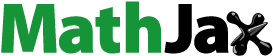
ABSTRACT
Survey forecasts are prone to entry and exit of forecasters as well as forecasters not contributing every period leading to gaps. These gaps make it difficult to compare individual forecasters to each other and raises the question of how to deal with the missing observations. This is addressed for the variables GDP, CPI inflation, and unemployment for the US. The theoretically optimal method of filling in missing observations is derived and compared to several competing methods. It is found that not filling in missing observations and taking the previous value do not perform particularly well. For the other methods assessed, there is no clear superior approach for all use cases, but the theoretically optimal one usually performs quite well.
KEYWORDS:
1. Introduction
There is an extensive literature on the use of survey data of professional forecasters and how to compare and combine individual contributors of these surveys going back to at least Bates and Granger (Citation1969). One difficulty when working with survey forecasts is the non-response of survey contributors including the extensive entry and exit of individuals. For example, over the entire 210 quarter history (Q4 1968-Q1 2021) of the Survey of Professional Forecasters (SPF) conducted by the Philadelphia Fed, there are almost 450 individual contributors, and each has contributed 19 forecasts on average. While entry and exit play a large role over such a long period, the non-response is also an issue when looking at a shorter time period. For example, for the four surveys in 2020, there were 49 contributors with a total of 151 forecasts, implying that forecasters on average did not contribute a forecast for around 25% of that year.
In turn, the missing observations are not fully random and can influence the analysis. For example, Bürgi (Citation2017) has shown that the common finding that a majority of individual forecasters appear biased can mainly be attributed to the gaps in the survey, rather than asymmetric loss function or sub-optimal forecasting behavior. Due to the potential impact of missing observations on inferences, it is instrumental to address how to best deal with the gaps in forecasting surveys.
While there is an extensive literature on how to fill in missing observations in general survey data (e.g., see Andridge and Little (Citation2010) or Little and Rubin (Citation2019) for a review), forecasting surveys have some key features that many other surveys do not share. For example, the forecasters are asked to predict the same event across multiple surveys, only few forecasters are included in the surveys, and a large share of responses is missing. These features can limit options but also open up new avenues to handle non-response and have led to a multitude of methods. For example, one can use a previous response regarding the same event to fill in a missing observation, which is not possible in surveys where people are not asked repeatedly about the same event. Indeed, under quite general assumptions, the optimal method of filling in missing observations are derived. It is shown that the method proposed in Genre et al. (Citation2013) coincides with this optimal method and it is compared with the different methods suggested in the literature. These methods for surveys of professional forecasters can be put into four groups: not filling in missing observations, replacing missing observations with the simple average, filling missing observations with a function of previous predictions of the same event made by the same forecaster, and filling gaps with the predictions made by a similar forecaster.
The results presented here also have important implications for methods used in the context of surveys of professional forecasters that are robust against missing observations. Examples of these methods include Mack and Skillings (Citation1980), D’agostino et al. (Citation2012), or Bürgi and Sinclair (Citation2017). While these methods do not explicitly fill in missing observations, they make specific assumptions about the missing observations. These assumptions can then be linked to a specific method of explicitly imputing the missing observations and using the corresponding method of explicitly filling in the missing observations, one can obtain the same result as using the robust method directly. For example, the method in Bürgi and Sinclair (Citation2017) implicitly assumes that the forecast performance for missing observations is similar to the non-missing observations and would be equivalent to leaving gaps explicitly missing.Footnote1 If it was found that there are superior methods to explicitly fill in missing observations than the one implied by the assumptions of the robust method, it might be worthwhile to first fill in the missing observations using the superior method before applying the robust method. This would also allow to more easily compare methods that are robust against missing observations to ones that are not.
In order to provide results that are broadly applicable, the analysis is done for GDP growth, unemployment and CPI inflation at the quarterly frequency and for various horizons in the Bloomberg survey and for GDP in the Wall Street Journal survey from 2002 to 2015.
The reminder of the paper is structured as follows: the next section describes the theoretically optimal approach followed by different approaches. Section 4 runs the simulations followed by the application to a forecast combination problem. The final section concludes.
2. Optimal signal extraction
Explicitly filling in the missing observations is typically done in two steps and the second step is the one of interest here. First, survey participants that only made few predictions got excluded from the analysis. In the extreme case, only those participants are kept in the sample that participated in every survey round like Issler and Lima (Citation2009) and this already solves the data gaps issue. However, this extreme measure dramatically reduces the sample both in the number of forecasters included and the time period over which forecasts can be assessed. As the missing observations are not necessarily random (e.g., see Bürgi (Citation2017)), this can cause a sample selection bias. Because of this, the extreme case is not often pursued in the literature. Instead, a second step is added where models are used to replace the (few) remaining missing observations. This second step is of main interest here.
