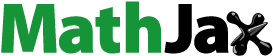
ABSTRACT
The study examines the long and short-run impact of different explanatory variables on manufacturing development the time span from 1996 to 2021. The results indicated that economic freedom, FDI inflows, financial development, political instability, corruption control, government effectiveness, the rule of law, voice and accountability, and regulatory qualities significantly expand industrialization at the 1% level in the short-run, whereas they significantly reduced industrialization at the 1% level in the long run. African manufacturing transformation is significantly and positively determined by income per capita in the long and short run. The study suggests that policy measures must be taken to combat corruption and improve government effectiveness to achieve manufacturing growth. Maintaining a sound individual and whole institutional quality is very crucial for manufacturing development in the short run.
1. Introduction
Industrialization plays a critical role in the process of a nation’s economic development. Manufacturing expansion serves as the main justification for industrialization (Szirmai, Citation2012). It could help close the technological gap by promoting the adoption of new technologies and the creation of high-productivity employment (Rodrik, Citation2013). The fastest-growing manufacturing sectors have increased manufacturing employment and output in developed countries. In contrast to this, it is believed that the ability to sustain industrial production growth is critical to achieving structural transformation. Manufacturing development is inextricably tied to manufacturing productivity, which determines the share of manufacturing sectors and the level of employment (Shikur et al., Citation2020a). Since the manufacturing share of GDP is positively connected to company productivity and output growth (Shikur, Citation2022c).
Emerging countries tend to pursue growth that is not always driven by industrialization, which contrasts with this early evidence (Diao et al., Citation2017; Rodrik, Citation2016). In reality, a variety of industries outside of manufacturing show higher productivity levels and a greater ability to absorb the labor force. The service sector development plays a crucial role in substantially influencing income, trade, employment, and even productivity growth (International Monetary Fund (IMF), Citation2018), this is not always the case in developing countries, where a process of “tertiarization” is still taking place in low-wage/low-productive non-tradable activities with a subsequent halt of economy-wide growth in the presence of low domestic demand. Today’s developing countries face challenges in achieving manufacturing development (Eichengreen & Gupta, Citation2009; International Monetary Fund (IMF), Citation2018).
In light of the discussion above, it is now even more crucial to understand what truly determines manufacturing development and why certain countries become more industrialized, and able to sustain an industrialization pattern over the previous few decades than others. Against such a background, the motivation of this study is to identify the driving factors of manufacturing development in Africa. A large body of literature argues that economic and development policies play a crucial role in achieving faster and more sustained manufacturing development. However, these economic policies could not successfully enable African countries to achieve industrialization goals (Shikur, Citation2020a). These policies did not a guarantee successfully continuous business profitability, and high rate of manufacturing production growth since industries have not been provided adequate and continuous incentives that lead them to have the low ability to sustain industrial productivity (Shikur et al., Citation2020a). Similarly, a strong institution plays a vital role in reducing transaction costs and production costs, and shaping the appropriate incentives and advancing manufacturing output growth by improving business profitability (Shikur, Citation2022d, Citation2022e) and through enhancing education, higher investment shares and lower fertility rates. Institutional quality has a big role in industrialization, which promotes inclusive and peaceful societies and gives equitable access to justice. Countries with strong institutions that uphold the rule of law, protect human rights, and combat corruption are more likely to assist a country in accomplishing its goals and promote industrialization growth.
This study is needed because it goes in several ways beyond previous research. The body of literature suggests a positive link between institutions and economic growth. However, the relationship between institutions and economic growth varies depending on countries’ income levels. The effects of institutions are determined by a variety of factors, including an individual’s perception of the institutions and the social mores and practices of a particular community. Although many studies examined the role of institutional quality in economic growth in Africa, only few studies studied the role of institutions in explaining industrialization. Only two papers are available on similar topic. Thus, this study chooses low middle income (LMI) Sub- Saharan African countries (excluding high income) that had high population density with notable regional similarity. The countries of Sub-Saharan Africa are classified as IDA and IBRD by World Bank Groups. The nations classified as IDA are those that are unable to obtain loans from IBRD. “IDA credits” are very substantial interest-free loans and subsidies for projects aimed at improving economic growth and rising living standards.
The earlier literature has yet to reach consensus on either institutional quality does affect manufacturing production growth or nor. Thus, theoretically, impact of institutional quality on manufacturing growth is ambiguous. The findings of Haraguchi et al. (Citation2018) and Mijiyawa (Citation2017) showed contradicting results and identified the existence of sign and magnitude of coefficient heterogeneity in their empirical results, it is challenging to identify heterogeneity. This implies that results may be more sensitive to sample size, sampled countries types, data types, model selection, periods, and economic policies. This paper dilemma is similar to the literature on nexus between institutional quality and manufacturing growth. These earlier studies did not evaluate the contribution of regulatory quality, rule of law, absence of violence, voice and accountability, and legal system to manufacturing development in African countries (e.g., Haraguchi et al., Citation2018; Mijiyawa, Citation2017). Economic freedom is included in the model as the potential determinant of industrialization that has not been so far considered in the previous studies (e.g., Haraguchi et al., Citation2018; Hartwell, Citation2016; Horváth & Zeynalov, Citation2016; Mijiyawa, Citation2017). The corruption has negative effect on growth (Uddin et al., Citation2023).
