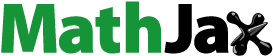
ABSTRACT
This study presents a meta-regression analysis on the literature of courts efficiency. The metadata set comprises 264 efficiency scores retrieved from 36 papers published from 1992 to 2019. Our models explain a substantial proportion of both within- and between-study heterogeneity. Estimates indicate that the efficiency score of primary papers decreases when the sample size and the sum of inputs and outputs increase. It is also found that parametric papers yield higher efficiency scores than non-parametric studies. Furthermore, the scores obtained in studies that analyse first-instance courts are significantly higher than those from studies on appeal courts. Finally, papers focusing on specific courts (tax, civil or criminal) yield efficiency scores that are higher than those obtained in studies where the analysed courts are mixed.
1. Introduction
This paper analyses the literature focusing on judicial efficiency through a meta-regression analysis (MRA). The aim of the study is twofold: on the one hand, we detect and measure the differences in the efficiency scores obtained when collecting data from primary papers that estimate a frontier for courts; on the other hand, we refer to the recent literature on MRA to explain the heterogeneity of the efficiency scores with respect to the methodological choices made in prior studies and some sector-specific variables.
This study has three motivations. First, the judicial system is a sector producing a specific service – justice - and, accordingly, it can be studied by using the customary tools of production and efficiency theory (Falavigna et al., Citation2018; Marciano et al., Citation2019; Voigt, Citation2016). Since the seminal work by Farrell (Citation1957), it has been clearly explained whether a decision unit is efficient or not. However, from an empirical perspective there is no universally agreed-upon consensus regarding a preferred frontier model, as the parametric and nonparametric approaches have own advantages and limitations. The choice between them typically hinges on factors such as data characteristics, assumptions, and the research question at hand (Berger & Humphrey, Citation1997; Coelli & Perelman, Citation1999; Fethi & Pasiouras, Citation2010). After collecting data and highlighting the heterogeneity in courts efficiency, this meta-study serves the purpose of assessing if and to what extent the methods used by primary scholars influence the estimates of efficiency of judicial systems. This is a line of research widely explored in the scientific community of efficiency analysts, with the result that a number of meta-reviews have been recently performed by focusing on specific sectors (i.e., Aiello & Bonanno, Citation2018, Citation2019; Bravo-Ureta et al., Citation2007; Brons et al., Citation2005; Chaffai, Citation2022; Fall et al., Citation2018; Ogundari, Citation2014; Trong Ho et al., Citation2022; Xu et al., Citation2023).
Second, at a time of restrictive fiscal policies, the need for evidence-based policy decisions drives the demand for efficiency analyses on how public sectors work. In our context, citizens expect efficiency from the judicial systems and, thus, investigating whether a sample of courts acts in a technically efficient way becomes an issue of great interest not only for academics, but also for practitioners and policy-makers. In this respect, the relatively high amount of government expenditure on justice, in conjunction with the time which courts need for issuing judgements in cases, is part of a long-dated debate, in which criticism related to the functioning of the justice system has been raised for years (Major, Citation2015). All this highlights the need to apply suitable methods to assess courts’ efficiency, thereby helping public management in improving the offer of judicial services.
Third, the institutional architecture of many countries has changed rapidly since the 1990s due to extensive deregulation aimed at optimizing the use of public resources in offering services of general interest at the local level. Institutional reforms spur the interest of economists and public administration to evaluate the efficiency level and the key-factors influencing the performance of the public sector (European Commission, Citation2017; Lovell, Citation2002). Importantly, the institutional framework on how courts work differs country-by-country and, therefore, it is reasonable to expect that the heterogeneity in national norms translates into heterogeneity in judicial efficiency.
As in other sectors, the empirical literature on judicial efficiency documents that the results are sensitive to study design, model specifications and to sectoral specific variables (Marciano et al., Citation2019; Voigt, Citation2016). Marciano et al. (Citation2019) address an intriguing issue in this field of research in regard to the difference between efficiency and efficacy of judicial institutions. These scholars propose theoretical and empirical evidence supporting the necessity of a clear distinction between the two dimensions of courts. The qualitative survey provided by Voigt (Citation2016) is closer to the specific scope of our paper. While Voigt (Citation2016) offers valuable arguments in terms of why efficiency differs when focusing on courts’ delays, our study enriches this debate because it detects heterogeneity in efficiency scores and provides a quantitative evaluation on the variability of courts’ efficiency determined by the study design of primary-papers and the courts specific variables used in the primary papers. This is the novelty of this study, and at this end an MRA is performed.
MRA is a method used to collect and integrate the results from individual papers. It allows evaluating whether, and to what extent, the features of each work (i.e., estimation method, year of publication, functional form, court-type, sample size, and dimension) affect the findings when performing an efficiency study (Stanley, Citation2001). As Glass (Citation1976) says, MRA “connotes a rigorous alternative to the casual, narrative discussions of research studies which typify our attempt to make sense of the rapidly expanding research literature” (Glass, Citation1976, p. 3).
Given that the literature on judicial efficiency lends itself well to being summarized through an MRA, it is noteworthy that no exhaustive work has explored the “court efficiency-study design” nexus. In attempting to fill this gap, we consider all the judicial studies published from 1992 to 2019 (which were available in August 2021). The final meta dataset comprises 264 observations from 36 papers. It comes from different sources with no restriction on the countries included in the original studies, thereby assuring full coverage of the literature based on studies that estimate a frontier for judicial courts.
When specifying our meta regressions we follow two recent papers (Aiello & Bonanno, Citation2018, Citation2019) which – in explaining the variability of efficiency in banking and municipalities – firstly estimate a random effects model and then consider a Weighted Least Squares (WLS), as suggested by Gallet and Doucouliagos (Citation2014).
Owing to its main research focus, i.e., measuring the impact of potential sources of heterogeneity on judicial efficiency, this article contributes to the debate assessing the role of methodological choices made by researchers when specifying the frontier of courts. Interesting results come from the role played by courts specialization and the judgment level. Therefore, the paper’s contribution lies in the fact that it addresses a number of issues, such as: whether the efficiency scores retrieved from non-parametric papers differ from those estimated by parametric papers; whether the number of inputs and the number of outputs affect the results; whether the sample size of courts covered by primary papers matters in influencing the results; and whether papers focusing on generic courts provide different efficiency scores than papers focusing on specialised courts. All this also helps to identify better the variability in judicial efficiency scores and thus addresses the so-called “apples and oranges” issue due to the fact that the metadata set comes from studies which are different from one another (Glass et al., Citation1981).
The paper is organised as follows: Section 2 presents the method used to create the meta-dataset; Section 3 briefly describes the distribution of court efficiency scores; Section 4 presents the meta-regression and describes the variables used in the analysis; Section 5 discusses the results; finally, Section 6 concludes.
2. Construction of the meta data-set on court efficiency scores
The number of papers analysing the efficiency of courts is very limited and, thus, this study covers all the related literature. In order to attain the full coverage of prior research, a search based on three different phases was performed.
Firstly, the main and most consulted scientific paper repositories were queried, and the research results for “judicial efficiency” and “court efficiency” were analysed. For the first term, 106,000 results were obtained in Google, and 12,100 in Google Scholar, while in the scientific databases the number was significantly lower: 120 in SSRN, 48 in Science Direct and 74 in WoS. With regard to the second search item, “court efficiency”, the results were 28,900 in Google, 2,270 in Google Scholar, 248 in Science Direct and 16 in WoS.
Secondly, the search was refined searching for “judicial”, “efficiency” and “frontier” in the titles, abstracts and keywords. The main journals on the fields were manually consulted, and papers were further selected with a focus on efficiency. The references from the qualitative surveys made by Marciano et al. (Citation2019) and Voigt (Citation2016) have been subsequently scanned. Before filtering this sample of works, we ensured that they (a) focused on the efficiency of the judicial system; (b) estimated a frontier using parametric or non-parametric methods (c) reported the results required to run a meta-regression (that is the average efficiency score and its standard deviation); (d) were published in English in a journal or as a discussion paper. This process resulted in the selection of 44 papers and was concluded on 31 August 2021.