In order to obtain a theoretically optimal way to fill in the missing observations, assume that each period, forecasters receive (forward looking) noisy signals about each future date (event) of the underlying variable as in Bürgi (Citation2020). These signals might mainly include the data releases of the variable of interest as well as the ones of related variables. They could also include forward looking information like the announcements by policymakers which only affect the underlying variable at specific future dates (e.g., a tax change typically only has a transitory effect on inflation at the effective date). The optimal prediction for a specific event in period t then becomes the weighted average of all signals received prior to t and the current signal received in period t. This is a flexible generalization of the standard Kalman filter setup with three key advantages: It does not require any assumptions about the data generating process, it has horizon-specific signals and there is no need to assume that the underlying variable is unobservable. In this setup, a forecast is made up by
where is the prediction for variable y in period t made by individual i in period t-h and made up by the (optimally) weighted average between
; the forward looking signal with noise
and the previous prediction
(which in turn is a weighted average of signals). Assuming
is the prediction error made when predicting
, the optimal
becomes
. Unfortunately,
is unobservable and hence the missing predictions cannot be directly constructed. However, if
has a common component across forecasters (e.g.,
), one can use this information to get an estimate for
. Specifically, the simple average can be used to estimate
. Equation 1 can be reformulated for the simple average as
assuming the same weighting is optimal for the aggregate and the individual level.Footnote2 Replacing the individual signal with the aggregate signal in equation 1, can be rearranged to
as the forecasted prediction. If the signal noise for individual i is assumed to be of the form with individual component variances
, the prediction error made with this approach is
. As
is assumed to be unobserved white noise and
is the optimal (inverse variance) weight, one cannot improve upon this prediction.Footnote3
This approach has been used in the literature previously but without any theoretical foundations. Specifically, it was introduced by Genre et al. (Citation2013) and also applied by Kenny et al. (Citation2015b), Kenny et al. (Citation2015a) and Diebold and Shin (Citation2019) who estimate the equation
This approach is also closely related to the Kalman filter (e.g., as described in Ghysels and Wright (Citation2009) or Grishchenko et al. (Citation2019)). Specifically, one could assume that for each horizon, the (unobservable) true difference between the individual prediction and the simple average follows an AR(1) process and one observes an iid signal of this difference. The optimal prediction then becomes the weighted average between the previous prediction of the state and the signal, resulting in the new optimal prediction
. While this way of motivating the regression leads to the same estimation equation, the assumptions are a bit more stringent as an AR(1) process is assumed, it is assumed that the true state is unobservable and a separate model is assumed for each horizon.Footnote4
This approach can also be applied to consumer surveys where participants are repeatedly asked about a fixed horizon forecast for a serially correlated variable (e.g., inflation). In this case, the signal reflects the change from one event to another and the weight on the previous prediction is directly related to the serial correlation of the underlying variable. However, the approach might not be optimal anymore as the variables predicted are not necessarily the same for all participants.
3. Alternative approaches
In order to assess the performance of the theoretically optimal approach, it is compared to six other individual approaches proposed in the literature as well as the average across all methods.
The first approach to handle missing observations is to leave them missing (e.g., see Capistrán and Timmermann (Citation2009), or Bürgi and Sinclair (Citation2017) for examples). This approach assumes that replacing the missing observations does more harm than good.
The second approach replaces the missing observations with the simple average across all forecasters (e.g., see Capistrán and Timmermann (Citation2009) or Lahiri et al. (Citation2017) for examples). This approach is the only approach discussed here which always fills all missing values. All other approaches might leave some observations missing unless the sample is restricted in a specific way. Due to this property, the simple average is used as a fallback option in a robustness check.
The next approach uses prior predictions for the same event made by the same forecaster to fill in missing observations (e.g., see Poncela et al. (Citation2011) or Conflitti et al. (Citation2015)). For example, a forecaster might contribute to a survey in the first quarter of 2005 but not the second quarter of the same year. If forecasts for the fourth quarter of 2005 are made in both surveys, one could use the first quarter survey predictions to replace the missing observation. This approach cannot replace missing observations at the beginning of the sample and is implicitly assuming that forecasts remain unchanged.
The assumption of an unchanged forecast might not necessarily be adequate even in monthly surveys (see Sheng and Wallen (Citation2014), Andrade and Le Bihan (Citation2013), or Bürgi (Citation2020)) and ignore the reduction in uncertainty over time. This is also why the theoretically optimal approach derived above takes into account changes in the simple average to fill in missing observations. Approaches four and five are variations of this approach. Specifically, Lahiri et al. (Citation2017) and Zhao (Citation2020) proposed an approach that can deal with multi-period gaps. Specifically, they estimate
That is, the past four deviations from the simple average by forecaster are averaged and then regressed on the current deviation. While this approach can handle gaps that are larger than one period, it averages negative and positive deviations, causing a trade-off. As with the previous approach, the estimation uses predictions pooled across horizons.