Second, the most of the earlier empirical studies focus on cross-sectional and panel data. Further, the study is differentiated from the existing research investigating the relationship where in economic freedom is examined in the both long and short-runs and hypothesized that economic freedom can affect industrialization in the long run, unlike short run. The application of the panel ARDL models is very crucial to distinguish between the short-run and long-run impacts, which make this study the first. The sample of sub-Saharan African countries and econometric framework provide a unique opportunity to more fully investigate the impacts of institutions and address heterogeneity in their empirical results due to models used. Earlier authors focus on the dynamics panel models that could not identify heterogeneity on dynamic adjustment in the short-run and long-run. For example, generalized two-stage Least Squares (2SLS), System GMM methods and dummy panel models were employed by Horváth and Zeynalov (Citation2016), Hartwell (Citation2016), Mijiyawa (Citation2017) and Haraguchi et al. (Citation2018) respectively to measure the effect of institutional quality on manufacturing expansion. The panel ARDL framework explains some of the shortcomings of previous empirical studies that explain the short-run or long-term impacts. Institutions do not typically change significantly over short run and are persistent for a very long-run. However, institutional frameworks have drastically changed during the course of the last two decades in several post-Soviet countries. The study investigates the impact of institutional quality and economic freedom on manufacturing production growth in Sub- Saharan
African context using panel ARDL models, which make this study the first. These models make them possible to distinguish between the short-run and long-run impacts. The aim of the study is to identify heterogeneity on dynamic adjustment in the short-run and long-run. The study provides a new insight to the region-based interactions of institutional quality with manufacturing development. This study attempts to fill this gap between practice and theory by including neglected low-middle income Sub-Saharan African countries in earlier studies.
The subsequent sections of the article are structured as follows. The theoretical and empirical literature on drivers of industrialization is discussed in Section 2. Data and methods of analysis are presented in Section 3 of this article. To examine the variables driving the manufacturing development in Africa, data are analyzed using panel ARDL models; Empirical results are presented and discussed in Section 4. The study is concluded with a discussion of possible policy implications in Section 5.
2. Literature review
First institutions are driving factor, even though it is now clear that achieving economic growth and manufacturing development depends on the complex interaction of many factors, including macroeconomic and sectoral policies, investments in physical capital, research and public infrastructure, and geography. H. Chenery (Citation1988) argues that both macroeconomic and sectoral policies influence structural transformation. Industrialization is derived from industrial policies (Haraguchi et al., Citation2018; Shikur, Citation2020b; Shikur etal., Citation2021) which are consistent with Chenery’s views. This integrated approach involves reciprocal interactions between rising income and changing demand and supply proportions. The dynamic version of the Keynesian model argues that accumulation (i.e., investments in physical capital, research and public infrastructure) and sectoral decomposition affect manufacturing development. Investments in infrastructure, and adoption of science and technology play significant roles in ensuring sustained structural transformation, thereby enhancing economic growth (Rostow, Citation1960). The infrastructure sub-indices (transport infrastructure, energy infrastructures, financial infrastructure and communication infrastructure) are among major drivers of sustained structural transformation by ensuring sustained profits, and lowering entry costs into various sectors with a greater public capital intensity.
Institutions are one of the most significant and “deep” determinants for sustained manufacturing development by influencing the structure of economic incentives in the society (Acemoglu et al.,). Institutions are crucial to the process of economic development because they shape the incentives of the key economic actors, in particular because they influence investments in technology, human and physical capital, the structure of production. The strong institutions guarantee the highest degree of economic liberty that could promote economic growth and advancement. According to Singh and Pradhan (Citation2020), governance is the set of practices and institutions that affect economic development. Establishing quality institutions is crucial for advancing a nation’s economic growth. Economic growth differences across countries are explained by institutions, which also play a key role in variations in capital accumulation, productivity growth, and educational attainment. Mijiyawa (Citation2017) examined the relationship between institutions and industrialization in the case of Africa. He found that low level of corruption and better government effectiveness has a significant contribution to African manufacturing development using the system GMM method. Therefore, combating corruption and improving government effectiveness are key for manufacturing growth. Haraguchi et al. (Citation2018) studied the relationship between the political stability and industrialization in Africa countries. The results indicated that political stability significantly increases industrialization over the whole periods and post-1990 whereas it decreases industrialization pre-1990. Institutional quality affects growth through enhancing education, higher investment shares and lower fertility rates. In that sense, a poor country improves the quality of its institutions in the direction of stronger protection of property rights, it increases the likelihood that the country would experience higher levels of economic success.
The best institutions that are liberalized markets are important mechanisms to promote investment, and thus economic growth. Liberalized economies provide the highest level of economic freedom and protection for private property rights, especially for profit-seeking businesses. On the other hand, market failures are more common in countries with high levels of corruption, and the government’s efforts to rectify these failures are probably less successful. But a lack of institutions discourages investment, particularly foreign investment, and impedes the dissemination of innovations both domestically and globally. The limited impact of flawed institutions affects all economic sectors. According to Aron (Citation2000), in situations where institutions are weak, resources are confined to areas with poor productivity and economic activity is limited to individual transactions. Empirical results confirm that finding that the qualities of institutions (such as rule of law o control of corruption) affect manufacturing expansion using Generalized two-stage Least Squares (2SLS) in post-Soviet countries (Horváth & Zeynalov, Citation2016) and using GMM methods in rich resource countries (Hartwell, Citation2016). In reality, FDI inflows had little contribution to structural transformation (Mijiyawa, Citation2017). Yuldashev et al. (Citation2023) claimed institutional quality responds positively to economic growth using series data and the OLS technique from 1979 to 2018. But EG is harmed by both international and indigenous investment. Between 2000 and 2018 (Ngo and Nguyen, 2020) research was done in low- and moderate-income Asian nations. The study looks at EG, overall productivity, and institution quality using quantile regression. The findings showed that while TFP and interaction variables had a positive impact on EG, institutional determinants had a negative effect since low-middle-income countries have weak institutions. Ouoba and Sawadogo (Citation2019) contend that ineffective resource management occurs in African countries as a result of weak, and unreasoned institutions. Using the GMM methodology, Khan and Hanif (Citation2020) looked at the relationship between inflation, economic growth, and institutional quality for a large panel of 113 economies for the years 1981–2015. They demonstrated that while there is a negative correlation between growth and inflation, IQ has a favorable impact on EG. Jude and Levieuge (Citation2013) examined the relationship between IQ and the growth effect of foreign direct investment (FDI) in developing nations and found that FDI has a positive growth effect above a particular IQ threshold. Bhattacharjee and Haldar (Citation2015) focused on the institution while examining growth drivers.
