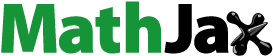
ABSTRACT
This paper examines how the corporate life cycle affects credit scoring. Previous empirical studies have shown that the life cycle has an impact on financial policies, creditworthiness, risk and performance. This study utilizes panel data of U.S. listed companies for the period 1985–2017. The Dickinson model, which divides the life cycle into four stages (introduction, growth, mature, and decline), is used. The Probit model is employed to investigate this relationship. The findings show that firms in the introduction, growth, and maturity stages have a favorable and significant impact on the likelihood of a positive credit rating change (upgrade). Conversely, firms in the decline stage show a negative relationship with credit rating positive upgrades. This suggests that credit rating agencies consider a firm’s life cycle status. Therefore, firms should strive to reach the growth and mature phases in order to benefit from higher credit ratings.
KEYWORDS:
1. Introduction
Credit rating agencies, such as S&P, assign credit rating scores to most publicly traded U.S. firms based on their perception of the firms’ creditworthiness and credit quality. These agencies rely on three major categories of information to assign credit ratings: the firm’s financial data, its corporate governance mechanism, and the macroeconomic conditions in which it operates (see AlHares et al., Citation2018). A vast amount of empirical literature has been published that aims to determine the factors affecting firm rating decisions. In their survey, Graham and Harvey (Citation2001) indicate that credit rating is one of the most important factors that affect the financing decision of firms because it can be a good indicator of a firm’s financial creditworthiness. Credit rating is one of the most important factors that is used by both issuers and investors of bonds to determine risk premium and bond marketability. Thus, it is very important to examine the factors that affect a credit rating decision. Previous researches have demonstrated that credit ratings are influenced by a variety of factors, including capital structure (Kisgen, Citation2006), cost of equity (Hasan et al., Citation2015), bankruptcy and financial distress (Akbar et al., Citation2020; Al‐Hadi et al., Citation2019); operational leanness (Bendig et al., Citation2017), earnings management (Hill et al., Citation2019; Kim et al., Citation2013), corporate governance and ownership structure (Ashbaugh-Skaife et al., Citation2006; Kiesel & Lücke, Citation2019; Lin et al., Citation2020), sentiments-risk relationship (Akbar et al., Citation2021) financing restructuring decision (Akbar et al., Citation2022); macroeconomic factors (Boumparis et al., Citation2019; Figlewski et al., Citation2012), auditing firm (Bhandari & Golden, Citation2021), capital intensity (Attig et al., Citation2013), working capital management (Abuhommous et al., Citation2022); and lending spread (Amin et al., Citation2023). These studies highlight the significant impact of the firm life cycle on financial decisions, investment choices, profitability, and risk. These factors collectively influence firm creditworthiness, which in turn impacts firm credit ratings.
Building upon the existing literature, our study contributes significantly by investigating the association between the corporate life cycle and credit scoring. We aim to examine whether a firm’s credit rating is influenced by its current stage in the life cycle and whether downgrading or upgrading is affected by the life cycle. Through this exploration, we provide valuable insights to the field.
The “dynamic resources-based view” of the firm indicates that a firm’s competitive advantage is derived from the interaction between the resources and capabilities it possesses. The firm’s competitiveness evolves over time due to the evolution of its resources and capabilities. Specifically, the dynamic resources-based theory discusses how the life cycle (introduction, development, and maturity) of a firm’s capabilities may positively or negatively impact its performance (see Hasan et al., Citation2015).
This paper demonstrates how the life cycle theory provides valuable insights into the shifts in credit ratings, driven by factors such as competitiveness, financial performance, debt levels, business stability, and capabilities that transform across life cycle stages. Credit scoring agencies take these considerations into account when assessing a firm’s creditworthiness. According to this theory, the firm’s life cycle comprises four distinct phases: introduction, growth, maturity, and decline.
The life cycle theory postulates that a firm’s characteristics, including aspects like asymmetric information, risk, agency costs, and creditworthiness, vary across the different stages of the firm’s life cycle. Consequently, the firm’s credit rating undergoes changes.
Firms in the introduction phase often grapple with issues of information asymmetry, coupled with uncertainty about future operating and cash flows. During this stage, these firms typically encounter challenges such as low operating cash flow, negative or modest profit margins, and an elevated likelihood of debt default. Consequently, companies in the introduction stage tend to hold lower credit ratings. Similarly, firms in the decline phase contend with heightened competition, reduced productivity, notably low cash flow per customer, and declining sales. In contrast, the growth and maturity phases yield greater profitability for a firm, characterized by increased revenue, heightened employee productivity, stable revenues and operational cash flows, and robust market acceptance of their products.
Recent research has been delving into the relationship between the firm’s life cycle and finance and accounting decisions (refer to Wahlen et al., Citation2018, pp. 128–131). Dickinson (Citation2011) utilizes the cash pattern involving operating, investing, and financing cash flows to measure the firm life cycle. She suggests that categorizing a firm’s life cycle based on the cash flow pattern can aid creditors and analysts in identifying potential risks associated with the firm.