The fifth approach aims to more explicitly model the signal that agents use to update. Under the assumption that forecasters base their forecasts on similar data, they might weight different data differently. For example, two forecasters might react differently to a higher than expected payroll release, even if they both watch it closely. In order to capture this, a mixed data sampling (MIDAS) approach is utilized where data surprises from Scotti (Citation2016) are added to the optimal model. Specifically,
is estimated where SurpM1 is the value of the Scotti (Citation2016) surprise index at the end of the first month of the quarter and SurpM2 the value at the end of the second month of the quarter. A related approach has been used by Ghysels and Wright (Citation2009) where they used daily market returns in a MIDAS regression to increase the survey frequency to daily.
The sixth and last individual approach considered follows the idea in Andridge and Little (Citation2010) that one can replace missing observations with the predictions of a similar forecaster. The specific approach taken here is to replace missing observations of forecaster with the ones made by the forecaster that has the highest correlation across horizons. Specifically, the forecasts made by the forecaster whose predictions satisfy
where are the predictions made by forecaster
with horizon
. As with the previous approaches, using multiple horizons in the model improves the prediction. This approach does not have the restriction for the first period in the sample but leaves random observations missing. The reason for this is that the highest correlated forecaster might miss some of the same predictions as the one whose missing predictions he should replace.
In addition to these six alternative approaches plus the theoretically optimal one, the simple average across the filled in values is also considered. This average uses the simple average approach if no other approach filled in a specific value and the average of up to six values (one of the seven approaches was to leave it blank).Footnote5
4. Simulation
In order to assess how the above approaches compare in filling the missing observations, a simulation is run. The sample over which the performance is assessed is the quarterly forecasts made in March, June, September, and December starting in December 2002 and ending in March 2015; a total of 50 observations. The 2002 start date is the first date when the WSJ survey moved from a semi-annual survey to a monthly survey and the quarterly Bloomberg data is from Bürgi (Citation2017) and ends in March 2015. Due to entry and exit, longer samples are not necessarily better than shorter samples as forecasters become less likely to overlap. In addition, any forecaster with less than 16 predictions (equivalent to 4 years) is not included in the simulation. This leaves 75 individual forecasters in the Bloomberg survey and 65 individual forecasters in the WSJ survey.
In order to be able to compare the filled in values to actual values, it is necessary to randomly replace values with missing observations. To this end, the simulation with 100 replications replaces the actual predictions with missing observations for 10% of the date-forecaster pairs.Footnote6 This format of randomly replacing values mimics that forecasters either contribute forecasts for all horizons in a survey round or do not participate. Once the forecasts have been replaced with missing observations, the above methods are used to fill in these missing observations. As mentioned above, some of the approaches will leave some observations missing. To maintain comparative results, the missing observations are left missing for one set of simulation results and replaced with the simple average for another set of results. While it is possible to fill in missing values in an iterative approach, this is not chosen for two reasons. First, even under the iterative approach, the regression-based approaches might still leave some observations missing. Second, each iteration will fill the missing observations with inferior predictions and the goal is to compare the approaches using a best case scenario.
In order to compare and assess the approaches, a total of six metrics are calculated for the current quarter, one-quarter ahead and two-quarter ahead predictions.Footnote7 These six measures can be grouped into (root) mean squared measures that strongly penalize large deviations and absolute measures that penalize large deviations to a lesser extent. In order to keep the results compact, mainly the (root) mean squared results are reported in the main text and the absolute results are shown in the appendix.
The first measures compare the predicted value to the actual value. These are the root mean squared difference (RMSD) and the mean absolute difference (MAD) between the filled values and the actual values. That is
where is the actual value in the survey and
is the filled in value by the different approaches. For MSD, only observations that were filled in are included.
The second set of measures compares the pairwise correlation matrix for both the actual and the filled values across all forecasters. They are the root mean squared correlation difference (RMSCD) and the mean absolute correlation difference (MACD). That is
where . This is equivalent to dividing the Frobenius norm of the difference by k and taking the square root.
The last set of measures compare the variances in the actual and filled predicted values for each forecaster. These are the root mean squared variance differences (RMSVD) and the mean absolute variance differences (MAVD):
These three metrics are chosen, as they are some of the most common inputs used in the forecast combination literature (e.g., see Genre et al. (Citation2013) or Bürgi and Sinclair (Citation2017) for a summary of methods).
5. Results
shows the simulation results for the GDP growth predictions in the Bloomberg survey for various approaches. As shown in the bottom panel of the table, aside from the simple average (SA), all approaches leave quite a few observations missing with the correlation-based approach leaving more than half of the observations remain missing, followed by the approaches taking the previous value where a bit over a quarter of observations remain missing.
Table 1. GDP prediction in the bloomberg survey.