Above scholars argue that institutions affect the price we pay for our actions, thus determining the profitability and feasibility of engaging in the economy. It provides the incentive structure of an economy; as that structure evolves, it shapes the direction of economic change toward growth, stagnation, or decline activity. In that sense, strong institution plays vital role in reducing transaction costs and production costs, and shaping the appropriate incentives that drive long-run growth and development. Low-risk investments and inexpensive transactions are associated with strong institutions. They encourage the creation and use of cutting-edge technologies that boost industry production and gather material and people resources. As a result, institutions have a big say in how the economy allocates resources and how industrialization proceeds.
Economic institutions consistent with the principles of monetary policy and economic freedom are associated with higher manufacturing development. Formal economic institutions, such as the banking system, property rights, and competitive markets, have a significant impact on how society invests in capital – human, physical, and technological – and how production is organized. These institutions are especially vital for ensuring a sustainable society, and promoting societal wellbeing and socioeconomic growth. Financial development plays a vital role for industrialization that ensures funding for the best investment opportunities, promoting capital formation and improving distribution of risks. Well-functioning financial institutions promote technological innovation by selecting and funding the winners, i.e., entrepreneurs with a high likelihood of implementing innovative processes and producing innovative products. The research result indicates that credit development is the main driving factor of structural transformation (Haraguchi et al., Citation2018; Shikur, Citation2023).
The long-term economic growth is determined by the Economic Freedom of the World index, using genetic variation as a trustworthy source of exogenous variation. According to Gwartney et al. (Citation2015), the essential elements of economic freedom are the protection of private property rights, the ability for voluntary exchange, the freedom to enter and compete in markets, and the exercise of individual choice. The “rules of the game,” or the establishments and behaviors that are most usually associated with economic freedom, are open markets, limited government, private property rights, and sound monetary policy. International openness can influence the structural transformation directly or indirectly (Rodrik, Citation2008). Improvement in economic freedom has a significant role in attracting FDI. Quality institutions have significant contribution to the establishment of a positive business climate for the private sector and as an essential prerequisite for attracting FDI. The essential premise is that a powerful and stable institution can guarantee the successful execution of a long-term strategy, which may be required to encourage the development of new sectors (Shikur et al., Citation2020a, Shikur, Citation2024).
Good institutions are also the ways to overcome the natural resource curse (Hartwell, Citation2016; Horváth & Zeynalov, Citation2016). These authors argue that the natural resources are cursed only in the countries characterized by weak institutions. They find that natural resource exports crowd out manufacturing sector. Regarding the mechanism by which a rise in natural resource exports may increase the prices of natural resources, thus may crowd out the other sectors in the economy, it is useful to think of Dutch disease effect. Other mechanisms include a reduction in human capital, retarding development of economic institutions, a skewing development of incentives for prudent policies due to a greater sense of security, fostering conflicts, increasing economic fragility, changing the development of political institutions, and especially in encouraging rent seeking (Hartwell, Citation2016). As a result, countries with more resources generally grow more slowly than those with fewer. This implies that strong institutions are main driving factor in the nexus between natural resources and manufacturing expansion. Similarly, Haraguchi et al. (Citation2018) find that natural resource rent is negatively correlated with industrialization using dummy panel models in developing countries (Haraguchi et al., Citation2018). Alexeev and Conrad (Citation2009)‘s contention that there is little to no net influence of natural resources on growth. However, Ahrend (Citation2012) finds that natural resources in Russian regions had a positive effect on economic growth early in the transition.
Collectively, the existing literature does not provide a conclusive answer. Thus, the potential theoretical effects of economic freedom and institutional quality on manufacturing expansion are unknown. The earlier findings demonstrate that institutional quality reacts differently based on the institutional framework and that these outcomes are influenced by the identification approach. Our results validate the lack of robustness found in earlier studies and emphasize the challenge of creating compelling policy recommendations. Empirical investigations might also yield contradictory evidence. According to Haraguchi et al. (Citation2018), political stability decreases manufacturing development prior to 1990 whereas it increases manufacturing development post -1990. Moreover, the drivers of structural changes vary across periods and countries (Haraguchi et al., Citation2018). The impact of institutional quality on industrialization is still a topic of debate in historical study on transition economies, including studies on African countries. In a follow-up study, Horváth and Zeynalov (Citation2016) and Hartwell (Citation2016) conclude that institutions have no discernible effect on manufacturing growth.
The panel ARDL model results support the idea that each institutional quality could increase economic freedom in democracies, but they also imply that low-quality institutions reduce economic freedom. Furthermore, our findings imply that the application of panel ARDL model increases manufacturing development and reduces economic freedom; nevertheless, in the panel ARDL model conducted in democracies, there is no appreciable correlation between institutional quality and manufacturing expansion. This study also includes the various driving factors determining manufacturing development to fill this scientific gap that could enable the countries to explain the strategic role of manufacturing in the development process. Therefore, by resolving the long and short-run issues with the macro-level application of the panel ARDL models, this research provides a solid foundation for evidence-based policymaking on the factors influencing the development of manufacturing in Africa.
3. Data and methods of analyses
3.1. Data
The dataset used for empirical analysis cover the annual observations for the 32 low-middle -income African countries from 1990 to 2021. The sources of data are the Fraser Institute databases and World Bank databases. The panel data combine cross-sectional and time-series data. The study chooses six institutional quality measures from the World Bank Governance Indicators because these variables are widely used in the literature. The selection of control variables is based on the findings of earlier research. The study considers variables that capture time varying heterogeneity. The benchmark control variables include GDP per capita, economic freedom, population growth, trade openness, FDI inflows, natural resource, inflation and financial development (Haraguchi et al., Citation2018; Hartwell, Citation2016; Horváth & Zeynalov, Citation2016).’ The size of the GDP across countries is not homogenous, thus, the paper uses per capita GDP measured at the constant price. All control variables are collected from World Bank databases.