Our findings make a valuable contribution to the expanding body of literature exploring the firm’s life cycle. Firstly, we extend prior research that focused on how a firm’s characteristics influence credit ratings. This comprehensive perspective improves our understanding of the interplay between the life cycle and market evaluation of the firm-specific risk (credit rating). Secondly, we document the impact of the firm’s life cycle on changes in credit rating (upgrading or downgrading). We find that firms in the introduction, growth, and mature stages have a positive impact on the likelihood of experiencing a positive change (upgrading) in credit ratings. This research aims to extend our understanding of the relationship between a firm’s life cycle and its credit rating. This understanding help the firm’s managers in comprehending the relationship between their strategic decisions and the current stage of the firm’s life cycle, which in turn affects the market valuation of the firm’s risks. Therefore, the efforts firm’s management should be directed toward reaching the maturity stage, as it increases the likelihood of obtaining a higher credit rating.
Using a sample of listed firms in the U.S. stock market from the period 1985 to 2017, this paper unveils a significant impact of the firm’s life cycle on its credit rating. The findings indicate that the firm’s risk undergoes changes as it progresses through different stages. The primary contribution of this paper lies in enhancing our comprehension of the interplay between the corporate life cycle and credit scoring.
The subsequent sections of this paper are structured as follows: Section 2 offers a comprehensive review of the existing literature and delineates the process of hypothesis development. Section 3 presents the data and descriptive statistics. In Section 4, the econometric specifications and methods are outlined. The analysis and interpretation of the results are provided in Section 5. Lastly, Section 6 concludes the paper by summarizing the research findings and their implications.
2. Literature review and hypotheses
Numerous studies have investigated the impact of a firm’s life cycle on the financial performance, risk, management practices of the firm. Jenkins et al. (Citation2004) highlight that firms adopt different market and management strategies at different life phases. They emphasize that cost efficiency or product differentiation strategies are implemented based on the life stage, wherein firms tend to incur losses during early and introduction phases and generate profits during maturity. Agarwal and Audretsch (Citation2001) explore the relationship between firm size and survival likelihood, considering the industry life cycle as a moderating factor. Their findings propose that small entrant firms face lower survival prospects, while mature firms are more likely to survive. Hasan and Cheung (Citation2018) investigates the association between corporate life cycle phases and firm capital. He argues that corporate life cycle has implications for strategic management decisions, productivity, and efficiency.
In accordance with the life cycle hypothesis, a firm’s journey can be classified into four distinct stages: introduction, growth, maturity, and decline. The introduction stage, the initial step of a company’s life cycle, indicates its market entry. Firms are dealing with an asymmetric information problem and limited human capital investment at this stage. These variables contribute to investment inefficiency (Ahmed et al., Citation2020) which promotes a prudent capital structure strategy that relies more on equity financing (Hasan & Cheung, Citation2018). Furthermore, organizations are more likely to use earnings management practices (Hussain et al., Citation2020) and have lower levels of organizational capital (Hasan and Habib, Citation2017). As a result, asymmetric information has a substantial impact on a firm’s trade credit strategy. Consequently, the presence of asymmetric information significantly shapes a firm’s trade credit strategy. This leads to a preference for trade credit over more expensive bank loans. In this context, Hasan et al. (Citation2021) emphasize that firms in the introduction stage often encounter financial constraints, requiring their reliance on trade credit due to elevated risk and operational uncertainty. Firms in the introduction phase experience more idiosyncratic and cash flow volatility, information asymmetry, and a higher possibility of facing bankruptcy risk in comparison to firms in the growth and mature phases (Habib & Hasan, Citation2017). This stage in the life cycle elevates the potential for financial distress (Al‐Hadi et al., Citation2019). Furthermore, firms in the introduction phase incur greater lending spreads than their mature-phase counterparts (Amin et al., Citation2023). These evidences have a negative impact on the creditworthiness of the firm, leading to lower credit ratings.
According to the life cycle theory, firms proceed from the introduction stage to the growth stage, and then to the mature stage. During the growth stage, firms expand in terms of sales, demand, market share, and profitability (Dickinson, Citation2011). Furthermore, this growth is frequently differentiated by improved product differentiation. Firms in the mature stage maintain a more consistent market share while experiencing slower growth in sales, revenue, and profits. Firm’s risk is reduced during growth and mature stages, as indicated by lower firm-specific risk, information asymmetry, cash flow volatility, and financial distress (Dickinson, Citation2011; Habib & Hasan, Citation2017; Hasan & Habib, Citation2023; Hasan et al., Citation2021). Firms in these stages enjoy financial stability, exhibiting low probabilities of bankruptcy and financial distress (Al‐Hadi et al., Citation2019; Akbar et al., Citation2019). The cost of debt is low, and equity costs are also reduced (Amin et al., Citation2023; Hasan et al., Citation2015). Additionally, firms in growth and maturity stages have a good financial reputation (Akbar et al., Citation2022). Mature firms exhibit more stable dividend policies and higher returns on investment (DeAngelo et al., Citation2006). Furthermore, Wang et al. (Citation2020) find that mature firms, benefiting from a surplus of funds, can make excessive investments in working capital without experiencing a decline in profitability. Additionally, investment efficiency begins to improve during the growth and mature stages, with mature firms having the maximum level of efficiency (Ahmad et al., 2021). Consistent findings within the relevant literature consistently indicate that a firm’s creditworthiness improves as it progresses from the introduction stage through the growth stage to the mature stage. Consequently, firms in these stages demonstrate heightened market stability, sufficient resources, considerable expertise, operational and financial stability, and a track record of higher profitability (Habib & Hasan, Citation2019; Akbar et al., Citation2019). Therefore, we anticipate that firms in this life cycle stage will exhibit an elevated level of creditworthiness, leading to higher credit ratings.