Based on the root mean squared difference to the actual values, it is clear that using the previous value is not a good idea. The theoretically optimal regression-based approach derived above performs the best throughout and adding the surprise indices only makes it marginally worse. At the same time, most of the advantage relative to almost all other methods vanishes, once the observations that remain missing are filled by the simple average. However, the regression-based approaches and the average across approaches remain the best or very close to the best of all other approaches.
This table reports the simulation results for the various approaches with 10% of the predictions replaced with missing observations and 100 replications.
For the covariance (RMDCD), leaving the observation missing, using the previous value or highly correlated forecasters to fill it in are performing the worst, while the simple average, the average across approaches and the theoretically optimal approach perform best. In contrast to the RMSD, the filling the remaining missing observations with the simple average even improves the fit. For the variance (RMDVD), the simple average performs better than leaving any observations missing but filling the missing observations from the optimal approach with the simple average performs best together with the approach adding the surprise indices.
Comparing the different horizons shows that the approaches that are based on previous predictions (be it outright or based on regressions) perform better at longer horizons relative to the simple average. For example, based on the RMSD, the simple average performs slightly worse for the two-quarter ahead horizon, but the three methods based on the previous value all perform better.
Overall, for GDP growth it can be concluded that the theoretically optimal approach performs very well, and leaving the missing observations missing or filling them with the previous value does not perform particularly well.Footnote8
5.1. Other variables
shows the summary of the simulation results for the CPI and the unemployment forecasts in the Bloomberg survey and the GDP forecasts in the WSJ survey for various approaches. The first three columns show that the ranking of the root mean squared difference (MSD), the correlation (Cor) and the variance (Var) do not change the ranking substantially and leaving the observations missing or taking the previous value ranks leads to a high rank, meaning large differences. Conversely, the regression-based approaches appear to perform well consistently. The average across approaches performs very well for the variance and correlation but not for the MSD. The last two rows are the Friedman test whether the ranks are equal across methods as used in Stekler (Citation1987) or Batchelor (Citation1990) according to the formula
Table 2. Average ranks across variables.
where k is the number of ranks and either six or seven, depending on the column; n is 18 (6 comparisons times 3 variables); and is the average rank of method j across the 18 rankings.Footnote9 Based on the p-value, it is clearly rejected that the seven methods have the same ranks on average.
This table reports the average rank for the CPI and the unemployment forecasts in the Bloomberg survey and the GDP forecasts in the WSJ survey in each case of (Rank 1 means lowest value). The Chi-squared test reports the statistic, if all ranks are equal (degrees of freedom in brackets). The last column reports the average number of missing observations.
The last column shows the average number of missing observations across the 9 variations when the remaining missing observations are not replaced with the simple average. They roughly correspond to the columns 1, 3, and 5 in .
5.2. Absolute differences
So far, the analysis focused on the root mean squared differences between the filled in data and the actual. Instead of this, one could also use the mean absolute difference instead as defined above. The mean absolute difference penalizes large deviations by less than the root mean squared measures. shows the ranks of the various approaches for the three variables in as well as the GDP numbers in the Bloomberg survey, meaning that the average ranks include 24 variations instead of the 18 in the previous table. The results are qualitatively unchanged and the regression-based and average across all methods perform well, while leaving the observations missing or taking the previous value do not perform as well.
Table 3. Average ranks across variables-absolute.
This table reports the average rank for the GDP, CPI, and the unemployment forecasts in the Bloomberg survey and the GDP forecasts in the WSJ survey in each case of (Rank 1 means lowest value). The Chi-squared test reports the statistic, if all ranks are equal (degrees of freedom in brackets). The last column reports the average number of missing observations.
5.3. Overlapping survey
The previous section showed that leaving missing observations unchanged or replacing them with the previous prediction does not provide the best results based on the root mean squared difference, the variance, and the correlation. At the same time, while the simple average is not always the best performer, it has the advantage that it does not require any estimation or produce missing observations and can be applied to all horizons. One aspect not addressed so far is that with two surveys, it is possible to match forecasters from one to the other. Indeed, based on the sum of correlation for the horizons H0-H3 being larger than 3.85, there are 31 matches across the two surveys.Footnote10 This implies that the overlap between the two surveys is at least 50%. While it is possible to match individual forecasters by their name, this raises potential problems. For example, if the two values do not match, which prediction should be chosen? Similarly, the exact definitions of variables across surveys might not match perfectly. For example, the annual GDP number in the Bloomberg survey is percentage change of the average annual GDP level, while the WSJ survey uses the year over year change in the fourth quarter. To avoid these issues, only predictions for quarterly GDP growth are compared and the most highly correlated predictions are taken instead of matching the names.
shows the comparison of the simple average, the theoretically optimal approach and the correlation including the WSJ survey. Including the WSJ survey improves the fit of the correlation method, but it is still not as good as the other two approaches. This suggests that finding highly correlated predictions performs similar to the simple average even if there is substantial overlap.