Finally, the authors of the selected papers were contacted for further information or clarifications, and eight papers were removed following this interaction with the authors. This decision was due to the fact that the authors of eight studies do not provide the essential data required for conducting the meta-analysis (i.e., the efficiency scores and the standard deviation). Conversely, other scholars have contributed supplementary information that was not documented in the published manuscripts. The search then yields a sample of 36 papers published from 1992 to 2019 ().Footnote1 Since from each paper more than one point-estimation was extracted, the final comprises 264 observations.Footnote2
3. The heterogeneity in the literature on courts’ efficiency
presents some descriptive statistics of the collected data by grouping efficiency scores according to (a) the approach used in the estimations (parametric or non-parametric), (b) the structure of the data (panel or cross-sectional), (c) the hypotheses regarding returns to scale (constant or variable) in non-parametric studies, (d) the judicial degree (first instance or others), (e) the type of court (if specialised on a specific matter or generalist)Footnote3
Table 1. Average, standard deviation and number of observations of efficiency scores, by group.
Overall, the sample of 264 observations yields an (unweighted) average technical efficiency of 0.752.Footnote4 It also emerges that the difference in mean (0.154 = 0.885–0.731) is statistically significant when comparing the 37 observations from parametric studies and the 227 observations from non-parametric papers. With regard to the data used in primary studies, 60% of the observations come from papers using cross-sectional data and 40% from panel data studies.
It is found that the efficiency score is 0.783 for the studies that use panel data, while is 0.732 for cross-sectional studies. A similar difference in mean is found between the efficiency reported in published papers and that of unpublished works (0.774–0.643 = 0.131). When considering the sub-sample of non-parametric frontiers, we find that papers assuming variable returns to scale (VRS) register a mean efficiency of 0.757, which is higher than 0.699 that is observed for papers working under the hypothesis of constant returns to scale (CRS). Moreover, the difference between the average efficiency scores for estimations referring to European countries and those for estimations related to non-European countries is statistically significant (0.780–0.697 = 0.083).
As regards the structure of the degree of judgement, it should be noted that 225 out of 264 observations refer to the judgement of “first instance”. The test to determine whether the difference in means group indicates that the papers on first-instance courts yields efficiency scores that do not differ from those obtained in papers focusing on appeal courts. Finally, there is a significant difference in average efficiency if we consider specialised courts in a specific subject (0.769) or generalist courts (0.726).
If on one hand, the mean of court efficiency differs by group (), it is interesting to consider the entire efficiency distribution. shows not only that there is much variability group-by-group, but also that the efficiency score distributions have different shapes and forms. All this indicates that any choice made by researchers in performing a court efficiency study significantly affects final estimations.
From this discussion, it emerges that the study design of primary papers plays an important role in determining differences, not only in the means but also in the distributions of judicial efficiency scores.
4. Meta-regressions for judicial efficiency literature
The literature on efficiency indicates that the results are highly heterogeneous across studies, whatever the sector.Footnote5 As we have shown in Section 3, this holds true for judicial literature, thereby motivating a meta-study aimed to detect the factors that explain such a variability. To this scope, let’s consider the following model:
where the dependent variable Ei is the i-th technical efficiency from each primary paper.Footnote6 The matrix Xij comprises the explanatory variables that summarise various model characteristics of every study. The error component εi is heteroskedastic because each estimated efficiency scores Ei retrieved by the primary papers has its own measure of variability, leading to the heteroskedasticity of the distribution of the error term εi. To address this issue, the weight we use is the standard deviation (S) of each observation of our meta-study. Thus, the estimated meta-regression is:
where the disturbance is corrected for heteroskedasticity.
The method used in estimating Equationeq. [3][3]
[3] can be a fixed effects or random-effects model. These methods differ in terms of treatment of heterogeneity. In detail, a fixed effects meta-regression assumes that all the heterogeneity is explained by the covariates and leads to excessive type I errors when there is residual, or unexplained, heterogeneity (Harbord & Higgins, Citation2008). On the contrary, a random-effects meta-regression allows for such residual heterogeneity (the between-study variance not explained by the covariates), thereby extending the fixed effects model. Formally, under the random-effects framework, Equationeq. [3]
[3]
[3] becomes:
where ei ~ N(0 , σ2i) is the within-study disturbance and ui ~ N(0 , τ 2) is the deviation due to the residual non-observable heterogeneity (between-study variance). Parameter τ2 is a measure of between-study variability and is estimated as in Harbord and Higgins (Citation2008).Footnote7
We adopt a two-step procedure as proposed in Gallet and Doucouliagos (Citation2014) and applied in Aiello and Bonanno (Citation2018, Citation2019). A Random Effect Maximum Likelihood (REML) regression is run in the first step, and, in the second step, a WLS regression is employed in which the weights also include the value of τ2 retrieved from the first step. This ensures that the estimates will be robust to clustering at the study level.
Before focusing on the empirical setting and on the results, here it is worth pointing out how Equationeq. [4][4]
[4] helps in addressing the “apples and oranges” issue which is suspected to hold when comparing results from different studies. In the efficiency literature the comparability problem has been introduced by Brons et al. (Citation2005), according to which performing an MRA on efficiency scores “means comparing values that consist of a heterogeneity component and an efficiency component” (p. 7). In the following, we take advantage of the arguments proposed by Brons et al. (Citation2005) and assume that there is a theoretical frontier C with a universal value. From , it emerges that in A the efficiency is, on average, higher than in B, but the real efficiency in sample B is higher than in sample A. Hence, taking the mean value of the estimated efficiency, the meta-study does not compare the single point-observations but compares the sample heterogeneity (heterogeneity component). Again, if the specific frontiers are the highest attainable efficiency levels given the study-specific conditions, then the exercise to compare the mean efficiency scores will be a mode to compare the actual efficiency scores (efficiency component) (Brons et al., Citation2005).
Figure 3. The comparison of mean efficiency from different studies.

Our MRA takes into account these two components because, on the one hand, the study-specific heterogeneity arising from differences in sample composition can be handled by applying an unobserved effects framework (Brons et al., Citation2005; Merkel & Holmgren, Citation2017). In particular, study-specific heterogeneity is treated with individual intercept terms to cope with differences in sample composition (the ui term in our Equationequation [4][4]
[4] ). On the other hand, when considering the efficiency component, it is known that in the meta-regression context, the aim is to explain the variation in efficiency scores by using the variation in a number of moderator variables. Therefore, the feasible way to deal with the problem of study comparability is to specify an econometric model in such a way that the within-study variation is used to explain the variation in the efficiency estimations (Brons et al., Citation2005). In other words, the differences in the efficiency of primary papers (efficiency component) are handled by including the moderator variables in the analysis (Merkel & Holmgren, Citation2017).
4.1. The explanatory variables in the meta-regression analysis
The explanatory variables that enter the regression are identified by following the meta-studies that review the efficiency literature in specific sectors. These variables can be classified into three groups, which are discussed in the following.
The variables included in the first group are retrieved from prior literature on meta-regression analysis which are similar to this study with the scope to meta-review the efficiency scores estimated for a specific sector. Differently phrased, this group of variables overlaps those employed in the related MRA on efficiency studies (cf. footnote 5). When the interest is in the approaches and methods used to estimate the frontier, a first control comes from distinguishing the primary papers applying a parametric approach and the non-parametric studies. To this end, the dummy variable used is Parametric, which is equal to unity for studies using parametric methods and 0 for the others. The dummy variable Panel is equal to unity if the original works used panel data, and 0 otherwise. Including the variable Year of publication of the primary paper provides information on how the estimated efficiency scores vary over time. The variable Published is a dummy equal to 1 if the paper is published in a peer-review journal, and 0 otherwise. Furthermore, the variable Dimension is the sum (in logs) of inputsFootnote8 and outputsFootnote9 used in the primary efficiency studies, while the variable Sample Size (in logs) is the number of observations, that is, the number of courts analysed in each primary paper.Footnote10
The second group of regressors takes into consideration some specific factors related to the judicial literature. To this end, we employ two dummies. The first is the dummy Specialised courts, which is equal to 1 if the primary paper focuses on a specific type of court (commercial, tax, civil, criminal) and 0 if estimations refer to more than one type of court or to a national judicial system as a whole.Footnote11 The second dummy is First instance, which is equal to 1 if the primary paper investigates the efficiency of courts of first instance and 0 when the efficiency is done for appeal courts.