The last stage of the firm’s life cycle is the decline stage occurs when it has significantly low profit margins, operational and financial losses, reduced sales volume, and the need to cut investments and sell assets owing to decreased demand. During this stage, the firm must choose between two options. The first alternative entails shutting down operations and selling the company. Alternatively, the firm can choose to reshape its competitive advantage through innovation and so begin a new life cycle. Overall, this stage is characterized by heightened risk and operational uncertainty (Dickinson, Citation2011), compounded by low investment efficiency (Ahmad et al., 2021) and an elevated likelihood of bankruptcy risk (Al‐Hadi et al., Citation2019; Akbar). The shake-out stage of the company’s life cycle occurs when the firm’s profit margin ratio is low due to poor sales, alongside a low and volatile cash flow level (Hasan et al., Citation2021). However, there is a lack of theoretical support for the firm’s characteristics during the shake-out stage. In line with the work of Hasan et al. (Citation2015), we utilize the shake-out stage as a base to compare our results.
The fundamental premise that forms the foundation of our research is the association between corporate life cycle stages and the market’s perception of a firm’s creditworthiness, subsequently influencing credit scoring. The primary goal of this paper is to illustrate that the corporate life cycle can lead to an increase or decrease in a firm’s credit scoring by influencing the perceived risk of financial bankruptcy. As a result, our paper establishes the following hypotheses:
H1.
Firms in the introduction, growth, and mature life stages have a positive relationship with credit rating upgrading.
H2.
Firms in the decline stage have a negative relationship with credit rating upgrading.
H3.
Firms in the introduction, growth, and mature stages of their life cycle have a negative impact on the credit rating downgrading.
H4.
Credit rating will decrease from year to year (downgrading) for firms in the decline stage of their life.
3. Data and descriptive statistics
3.1. The sample and the data
The sample is derived from the annual Compustat database accessed through WRDS, where S&P’s long-term issuer credit ratings are available for the period 1985–2017. It’s important to acknowledge that due to data availability constraints, the last year included in our regression analysis is 2017. It is worth noting that our analysis involves the loss of three years’ worth of observations. This is mostly due to the need to calculate changes in the dependent variable, incorporating the previous year’s loss as a control variable, and use lagged values of the independent variable for robustness checks. Consistent with previous studies, financial firms with SIC codes between 6000 and 6999 are excluded from the study, as well as firms with missing observations of total assets. The dataset comprises a total of 4,366 firm observations. The distribution of the data, categorized according to Fama and French’s (Citation1997) industry classification, is detailed in .
Table 1. Sample distribution by industry classification.
3.2. Variable definitions and empirical methods
3.2.1. Dependent variable measurement
The first task in this study is to calculate the dependent variable, which is the S&P’s long-term issuer credit rating. This rating is used to evaluate the obligator creditworthiness for the long-term horizon, where grades are allocated according to the current and future ability of the obligator to fulfil its debt obligations. The rating is assigned on an ordinal scale, ranging from AAA for firms that are extremely strong through to the D assigned for firms in default. In line with previous studies (Attig et al., Citation2013; Dorfleitner & Grebler, Citation2020), we use eight categories (AAA, AA, A, BBB, BB, B, CCC, and CC) to represent the credit rating where the value of 8 is assigned for firms with an AAA rating, and the value of 1 for firms with a C rating. Firms with a rating below CC are excluded from the research because they are already bankrupt or have filed for bankruptcy. The total number of observations equals 4,366 firm. The next step is to calculate the main dependent variable of this study, which is the change of credit rating (RATINGCHANGE), where it takes the value of 1 if the firm’s credit rating increases from the previous year to the current year, and zero otherwise.
For consistency with previous studies shows the distribution of the firms across the eight credit rating categories – firm-years with an AAA credit rating only equate to 1.32% of total observations, while the most common credit rating is BBB with 26.25% of total observations, and the percentage of total firm-years considered to be of investment-grade is 52.01%, which is less than the 57.0% found by Alissa et al. (Citation2013).
Table 2. Sample distribution by credit rating.
3.2.2. Independent variable of interest
The subsequent phase involves the computation of the primary independent variable, namely the firm life cycle. Gort and Klepper (Citation1982) proposed a classification of a firm’s life cycle into five distinct stages: the introduction stage, where firms introduce a new product to the market (the first producer); the growth stage, characterized by a rapid increase in product producers; the maturity stage, where the net number of new firms entering the market and those firms leaving the market is approximately zero; the shake-out phase, where the number of firms leaving the market is higher than the number of firms entering the market, thus the total number of firms in the stage starts to decline; the decline phase, where without new entrants. This framework provides a structure for understanding a firm’s evolution over time. To establish a connection between a firm’s life stage and its external estimation of creditworthiness (credit rating), we adopt the methodology introduced by Dickinson (Citation2011). This procedure entails using a firm’s cash flow as a proxy for its creditworthiness., such as profitability, growth, and risk, all of which are influenced by the various stages of a firm’s life cycle. Following Dickinson (Citation2011) and Hasan et al. (Citation2015), this paper uses the cash flow from operating activities (OCF), cash flow from investing activities (ICF), and cash flow from financing activities (FIN) to classify firms based on their life cycle stages. The relationship between cash flow and firm life cycle stage will be as follows:
Introduction stage: where OCF < 0, ICF < 0, and FIN > 0;
Growth stage: where OCF > 0, ICF < 0, and FIN > 0;
Mature stage: where OCF < 0, ICF < 0, and FIN < 0;
Decline stage: where OCF < 0, ICF > 0, and FIN≤ or ≥ 0;
Shake-out stage: all firms that not met the above-mentioned rules.