Table 4. GDP prediction in the bloomberg survey with WSJ predictions.
Taking these aspects together, the theoretically optimal approach is the best approach considered here based on the statistics looked at, provided the furthest horizon is not important for the analysis. If all horizons are important, then the simple average might be the approach of choice. In either case, if an additional survey is available with an overlap in contributors, one might first use the overlap to fill in as many missing observations as possible.
5.4. Alternative missing data pattern
So far, it was assumed that the missing observation pattern is completely random. However, this is generally not the case and there is extensive entry and exit of forecasters. In turn, this could result in the above results not appropriately reflecting the missing observation pattern found in the data. In order to remedy this, an alternative simulation is run. Specifically, instead of randomizing which observations are missing, this simulation uses the missing observation pattern of the actual survey data and randomizes the forecasts instead. Specifically for GDP, 75 forecasters contributed to the Bloomberg survey during the sample period considered. For each period and horizon, the goal is to pick 75 random forecasts among the non-missing forecast which in turn constitutes the complete sample. Three of the methods looked at here use previous forecasts to impute the missing values. Completely random sampling the forecasts could cause these methods to perform worse than in actuality. As a consequence, the forecasts are sampled in groups to accommodate for this. Specifically, forecasts made for a specific period are grouped for the four horizons (current quarter through three-quarters ahead) and only groups retained which do not contain missing observations. The remaining groups are then sampled 75 times to produce the forecasts in the complete sample. Due to this sampling approach, each horizon is missing three periods of forecasts at the start of the sample (current quarter), at the end of the sample (three-quarter ahead) or split between the start and end of the sample (one and two-quarter ahead).
Once the complete sample has been produced, the three statistics to assess the performance of the six methods are calculated. Then, the missing observation pattern of the actual survey is used to make observations missing in the simulation. The missing observations are then filled in using the six methods and the match compared to the data without missing observations. This is repeated 100 times and the ranks of the six methods are shown in .
Table 5. Average ranks across variables-alternative.
This table reports the average rank for the GDP, CPI, and unemployment forecasts in the Bloomberg survey in each case of for the simulated forecasts (Rank 1 means lowest value). The Chi-squared test reports the statistic, if all ranks are equal (degrees of freedom in brackets). The last column reports the average number of missing observations.
Compared with the other simulation method shown in , leaving the missing observations unchanged or filling them in with the previous value does not perform well. The most consistent of the methods is the simple average, which is consistently at least the second-best method. The best approach depends on the specific metric looked at. Specifically, the theoretically optimal approach performs well based on the Mean Squared Difference while the correlation approach performs best when comparing the correlation matrix. For the variance, the simple average performs best.
5.5. Performance over time
One important question to address is whether the methods perform worse across time periods. In order to test this, an additional 10% of additional observations are deleted. Then, the missing observations are filled in using the various approaches. The root mean squared difference is then calculated for every period. This leads to a time-series for all the methods which can then be compared. plots these differences for the current quarter predictions where the remaining missing observations are filled in using the simple average across forecasters. As a result, there is no difference between the simple average approach and leaving the missing observations missing, so only the line for the former is shown. Overall, the pattern across time is similar to the pattern found so far. Specifically, using the previous value performs quite badly, while the regression-based approaches perform quite well. It is notable that the theoretically optimal approach remains close to the lower bound throughout the sample period, even during the period of large differences in 2008. This contrasts with the two correlation-based methods which perform particularly poorly during the 2008 period. The performances of the regression-based approaches are quite comparable to each other.
Footnote11The figure shows the root mean squared forecast difference for each survey round of eight methods to deal with missing observations. Note that the leave missing approach is excluded as it would be identical to the simple average approach.
Another key insight gained from is the changing performance of the correlation approach including the WSJ survey. Before 2009, this approach broadly shows a similar performance to the other approaches with a few outlier periods. After 2009 however, this approach performs the best across the board. This suggests that a substantial number of forecasters in the Bloomberg survey can be found in the WSJ survey after 2009 in periods, where they fail to contribute to the Bloomberg survey. This pattern is even more pronounced when using the mean absolute distance instead of the root mean squared distance as shown in . This sudden improvement in the performance of this method implies that it is important to check whether forecasters are present in other surveys. If this is the case, one should first fill in missing observations using the other survey before utilizing the theoretically optimal approach or one of the other regression-based approaches.
The figure shows the mean absolute forecast difference for each survey round of eight methods to deal with missing observations. Note that the leave missing approach is excluded as it would be identical to the simple average approach.
Other than this insight, the absolute difference are in line with the root mean squared difference, even if the approaches have a more comparable performance overall. For other horizons, the differences between the methods become even less pronounced that the advantage of using the WSJ survey after 2009 largely vanishes.Footnote12
6. Application forecast combination
Given the approaches to deal with missing observations lead to different outcomes in key statistics, this leaves the question as to how large the impact in follow-up applications is. While it is not possible to make a general statement for every eventuality, this section applies it to one specific case – forecast combination.