Finally, the third group of regressors is to control for potential country-specific effects and includes two observables. They are the GDP per capita and the indicator Legal enforcement of contracts. The GDP per capita refers to the country analysed in the primary paper and is from the World Bank database (data are in PPP-constant 2011 dollars). The variable Legal enforcement of contracts, is used as a proxy for the quality of the legal system of each country, and it is from the Fraser Institute reports. It is measured through a scale – ranging from 0 to 10 - for which higher values indicate better performance. Finally, the dummy Europe - which is equal to 1 if the study used data from a European country (the reference group comprises efficiency scores from papers focusing on the rest of the world) serves also to control for unobservable territorial fixed-effects. in Appendix reports the correlation matrix for the variables included in our regressions.
5. Estimated models
In presenting the results, we depart from that reports the estimations of models including the variables related to the study design. We show different specifications gradually adding the regressors retrieved from (i) the literature of meta-regression on efficiency scores and (ii) two sector peculiar factors. displays the estimates obtained when using two country observables and considering two additional regressions to check the robustness of results.
Table 2. Meta-regression analysis of judicial efficiency scores. The role of the study design and of sector-specific explanatory variables1.
Table 3. Meta-regression analysis of judicial efficiency score the role of country observables and a sensitivity analysis1.
As presented in , the proportion of the residual variance due to between-study heterogeneity is very high: in the most parsimonious Model 1, it is 74.04%, while the proportion of between-variance explained by the covariates is about 19% (the within-study sampling variability). As expected, when we include additional regressors, the between-study heterogeneity tends to decrease (in Model 6 of it is equal to 48.39% and becomes 12.23% in Model 1 of ), while the proportion of between-variance explained by the covariates tends to increase: indeed the adjusted R-squared ranges from 18.62% of Model 1 of to more than 98% of the full model specifications in . It is worth pointing out that the residual variance due to the between-study heterogeneity falls to about 12% of Model 1 of , thereby signalling that this MRA analysis explains a very high proportion of variability of courts’ efficiency. Finally, the statistic F-Fisher indicates that the joint significance of the explanatory variables is always high in each regression.
To ensure clarity in the exposition, the discussion of results is divided into three sub-sections. While sub-section 5.1 is devoted to the baseline regressions including the study-design related variables, sub-section 5.2 focuses on specific factors for judicial efficiency. In sub-section 5.3 we discuss the effects exerted by the country observables and present the results from two robustness checks.
5.1. Estimation methods and other econometric issues
The estimated coefficient associated with Year of publication is always negative and significant (with the exception of Model 5 of ). A similar outcome is found for the efficiency of municipalities by Aiello and Bonanno (Citation2019). In our case, the result means that as the year of publication increases (i.e., more recent studies), the efficiency of courts found in primary papers tends to decrease.Footnote12 Furthermore, the estimates referring to published primary papers yield, on average, higher efficiency scores compared to estimations retrieved from working papers (indeed, coefficient
is always positive and significant).
Our regressions also indicate that the method used to model court frontiers matters: coefficient associated with the dummy Parametric is significant and positive in all specifications we estimate. In other words, parametric studies achieve a higher efficiency level than non-parametric estimations: on average, the differential impact of the parametric method ranges from 0.1344 in column (2) to 0.1533 in column (4). The conclusion from this meta-study is that the methodological approach to estimate the frontiers is a significant source of heterogeneity in courts’ efficiency. This evidence on how the methods are impacting in explaining the variability in results is in line with other meta-studies, although the sign of the impact differs sector-by-sector. For instance, Nguyen and Coelli (Citation2009) find a significant and negative coefficient for parametric studies in health care. Conversely, the effect is positive in Bravo-Ureta et al. (Citation2007) for the agricultural efficiency in developed and developing economies, Odeck and Bråthen (Citation2012) for efficiency in seaports, Aiello and Bonanno (Citation2018) for banking and Aiello and Bonanno (Citation2019) for local government. It is also worth pointing out that the parametric effect is neutral in Thiam et al. (Citation2001) for agriculture in developing countries, Nguyen and Coelli (Citation2009) for hospitals, Brons et al. (Citation2005) for transport and in Ogundari and Brümmer (Citation2011) for Nigerian agriculture.
We do not find conclusive results about the data type: the coefficient associated with the dummy Panel is not significant in , while it is significantly negative in some models of . Finally, there is no difference between the efficiency scores when distinguishing by geographical area (in , the coefficient of Europe,
is always not significant).
As far as the role of Dimension is concerned, it emerges that is negative, thereby indicating that an increase of inputs and outputs used in estimating the frontier is associated with a decrease, on average, of court efficiency. This result on judicial efficiency contrasts with Aiello and Bonanno (Citation2018, Citation2019), Nguyen and Coelli (Citation2009), Ogundari and Brümmer (Citation2011) and Thiam et al. (Citation2001), who all found a positive impact of Dimension on the efficiency of the sector they meta-review. There are several reasons for the unexpected sign of our estimate. While an important role might be played by the nature of the production function of the courts, further factors that affect the results are the type of inputs and outputs and the model orientation used in DEA papers (Banker et al., Citation1984; Cook et al., Citation2014; Kao & Hung, Citation2008; Park & Zhu, Citation2011; Seiford & Thrall, Citation1990). While we will further explore the impact of Dimension in a robustness check (cf. Section 5.3), here it is fruitful calculate the marginal effect on mean efficiency at different values of Dimension. Considering the use of logs, when Dimension is equal to the overall mean of the sample (1.680), the marginal effect is −0.0193 (given that
in Model 3 of , the marginal effect is measured as the ratio between −0.0325 and 1.680). Again, when the number of inputs and outputs is 10, the marginal effect of Dimension on the variability of courts’ efficiency is approximately 0 (, Panel a).
The estimated coefficient of the relationship between courts’ efficiency and the number of observations provides further interesting findings. Size can exert a non-linear effect and, thus, it enters into regressions in logs. The estimated coefficient signals that the link between Sample Size and the efficiency scores of primary papers is negative. However, the magnitude of the marginal effect is very small, being −0.001 when the Sample Size is 40 and quickly tends to zero if courts are about 100 (, Panel b).Footnote13
Estimates refer Equationeq. 4[4]
[4] with the standard deviation of each point-observation, that is the efficiency score, used as weights to control for heteroskedasticity
5.2. The role of specific factors in the judicial literature
Columns 4–6 of display the results for specifications with the two sector-specific variables First instance and Specialised courts used in the analysis. Importantly, augmenting the meta-regressions with court-specific factors does not have an impact on the estimates of the explanatory variables so far discussed.
We firstly introduce one by one the variables First instance and Specialised courts, and then they are in the full Model 6 of . The evidence is robust and indicates a positive impact for both First instance and Specialised courts.
We find that papers focusing on courts in the first instance provide higher efficiency scores than papers analysing the efficiency of appeal courts. This is probably due to the incentive for this type of tribunal to be more efficient because it has to treat a massive number of disputes promptly. Another reason might be attributed to the fact that, in the common law system (cf. § 5.3), the court of appeal serves as the court of last resort, whereas in other systems, the court retains its role as a body that also adjudicates on the merits. Considering that the judicial cases on appeal are generally complex (for reasons related to the merit of the dispute or for reasons purely related to the judgment of the previous instance), it is possible that more time is needed to reach a sentence. This could explain a lower efficiency, in terms of resolved cases, in the case of appellate courts.
Furthermore, the primary studies on specialised courts yield a higher level of efficiency than the papers referring to more than one type of court or to a national judicial system as a whole. These results might depend on the homogeneity of the sample of courts used in estimating the frontier: it is expected that the efficiency scores from homogenous courts are higher than those obtained when considering heterogeneous samples (i.e., data of courts dealing with different judicial topics and different judgement levels). At some extent, we find that similar courts perform similarly with the result that they are more clustered around a frontier than different courts with divergent aims. This might be driven by the fact that for specialized courts the possibility of standardization of decisions and economy of procedures grows with respect to generalist courts.Footnote14 Expressed differently, specialised courts can develop high-level expertise in a particular and complex field of law and enhance the uniformity of decisions in that area, thereby achieving a high level of efficiency. Finally, courts specialisation can lead to a shorter resolution time with respect to general courts, so that the number of pending cases would be reduced.