The matrix in shows the distribution of credit rating for firms in each stage of the firm life cycle; consistent with Dickinson (Citation2011) and Hasan et al. (Citation2015), the largest number of firms are allocated to the maturity phase with 49% of observations, followed by growth with 30.2%, and then introduction with 4.5% of firms, while the decline stage has the lowest percentage at 2%. The overall result shows that the most AAA credit ratings are for firms in the maturity and growth phases of the life cycle, while we cannot find firms from the introduction and decline stages that have such a rating. Furthermore, firms in the maturity and growth stages with credit ratings of AA, A, or BBB have the highest percentage (e.g., in the AA credit rating column the percentage of mature firms with an AA credit rating is 7.4% of total observations, and the highest percentage of firms in the BBB category is for maturity firms, and so on). For the B rating we can see that firms in the introduction phase have the highest percentage, where 54.2% of total observations have a B rating, and firms in the decline stage have 52.8%. Consistent with their lower riskiness, mature and growth firms have the lowest percentage of low credit ratings, where only 1.3% of their total observations have a CCC credit rating, and this is lower still for a CC credit rating. Moreover, firms in the introduction and decline stages have the highest percentage of firms having either a CC or a CCC credit rating; for example, the percentage having a CCC rating is 9.9% for the introduction stage and 14.2% firms for the decline phase. We can also observe that the credit rating considers firms in the decline stage to be riskier than those in the introduction stage, given that firms in the introduction stage have the potential to transfer to the growth stage. However, the market might view firms in the decline stage as facing challenges in restructuring themselves to return to earlier phases. Hence, it can be concluded that the market considers it to be more feasible for firms to progress toward the next life stage than to move backward to a preceding one. shows that firms in the growth and maturity stages have high correlation in terms of their credit rating, where most of these firms have a BBB rating or higher. On the other hand, firms in the introduction and decline stages demonstrate a similar trend in their credit rating distribution, with a majority of observations falling below a B credit rating. presents graphically the numbers shown in .
Table 3. Descriptive statistics.
3.2.3. Control variables
The main variables that may explain the observed relationship with credit rating are included in the regression model. Based on the theoretical and empirical results from existing literature (Ashbaugh-Skaife et al., Citation2006; Attig et al., Citation2013; Bendig et al., Citation2017), we add seven control variables (size, interest coverage, financial obligation, capital intensity, losses in the previous year, auditing firm, and subordinate debt). Firm size (SIZE) is measured by the natural logarithm of total assets, where large firms are less risky since the bankruptcy cost is high and large firms tend to have a portfolio of products, which reduces product risk. Interest coverage ratio (INTCOV) is measured by dividing operating income before depreciation by total interest expenses, where firms with higher earning to obligation financial charges may have a lower probability of not paying the financial charges to obligators. Debt ratio (DEBT) is measured by the ratio of long-term financial obligation over total assets, where a higher debt ratio may increase the firm’s riskiness and decrease the credit rating. Corporate capital intensity (INTENSITY) measures the amount of fixed assets such as property, plant, and equipment, where firms with a higher fixed assets ratio can use them as collateral for creditors. The variable “Net income losses” (LOSS) serves as a binary indicator with a value of 0 assigned to firms that have losses both in the preceding years and the current year. This variable captures the scenario where firms face challenges in generating sufficient cash flow to meet their financial commitments, which increases the firm’s riskiness. The presence of losses underscores the heightened riskiness of such firms. In line with the rationale that external auditors play a role in enhancing transparency and mitigating risk, the variable “Auditing firm” (AUDIT) is introduced. This variable assumes a value of 1 if the firm’s auditors belong to the prestigious “big four” auditing firms, renowned for their comprehensive disclosure practices. Conversely, it assumes a value of 0 if the auditors fall outside this category. Firms with subordinated bonds (SUBORD) are considered riskier; thus, a dummy variable is created for firms that have issued subordinated bonds. We also control for the industry-specific effect by including a dummy variable for each industry based on the Fama and French (Citation1997) classification, since the default risk is not same across industries.