Specifically, for the 50 period sample 2002Q4-2015Q1 for GDP, the nine approaches are utilized and any remaining missing observations are replaced with the simple average.Footnote13 These nine separate samples each for the current quarter, one-quarter ahead and two-quarters ahead are then each split into a training sample of the first 40 periods and a forecast sample of the remaining 10 periods. Five real-time approaches are then utilized to combine the individual forecasts: the simple average of individual forecasts, the first principal component of all forecasts (PCA), forecasts are weighted according to the inverse of the prediction error variance, the five best forecasts based on the mean squared error and finally the subset approach proposed by Bürgi and Sinclair (Citation2017) where forecasters that are more accurate than the simple average at least 52.5% of the time are placed into a subset. The combined prediction then becomes the simple average across forecasters in the subset. The respective weights are estimated in the training sample and then applied to the forecast sample.Footnote14
Once the combined forecasts for these 24 data sets are calculated, they are compared to each other using mean squared forecast errors (see Equationequation 8(8)
(8) ) over the forecast sample. As the principal component can only be calculated for complete samples, the approach cannot be used if missing observations are left blank. shows the mean squared forecast errors for all cases for the current quarter horizon. It is clear from the graph that the relative performance of the forecast combination method depends on how the missing observations are dealt with. For example, the Bürgi and Sinclair (Citation2017) perform very well when the forecasts are left missing, but perform much worse under the correlation approach. While the regression-based approaches and the simple average appear to have smaller mean squared errors than the correlation methods or repeating the previous value, the difference is small and there is also quite a bit of variation.
The figure shows the mean squared forecast error for each of the five forecast combination methods and each of the eight methods to deal with missing observations. The combination methods are estimated over the first 40 periods and the resulting combination weights are then used for the last 10 periods in the sample from 2002Q4-2015Q1.
In order to test if the variation found in the mean squared errors for a specific method to fill in the missing observations is statistically significant, the Diebold and Mariano (Citation1995) test with the adjustments in Harvey et al. (Citation1997) is calculated. With the simple average as a benchmark, only the difference to the Bürgi and Sinclair (Citation2017) approach is significant at the 10% level when the missing observations are replaced with highly correlated values. For all other comparisons, the p-value is larger than 0.1.
The pattern for the one and two quarter horizons have similar properties to the current quarter horizon as shown in and . Specifically, the ranking of the different forecast combination methods changes, depending which approach is used for filling in missing observations. At the same time, these differences are not statistically significant based on the DM statistic. Indeed, the longer horizons make the difference even less pronounced.
Figure 4. One quarter ahead comparison of forecast combination methods.

Figure 5. Two quarter ahead comparison of forecast combination methods.

This result leads to two conclusions. First, the approach chosen to fill in missing observations can influence the relative performance of different forecast combination methods. However, given that only 10 pairwise observations were used to test for the statistical significance of the differences, the exact extent of this could not be fully determined. Second, the method to fill in the missing observations does not generally lead to a substantially better or worse performance of the combination approaches. Or put differently, while the ranking of the different combination methods might change with the approach used to fill in missing observations, it is not the case that picking a specific approach to fill in missing observations leads to a dramatic improvement or worsening of all combination approaches.
7. Conclusion
This paper compared the theoretically optimal approach to a number of approaches proposed in the literature to fill in missing values in surveys of professional forecasters. To this end, the approaches were compared based on the root mean squared and absolute difference to the actual predictions, how much they alter the correlation between forecasters and how much they alter the variance of the forecaster. Based on simulations, it was found that taking the previous value or not filling missing observations perform worse than the other approaches considered. This suggests that these two approaches should be avoided unless the analysis is specifically geared towards them. While there is no approach that is superior to the others in all potential use cases, the theoretically optimal approach usually performs quite well.
Beyond surveys of professional forecasters, the results presented here also have implications for any survey where the participants are asked questions about the same event in successive rounds or surveys where participants are repeatedly asked about the same horizon forecasts with a serially correlated underlying variable. Specifically, it was shown that taking into account the previous responses and how they typically change from one survey round to another can lead to more accurate imputation of missing observations than other methods. Further research might be able to assess whether this result also holds for surveys with a much larger number of participants.
The findings hold across several variables and forecasting surveys. At the same time, a substantial overlap between forecasting surveys was found. This has two important implications for the use of forecasting surveys. First, if one has access to multiple forecasting surveys for the same variables, one can use the overlap to reduce the number of missing observations before applying the theoretically optimal method to the remaining missing observations. Second, as the overlap between surveys for the same variable can be 50% and more, one might consider merging multiple surveys instead of testing a model for each survey separately. The overlap likely limits the usefulness of additional surveys as robustness checks and could limit the anonymity of contributors in certain surveys like the Survey of Professional Forecasters conducted by the Philadelphia Fed.