3.2. Dynamic panel model ARDL
This paper follows econometric framework panel ARDL model developed by Pesaran et al. (Citation1999) to investigate the short-run and long-run impacts of multi-explanatory variables on manufacturing development. The panel ARDL model simultaneously estimates both short- and long-term dynamics. It means that it allows for the investigation of adjustment of variables toward both short- and long-term equilibrium conditions. This implies that this model makes it possible to differentiate between the short-run and long-run effects of multi-explanatory variables on industrialization. It accommodates various orders of integration, such as I (0), I(1), or a combination orders of integration, namely, I (0), I (1) variables as long as none of variables are I(2). Also, it accommodates a different number of lags on each variable.
This kind of model is based on three estimators: the Mean Group (MG) estimator, the Pooled Mean Group (PMG) estimator, and also the Dynamic fixed Effects (DFE) estimator. The PMG developed by Pesaran et al. (Citation1999) considers the long-run slope parameters homogenized throughout countries, but intercepts, the short-run coefficients and error variances are heterogeneous. The PMG estimator is an intermediate estimator between MG and DFE estimators, which simulating the structure of the ARDL model in a panel setting under the assumption of long-run homogeneity. It involves both pooling and calculating coefficient means, and generates consistent estimates of mean short run coefficients by taking a simple average of individual coefficients. The DFE is very similar to PMG; however, it furthermore restricts currently not the long-run coefficients but also the short-run to being identical throughout countries.
Under the dynamic fixed effect, it is assumed that slope coefficients and error variance are identical, but intercepts differ across groups. The DFE employs dynamic specification to generate heterogeneous parameters across groups. The MG suggested by Pesaran and Smith (Citation1995) that permits estimate groups/countries (N) separate regressions in the short and long runs. Specifically, this method estimates individual regressions for each country and after that, the unweighted mean is calculated. MG framework produces consistent estimates of average coefficients. MG estimator does not apply any homogeneity restriction, even if they are valid, while the assumption of parameter homogeneity that is the central to pooled MG estimators is restrictive.
This study applies these three estimators because the conventional estimating techniques, such as pooled OLS, fixed effect, or random effect do not permit the analysis of adjustment variables to both short- and long-term equilibrium scenarios. Moreover, these dynamic panel models require the large sample size of countries relative to the time periods. The GMM is largely targeted at the short-run dynamics. Henceforth, considering these shortcomings, the panel autoregressive disbursed lag (ARDL) models are sounder than dynamic panel models to overcome those drawbacks. Based on a review of empirical literature, this would be the first macro-paper that uses the panel ARDL to identify the determinants driving manufacturing development in low- and middle-income African countries. Furthermore, the report specifically looks at the impact that institutions and economic freedom have on the growth of Africa’s manufacturing sector.
A Hausman test has been conducted to compare standardized coefficients in the models. The Hausman specification test compares an efficient estimate under the test assumption to an estimator that is known to be consistent. It is widely known that PMG and MG are consistent; nevertheless, PMG is efficient under the assumption of long-run homogeneity. Therefore, the study has tested the null hypothesis that the difference between PMG and MG estimators is not statistically significant and, in addition, there is no significant difference between PMG and DFE estimators. If this is the case, then there should be no significant difference between the two estimators. If there is a systematic difference in the estimators, we have reason to doubt the assumptions that underlie the efficient estimator. The result indicates that the null hypothesis is accepted, so, PGM is more efficient than MG. The null hypothesis is that the estimator is a consistent and efficient estimator of the true parameters. The results indicated that the null hypothesis is consistent and efficient under the assumption that the long-run slopes and error variance are homogeneous. There is no statistical difference between PMG and DFE estimators. In this study, the long-run panel ARDL (p, q) is specified as follows:
Where, the panel dataset with groups/countries indexed by i = 1, 2, … ,N; time periods indexed by t = 1, … ,T; j is the number of lags; p and q are the lags of the dependent and independent variables, respectively. All variables represent country i in period t. Yi,t represents a manufacturing value added as a share of GDP per capita at constant prices in the period i-t; CV i,t is a set of control variables and Ii,t represents the institutional quality measures. In specific, φi,t represents the long-run parameters of the lagged dependent variable; ηi,t, and χi,t refer in addition to the long-run coefficients, but also denote the lagged independent variables, that in our case is control variables and the institutional variable. To estimate adjustment coefficient and long-run dynamics, the dynamic panel ARDL (p, q) is represented by the following equation:
Where, βi,t represents the short-run parameters of the lagged dependent variable; αj,t, and σi,t represent the short-run coefficients linking manufacturing development with its past values and the variables of interests CV i,t and Ii,t, but of the lagged independent variables, that in this case is the institutional variable. θi is adjustment coefficient of the long-run dynamics. γi,t displays the speed of adjustment to the long-run equilibrium. The long-run relationship between multi-explanatory variables and manufacturing development exists if θi is negative and significant, then there is cointegration relationships among variables.
The study uses six measures of institutional quality: include control of corruption, regulatory quality, rule of law, political stability and absence of violence, and voice and accountability. The indicators range from −2.5 to 2.5. Low value reflects bad quality of, whereas a high value indicates good-quality of institutions. In this study, the Government Effectiveness Index is used as a proxy for the quality of bureaucracy and the Corrupt Practices Index as a proxy for level of corruption in the country. The degree of political pressure independence, the standard of public and civil service, the efficiency of policy formulation and implementation, and the government’s confidence in carrying out these policies are all evaluated by the government effectiveness index.
Economic freedom is included in the model as the potential determinant of industrialization that has not been so far considered in the previous studies (e.g., Haraguchi et al., Citation2018; Hartwell, Citation2016; Horváth & Zeynalov, Citation2016; Mijiyawa, Citation2017). Developed index takes of quality of investment climate like, trade policy, the fiscal burden of government, government intervention in the economy, monetary policy, capital flows, and foreign investment, banking and finance, wages and prices, property rights, and regulation and black-market activity, averaging these ten indicators with aim of reflecting investment climate.