5.3. The impact of country observables and a sensitivity analysis
Additional considerations come from and regard the results obtained when including in the MRA two country observables, that is the GDP per capita and the Legal enforcement of contracts.
As far as GDP per capita is concerned, the expected results are uncertain. Indeed, courts’ efficiency would be high in developed countries, as higher-income residents pay greater taxes and have more requirements on services and facilities. On the other hand, in high-income countries, courts are expected to manage higher financial resources, thereby implying that the efforts to be efficient might be reduced. The positive and significant coefficient (around 0.04 in Model 1 of ) indicates that the efficiency scores of courts in rich countries are, on average, higher than the studies focusing on less rich countries. This is in line with the argument that people living in developed nations expect to receive good services from public institutions because they pay more taxes.
From it also emerges that the estimated coefficient of Legal enforcement of contracts is positive (0.0093 in Model 2; 0.01 in Models 3 and 4) and highly significant, confirming the role of the institutional context in explaining how the courts effectively work when offering judicial services. The estimates suggest that primary papers focusing on countries with high-quality legal systems yield, on average, higher efficiency scores than studies focusing on countries with weak legal systems. Differently phrased, in countries with high levels of enforcement of contracts the disputes are easy to be fulfilled and, thus, courts work in a timely and efficient manner. The opposite holds in countries with low enforcement of contracts. In such a case, disputes are complex and difficult to fulfil, thereby having an impact on courts’ efficiency. In a nutshell, institutions differ country-by-country and thus it is expected that these differences translate into heterogeneity in efficiency scores of courts when performing a frontier analysis.
Finally, displays the estimates when performing two robustness checks, related to the variables Europe and Dimension.
In the Model 3 of the variable Europe is replaced with the binary variable Common Law, which is equal to 1 if a country uses a common law system and 0 otherwise. The evidence is twofold. On the one hand, it emerges that previous results are robust: the sign and the significance of the coefficients do no vary passing from Model 2 to Model 3. On the other hand, the coefficient of Common Law is negative, albeit not highly significant. The conclusion is that in our sample there is no relationship between efficiency of courts and the system of common law, despite it is less prescriptive than a system of civil law.
All previous considerations refer to meta-regression results indicating that the sum of the number of inputs and outputs originally used by primary scholars is negatively correlated with the court efficiency scores (for instance, in model 2 of coefficient β7 is −0.0509). As this is a different result compared to many meta-studies on efficiency (i.e, Aiello & Bonanno, Citation2016, Bravo-Ureta et al. Citation2007; Fall et al., Citation2018; Iliyasu et al., Citation2014; Nguyen & Coelli, Citation2009; Ramello & Voigt, Citation2012; Thiam et al., Citation2001), in Model 4 of we performed an additional test by splitting the variable Dimension in the number of Inputs and the number of Outputs. The expected results are mixed because the net outcome is determined by many factors, some of which are the type of inputs and outputs, the specific characteristics of the courts and last but not least, the complexity of prior analysis, as increasing inputs and outputs involves a larger number of dimensions and may require more computational resources (Banker et al., Citation1984; Cook et al., Citation2014; Kao & Hung, Citation2008; Park & Zhu, Citation2011; Seiford & Thrall, Citation1990). The estimates obtained when separating inputs and outputs indicate that the efficiency scores from primary papers with a large number of inputs are, on average, higher than papers with fewer inputs (the estimated coefficient β7IN is + 0.0448). The opposite holds for the number of ouputs (the estimated coefficient β7OUT is −0.0623). In the following we discuss these findings.
As argued in the seminal paper by Banker et al. (Citation1984) our positive effect associated with the number of inputs could be the net outcome of different forces. On the one hand, the addition of relevant inputs to the frontier analysis can improve the accuracy and completeness of the model, which may lead to better identify the efficient courts and, accordingly, obtain more accurate efficiency scores. In some cases, additional inputs may allow a court to offer the same level of services with fewer resources, resulting in higher efficiency scores. This can be particularly true if the additional inputs are important and complementary factors that were previously overlooked in the analysis. According to our results, it emerges that this positive effect is sizable. On the other hand, the addition of inputs can have a negative effect on efficiency scores. If the added inputs are not relevant to the production process or are redundant with other inputs, they may not contribute to the output in a meaningful way and can even increase the amount of noise in the analysis. In these cases, the inclusion of additional inputs can lead to lower efficiency scores. On the issues related to the choice of increasing the number of inputs when estimating a frontier, see also Kao and Hung (Citation2008).
In order to interpret the negative sign of β7OUT it is worth considering the relationship between the outputs. If the new judicial services are complementary to the existing ones, an increase in court efficiency is expected as, other things being fixed, the inputs are used more effectively in producing a greater range of outputs. However, if the new services are substitutes for the ones previously included in the frontier, the picture changes. Indeed, the efficiency score can decrease: courts use resources less efficiently to offer a given set of outputs as the new services replace the pre-existing ones. In our setting, it appears that the substitutability effect prevails over the complementarity effect (Park & Zhu, Citation2011; Seiford & Thrall, Citation1990).
6. Conclusions and future work
Improving our understanding of how prior research evaluates the efficiency of courts is an important issue in economics. In fact, a justice system that effectively interprets and applies the law fairly and impartially is fundamental to safeguarding citizens’ rights and promoting a well-functioning economy (European Commission, Citation2017). Along this line of reasoning a well-functioning and efficient judicial system is crucial for government promises regarding the enforcement of private property rights to be considered credible by potential investors. This credibility, in turn, has significant implications for national economic growth (Voigt, Citation2016; Ramello and Voigt Citation2012).
Referring to 264 observations of courts efficiency collected from 36 primary studies published from 1992 to 2019, a meta-analysis is carried out to explain the heterogeneity in efficiency. Compared to other qualitative surveys (Marciano et al., Citation2019; Voigt, Citation2016), we contribute to the debate by identifying the direction and quantifying the size of the effects that methodological choices exert on the mean efficiency scores of courts. Differently said, our scope is to explain the variation in courts efficiency across primary papers by identifying the sources of heterogeneity and assessing their effect on the observed variation in efficiency scores. We find not only that much of the variability of the judicial efficiency scores is due to the study design of each primary paper, but also that the court types that are scrutinized by prior research and the country characteristics help in explaining why the judicial literature yield a remarkable heterogeneity in the efficiency scores.
The results of this meta-study are threefold.
First, the descriptive section of the paper highlights the significant heterogeneity in court efficiency. For instance, panel data studies show higher unweighted mean efficiency scores compared to cross-sectional studies. Similarly, published papers tend to report higher scores than unpublished works, and the same pattern is observed for parametric versus nonparametric studies. Based on the related literature, it becomes evident that first-instance courts exhibit similar average efficiency scores to appeal courts, while specialized courts tend to have higher efficiency scores than generalist courts. Besides differences in means, our metadata also emphasises the existence of substantial differences in the form and shape of courts efficiency distribution. Here, it is important to point out that the meta-regression specification used in the paper fits well the data, as the residual variation that is attributable to between-study heterogeneity remains low, while the proportion of between-study variance explained by the covariates is high.
Second, the meta-regression analysis provides solid evidence that some methodological choices can significantly affect the variability of courts efficiency provided by prior research. In this regards, a factor driving heterogeneity is the approach used to estimate the frontier. For instance, it is found that primary papers using parametric methods yield significant higher efficiency scores than non-parametric studies. There is also significant evidence to support the hypothesis that efficiency scores decrease as the number of observations increases. Because of non-linearity, the effect of size is more pronounced when the sample size is small and its marginal effect quickly tends to zero when sample size in primary papers comprises about 100 courts. Similarly, courts’ efficiency scores fall as the number of inputs and outputs increases. In this case, the marginal effect of dimension is very small (although it remains negative) when the sum of inputs and outputs is about 10. In this respect, additional results show that that the overall effect of Dimension is positively driven from the number of inputs and negatively from the number of outputs. Furthermore, meta-regressions suggest the efficiency estimates from published primary papers are higher than those reported in working papers. The effects of other modelling choices, such as the assumption on the return to scale, are not found to be statistically significant at any level; moreover, the effect of data (panel vs cross-section) is not always robust but depends on the specifications of the meta-regression.