3.3. Descriptive statistics
reports the descriptive statistics for the main variable in the regression model. Panel A shows the descriptive statistics for all observations in the sample firm-years, while Panel B shows the descriptive statistics for firm-years in each stage of the firm life cycle. In Panel A, the dataset of firm-year observations reveals an average coverage ratio (INTCOV) of 7.625. This finding implies that, on average, firms’ earnings exceed the amount spent on interest payments. The average size of firm in the total assets sample is $10.49 billion and the median is $2.44 billion, indicating that most of the firms are relatively large (all firms included in the sample are publicly traded and have issued long-term debt). The average debt ratio is 33.2%, and average capital intensity is 70.7%, which indicates that the sample consists of financially healthy firms. In Panel B life cycle-wise statistics are presented for different control variables with life-cycle stages. In all models we find that the largest average firm size is for firms in the growth and mature stages, with the values being 7.880 and 8.174 respectively, whereas firms in the introduction and decline stages have the lowest average size, with values of 6.827 and 6.878 respectively. This indicates that the firms in the introduction and decline stages are smaller than in the growth and mature stages. Also, we can see the value of interest coverage ratio (INTCOV) for growth and mature firms is 6.783 and 9.238 respectively, which is higher than for introduction and decline firms, which are 2.736 and 2.562 respectively. This means that introduction and growth firms generate lower earnings to cover their interest-bearing debt than that of growth and mature firms, which indicates that they are riskier than the other stages. In addition, we find that the capital intensity ratio (INTENSITY) is high for growth and mature firms, 70.9% and 74.6% respectively, while the lowest capital intensity rations are 48.7% and 50%, for introduction and decline firms respectively. The riskiness of firms with lower capital intensity is higher since they may replace secure assets with high risk assets. For firms that have losses, the average (LOSS) for growth and mature firms is 24.7% and 15.7% respectively, whereas the growth and decline firms have the highest loss percentage, at 65.9% and 65.7% respectively. In general, the overall descriptive statistics in Panel B show that growth and mature firms have low riskiness ratios, where they have large size, high coverage ratio, low debt ratio, high capital intensity, and low rate of loss percentage per year. On the other hand, the descriptive statistics show that firms in the introduction and decline stages are relatively riskier compared to firms in other stages. These firms tend to be smaller in size, have lower earning to interest ratios, higher debt levels, lower capital intensity, and a higher percentage of losses. Furthermore, all of the aforementioned variables that represent the firm’s riskiness show progressive change as we move through the firm life cycle. The mean of firm size (SIZE) starts to increase as we move from introduction to growth, and increases again from growth to mature, but decreases as we progress from mature toward firms in the decline stage. We find a similar pattern for all the other variables; coverage ratio (INTCOV) is increasing as we progress from introduction to growth and mature, but decreases as firms move from mature to decline, which also reflects the change of firm riskiness between firm life stages. The financial obligation ratio (DEBT) tends to be higher for firms in the introduction stage, and decreases as we move from firms in the introduction stage toward mature firms. However, it increases again for mature firms. Interestingly, decline stage firms have the lowest debt ratio compared to introduction stage firms, as they rely more on equity capital rather than debt financing. We find the same pattern for INTENSITY and LOSS variables, where growth and mature firms have higher (lower) values, compared with firms in the introduction and decline stages that have lower (higher) values, supporting that these latter firms are riskier. We also test for the correlation coefficient between the variables; the correlation is not high, thus the multicollinearity is not a concern.
Table 4. Tc.
4. Econometric specifications and methods
Kim et al. (Citation2013) and Bendig et al. (Citation2017) demonstrate that credit rating agencies conduct comprehensive analysis before assigning a credit rating to a firm or changing a firm’s credit rating. Thus, the following model examines whether a firm’s life cycle has an effect on credit rating changes, as shown in Equation 1:
We estimate two regressions: the first estimation is for a positive credit rating change (Positive RATINGCHANGE), where it takes the value of 1 if the firm’s credit rating increases (upgrading) from year to year, and zero otherwise; the second estimation is for a negative credit rating change (Negative RATINGCHANGE), where the value of 1 is assigned for firms whose credit rating decreases from year to year (downgrading), and zero otherwise.
We also examine whether the firm’s life cycle affects its long-term credit rating. Since the dependent variable has a discrete value, where it takes a value between 1 and 8, we follow previous research econometric estimation (e.g., Alissa et al., Citation2013; Papadimitri et al., Citation2020) and use an ordered Probit model because it is more suitable than an ordinary least squares model; thus, we model the credit rating as a function of firm life cycle and firm- and industry-specific characteristics, as shown in Equation 2:
Where CREDITRATING is the firm’s credit rating of AAA, AA, A, BBB, BB, B, CCC, or CC, we replace these values by 8, 7, 6, 5, 4, 3, 2, or 1 respectively.
5. Empirical findings
5.1. Life cycle stage and credit rating changes
This section presents the results of investigating the impact of the corporate five life cycle stages on the change of credit rating, and also examines whether a firm’s life cycle stage influence credit rating. Dickinson (Citation2011) proposes five life cycle stages: introduction, growth, mature, decline, and shake-out. The shake-out stage is dropped from the regression to overcome the problem of multicollinearity. In addition, the theory behind the shake-out stage is ambiguous, so has been dropped from the regression (see Dickinson et al., Citation2018; Hasan et al., Citation2015). To control for the year-specific effect, year dummies are added to the model. Also, the industries may differ in terms of their risk, so dummies are included to control for the industry-specific effect. All standard errors are robust and firm-clustered to control for heteroscedasticity.
Hypothesis 1 states that introduction, growth, and mature life stages have a positive relationship with credit rating upgrading. As shown in Panel 1, firms in the introduction, growth, and mature stages have a positive and significant impact on the probability of the firm to have a positive change (upgrading) of credit rating (P-value < 0.000). Hence, the credit rating of a firm is enhanced if the firm is in the following firm’s life cycle stages: introduction, growth, and mature. This supports the main argument that risk for firms in the introduction, growth, and mature stages is decreasing over years, so the credit rating is upgraded by credit rating agencies over years. Hypothesis 2 argues that firms in the decline stage have a negative relationship with credit rating upgrading. The result of firms in the decline stage shows a negative relationship with positive change of credit rating (P-value < 0.01), which indicates that firms in the decline stage have a negative impact on upgrading of credit rating. Since the riskiness of firms in the decline stage increases over years, their credit rating will not be enhanced. Therefore, Hypothesis 2 is confirmed. To examine whether life cycle stage will enhance the explanatory power of the model, Bayesian Information Criteria (BIC) is used to compare the two models. The results of BIC for the model where firm life cycle is added to the control variables support that the firm life cycle stages will add more explanatory power if added to the model; the BIC value is higher by 198.96 for the model including the firm life cycle variables. Column 2 shows the estimation results for the model with control variables; in unreported results, SIZE, INTENSITY, and LOSS negatively affect the positive change of credit rating While INTCOV and DEBT have a positive impact on credit rating, AUDIT and SUBORD have a weak relationship with positive change of credit rating.