Economic Freedom Index (EFI) is a broad measure of (formal) institutions in empirical research that either integrates with or significantly correlates with other widely used indices of institutional quality. Executive constraints, legal origins, protection from expropriation, and a range of democracy and governance indices that gauge the effectiveness of governance in terms of things like bureaucratic efficiency and corruption control are a few examples of related measures. In this paper, economic freedom index, which is a composite score constructed employing data from Fraser Institute databases. The economic freedom index ranges from o to 5 with the higher score reflecting a greater economic freedom and a score of 1 indicating a consistent set of policies least conducive to economic freedom. The countries with lower economic freedom index ratings are less likely to influence the manufacturing value added as a share of GDP.
FDI inflows boost industrialization by promoting technology transfers. According to Shikur (Citation2024), FDI inflows are needed to support industrialization and economic diversification, accelerate structural transformation, and make stronger international trade integration. Per capita FDI inflows is calculated as a percentage of GDP per capita in the constant 2015 prices. A large body of literature argues that successful financial development stimulates industrialization by identifying and assisting the winners – entrepreneurs who have a high likelihood of implementing innovative practices and producing innovative goods (Shikur, Citation2023). The study uses data on domestic loans to the private sector (as a percentage of GDP) as a proxy for the level of Financial Development (FD). This study employs the number of telephones available per 1000 inhabitants as a proxy for infrastructure quality (IFSQ). However, it should be noted that, in addition to availability, infrastructure reliability (such as the frequency of telephone or power outages) is an important indicator of overall infrastructure quality, for which quantitative data are not readily available in most developing nations.
GDP per capita (GDPpc) increases the manufacturing development by creating demand for local and foreign firms’ products (Mijiyawa, Citation2017). GDP per capita is included in the model as proxy for income per capita and operationalized as GDP per capita in the constant 2015 prices. Population growth stimulates industrialization by providing cheap labor forces and creating huge demand for manufacturing goods. Economic openness (EO) is the sum of exports and imports as a percentage of GDP in constant 2015 prices. Trade openness has a vital role for manufacturing development by promoting technology transfer and increasing foreign market access (Mijiyawa, Citation2017). As a result, trade openness is predicted to boost the manufacturing sector’s share of GDP.
The natural resources reduce industrialization by reducing competitiveness, retarding development of economic institutions, fostering conflicts, increasing economic fragility, changing the development of political institutions, and especially in encouraging rent seeking (Hartwell, Citation2016). The resource rents are calculated as a percentage of GDP by adding the hard coal rents, soft coal rents, mineral rentals, oil rents, natural gas rents, and forest rents. Similarly, natural resource rent is negatively correlated with industrialization (Haraguchi et al., Citation2018).
4. Results
4.1. Summary of the descriptive statistics
The descriptive statistics for the chosen variables are shown in . The matched mean values of CC, GE, RQ, and RL are −0.58, −0.67, −0.67 and −0.59, respectively. The GDP per capita, manufacturing value added as % of GDP, financial development and population growth rate have means of 12.67, 37.67, 2437.84 and 2.33, respectively.
Table 1. Summary of the descriptive statistics.
FDI stands for volume of FDI inflows. FD refers to financial development. PGR, and GDPpc represent population growth rate, and per capita GDP, respectively.
The countries of Sub-Saharan Africa are classified as IDA and IBRD by World Bank groups. The nations classified as IDA are those that are unable to obtain loans from IBRD. “IDA credits” are very substantial interest-free loans and subsidies for projects aimed at improving economic growth and rising living standards. IBRD loans are not revolving. Blend countries are eligible for both IDA and IBRD credits because of their low per capita income and strong creditworthiness. (Sub-Saharan African countries differ from other economic regions as classified by the World bank. per capita income trends are displayed for each region separately in .
Low income (LIC), Least developed countries (LDC), Sub-Saharan Africa (SSF), Sub-Saharan Africa (excluding high income) (SSA), Middle income (MIC), Sub-Saharan Africa (IDA & IBRD countries) (TSS), Africa Eastern and Southern (AFE) Africa Western and Central (AWC), Arab World (ARB), Caribbean small states (CSS), and Euro area (EUM)
summarize manufacturing development trends for various regions separately.
Figure 2. Manufacturing value added (% of GDP) growth trends. It describes the share of manufacturing to GDP trends the y-axis refers to manufacturing, value added measured using constant 2015 US$; the x-axis refers to the time periods over the period 1996–2022.

Figure 3. Manufacturing, value added (% of GDP) growth trends the y-axis refers to manufacturing, value added measured using constant 2015 US$; the x-axis refers to the time periods over the period 1996–2022.

Figure 4. Manufacturing, value added (constant 2015 US$) trend overtime. The y-axis refers to manufacturing, value added measured using constant 2015 US$; the x-axis refers to the time periods over the period 1996–2022.

In addition to the comparison of PMG, Mg, and DFC, the estimated coefficients in show that the estimated coefficients are stable in terms of trend and significance. The results show that CD tests reject strongly null hypothesis of the cross-sectional dependence in .
Table 2. Results of the cross-sectional dependence test with the help of pesaran CD test.
4.2. Model results
The results indicate that economic freedom significantly leads to industrialization expansion at the 1% level in the short-run () whereas it significantly results in a shirking manufacturing sector at the 1% level in the long run (). A 1% rise in economic freedom is associated with a 0.07% decrease in the manufacturing proportion of GDP in the long-run. This is an intriguing discovery, and it demonstrates that economic freedom would fail to shift resources from less efficient sectors to more productive sectors in the long run, just as it did during the short-run in Africa. In the long run, industrial expansion can no longer be achieved by improvement in economic freedom. Indeed, in contrast to the East Asian Tigers, African governments frequently adopted inconsistent incentives or industrial policies to stimulate industrial development by improving economic freedom. Growth must be continuous policy-based improving productivity. Since, the policies have failed in Africa (Shikur, Citation2020b) because it does not guarantee continuous profitability that matters manufacturing growth by decreasing the entrance of new firms into sectors, and hampers the growth of domestic production links, both of which are required for economic transformation (Di Maio, 2009).
Table 3. The effect of institutional quality on structural transformation in the short-run.
Table 4. The effect of institutional quality on structural transformation in the long run.