Third, heterogeneity in the results of primary papers is also explained by the types of court that researchers include in their efficiency study. This meta-analysis suggests that efficiency scores from specialised papers, that is, papers that focus on one court type (commercial, tax, administrative, criminal), are significantly higher than results from unspecialised papers. Similar evidence emerges when considering the level of judgement: the efficiency of papers focusing on first-instance courts is statistically higher than that estimated by papers analysing appeal courts or mixed samples (first-instance and appeal courts). This might be driven by the fact that the efficiency analyses focusing on a specific sample of courts (first-instance or sector-specialised courts) benefit from data homogeneity compared to primary samples of mixed courts. With homogenous data, it is expected that the clustering around the frontier of courts pursuing the same scope is pronounced, thereby translating into high-efficiency estimates.
While the key results of this meta-study are robust to different samples of observations, the analysis is subject to limitations related to data quality. In other words, several areas require further exploration, prioritizing research on exploring accurately the sources of heterogeneity in court efficiency. This emphasis is important as many papers are policy-oriented. Consequently, any effort to grasp better the heterogeneity of efficiency scores and accurately identify the best practices will assist policymakers in implementing effective responses to enhance courts efficiency (Silva, Citation2018).
The first limitation is due to the circumstance that scholars do not report details of the empirical setting. This hidden information in primary papers impedes replicability. Therefore, providing full explanations would be a good practice not only to let readers be informed on each study, but also to understand better the role of crucial choices that researchers made when estimating a frontier. For instance, it would be valuable for practitioners and researchers to know whether heterogeneity in judicial efficiency might be explained by orientation in technology (input- vs output-oriented models). This recommendation holds though in the public sector context the default assumption is to use an input-oriented technology. Indeed, a clear indication of this choice in the study design of primary paper increases the study replicability, thereby enriching its reliability. Similarly, the meta-dataset signals that there has been a predominance of non-parametric techniques (DEA), whereas the parametric approach is disregarded by scholars. As we find that parametric studies yield on average higher efficiency scores than non-parametric papers, the choice of massively using DEA in the judicial literature is not neutral. Indeed, it could lead to a risky conclusion, that is, courts being perceived as less efficient than they effectively are. This risk should be limited by encouraging more studies of courts through parametric frontiers, with the result of balancing the efficiency scores from the two approaches. Another aspect of model specification that would be better to analyse is the effect of country-level characteristics. In this, future depth analyses of reforms of national judicial systems might be highly informative for evaluating whether and to what extent the estimations of efficiency in primary works are related to changes in court systems, legal reforms or other contextual variables. Within this area of research, the assessment of the time effect on court efficiency determined by new institutional regimes compared to pre-existing ones is left for future research agendas.
Furthermore, taking advantage of a novelty of this paper, a future meta-study could dig deeply into understanding whether heterogeneity in estimations reflects differences in court-type (i.e., civil, commercial, tax, criminal). If one had a large metadata set by court type, results would be useful to understand whether estimates of primary papers focusing on a court-group differ from others, given the efficiency profile retrieved from estimating a production frontier. A final need regards the geographical coverage of efficiency studies. The literature counts only 36 studies. This does not reflect the relevance of the judicial efficiency throughout the world. Therefore, a recommendation for next research is to focus more on the courts efficiency of the countries that have so far disregarded (i.e., Japan, the US, Germany, the UK).
While these issues are left for future work, it is evident that addressing them can extend this research and lead to a better understanding of the sources of heterogeneity in court efficiency. This, in turn, can assist practitioners and policymakers in implementing effective measures in favor of sectoral efficiency.
Acknowledgments
The authors acknowledge Antonio Álvarez Pinilla, Giovanni D’Orio, Filippo Domma, Sergio Destefanis, Paolo Piselli, Angelo Zago, and two anonymous referees for helpful comments on earlier versions of the paper. They also benefited from discussions with participants at the 16th European Workshop on Efficiency and Productivity Analysis (EWEPA) help in London (June 10-13 2019) and at seminars at the Bank of Italy, Universities of Rome and Naples. Usual disclaimers apply.
Disclosure statement
No potential conflict of interest was reported by the author(s).
Additional information
Notes on contributors
Francesco Aiello
Francesco Aiello, PhD, correspondent author. An applied economist and Full Professor in Economic Policy at the University of Calabria (Italy). His email is [email protected]. His interest research topics are firm productivity, efficiency, applied micro-econometrics and regional economic divide.
Graziella Bonanno
Graziella Bonanno, PhD. Associate Professor of Economic Policy at the Department of Economics and Statistics of the University of Salerno. Her research interests include economic efficiency and the quantitative analysis of productive industries, banking, trade, regional divide, firm behaviour, innovation and firm internationalization strategies.
Francesco Foglia
Francesco Foglia, PhD, works at the European Commission in Brussels at the Joint Audit Directorate for Cohesion (Directorates-General for Employment, Social Affairs and Inclusion and for Regional and Urban Policy). His research interests include regional and financial economics, innovation policies and public audit.
Notes
1 Antonucci et al. (Citation2014); Castro (Citation2009); Elbialy and García-Rubio (Citation2011); Espasa and Esteller-Moré (Citation2015); Falavigna et al. (Citation2015); Falavigna et al. (Citation2018); Fauvrelle and Almeida (Citation2018); Ferrandino (Citation2012); Ferro et al. (Citation2018); Finocchiaro Castro and Guccio (Citation2014); Finocchiaro Castro and Guccio (Citation2018); Fusco et al. (Citation2018); Gorman and Ruggiero (Citation2009); Guzowska and Strak (Citation2010); Ippoliti et al. (Citation2015a; Citation2015b); Ippoliti and Ramello (Citation2018); Ivanova (Citation2014); Kittelsen and Førsund (Citation1992); Major (Citation2015); Mattsson and Tidanå (Citation2019); Melcarne and Ramello (Citation2015); Nissi et al. (Citation2019); Nissi and Rapposelli (Citation2010); Odhiambo (Citation2014); Pedraja-Chaparro and Salinas-Jimenez (Citation2010); Peyrache and Zago (Citation2016); Rushid (Citation2018); Santos and Amado (Citation2014); Schneider (Citation2005); Silva (Citation2018); Sousa et al. (Citation2018); Tsai and Tsai (Citation2010); Yeung (Citation2014); Yeung and Azevedo (Citation2011, Citation2011).
2 Each paper, on average, contributes about 7 estimates of the efficiency scores (the median value is 3; the minimum is 1 and the maximum is 40). The efficiency score is that obtained from final models of primary papers and is treated as an individual observation, thereby preserving the case that a single study presents variability in results when estimating the courts frontier. This within-study variability is a value in meta-regression approach (cf. Section 4).
3 PRISMA is the acronym of Preferred Reporting Items for Systematic Reviews and Meta-Analyses (Havránek et al., Citation2020)..
4 Our metadata set comprises only the technical efficiency from papers analysing production frontiers. The reason is that the allocative efficiency is totally disregarded in the judicial efficiency literature as motivated by Pedraja-Chaparro and Salinas-JimeÂnez (2010). These scholars argue that estimating allocative efficiency of courts leads to approximate results because of the “absence of and of uncertainty surrounding prices in the non-market sectors “and “trade-off between public economy objectives and the possible justification of allocative inefficient behaviour on the basis of other objective, we find ourself on an unstable ground when evaluating allocative efficiency in this sector” (pp. 1401–2).
