR regression results of the firm-level production and average wage Equationequations (6
(6)
(6) and Equation7
(7)
(7) ) using the Hellerstein et al. (Citation1999) approach.
Table 4. Estimation results of the simultaneous regressions of the non-linear production and average wage functions: Hellerstein et al. (Citation1999) approach.
The coefficients of interest are the female-male and unskilled-skilled wage and productivity ratios captured by the non-linear structure of the two equations which incorporate the shares of female and unskilled workers except in column 6 where we only include share of female workers. Consequently, we perform statistical tests to examine the presence of gender and skill-based wage and productivity differentials. We also test for the equality of the wage and productivity ratios for female-male and unskilled-skilled workers.
Results show that the productivity ratio of female to male workers is about 0.4, suggesting that the productivity of female workers is about 40% of that of their male counterparts. This result holds irrespective of the controls for location, sector, and skill composition of workers. The null hypothesis that the productivity of male and female workers are equal is rejected at the 1% level in all model specifications. Similarly, the unskilled-skilled productivity ratio suggests that unskilled workers are about 45% less productive than their skilled counterparts. The hypothesis that skilled and unskilled workers are equally productive is also rejected at the 1% level.
In the average wage equation, the female-male wage ratio ranges from about 0.5 in the parsimonious regression (column 1 of ) to about 0.4 in the more general models incorporating sector and region fixed effects. This finding suggests that the average wage of female workers is about 40% to 50% of the wages of male workers. The hypothesis that average wages of male and female workers are equal is rejected at the 1% level. Compared to the OLS results in , the estimated gender wage gaps are similar. However, the SUR estimates in do not control for firm heterogeneity due to the complexity of the nonlinear estimation technique that does not allow to include fixed effects. In addition, the wage ratio of unskilled to skilled workers is about 0.7, suggesting that unskilled workers earn about 70% of the earnings of skilled workers.
In this approach, we also compare the female-male productivity gap with the corresponding wage gap. The null hypothesis that the estimated female-male wage and productivity ratios are equal cannot be rejected, implying that the observed gender wage differentials are statistically equivalent with the productivity gaps. These results contradict with those based on the linear wage cost equation proposed by Bartolucci (Citation2014) () which suggests that controlling for productivity does not account for the total within firm gender wage gap. A few notable reasons for the diverging results are provided next. First, the assumption of perfect competition market may not be applicable in the Ethiopian labor market context. Second, the wage-productivity elasticities in are significantly different from one, suggesting that a change in productivity leads to change in wages but not proportionally. Thus, the assumption of linear wage and productivity relationship in the Hellerstein et al. (Citation1999) approach may not be relevant for the current dataset. Third, the firm-level dataset allows to classify workers by gender and skill levels. However, unlike matched employer-employee data, it does not provide human capital and other controls for workers’ characteristics. Thus, future studies could utilize such dataset to determine the contribution of workers’ characteristics on the observed gender wage gap unaccounted for by productivity differentials and market segregation.
With respect to skill differentials, the hypothesis that skilled and unskilled workers are paid based on their marginal productivity is rejected at the 1% level in the regressions without sector and location fixed effects. The estimated wage ratios are greater than the productivity ratios, which suggests that unskilled workers earn more than their marginal productivity. However, the unskilled-skilled wage and productivity ratios are statistically equal to each other when sector and location fixed effects are controlled for. These results support those in based on the Bartolucci (Citation2014) approach, which indicates no wage gap between skilled and unskilled male workers after controlling for productivity and firm fixed effects.
Results using the Hellerstein et al. (Citation1999) simultaneous wage and production regressions provide contrasting results for unskilled and female workers. Both female and unskilled workers have lower marginal productivities than their skilled and male counterparts, respectively. However, the statistical tests indicate that females are paid less than or equal to their productivities, whereas unskilled workers are paid more than or equal to their productivities. In effect, the wage setting process seems to favor unskilled workers against female workers. Besides, results using the Bartolucci (Citation2014) wage regression equation indicate significant wage gaps unexplained by productivity and firm fixed effects between skilled male and skilled female as well as between unskilled male and unskilled female workers. Although the Bartolucci (Citation2014) approach is preferred in that it does not make assumptions regarding the labor market structure and the equality of productivity and wages, future studies using matched employer-employee data considering workers characteristics are needed to further investigate the unexplained components of the gender and skill wage differentials.