The findings suggest that the rule of law, voice and accountability, and regulatory quality indices have a positive and significant impact on the manufacturing share of GDP in the short-run (), corresponds to the previous findings of Horváth and Zeynalov (Citation2016), using generalized two stage square, and Hartwell (Citation2016), using System GMM methods. The results of the study indicate that enhancing political stability has a significant and positive contribution to the development of manufacturing activity in Africa in the short-run. Thus, an improvement in political stability is beneficial for industrialization in Africa. According to the findings, a one unit increase in the political stability index is associated with a 0.06% increase in the manufacturing share of GDP. This finding is in line with the work of Haraguchi et al. (Citation2018). The results indicate that government effectiveness and control of corruption significantly expand the industrialization that is similar to the work of Mijiyawa (Citation2017). Thus, combating corruption and improving government effectiveness are key for manufacturing growth.
However, all six dimensions of the institutions significantly impede the manufacturing development in the long run (). This could lead to relative reduction in manufacturing share of GDP. The results contradict with the theory that arguing institutions as a fundamental cause of long-run growth. The significant change in institutional quality has not been coinciding with structural transformation in the long-run due to low manufacturing production and productivity in African countries. The negative effect of institutional quality in this article implies that manufacturing sectors do not have attractive incentives that ensure continuous productivity growth in manufacturing sectors. The profitability of sectors is relatively lower compared to service sectors in Africa. Manufacturing productivity is positively associated with manufacturing output growth and employment growth. This runs counter to the economic route taken by industrialized countries, in which the reduction of agricultural share was replaced by a rise in the manufacturing sector’s part of the economy, followed by the development of the service sector (Shikur, Citation2020a, Citation2020b, Shikur etal., Citation2021). In Africa, however, the agricultural sector’s declining share of the economy has been replaced by a thriving low-productivity service sector, while the industrial sector has fallen. Thus, the negative relationship found in this paper between these variables and the manufacturing share of GDP would also reflect the development path taken by African countries, where the service sector has been booming while the manufacturing sector has been declining at a very low-level per capita income. Overall, the debates stress a lack of competitiveness, digitization of manufacturing activities, and African countries’ growth paths as plausible causes for the negative link between the manufacturing sector’s proportion of GDP and institutional quality.
The results of models showed that GDP per capita significantly increased African manufacturing development at the 1% level in the long-run and short run. According to the findings, increasing per capita GDP is accompanied by an increase in the manufacturing share of GDP in the short-run and long-run. The income per capita stimulates the manufacturing development by improving the competitiveness of the economy, as well as by increasing the demand for manufactured goods. On the contrary of this finding, Müller (Citation2021) finds that effects of GDP per capita are negative and positive for squared GDP per capita using panel regressions.
The findings also demonstrate that the size of the domestic market significantly stimulated African manufacturing development at the 1% level in the short run (). Domestic market size is a proxy variable for population that positively and significantly influence industrialization in the short-run. The population variable’s coefficient is statistically significant at 1% significance level, but the effect of population on structural transformation is negative in the long-run as unexpected. Large domestic markets generate scale economies that drive down production costs. A substantial demand in the future is also suggested by a sizable local market. The demand for manufactured goods should normally increase with per capita income, according to Engel’s law, which holds that as money increases, the need for manufactured goods should take the place of the need for food. In the long-run, labor growth at a constant rate impedes the shift of resources to more productive uses (efficiency vs. equity) resources (movement from the agricultural sector to manufacturing sectors) because labor growth adversely affects income per capita, which in turn reduces demand for manufacturing products (Shikur, Citation2020a). The country with a higher rate of population growth has a much lower steady-state than a country with a lower rate of population growth.
Economic openness plays a critical role in driving structural changes in African economies in the short run, but the effects are negative and significant in long run. In terms of trade openness, it is projected to have a positive impact on the manufacturing share of GDP by promoting technology transfer and access to foreign markets. The findings of this article show that industrialization in Africa is achievable by promoting trade openness strategy, but only in short run. Therefore, to increase demand in African countries, governments should take advantage of international and regional integrations to enhance the volumes of exports by providing export incentives for firms that engage in the production of goods for exports (Shikur, Citation2022a, Citation2022d); one possible incentive should be increment in the rate of exported goods. The findings highlight the need to strengthen regional integration, as this could be means to create a large domestic market and stimulate large volumes of sectors’ outputs by increasing incentives. In this study, countries with a higher degree of integration among countries, the economic growth increases significantly with export. Both conventional wisdom and empirical findings support the findings (i.e., suggesting that export is among key drivers of economic growth). For instance, significant economic growth has been achieved by promoting export-led growth policies in some Asian countries. The effect of export on agricultural growth is also significant and positive is consistent with the work of Shikur (Citation2022a). The study observes that FDI has a negative effect on structural transformation in the long run. In the short-run, FDI inflows have a significant role for manufacturing development, which does not correspond to the finding of Müller (Citation2021).
In this study, the finding showed that the abundance of natural resources has a negative impact on the manufacturing development which is in line with the findings of Horváth and Zeynalov (Citation2016), and Hartwell (Citation2016). The explanation for why natural resources has a detrimental impact on manufacturing development. First, natural resource richness may crowd out the manufacturing sector due to the so-called Dutch Disease. The natural resource endowment reduces manufacturing development by encouraging rent-seeking and corruption, lowering government effectiveness, and lowering the sector’s competitiveness. The effect of inflation on structural transformation is negative and significant in the long-run and short-run. African countries should avoid high inflation; this has the potential to contribute to the development of the manufacturing sector. Therefore, the government should maintain price stability through a variety of policy measures, including monetary, fiscal, exchange rate, supply-side, wage, and price restrictions. People and businesses can plan ahead and make more confident judgments when prices are stable. Finally, the government ought to increase its spending on infrastructure that generates employment. The governments can promote industrialization expansion as well as the creation of jobs by investing in public work projects.