Table 5. The impact of firm life cycle on positive credit rate change,
shows the impact of a firm’s life cycle stages on the downgrading of a firm’s credit rating. Hypothesis 3 proposed that firms in the introduction, growth, and mature stages of their life cycle have a negative impact on the credit rating downgrading. The results in Column 1 show that the firm’s life cycle stages (Introduction, Growth, and Mature) have a negative and statistically significant impact (P-value < 0.01) on the probability of negative change (downgrading) of credit rating, since these stages may not have a negative impact on the firm’s creditworthiness in the future. Therefore, the results support Hypothesis 3.
Table 6. The impact of firm life cycle stage on negative credit rate change, .
Hypothesis 4 proposed that credit rating will decrease from year to year (downgrading) for firms in the decline stage of their life. also shows that firms in the decline stage have a positive impact on the probability of the firm to have downgrading from the previous year’s credit rating. Thus, Hypothesis 4 is confirmed.
The paper also tests whether adding these variables to the most commonly used explanatory variables will enhance the model fit. The BIC test shows that the model with life cycle stage has a higher value, equal to 158.909, which indicates that adding the firm’s life cycle stage will increase the model explanatory power.
5.2. Life cycle stage and credit rating
shows the results for the impact of a firm’s life cycle stage on the credit rating, the ordered Probit estimation control for year effects and industry effects. In Column 1 the independent variable is credit scoring, which takes the value from 8 down to 1 corresponding to the eight credit scores ranging from AAA through to CC. These results show that the introduction and decline stages of a firm’s life cycle have a significantly negative impact on the probability of the firm to have a high credit scoring and rating (P-value < 0.01), indicating that firms in the introduction and decline stages are more likely to have a low credit scoring. These firms are riskier and may not be able to fulfil their financial obligation, the firms in the introduction stage may face the problems encountered when people might not accept their product, and the firm is not able to generate the cash to service their debt, so their credit rating is expected to be low. This result does not contradict the results in Tables V or VI, where firms in the introduction phase have a positive relationship with positive change to rating and a negative relationship with downgrading. The results can be explained by the fact that the sample consists of listed companies with a credit rating because they have the ability to issue long-term debt, so they are large and well known, but some of them are still in the introduction phase. Thus, if the firm is in the introduction phase it will be more likely to have a low credit rating; at the same time, the credit rating agencies are more likely to enhance the credit rating for introduction phase firms if they want to upgrade the credit score of these firms.
Table 7. The impact of firm life cycle stage on credit rating.
Firms in the decline stage of their life also have an inverse impact on their rating scoring; since these firms are in the decline stage they are facing low demand for their product. Thus, the firms prepare themselves to exit the market or restructure their operations and produce new product or enter new markets the results are consistent with findings of Blomkvist et al. (Citation2021).
In order to address the potential endogeneity issue between the dependent variable and the independent variables, a robustness check is conducted in this study. Following the approach used by Attig et al. (Citation2013) and Bendig et al. (Citation2017), the lagged values of all independent variables are included in the analysis. This helps to mitigate any bias that may arise from endogeneity. The untabulated findings show that the results remain qualitatively similar to the main results, providing further support for the robustness of the findings. Furthermore, as an additional robustness check, we explore alternative measures of firm size. In this case, we use total revenue as a proxy for firm size. The results obtained using this alternative measure are also consistent with the main results. Additionally, we include short-term debt as a control variable to account for short-term solvency that may affect the credit rating. furthermore, to test the impact of cash flow volatility on credit rating, we add control for the standard deviation of cash flow from operations from year t-2 to year t. The results obtained with the inclusion of this variable are similar to the main results, the results are available upon request.
6. Conclusion
The study findings provide robust empirical evidence supporting the hypotheses that establish a significant relationship between the firm life cycle and credit scoring. These findings align with the dynamic resources-based view, where firm resources and capabilities evolve during a firm’s life, which in turn affects the firm’s competitiveness and its financial performance. The results indicate that introduction, growth, and mature life cycle stages have a positive relationship with credit rating upgrading. On other hand, firms in the decline stage have a negative relationship with credit rating upgrading. In addition, the results show that firms in the introduction, growth, and mature stage of their life cycle have a negative impact on the credit rating downgrading.
In general, it may be concluded from the above results that the decisions of credit rating agencies are influenced by the life stage of the firm. This is due to impact of the firm life cycle on the risk perceived by external analysts regarding the firm’s financial performance. This finding has important implications for the strategic decision-making of firms and their long-term financial stability. Therefore, it is recommended that firm management directs their efforts towards reaching and maintaining the maturity stage, as this increases the likelihood of obtaining a higher credit rating. The main limitation of this study lies in the use of publicly listed firms from a single country, as data for private firms were unavailable. To enhance the generalizability of the findings, future research can expand the scope by including firms from different countries with varying financial systems. This would provide a more comprehensive understanding of the relationship between firm life cycle and credit ratings across diverse contexts. Furthermore, the results can be enriched by incorporating mediating and moderating variables that may explain and influence the association between firm life cycle and credit ratings.