5. Conclusions
This study examines gender and skill wage differentials after controlling for factors affecting the firm-level average wages using a panel of Ethiopian manufacturing firms for the period 1996 to 2010. Results indicate that an increase in the female employment share serves to deteriorate the firm-level per-capita wage bill. These results complement findings of earlier studies showing the presence of significant gender wage gap in the manufacturing sector and other sectors of the Ethiopian economy (Fafchamps et al., Citation2009; Kolev & Robles, Citation2010; Temesgen, Citation2006). Our findings also show that globally connected firms offer higher average wages than firms with no links to the international market in terms of ownership and trade. In contrast, results suggest that exporting and foreign ownership play little or no role in reducing the gender wage gap. We also find that higher skill levels are rewarded with higher wages.
Gender and skill wage differentials can be partially explained by market segregation and productivity. Using a single wage regression equation proposed by Bartolucci (Citation2014), we find that productivity and firm heterogeneity explain a significant portion of the gender wage gap. Female workers appear to be segregated into low-wage firms and face lower within firm gap. Controlling for productivity explains some of the remaining gender pay gap, 5 to 8 percentage points. However, significant gap remains unexplained by labor market segregation, productivity, and other firm characteristics. With respect to skill pay gaps, results suggest no pay gap between skilled and unskilled male workers after controlling for productivity and firm heterogeneity. On the other hand, unskilled female workers face higher gap than skilled female workers while both face pay gap against their male counterparts. We also estimate the Hellerstein et al. (Citation1999) firm-level wage cost and production regression equations simultaneously using a nonlinear SUR technique. In this approach, we observed that the female-male wage and productivity ratios are statistically not different from each other. However, this conclusion contradicts with the results of the single wage regression equation which indicates significant gender pay gap after controlling for productivity. The results using the Hellerstein et al. (Citation1999) approach may suffer from biases due to the assumptions of perfectly competitive labor market, linear wage and productivity relationship, and the functional form of the production function. Further, the results from this model do not account for firm heterogeneity.
Based on the results outlined in this paper, efforts to reduce the gender pay gap in the Ethiopian manufacturing sector should focus on skill development to improve the productivity of female workers through training and vocational education and tackling segregation in the labor market. Improving the skills of female workers may make them more productive which may in turn allow them to compete with their male counterparts for the more productive jobs. It is also important to note that merely providing women with the skill sets may not address other reasons for wage gaps such as women sorting into the less discriminatory firms or sorting according to productivity, among others. In addition to improving women skills, industry wide policies tailored on promoting equality among men and women in the workplace may help reduce the issue arising from ranking firms according to how discriminatory they are in making the decision to work for them or not. Further, combating institutional, cultural, and political bottlenecks that hinder female workers from pursuing careers in higher paying firms and sectors may help improve parity in the workplace.
In the current study, we were not able to distinguish sources of the gender gap arising from human capital characteristics of workers and market discrimination due to data limitations. Future studies utilizing linked employer-employee data may help identify different sources of the gender pay gap considering the characteristics of workers and firms.
Disclosure statement
No potential conflict of interest was reported by the authors.
Additional information
Notes on contributors
Melaku Abegaz
Melaku Abegaz is an Assistant Professor of Economics at the University of Wisconsin-River Falls. His research interests include international trade, development, gender, and education. He holds a Ph.D. in Economics from Southern Illinois University Carbondale.
Gibson Nene
Gibson Nene is an Associate Professor of Economics at the University of Minnesota Duluth. His research interests include development economics, environmental economics, economics of tourism and industrial organization. He holds a PhD in Agricultural Economics from the University of Nebraska-Lincoln.