Acknowledgments
I would like to thank the participants of the 2021 Federal Forecaster Conference and the 24 Dynamic Econometrics Conference for their valuable comments.
Disclosure statement
No potential conflict of interest was reported by the author.
Additional information
Notes on contributors
Constantin Rudolf Salomo Bürgi
Constantin Rudolf Salomo Burgi, is an Assistant Professor of Economics at University College Dublin and holds a PhD from George Washington University. His research interest include expectation formation, monetary policy and international finance.
Notes
1 Mack and Skillings (Citation1980) implicitly replace missing values with the median and the robust measure in D’agostino et al. (Citation2012) would also leave gaps missing as it assumes that missing observations behave the same way as non-missing observations.
2 If the s are different, one would estimate
instead of the expression in Genre et al. (Citation2013). However, this method performs worse based on simulations than if the same
s are assumed. This suggests that the
s are relatively close to each other. These results are available upon request.
3 If was correlated with
for two forecasters i and j, one might be able to improve upon this prediction. However, this would require a two step approach where one needs to find the most correlated forecaster and then estimate the regression. As shown below with the covariance approach, this does not often produce better predictions.
4 Without this last assumption, a more standard noisy information model would result in longer horizons being just an autocorrelation coefficient times the next shorter horizon (e.g., see Coibion and Gorodnichenko (Citation2015).
5 Another approach used in Steira (Citation2012) proposes to make a linear projection of the quarterly forecast path to fill in missing observations at the longest horizon. Since the main objective is to fill in missing observations due to non-participation in a specific period, this approach is not suitable here.
6 The results are similar for 1% or 5% of observations being replaced with missing and for 20% of the observations, this causes issues as some forecasters randomly might not have any observations anymore.
7 While three quarter ahead predictions are also available, these are only used for the approaches that use the previous prediction.
8 Note that the differences between the approaches are not statistically significant based on a Diebold-Mariano test.
9 The six comparisons are the columns in and the three variables are the CPI and unemployment in the Bloomberg survey and the GDP for the WSJ survey.
10 The 3.85 threshold is chosen to ensure unique matches. There are several forecasters that are in both surveys with a lower sum due to some values not matching across the two surveys.
11 Correlation corresponds to the correlation approach using the same survey while Correlation 2 utilizes the WSJ survey to match correlations.
12 The graphs for the other horizons are available upon request. One could for example use the test by Giacomini and Rossi (Citation2010) to compare the performance between the approaches, but since they are very much clustered together, the power is likely insufficient to distinguish their performances.
13 The nine approaches are leaving missing, the simple average, previous value, theoretically optimal approach, Lahiri et al., optimal approach with surprise index, correlation within the BBG survey, correlation across BBG and WSJ survey, and the average across all methods.
14 The exception are the principal component analysis and the simple average for which the sample was not split.
References
- Andrade, P., & Le Bihan, H. (2013). Inattentive professional forecasters. Journal of Monetary Economics, 60(8), 967–21. https://doi.org/10.1016/j.jmoneco.2013.08.005
- Andridge, R. R., & Little, R. J. (2010). A review of hot deck imputation for survey non- response. International Statistical Review, 78(1), 40–64. https://doi.org/10.1111/j.1751-5823.2010.00103.x
- Batchelor, R. A. (1990). All forecasters are equal. Journal of Business & Economic Statistics, 8(1), 143–144. https://doi.org/10.1080/07350015.1990.10509784
- Bates, J. M., & Granger, C. W. (1969). The combination of forecasts. The Journal of the Operational Research Society, 20(4), 451–468. https://doi.org/10.1057/jors.1969.103
- Bürgi, C. (2017). Bias, rationality and asymmetric loss functions. Economics Letters, 154, 113–116. https://doi.org/10.1016/j.econlet.2017.03.002
- Bürgi, C. (2020). Expectation Formation and the Persistence of Shocks. Working Papers 2020-005. The George Washington University, Department of Economics, H. O. Stekler Research Program on Forecasting.