Acknowledgments
This research was conducted during my sabbatical year from Mutah University for the academic year 2019-2020, and I sincerely acknowledge their unwavering support.
Disclosure statement
No potential conflict of interest was reported by the author(s).
Additional information
Funding
Notes on contributors
Ala’a Adden Abuhommous
Dr. Ala’a Adden A. Abuhommous is a Finance PhD graduate from Brunel University, London. He currently serves as an Associate Professor at Mutah University, Jordan, with prior leadership roles at both Mutah University and Dar Al-Uloom University. Dr. Abuhommous specializes in capital structure theory, investment decisions, credit rating, and working capital management. He brings a wealth of experience from his time as a full-time Lecturer at London International College of Business and Technology at Brunel University and his four years in accounting and finance at Jordanian Electricity Power Co.
References
- Abuhommous, A. A. A., Alsaraireh, A. S., & Alqaralleh, H. (2022). The impact of working capital management on credit rating. Financial Innovation, 8(1), 1–18. https://doi.org/10.1186/s40854-022-00376-z
- Agarwal, R., & Audretsch, D. B. (2001). Does entry size matter? The impact of the life cycle and technology on firm survival. The Journal of Industrial Economics, 49(1), 21–43. https://doi.org/10.1111/1467-6451.00136
- Ahmed, B., Akbar, M., Sabahat, T., Ali, S., Hussain, A., Akbar, A., & Hongming, X. (2020). Does firm life cycle impact corporate investment efficiency? Sustainability, 13(1), 197. https://doi.org/10.3390/su13010197
- Akbar, M., Akbar, A., Maresova, P., Yang, M., & Arshad, H. M. (2020). Unraveling the bankruptcy risk‒return paradox across the corporate life cycle. Sustainability, 12(9), 3547. https://doi.org/10.3390/su12093547
- Akbar, M., Akbar, A., Qureshi, M. A., & Poulova, P. (2021). Sentiments–risk relationship across the corporate life cycle: Evidence from an Emerging market. Economies, 9(3), 111. https://doi.org/10.3390/economies9030111
- Akbar, M., Hussain, A., Sokolova, M., & Sabahat, T. (2022). Financial distress, firm life cycle, and corporate restructuring decisions: Evidence from Pakistan’s economy. Economies, 10(7), 175. https://doi.org/10.3390/economies10070175
- Al‐Hadi, A., Chatterjee, B., Yaftian, A., Taylor, G., & Monzur Hasan, M. (2019). Corporate social responsibility performance, financial distress and firm life cycle: Evidence from Australia. Accounting & Finance, 59(2), 961–989. https://doi.org/10.1111/acfi.12277
- AlHares, A., Ntim, C. G., & King, D. (2018). Block ownership and companies’ R&D intensity: The moderating effect of culture. Corporate Ownership & Control, 15(2), 19–32. https://doi.org/10.22495/cocv15i2art2
- Alissa, W., Bonsall, S. B., IV, Koharki, K., & Penn, M. W., Jr. (2013). Firms’ use of accounting discretion to influence their credit ratings. Journal of Accounting and Economics, 55(2–3), 129–147. https://doi.org/10.1016/j.jacceco.2013.01.001
- Amin, A., Bowler, B., Hasan, M. M., Lobo, G. L., & Tresl, J. (2023). Firm life cycle and cost of debt. Journal of Banking & Finance, 154, 106971. https://doi.org/10.1016/j.jbankfin.2023.106971
- Ashbaugh-Skaife, H., Collins, D. W., & LaFond, R. (2006). The effects of corporate governance on firms’ credit ratings. Journal of Accounting and Economics, 42(1–2), 203–243. https://doi.org/10.1016/j.jacceco.2006.02.003
- Attig, N., El Ghoul, S., Guedhami, O., & Suh, J. (2013). Corporate social responsibility and credit ratings. Journal of Business Ethics, 117(4), 679–694. https://doi.org/10.1007/s10551-013-1714-2
- Bendig, D., Strese, S., & Brettel, M. (2017). The link between operational leanness and credit ratings. Journal of Operations Management, 52(1), 46–55. https://doi.org/10.1016/j.jom.2016.11.001
- Bhandari, A., & Golden, J. (2021). CEO political preference and credit ratings. Journal of Corporate Finance, 68, 101909. https://doi.org/10.1016/j.jcorpfin.2021.101909
- Blomkvist, M., Anders, L., & Hitesh, V. (2021). Credit ratings and firm life-cycle. Finance Research Letters, 39, 101598. https://doi.org/10.1016/j.frl.2020.101598
- Boumparis, P., Milas, C., & Panagiotidis, T. (2019). Non-performing loans and sovereign credit ratings. International Review of Financial Analysis, 64, 301–314. https://doi.org/10.1016/j.irfa.2019.06.002
- DeAngelo, H., DeAngelo, L., & Stulz, R. M. (2006). Dividend policy and the earned/contributed capital mix: A test of the life-cycle theory. Journal of Financial Economics, 81(2), 227–254. https://doi.org/10.1016/j.jfineco.2005.07.005
- Dickinson, V. (2011). Cash flow patterns as a proxy for firm life cycle. The Accounting Review, 86(6), 1969–1994. https://doi.org/10.2308/accr-10130
- Dickinson, V., Kassa, H., & Schaberl, P. D. (2018). What information matters to investors at different stages of a firm’s life cycle? Advances in Accounting, 42, 22–33. https://doi.org/10.1016/j.adiac.2018.07.002
- Dorfleitner, G., & Grebler, J. (2020). The social and environmental drivers of corporate credit ratings: International evidence. Business Research, 13(3), 1343–1415. https://doi.org/10.1007/s40685-020-00127-9
- Fama, E. F., & French, K. R. (1997). Industry costs of equity. Journal of Financial Economics, 43(2), 153–193.