5. Conclusions and policy implications
This paper analyzes the driving factors of manufacturing development in Africa by applying the panel ARDL technique over the period 1990–2021. The study examines the long and short-run impact of different explanatory variables on manufacturing development. The novelty of the study lies in applying panel ARDL models to measure the effect of economic freedom and institutional quality on industrialization for the first time. The results indicated that economic freedom and institutional quality significantly and positively impacted industrialization at the 1% level in the short-run whereas they significantly and negatively reduced industrialization at the 1% level in the long run. The findings showed that political instability, corruption control, government effectiveness, the rule of law, voice and accountability, and regulatory qualities have a positive and significant impact on the manufacturing share of GDP in the short-run whereas they reduced significantly manufacturing development in the long run. The findings from panel ARDL models provide conflicting perspectives on the long run and short run dynamic relationships between economic freedom and manufacturing share of GDP. African manufacturing transformation is significantly and positively determined by GDP per capita in the long and short run. The FDI inflows, and financial development have a significant and positive impact on the manufacturing share of GDP in the short run, but significant and negative effects in the long run. The level of inflation affects the growth of manufacturing negatively in Africa. The results of models showed that GDP per capita significantly and positively increased African manufacturing development at the 1% level in the long and short run.
Based on findings, this empirical study proposes some significant policies for low-middle income African countries to accelerate their manufacturing development in the short-run. First, policymakers should give attention to individual and whole impacts of institutional quality on industrialization, policymakers also ought to consider economic freedom cumulative implications on manufacturing growth. They should make appropriate improvements on each dimension of institutional quality to achieve the countries’ full potential for manufacturing growth. Governments are able to create and uphold a strong legal system that safeguards property rights and guarantees just contract enforcement. This gives certainty to investors and business owners, promotes them to invest in the country, leading to manufacturing productivity, and increased manufacturing employment and output. Third, governments should create a predictable and transparent regulatory framework that lowers corruption and administrative barriers to doing business. This can promote firms’ investment, growth, and development as well as make their function more effectively. Fourth, the government ought to raise institutional quality which is attainable through transparency, foundation of institutional quality enhancement, to eradicate corruption and thus industrialization. this investment can create job, increase economic activity, and accelerate manufacturing development. Fifth, there is a need to improve economic freedom by including range of policies: trade policy, the fiscal burden of government, government intervention in the economy, monetary policy, capital flows, and foreign investment, banking and finance, wages and prices, property rights, and regulation and black-market activity. For instance, this can be done by promoting openness, combating corruption, and laying the groundwork for future institutional quality improvements. Sixth, a significant amount of administrative work is needed to promote manufacturing growth and fight corruption. A low level of corruption and better government effectiveness will stimulate manufacturing development in the short, which will generate more jobs, and more income, in African countries. Seventh, the government ought to support economic openness, enhance communication between global markets, and foster debate and comprehension of the economic potential of other nations. Therefore, the government must enact a number of measures, including monetary, fiscal, exchange rate, supply-side, wage, and price restrictions, in order to preserve price stability. People and businesses can plan ahead and make more confident judgments when prices are stable. Finally, the government ought to increase its spending on infrastructure to increase employment. The governments can promote manufacturing expansion and growth as well as the creation of jobs by investing in public works projects.
Simultaneously, population policy should be employed to reduce the population growth rate, thereby increasing per capita income, per capita savings, per capita investment in technology and human capital to increase the steady state. The population variable’s coefficient is statistically significant at 1% significance level, but the effect of population on structural transformation is negative in long-run as unexpected. In long-run, labor growth at a constant rate impedes the shift of resources to more productive uses (efficiency vs. equity) resources (movement from the agricultural sector to manufacturing sectors) because labor growth adversely affects income per capital, which in turn reduces demand for manufacturing products.
The existence of a negative relationship between the manufacturing share of GDP and institutional quality in the long-run suggests that an improvement in institutional quality is not automatically accompanied by an increase in the manufacturing share of GDP in Africa. Therefore, government should ensure continuous and adequate incentives for industries with higher performance, invest in infrastructures to reduce costs of production and improve profitability in the long run. It may be concluded that governments’’ interventions may change/reverse adverse effect of institutional quality on manufacturing production growth. The relationship between the manufacturing share of GDP and economic freedom indicates that an increase in the manufacturing share of GDP does not necessarily equate to an improvement in economic freedom in Africa. One possible explanation for such a result is the low level of competitiveness of African economies in the manufacturing sector. Thus, the findings of this paper suggest that African governments should improve the competitiveness of their economies; otherwise, the recent growth recovery would not be beneficial for the development of the continent’s manufacturing sector. Skills and infrastructure development are some of the key areas that need attention for the improvement of Africa’s competitiveness in the manufacturing sector. The fact that the paper finds that financial development matters for manufacturing development, suggests that African countries should supply adequate money and improve financial infrastructures.
Disclosure statement
No potential conflict of interest was reported by the author(s).
Additional information
Funding
Notes on contributors
Zewdie Habte Shikur
Zewdie Habte Shikur is a senior lecturer and researcher at Wolaita Sodo University in Ethiopia. His primary research interests are in agricultural policies, transportation policies, agricultural market analysis, industrial policies, trade, climate, value chain analysis, transaction cost economics, economic growth, and financial develop.
References
- Ahrend, R. (2012). Understanding Russian regions’ economic performance during periods of decline and growth—An extreme bound analysis approach. Economic Systems, 36(3), 426–21.
- Alexeev, M., & Conrad, R. (2009). The elusive curse of oil. The review of economics and statistics, 91, 586–598. http://dx.doi.org/10.1162/rest.91.3.586
- Aron, J. (2000). Growth and Institutions: A Review of the Evidence. The World Bank research observer, 15, 99–135. http://dx.doi.org/10.1093/wbro/15.1.99
- Bhattacharjee, J., & S. K. (2015). Haldar Economic growth in South Asia: Binding constraints for the future. Journal of South Asian Development, 10(2), 230–249.
- Chenery, H. (1988). Introduction to part 2. Handbook of Development Economics, 197–202. https://doi.org/10.1016/s1573-4471(88)80022-6
- Diao, X., McMillan, M., & Rodrik, D. (2017). The recent growth boom in developing economies: A structural change perspective. NBER Working Paper N, 23132.