Notes
1 Other empirical studies attempted to address the limited progress in narrowing the educational gap irrespective of the wider reforms undertaken thus far (Haile, Citation2018; Molla, Citation2013). Besides, attempts have been made to understand the overall gender inequality problem in Ethiopia (Bayeh, Citation2016; Dea, Citation2016) and investigate the impact of reforms in family law and land registration on the socio-economic activities of women (Hallward-Driemeier & Gajigo, Citation2015; Kumar & Quisumbing, Citation2015).
2 The data was used by Bigsten and Gebreeyesus (Citation2007), Gebreeyesus (Citation2008), Shiferaw (Citation2009), Bigsten and Gebreeyesus (Citation2009), and others to examine firm growth, productivity, firm turnover, export performance, and related issues.
3 Subscripts for time and firm indicators are dropped to avoid clutter.:
4 , where the
’s represent the wage bills paid to each group and
represents the weighted average total wage bill of a firm. The dataset provides information on the aggregate wage bill,
, but not disaggregated across the four groups. Assuming the skilled and unskilled workers female-male wage ratios are equal (
and the unskilled-skilled wage ratios of female and male workers are equal (
=
), the average wage equation can be simplified and written by taking natural logarithm as:
5 The coefficient can be interpreted as the effect of an increase in female employment share on the average wage cost of a firm: one percentage point increase in the employment share of female workers is on average associated with 0.49% decrease in the average per-worker wage bill.
6 Since the reference group is skilled male workers, to compare the wage gap between unskilled female and unskilled male workers, we must calculate the difference of the coefficients for the two groups.
References
- Abegaz, M., & Nene, G. (2018). Gender wage and productivity gaps in the manufacturing industry. The case of Ghana. Economic Papers, 37(3), 313–19.
- Agesa, R. U. (1999). The urban gender wage gap in an African country: Findings from Kenya. Canadian Journal of Development Studies, 20(1), 59–76.
- Appleton, S., Hoddinott, J., & Krishnan, P. (1999). The gender wage gap in three African countries. Economic Development and Cultural Change, 47(2), 289–312.
- Bartolucci, C. (2014). Understanding the native–immigrant wage gap using matched employer-employee data: Evidence from Germany. ILR Review, 67(4), 1166–1202.
- Bayeh, E. (2016). The role of empowering women and achieving gender equality to the sustainable development of Ethiopia. Pacific Science Review B: Humanities and Social Sciences, 2, 37–42.
- Becker, G. S. (1957). The economics of discrimination: An economic view of racial discrimination. Chicago: The University of Chicago Press.
- Bigsten, A., & Gebreeyesus, M. (2007). The small, the young, and the productive: Determinants of manufacturing firm growth in Ethiopia. Economic Development and Cultural Change, 55(4), 813–840.
- Bigsten, A., & Gebreeyesus, M. (2009). Firm productivity and exports: Evidence from Ethiopian manufacturing. The Journal of Development Studies, 45(10), 1594–1614.
- Blau, F., & Khan, L. (2000). Gender differences in pay. Journal of Economic Perspectives, 14(4), 75–99.
- Bøler, E. A., Smarzynska Javorcik, B., & Ulltveit-Moe, K. (2015). Globalization: A woman’s best friend? Exporters and the gender wage gap. CEPR Discussion Paper 10475.
- Chen, Z., Ge, Y., Lai, H., & Wan, C. (2013). Globalization and gender wage inequality in China. World Development, 44, 256–266.
- Dea, M. (2016). The prospectus, challenges and causes of gender disparity and its implication for Ethiopia’s development: Qualitative Inquiry. Journal of Education and Practice, 7(4), 24–37.
- Dong, X. Y., & Zhang, L. (2009). Economic transition and gender differentials in wages and productivity: Evidence from Chinese manufacturing enterprises. Journal of Development Economics, 88(1), 144–156.
- Fafchamps, M., Söderbom, M., & Benhassine, N. (2009). Wage gaps and job sorting in African manufacturing. Journal of African Economies, 18(5), 824–868.