- Bürgi, C., & Sinclair, T. M. (2017). A nonparametric approach to identifying a subset of forecasters that outperforms the simple average. Empirical Economics, 53(1), 101–115. https://doi.org/10.1007/s00181-016-1152-y
- Capistrán, C., & Timmermann, A. (2009). Forecast combination with entry and exit of experts. Journal of Business & Economic Statistics, 27(4), 428–440. https://doi.org/10.1198/jbes.2009.07211
- Coibion, O., & Gorodnichenko, Y. (2015). Information rigidity and the expectations formation process: A simple framework and new facts. The American Economic Review, 105(8), 2644–2678. https://doi.org/10.1257/aer.20110306
- Conflitti, C., De Mol, C., & Giannone, D. (2015). Optimal combination of survey forecasts. International Journal of Forecasting, 31(4), 1096–1103. https://doi.org/10.1016/j.ijforecast.2015.03.009
- D’agostino, A., McQuinn, K., & Whelan, K. (2012). Are some forecasters really better than others? Journal of Money, Credit, and Banking, 44(4), 715–732. https://doi.org/10.1111/j.1538-4616.2012.00507.x
- Diebold, F. X., & Mariano, R. S. (1995). Comparing predictive accuracy. Journal of Business and Economic Statistics, 13(3), 253–263. https://doi.org/10.1080/07350015.1995.10524599
- Diebold, F. X., & Shin, M. (2019). Machine learning for regularized survey forecast combination: Partially-egalitarian lasso and its derivatives. International Journal of Forecasting, 35(4), 1679–1691. https://doi.org/10.1016/j.ijforecast.2018.09.006
- Genre, V., Kenny, G., Meyler, A., & Timmermann, A. (2013). Combining expert forecasts: Can anything beat the simple average? International Journal of Forecasting, 29(1), 108–121. https://doi.org/10.1016/j.ijforecast.2012.06.004
- Ghysels, E., & Wright, J. H. (2009). Forecasting professional forecasters. Journal of Business & Economic Statistics, 27(4), 504–516. https://doi.org/10.1198/jbes.2009.06044
- Giacomini, R., & Rossi, B. (2010). Forecast comparisons in unstable environments. Journal of Applied Econometrics, 25(4), 595–620. https://doi.org/10.1002/jae.1177
- Grishchenko, O., Mouabbi, S., & Renne, J. -P. (2019). Measuring inflation anchoring and uncertainty: A US and euro area comparison. Journal of Money, Credit, and Banking, 51(5), 1053–1096. https://doi.org/10.1111/jmcb.12622
- Harvey, D., Leybourne, S., & Newbold, P. (1997). Testing the equality of prediction mean squared errors. International Journal of Forecasting, 13(2), 281–291. https://doi.org/10.1016/S0169-2070(96)00719-4
- Issler, J. V., & Lima, L. R. (2009). A panel data approach to economic forecasting: The bias-corrected average forecast. Journal of Econometrics, 152(2), 153–164. https://doi.org/10.1016/j.jeconom.2009.01.002
- Kenny, G., Kostka, T., & Masera, F. (2015a). Can macroeconomists forecast risk? Event- based evidence from the euro-area SPF. International Journal of Central Banking, 11(4), 1–46.
- Kenny, G., Kostka, T., & Masera, F. (2015b). Density characteristics and density forecast performance: A panel analysis. Empirical Economics, 48(3), 1203–1231. https://doi.org/10.1007/s00181-014-0815-9
- Lahiri, K., Peng, H., & Zhao, Y. (2017). Online learning and forecast combination in unbalanced panels. Econometric Reviews, 36(1–3), 257–288. https://doi.org/10.1080/07474938.2015.1114550
- Little, R. J., & Rubin, D. B. (2019). Statistical analysis with missing data (Vol. 793). John Wiley & Sons.
- Mack, G. A., & Skillings, J. H. (1980). A friedman-type rank test for main effects in a two-factor anova. Journal of the American Statistical Association, 75(372), 947–951. https://doi.org/10.1080/01621459.1980.10477577
- Poncela, P., Rodríguez, J., Sánchez-Mangas, R., & Senra, E. (2011). Forecast combination through dimension reduction techniques. International Journal of Forecasting, 27(2), 224–237. https://doi.org/10.1016/j.ijforecast.2010.01.012
- Scotti, C. (2016). Surprise and uncertainty indexes: Real-time aggregation of real-activity macro-surprises. Journal of Monetary Economics, 82, 1–19. https://doi.org/10.1016/j.jmoneco.2016.06.002
- Sheng, X., & Wallen, J. (2014). Information rigidity in macroeconomic forecasts: An international empirical investigation. [ Unpublished Manuscript]. American University Washington,
- Steira, Ø. (2012). How accurate are individual forecasters? an assessment of the survey of professional forecasters. Working Paper. SNF.
- Stekler, H. O. (1987). Who forecasts better? Journal of Business & Economic Statistics, 5(1), 155–158. https://doi.org/10.1080/07350015.1987.10509571
- Zhao, Y. (2020). The robustness of forecast combination in unstable environments: A monte carlo study of advanced algorithms. Empirical Economics, 61(1), 1–27. https://doi.org/10.1007/s00181-020-01864-w
A Absolute difference for alternative missing data pattern
repeats the analysis in but uses the absolute measures instead of the squared measures.
Table 6. Average ranks across variables absolute.
This table reports the average rank for the GDP, CPI and unemployment forecasts in the Bloomberg survey in each case of (Rank 1 means lowest value). The Chi-squared test reports the statistic, if all ranks are equal (degrees of freedom in brackets). The last column reports the average number of missing observations.