5 High heterogeneity in efficiency scores is found by Bravo-Ureta et al. (Citation2007) who investigated the efficiency scores of 167 studies conducted at the farm level over a span of four decades. The same applies for Thiam et al. (Citation2001) who conducted a review of 34 articles focusing on agricultural efficiency in developing nations. Sectoral variability in efficiency emerges in the MRA study on agriculture that was conducted by Ogundari (Citation2014), which examined the dynamics of African agricultural efficiency levels based on 442 frontier studies. Djokoto and Gidiglo (Citation2016) as well as Djokoto and Gidiglo (Citation2016) specifically explored the heterogeneity in efficiency scores derived from 34 primary papers on Ghanaian agriculture. Iliyasu et al. (Citation2014) aimed to elucidate the observed variability in 36 efficiency papers related to aquaculture. Fan et al. (Citation2018) conducted a comprehensive review of 49 studies providing field experimental results on water use efficiency in wheat and cotton cultivation. Brons et al. (Citation2005) focused their research on 45 studies concerning urban transport. Odeck and Bråthen (Citation2012) analyzed the variability of efficiency of seaports using insights from 40 published papers. Nguyen and Coelli (Citation2009) delved into hospital efficiency by examining 95 studies published between 1987 and 2008. Similarly, Kiadaliri et al. (Citation2013) performed a systematic review of Iranian hospital efficiency, drawing from 29 primary papers. Havranek et al. (Citation2012) reviewed 32 studies with 53 observations regarding banking efficiency in the US during the period 1977–1997. Banking efficiency was also the focal point of the MRA conducted by Aiello and Bonanno (Citation2018), who reviewed 120 studies comprising 1,661 observations published from 2000 to 2014. Assaf and Josiassen (Citation2016) conducted a meta-review of tourism literature by comparing the efficiency scores derived from 57 frontier papers. Tian and Yu (Citation2012) focused on the productivity growth in China based on over 5,000 point-observations extracted from 150 primary studies. Lastly, Simões and Marques (Citation2012) conducted an MRA on 107 studies focusing on efficiency in the waste sector.
6 Differently from other MRAs on efficiency – i.e., Aiello and Bonanno (Citation2019) distinguish data in even cost, production and profit banks efficiency – in the case of judicial efficiency, scholars estimate production frontiers and, in the majority of studies, take as output the resolved cases at court level (see footnote 9). Here, it is worth noting that in the studies of courts efficiency, the scholars using the parametric approach commonly employ a Cobb Douglas stochastic frontier and assume that the two components of the error term follow normal and half-normal distributions. These choices impede the investigation of whether the diversity in court efficiency can be attributed to different functional forms and error term distributions. For more insights into these methodological issues see, among many others, Bonanno et al. (Citation2017).
7 Technically, the second step of estimations employs 1/(σi2 + τ2) as weights, where σi2 is the standard deviation of the estimated effect in study i and τ2 is the between-study variability wchich is estimated in the first step.
8 The set of inputs chosen by scholars is mixed. Every paper uses the number of judges as input, which is combined with the number of the administrative staff in a high number of papers. Alongside these two main inputs, the caseload (civil and/or criminal) is used in few studies (i.e., Finocchiaro Castro & Guccio, Citation2018; Schneider, Citation2005; Tsai & Tsai, Citation2010). The number of pending and/or incoming cases is an additional input used by others (i.e., Falavigna et al., Citation2015; Finocchiaro Castro & Guccio, Citation2014; Melcarne & Ramello, Citation2015), while a proxy of physical capital, that is the number of computers, enters as input in three papers only (Elbialy & García-Rubio, Citation2011; Ivanova, Citation2014; Yeung & Azevedo, Citation2011). Additional details on the meta dataset are available upon request.
9 The output variable used in primary papers is the number of resolved cases: settling a case in due time is meant to be the positive output of a judicial system. The number of resolved cases is the unique and “overall” output of the production function in 21 out of 36 papers. When using frontiers with more than one output, the focus remains on the settled disputes, but with disaggregated data according to judicial-type (civil resolved cases; criminal resolved cases), court-type (adjudication first or second degree). Just in Gorman and Ruggiero (Citation2009), the misdemeanor cases closed, felony cases closed and felony jury verdicts are considered as outputs.
10 It is important to point out that the different ways of performing an efficiency study make conclusive expectations of the impact of each meta regressor difficult. In other words, despite the high degree of specialisation of efficiency scholars, the effect of some methodological choices remains uncertain. For instance, results in parametric papers may be higher or lower than those obtained in non-parametric papers, according to the nature of disturbances from the frontier (Nguyen & Coelli, Citation2009). Again, panel data would yield higher efficiency levels than cross-sectional data. Finally, efficiency would increase with the number of variables included in the frontier, while it would decrease with small sample sizes (Berger & Humphrey, Citation1997; Coelli, Citation1995; Fethi & Pasiouras, Citation2010; Nguyen & Coelli, Citation2009). However, while theory predicts the likely impact of any choice, the actual measure of how sensitive the results are to the study design is an issue to be addressed empirically.
11 Because of data limitation we cannot further disaggregate the information of the courts specialisation as retrieved from primary papers. For instance, the sample comprises 97 observations related to prior studies focusing on civil, commercial, criminal and family courts and 64 efficiency scores regard the entire national judicial systems of the country that are scrutinized in the primary works. The remaining observations come from papers focusing only on a specific court type, but the number is very limited (i.e., only 5 scores regard administrative courts, 4 commercial and interior, 16 labor, 10 social security and 6 for tax courts). If we had more disaggregated data we could have compared the results between more homogeneous groups (i.e, efficiency of civil versus criminal courts, civil versus tax courts and so on). On this point see also footnote 14.
12 A sensitivity analysis was conducted to assess the robustness of the findings by substituting the variable “Year of publication” with the variable “Year of estimation” as referenced in the primary study. Results are similar and available upon request.
13 Here it is important to point out that Equationeq. [4][4]
[4] has been also estimated only for non-parametric primary papers. This required to replace the variable Parametric with the dummy DVRS, which is equal to unity for efficiency scores obtained from DEA papers based on the assumption of variable returns to scale and zero otherwise. The evidence is twofold: first, assuming that the technology in producing judicial services exhibits constant or variable returns to scale does not help in explaining why efficiency scores obtained when applying DEA differ paper-by-paper (indeed, the coefficient associated with DVRS is not statistically significant); second, the focus on the sub-sample of nonparametric corroborates all findings reported in , in the sense that the sign and the magnitude of the other parameters hold. Estimations are available upon request.
14 Here, it is worth mentioning the value of analysing the existence of potential differences in results when considering different courts specialization. While we thank an anonymous referee for pointing this out, the meta dataset does not allow opening the variable Specialised courts into type of courts (i.e, civil, commercial, penal, tax, labor) because the number of observations of each group is very small (cf. footnote 11). This issue is left for future research, provided that the primary literature on the efficiency of judicial systems will increase over time.
References
- Aiello, F., & Bonanno, G. (2016). Efficiency in banking: A meta-regression analysis. International Review of Applied Economics, 30(1), 112–24. https://doi.org/10.1080/02692171.2015.1070131
- Aiello, F., & Bonanno, G. (2018). On the sources of heterogeneity in banking efficiency literature. Journal of Economic Surveys, 32(1), 194–225. https://doi.org/10.1111/joes.12193
- Aiello, F., & Bonanno, G. (2019). Explaining differences in efficiency: A meta-study on local government literature. Journal of Economic Surveys, 33(3), 999–1027. https://doi.org/10.1111/joes.12310
- Antonucci, L., Crocetta, C., & d’Ovidio, F. D. (2014). Evaluation of Italian judicial system. Procedia Economics and Finance, 17, 121–130. https://doi.org/10.1016/S2212-5671(14)00886-7
- Assaf, A. G., & Josiassen, A. (2016). Frontier analysis: A state-of-the-art review and meta-analysis. Journal of Travel Research, 55(5), 612–627. https://doi.org/10.1177/0047287515569776
- Banker, R. D., Charnes, A., & Cooper, W. W. (1984). Some models for estimating technical and scale inefficiencies in data envelopment analysis. Management Science, 30(9), 1078–1092. https://doi.org/10.1287/mnsc.30.9.1078
- Berger, A. N., & Humphrey, D. B. (1997). Efficiency of financial institutions: International survey and directions for future research. European Journal of Operational Research, 98(2), 175–212. https://doi.org/10.1016/S0377-2217(96)00342-6
- Bonanno, G., De Giovanni, D., & Domma, F. (2017). The ‘wrong skewness’ problem: A re-specification of stochastic frontiers. Journal of Productivity Analysis, 47(1), 49–64. https://doi.org/10.1007/s11123-017-0492-8
- Bravo-Ureta, B. E., Solís, D., Moreira López, V. H., Maripani, J. F., Thiam, A., & Rivas, T. (2007). Technical efficiency in farming: A meta-regression analysis. Journal of Productivity Analysis, 27(1), 57–72. https://doi.org/10.1007/s11123-006-0025-3
- Brons, M., Nijkamp, P., Pels, E., & Rietveld, P. (2005). Efficiency of urban public transit: A meta-analysis. Transportation, 32(1), 1–21. https://doi.org/10.1007/s11116-004-0939-4
- Castro, A. S. (2009). Court performance in Brazil: Evidence from judicature-level data. (No. ID 2612941). Social Science Research Network.