- Eichengreen, B., & Gupta, P. (2009). The two waves of service sector growth. NBER Working Paper N, 14968.
- Gwartney, J. D., Lawson, R. A., & Holcombe, R. G. (2015). Economic Freedom of the World: 2015 Annual Report.
- Haraguchi, N., Martorano, B., & Sanfilippo, M. (2018). What factors drive successful industrialization? evidence and implications for developing countries. Structural Change and Economic Dynamics, 49, 266–276. https://doi.org/10.1016/j.strueco.2018.11.00
- Hartwell, C. A. (2016). The institutional basis of efficiency in resource-rich countries. Economic Systems, 40(4), 519–538. https://doi.org/10.1016/j.ecosys.2016.02.004
- Horváth, R., & Zeynalov, A. (2016). Natural resources, manufacturing and institutions in post-soviet countries. Resources Policy, 50, 141–148. https://doi.org/10.1016/j.resourpol.2016.09.007
- International Monetary Fund (IMF). (2018). Manufacturing jobs: Implications for productivity and inequality, chapter 3 of the April world economic outlook.
- Jude, C., & Levieuge, G. (2013). Growth E ffect of FDI in Developing Economies: The Role of Institutional Quality. Available at SSRN 2409656.
- Khan, M., & Hanif, W. (2020). Hanif Institutional quality and the relationship between inflation and economic growth. Empirical economics, 58(2), 627–649.
- Mijiyawa, A. G. (2017). Drivers of structural transformation: The case of the manufacturing sector in Africa. World Development, 99(C), 141–159. https://doi.org/10.1016/j.worlddev.2017.07.007
- Müller, P. (2021). Impacts of inward FDIs and ICT penetration on the industrialisation of sub-saharan African countries. Structural Change and Economic Dynamics, 56, 265–279. https://doi.org/10.1016/j.strueco.2020.12.004
- Ouoba, Y., & Sawadogo, R. (2019). Natural Resources Effect on Economic Growth: The role of institutional quality. Journal of Policy Modeling. http://www.econmodels.com/upload7282/18468cfe824ca4067afca26e9d00b1c.pdf
- Pesaran, H., Shin, Y., & Smith, R. P. (1999). Pooled mean group estimation of dynamic heterogenous panels. Journal of the American Statistical Association, 94(446), 621–634. https://doi.org/10.1080/01621459.1999.10474156
- Pesaran, H., & Smith, R. (1995). Estimating long-run relationships from dynamic heterogenous panels. Journal of Econometrics, 68(1), 79–113. https://doi.org/10.1016/0304-4076(94)01644-F
- Rodrik, D. (2008). Industrial policy: Don’t ask why, ask how. Middle East Development Journal, 1(1), 1–28. https://doi.org/10.1142/S1793812009000024
- Rodrik, D. (2013). Unconditional convergence in manufacturing. Quarterly Journal of Economics, 128(1), 165–204. https://doi.org/10.1093/qje/qjs047
- Rodrik, D. (2016). Premature deindustrialization. Journal of Economic Growth, 21(1), 1–33. https://doi.org/10.1007/s10887-015-9122-3
- Rostow, W. W. (1960). The stages of economic growth: A non-communist manifesto. Cambridge University Press.
- Shikur, Z. H. (2020a). Agricultural policies, agricultural production and rural households’ welfare in Ethiopia. Journal of Economic Structures, 9(1), 50. Crossref, Google Scholar. https://doi.org/10.1186/s40008-020-00228-y
- Shikur, Z. H. (2020b). Industrial policy measure and economic structure in Ethiopia: The case of Oromia region. International Journal of Economic Policy Studies, 14(1), 255–274. Crossref], [Google Scholar. https://doi.org/10.1007/s42495-019-00031-z
- Shikur, Z. H. (2022a). Agriculture and commodity export and its productivity and production in sub-saharan Africa: Evidence from time series data of four countries. Regional and Sectoral Economic Studies, 22(1), 61–76. (RePEc: eaa:eerese:v:22:y2022:i:1_4). [Google Scholar].
- Shikur, Z. H. (2022c). Logistics performance, export, agricultural, manufacturing, and aggregate economic growth: A focus on sectoral perspectives. Journal of Economic Development, 47(3), 107–123. Google Scholar.
- Shikur, Z. H. (2022d). The role of logistics performance in international trade: A developing country perspective. World Review of Intermodal Transportation Research (WRITR), 11(1), 41–56. Google Scholar. https://doi.org/10.1504/WRITR.2022.123100
- Shikur, Z. H. (2022e). Transport policy, agricultural productivity and production in Ethiopia. Journal of Developing Areas, 56(3), 232–246. https://doi.org/10.1353/jda.2022.0052
- Shikur, Z. H. (2023). Credit market development and agricultural production in selected African countries: Climate change perspective. Region, 10(3), 1–18.
- Shikur, Z. H. (2024). Economic freedom, financial development and foreign direct investment inflows in African countries, 2007-2018. Applied Econometrics and International Development, 24(1).
- Singh, B. P., & Pradhan, K. C. (2020). Institutional quality and economic performance in South Asia. Journal of Public Affairs. https://doi.org/10.1002/pa.2401
- Szirmai, A. (2012). Industrialisation as an engine of growth in developing countries 1950–2005. Structural Change and Economic Dynamics, 23(4), 406–420. https://doi.org/10.1016/j.strueco.2011.01.005
- Uddin, I., Ahmad, M., Ismailov, D., Balbaa, M. E., Akhmedov, A., Khasanov, S., & Haq, M. U. (2023). Enhancing institutional quality to boost economic development in developing nations: New insights from CS-ARDL approach. Research in Globalization, 7, 100137. https://doi.org/10.1016/j.resglo.2023.100137
- Yuldashev, M., Khalikov, U., Nasriddinov, F., Ismailova, N., Kuldasheva, Z., & Ahmad, M. (2023). Impact of foreign direct investment on income inequality: Evidence from selected Asian economies. Public Library of Science ONE, 18(2), e0281870.