- Garnero, A., Rycx, F., & Terraz, I. (2020). Productivity and wage effects of firm‐level collective agreements: Evidence from Belgian linked panel data. British Journal of Industrial Relations, 58(4), 936–972.
- Gebreeyesus, M. (2008). Firm turnover and productivity differentials in Ethiopian manufacturing. Journal of Productivity Analysis, 29(2), 113–129.
- Glick, P., & Sahn, D. E. (1997). Gender and education impacts on employment and earnings in West Africa: Evidence from Guinea. Economic Development and Cultural Change 793-823.Goldin, C. (2014). A Grand Gender Convergence: Its Last Chapter. American Economic Review, 104(4), 45(4), 1091–1119.
- Haile, G. A. (2018). Intergenerational mobility in socio-economic status in Ethiopia: Intergenerational mobility in Ethiopia. Journal of International Development, 30(8), 1392–1413.
- Hallward-Driemeier, M., & Gajigo, O. (2015). Strengthening economic rights and women’s occupational choice: The impact of reforming Ethiopia’s family law. World Development, 70, 260–273.
- Hellerstein, J. K., Neumark, D., & Troske, K. R. (1999). Wages, productivity, and worker characteristics: Evidence from plant-level production functions and wage equations. Journal of Labor Economics, 17(3), 409–446.
- Jones, P. (2001). Are educated workers really more productive? Journal of Development Economics, 64(1), 57–79.
- Juhn, C., Ujhelyi, G., & Villegas-Sanchez, C. (2013). Trade liberalization and gender inequality. American Economic Review, 103(3), 269–273.
- Kampelmann, S., & Rycx, F. (2016). Wage discrimination against immigrants: Measurement with firm-level productivity data. IZA Journal of Migration, 5(1), 1–24.
- Klasen, S., & Lamanna, F. (2009). The Impact of gender inequality in education and employment on economic growth: New evidence for a panel of countries. Feminist Economics, 15(3), 91–132.
- Kolev, A., & Robles, P. S. (2010). Addressing the gender pay gap in Ethiopia: How crucial is the quest for education parity? Journal of African Economies, 19(5), 718–767.
- Kumar, N., & Quisumbing, A. R. (2015). Policy reform toward gender equality in Ethiopia: Little by little the egg begins to walk. World Development, 67, 406–423.
- Leopold, T. A., Ratcheva, V., & Tyson, L. D. (2016). The global gender gap report 2013. Geneva Switzerland World Economic Forum 2013.
- Molla, T. (2013). Higher education policy reform in Ethiopia: The representation of the problem of gender inequality. Higher Education Policy, 26(2), 193–215.
- Nordman, J. C., & Wolf, F. C. (2009). Gender differences in pay in African manufacturing firms. HAL Archives <Hal-00421227>.
- Ogato, G. S. (2013). The quest for gender equality and women’s empowerment in least developed countries: Policy and strategy implications for achieving millennium development goals in Ethiopia. International Journal of Sociology and Anthropology, 5(9), 358.
- Oostendorp, R. H. (2009). Globalization and the gender wage gap. The World Bank Economic Review, 23(1), 141–161.
- Shiferaw, A. (2009). Survival of private sector manufacturing establishments in Africa: The role of productivity and ownership. World Development, 37(3), 572–584.
- Siphambe, H. K., & Thokweng‐Bakwena, M. (2001). The wage gap between men and women in Botswana’s formal labour market. Journal of African Economies, 10(2), 127–142.
- Temesgen, T. (2006). Decomposing gender wage differentials in urban Ethiopia: Evidence from linked employer–employee (LEE) manufacturing survey data. Global Economic Review, 35(1), 43–66.
- Van Biesebroeck, J. (2011). Wages Equal productivity. Fact or fiction? Evidence from sub Saharan Africa. World Development, 39(8), 1333–1346.
- Zhang, L., & Dong, X. Y. (2008). Male–female wage discrimination in Chinese industry Investigation using firm‐level data. Economics of Transition, 16, 85–112.