- Chaffai, M. E. (2022). New evidence on Islamic and conventional bank efficiency: A meta-regression analysis. Bulletin of Economic Research, 74(1), 221–246. https://doi.org/10.1111/boer.12291
- Coelli, T. J. (1995). Recent developments in frontier modelling and efficiency measurement. Australian Journal of Agricultural Economics, 39(3), 219–245. https://doi.org/10.1111/j.1467-8489.1995.tb00552.x
- Coelli, T., & Perelman, S. (1999). A comparison of parametric and non-parametric distance functions: With application to European railways. European Journal of Operational Research, 117(2), 326–339. https://doi.org/10.1016/S0377-2217(98)00271-9
- Cook, W. D., Tone, K., & Zhu, J. (2014). Data envelopment analysis: Prior to choosing a model. Omega, 44, 1–4. https://doi.org/10.1016/j.omega.2013.09.004
- Djokoto, J. G., & Gidiglo, K. F. (2016). Technical efficiency in agribusiness: A meta-analysis on Ghana. Agribusiness, 32(3), 397–415. https://doi.org/10.1002/agr.21457
- Elbialy, N., & García-Rubio, M. A. (2011). Assessing judicial efficiency of Egyptian first instance courts: A DEA analysis. MAGKS Papers on Economics (No. 201119). Philipps-Universität Marburg, Faculty of Business Administration and Economics, Department of Economics (Volkswirtschaftliche Abteilung).
- Espasa, M., & Esteller-Moré, A. (2015). Analyzing judicial courts’ performance: Inefficiency vs. Congestion. Revista De Economia Aplicada, 23, 61–82.
- European Commission. (2017). European semester: Thematic factsheet – effective justice systems. European Commission. https://commission.europa.eu/system/files/2018-06/european-semester_thematic-factsheet_effective-justice-systems_en_0.pdf
- Falavigna, G., Ippoliti, R., Manello, A., & Ramello, G. B. (2015). Judicial productivity, delay and efficiency: A directional distance function (DDF) approach. European Journal of Operational Research, 240(2), 592–601. https://doi.org/10.1016/j.ejor.2014.07.014
- Falavigna, G., Ippoliti, R., & Ramello, G. B. (2018). DEA-based Malmquist productivity indexes for understanding courts reform. Socio-Economic Planning Sciences, 62, 31–43. https://doi.org/10.1016/j.seps.2017.07.001
- Fall, F., Akim, A.-M., & Wassongma, H. (2018). DEA and SFA research on the efficiency of microfinance institutions: A meta-analysis. World Development, 107, 176–188. https://doi.org/10.1016/j.worlddev.2018.02.032
- Fan, Y., Wang, C., & Nan, Z. (2018). Determining water use efficiency of wheat and cotton: A meta-regression analysis. Agricultural Water Management, 199, 48–60. https://doi.org/10.1016/j.agwat.2017.12.006
- Farrell, M. J. (1957). The measurement of productive efficiency. Journal of the Royal Statistical Society, 120(3), 253–281. https://doi.org/10.2307/2343100
- Fauvrelle, T. A., & Almeida, A. T. C. (2018). Determinants of judicial efficiency change: Evidence from Brazil. Review of Law and Economics, 14(1), 14. https://doi.org/10.1515/rle-2017-0004
- Ferrandino, J. (2012). The impact of revision 7 on the technical efficiency of florida’s circuit courts. Justice System Journal, 33(1), 22–46. https://doi.org/10.1080/0098261X.2012.10768000
- Ferro, G., Romero, C. A., & Romero-Gómez, E. (2018). Efficient courts? A frontier performance assessment. Benchmarking: An International Journal, 25(9), 3443–3458. https://doi.org/10.1108/BIJ-09-2017-0244
- Fethi, M. D., & Pasiouras, F. (2010). Assessing bank efficiency and performance with operational research and artificial intelligence techniques: A survey. European Journal of Operational Research, 204(2), 189–198. https://doi.org/10.1016/j.ejor.2009.08.003
- Finocchiaro Castro, M., & Guccio, C. (2014). Searching for the source of technical inefficiency in Italian judicial districts: An empirical investigation. European Journal of Law and Economics, 38(3), 369–391. https://doi.org/10.1007/s10657-012-9329-0
- Finocchiaro Castro, M., & Guccio, C. (2018). Measuring potential efficiency gains from mergers of Italian first instance courts through nonparametric Model. Public Finance Review, 46(1), 83–116. https://doi.org/10.1177/1091142116652723
- Fusco, E., Laurenzi, M., & Maggi, B. (2018). A data envelopment analysis of the Italian judicial efficiency. DSS Empirical Economics and Econometrics Working Papers Series (No. 2018/2). Centre for Empirical Economics and Econometrics, Department of Statistics, University of Rome.
- Gallet, C. A., & Doucouliagos, H. (2014). The income elasticity of air travel: A meta-analysis. Annals of Tourism Research, 49, 141–155. https://doi.org/10.1016/j.annals.2014.09.006
- Glass, G. V. (1976). Primary, secondary, and meta-analysis of research. Educational Researcher, 5(10), 3–8. https://doi.org/10.2307/1174772
- Glass, G. V., McGaw, B., & Smith, M. L. (1981). Meta-analysis in Social Research. Sage Publications, Beverly Hills.
- Gorman, M. F., & Ruggiero, J. (2009). Evaluating U.S. judicial district prosecutor performance using DEA: Are disadvantaged counties more inefficient? European Journal of Law and Economics, 27(3), 275–283. https://doi.org/10.1007/s10657-008-9093-3
- Guzowska, M., & Strak, T. (2010). An examination of the efficiency of Polish public sector entities based on public prosecutor offices. Operations Research and Decisions, 2, 41–57. https://ord.pwr.edu.pl/assets/papers_archive/161%20-%20published.pdf.
- Harbord, R. M., & Higgins, J. P. T. (2008). Meta-regression in Stata. Stata Journal, 8(4), 493–519. https://doi.org/10.1177/1536867X0800800403
- Havranek, T., Irsova, Z., & Janda, K. (2012). Demand for gasoline is more price-inelastic than commonly thought. Energy Economics, 34(1), 201–207. https://doi.org/10.1016/j.eneco.2011.09.003
- Havránek, T., Stanley, T. D., Doucouliagos, H., Bom, P., Geyer‐Klingeberg, J., Iwasaki, I., Reed, W. R., Rost, K., & Van Aert, R. C. M. (2020). Reporting guidelines for meta‐analysis in economics. Journal of Economic Surveys, 34(3), 469–475. https://doi.org/10.1111/joes.12363
- Iliyasu, A., Mohamed, Z. A., Ismail, M. M., & Abdullah, A. M. (2014). A meta-analysis of technical efficiency in aquaculture. Journal of Applied Aquaculture, 26(4), 329–339. https://doi.org/10.1080/10454438.2014.959829
- Ippoliti, R., Melcarne, A., & Ramello, G. B. (2015a). The impact of judicial efficiency on entrepreneurial action: A European perspective. Economic Notes, 44(1), 57–74. https://doi.org/10.1111/ecno.12030
- Ippoliti, R., Melcarne, A., & Ramello, G. B. (2015b). Judicial efficiency and entrepreneurs’ expectations on the reliability of European legal systems. European Journal of Law and Economics, 40(1), 75–94. https://doi.org/10.1007/s10657-014-9456-x
- Ippoliti, R., & Ramello, G. B. (2018). Governance of tax courts. Economics of Governance, 19(4), 317–338. https://doi.org/10.1007/s10101-018-0212-5
- Ivanova, N. B. (2014). Judicial reform and its effect on the economy: The case of Bulgaria [ Master Thesis]. Erasmus University Rotterdam,
- Kao, C., & Hung, H. T. (2008). Data envelopment analysis with common weights: The compromise solution approach. Journal of the Operational Research Society, 59(7), 893–903.