- Figlewski, S., Frydman, H., & Liang, W. (2012). Modeling the effect of macroeconomic factors on corporate default and credit rating transitions. International Review of Economics & Finance, 21(1), 87–105. https://doi.org/10.1016/j.iref.2011.05.004
- Gort, M., & Klepper, S. (1982). Time paths in the diffusion of product innovations. The Economic Journal, 92(367), 630–653. https://doi.org/10.2307/2232554
- Graham, J. R., & Harvey, C. R. (2001). The theory and practice of corporate finance: Evidence from the field. Journal of Financial Economics, 60(2–3), 187–243. https://doi.org/10.1016/S0304-405X(01)00044-7
- Habib, A., & Hasan, M. M. (2017). Firm life cycle, corporate risk‐taking and investor sentiment. Accounting & Finance, 57(2), 465–497. https://doi.org/10.1111/acfi.12141
- Habib, A., & Hasan, M. M. (2019). Corporate life cycle research in accounting, finance and corporate governance: A survey, and directions for future research. International Review of Financial Analysis, 61, 188–201. https://doi.org/10.1016/j.irfa.2018.12.004
- Hasan, M. M., & Cheung, A. (. K. (2018). Organization capital and firm life cycle. Journal of Corporate Finance, 48, 556–578. https://doi.org/10.1016/j.jcorpfin.2017.12.003
- Hasan, M. M., Cheung, A., Tunas, L., & Kot, H. W. (2021). Firm life cycle and trade credit. Financial Review, 56(4), 743–771. https://doi.org/10.1111/fire.12264
- Hasan, M. M., & Habib, A. (2017). Firm life cycle and idiosyncratic volatility. International Review of Financial Analysis, 50, 164–175.
- Hasan, M. M., & Habib, A. (2023). Corporate tax avoidance and trade credit. Accounting and Business Research, 1–30. https://doi.org/10.1080/00014788.2023.2196052
- Hasan, M. M., Hossain, M., Cheung, A., & Habib, A. (2015). Corporate life cycle and cost of equity capital. Journal of Contemporary Accounting & Economics, 11(1), 46–60. https://doi.org/10.1016/j.jcae.2014.12.002
- Hill, P., Korczak, A., & Wang, S. (2019). The use of earnings and operations management to avoid credit rating downgrades. Accounting and Business Research, 49(2), 147–180. https://doi.org/10.1080/00014788.2018.1479630
- Hussain, A., Akbar, M., Kaleem Khan, M., Akbar, A., Panait, M., & Catalin Voica, M. (2020). When does earnings management matter? Evidence across the corporate life cycle for non-financial Chinese listed companies. Journal of Risk and Financial Management, 13(12), 313. https://doi.org/10.3390/jrfm13120313
- Jenkins, D. S., Kane, G. D., & Velury, U. (2004). The impact of the corporate life‐cycle on the value‐relevance of disaggregated earnings components. Review of Accounting and Finance, 3(4), 5–20. https://doi.org/10.1108/eb043411
- Kiesel, F., & Lücke, F. (2019). ESG in credit ratings and the impact on financial markets. Financial Markets, Institutions and Instruments, 28(3), 263–290. https://doi.org/10.1111/fmii.12114
- Kim, Y. S., Kim, Y., & Song, K. R. (2013). Credit rating changes and earnings management. Asia‐Pacific Journal of Financial Studies, 42(1), 109–140. https://doi.org/10.1111/ajfs.12007
- Kisgen, D. J. (2006). Credit ratings and capital structure. The Journal of Finance, 61(3), 1035–1072. https://doi.org/10.1111/j.1540-6261.2006.00866.x
- Lin, C. M., Chen, C. C. S., Yang, S. Y., & Wang, W. R. (2020). ‘The effects of corporate governance on credit ratings: The Role of corporate social responsibility. Emerging Markets Finance and Trade, 56(5), 1093–1112. https://doi.org/10.1080/1540496X.2018.1512486
- Papadimitri, P., Pasiouras, F., Tasiou, M., & Ventouri, A. (2020). The effects of board of directors’ education on firms’ credit ratings. Journal of Business Research, 116, 294–313. https://doi.org/10.1016/j.jbusres.2020.04.059
- Wahlen, J. M., Baginski, S. P., & Bradshaw, M. T. (2018). Financial Reporting, financial statement analysis, and Valuation: A strategic Perspective (9th ed.). South-Eastern Cengage Learning.
- Wang, Z., Akbar, M., & Akbar, A. (2020). The interplay between working capital management and a firm’s financial performance across the corporate life cycle. Sustainability, 12(4), 1661. https://doi.org/10.3390/su12041661