- Kiadaliri, A. A., Jafari, M., & Gerdtham, U.-G. (2013). Frontier-based techniques in measuring hospital efficiency in Iran: A systematic review and meta-regression analysis. BMC Health Services Research, 13(1). https://doi.org/10.1186/1472-6963-13-312
- Kittelsen, S. A. C., & Førsund, F. R. (1992). Efficiency analysis of Norwegian district courts. Journal of Productivity Analysis, 3(3), 277–306. https://doi.org/10.1007/BF00158357
- Lovell, C. A. K. (2002). Performance Assessment in the Public Sector. In: Fox, K.J. (eds). Performance assessment in the public sector. Efficiency in the Public Sector, Studies in the Productivity and Efficiency Series (pp. 11–35). Springer, Boston, MA. https://doi.org/10.1007/978-1-4757-3592-5_2
- Major, W. (2015). Data envelopment analysis as an instrument for measuring the efficiency of courts. Operations Research and Decisions, 25(4), 19–34. https://doi.org/10.5277/ord150402
- Marciano, A., Melcarne, A., & Ramello, G. B. (2019). The economic importance of judicial institutions, their performance and the proper way to measure them. Journal of Institutional Economics, 15(1), 81–98. https://doi.org/10.1017/S1744137418000292
- Mattsson, P., & Tidanå, C. (2019). Potential efficiency effects of merging the Swedish district courts. Socio-Economic Planning Sciences, 67, 58–68. https://doi.org/10.1016/j.seps.2018.09.002
- Melcarne, A., & Ramello, G. (2015). Judicial independence, judges’ incentives and efficiency. Review of Law and Economics, 11(2), 149–169. https://doi.org/10.1515/rle-2015-0024
- Merkel, A., & Holmgren, J. (2017). Dredging the depths of knowledge: Efficiency analysis in the maritime port sector. Transport Policy, 60, 63–74. https://doi.org/10.1016/j.tranpol.2017.08.010
- Nguyen, K. H., & Coelli, T. (2009). Quantifying the effects of modelling choices on hospital efficiency measures: A meta-regression analysis. Centre for Efficiency and Productivity Analysis. (No. WP072009). School of Economics, University of Queensland, Australia.
- Nissi, E., Giacalone, M., & Cusatelli, C. (2019). The efficiency of the Italian judicial system: A two stage data envelopment analysis approach. Social Indicators Research, 146(1–2), 395–407. https://doi.org/10.1007/s11205-018-1892-5
- Nissi, E., & Rapposelli, A. (2010). A data envelopment analysis of Italian courts efficiency. Statistica Applicata Italian Journal of Applied Statistics, 22(2), 199–210.
- Odeck, J., & Bråthen, S. (2012). A meta-analysis of DEA and SFA studies of the technical efficiency of seaports: A comparison of fixed and random-effects regression models. Transportation Research Part A: Policy and Practice, 46(10), 1574–1585. https://doi.org/10.1016/j.tra.2012.08.006
- Odhiambo, O. J. (2014). Technical Efficiency of the Kenyan Judiciary: A case of the magistrates‘ courts [ Master Thesis].
- Ogundari, K. (2014). The paradigm of agricultural efficiency and its implication on food security in Africa: What does meta-analysis reveal? World Development, 64, 690–702. https://doi.org/10.1016/j.worlddev.2014.07.005
- Ogundari, K., & Brümmer, B. (2011). Technical Efficiency of Nigerian agriculture: a meta-regression analysis. Outlook on Agriculture, 40(2), 171–180 https://doi.org/10.5367/oa.2011.0038.
- Park, S. Y., & Zhu, J. (2011). An exact algorithm for the DEA problem with a large number of outputs. European Journal of Operational Research, 210(1), 69–76.
- Pedraja-Chaparro, F., & Salinas-Jimenez, J. (2010). An assessment of the efficiency of Spanish courts using DEA. Applied Economics, 28(11), 1391–1403. https://doi.org/10.1080/000368496327651
- Peyrache, A., & Zago, A. (2016). Large courts, small justice! The inefficiency and the optimal structure of the Italian justice sector. Omega, 64, 42–56. https://doi.org/10.1016/j.omega.2015.11.002
- Ramello, G. B, and Voigt S. (2012). The economics of efficiency and the judicial system. International Review of Law and Economics, 32(1), 1–2. https://doi.org/10.1016/j.irle.2011.12.003
- Rushid, A. R. (2018). Technical efficiency of Swedish district courts [ Master Thesis].
- Santos, S. P., & Amado, C. A. F. (2014). On the need for reform of the Portuguese judicial system – does data envelopment analysis assessment support it? Omega, 47, 1–16. https://doi.org/10.1016/j.omega.2014.02.007
- Schneider, M. R. (2005). Judicial Career Incentives and court performance: An empirical study of the German Labour courts of appeal. European Journal of Law and Economics, 20(2), 127–144. https://doi.org/10.1007/s10657-005-1733-2
- Seiford, L. M., & Thrall, R. M. (1990). Recent developments in DEA: The mathematical programming approach to frontier analysis. Journal of Econometrics, 46(1–2), 7–38. https://doi.org/10.1016/0304-4076(90)90045-U
- Silva, M. C. A. (2018). Output-specific inputs in DEA: An application to courts of justice in Portugal. Omega, 79, 43–53. https://doi.org/10.1016/j.omega.2017.07.006
- Simões, P., & Marques, R. C. (2012). Influence of regulation on the productivity of waste utilities. What can we learn with the Portuguese experience? Waste Management, 32(6), 1266–1275. https://doi.org/10.1016/j.wasman.2012.02.004
- Sousa, M. D. M., Guimaraes, T. A., Guimaraes, T. A., & Sousa, M. D. M. (2018). Resources, innovation and performance in labor courts in Brazil. Revista de Administração Pública, 52(3), 486–506. https://doi.org/10.1590/0034-761220170045
- Stanley, T. D. (2001). Wheat from chaff: Meta-analysis as quantitative literature review. Journal of Economic Perspectives, 15(3), 131–150. https://doi.org/10.1257/jep.15.3.131
- Thiam, A., Bravo-Ureta, B. E., & Rivas, T. E. (2001). Technical efficiency in developing country agriculture: A meta-analysis. Agricultural Economics, 25(2–3), 235–243. https://doi.org/10.1111/j.1574-0862.2001.tb00204.x
- Tian, X., & Yu, X. (2012). The enigmas of TFP in China: A meta-analysis. China Economic Review, 23(2), 396–414. https://doi.org/10.1016/j.chieco.2012.02.007
- Trong Ho, P., Burton, M., Ma, C., & Hailu, A. (2022). Quantifying heterogeneity, heteroscedasticity and publication bias effects on technical efficiency estimates of rice farming: A meta-regression analysis. Journal of Agricultural Economics, 73(2), 580–597. https://doi.org/10.1111/1477-9552.12468
- Tsai, C., & Tsai, J. (2010). Performance evaluation of the judicial system in Taiwan using data envelopment analysis and decision trees. 2010 Second International Conference on Computer Engineering and Applications, 2, 290–294. Bali, Indonesia.
- Voigt, S. (2016). Determinants of judicial efficiency: A survey. European Journal of Law and Economics, 42(2), 183–208. https://doi.org/10.1007/s10657-016-9531-6
- Xu, T., Dong, J., & Qiao, D. (2023). China’s marine economic efficiency: A meta-analysis. Ocean & Coastal Management, 239, 106633. https://doi.org/10.1016/j.ocecoaman.2023.106633
- Yeung, L. (2014). Measuring efficiency of courts: An assessment of Brazilian courts Productivity. In A. Emrouznejad & E. Cabanda (Eds.), Managing service productivity: Using frontier efficiency methodologies and multicriteria decision making for improving service performance (pp. 155–165). Springer.
- Yeung, L. L., & Azevedo, P. F. (2011). Measuring efficiency of Brazilian courts with data envelopment analysis (DEA). IMA Journal of Management Mathematics, 22(4), 343–356. https://doi.org/10.1093/imaman/dpr002
- Yeung, L., & Azevedo, P. (2011). Measuring the Efficiency of Brazilian Courts from 2006 to 2008: What Do the Numbers Tell Us?. Insper Working Paper.
Appendix
Table A1. Correlation matrix of the variables used in the analysis.