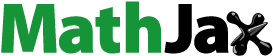
ABSTRACT
Commonly we see large within-age-group variations in physique, including body mass, stature, and percentages of predicted adult height, which suggests that age-specified training loads are flawed. Aims were to investigate how maturation impacts training load and neuromuscular response within academy soccer and to provide recommendations for practitioners. Fifty-five male soccer players (age 14.5 ± 1.2 years; stature 172 ± 10 cm; body mass 59.8 ± 10 kg; 94.1 ± 1.8% predicted adult height) reported differential ratings of perceived exertion (AU) across a season. Neuromuscular performance (countermovement jump, reactive strength index, absolute and relative leg stiffness) was measured at three time points across the season. Perceived exertion and neuromuscular performance were examined using linear mixed modelling, supplemented with non-clinical magnitude-based decisions. Analysis indicates every 5% increase in maturity status results in players perceiving overall session intensity 6.9 AU lower and 13.9 AU lower for a 10% maturity shift. Both 5% and 10% changes in maturity most likely resulted in higher countermovement jump, with likely to very likely differences observed for RSI and ABS. Maturity substantially influences neuromuscular performance over the season. Therefore, maturity-specific load prescription may prevent significant within age-group differences in accumulated load, possibly reducing injury risk and/or burnout.
Introduction
Although injury is multifactorial, it is now accepted that with adequate training load management, practitioners can mitigate injury risk to an extent (Impellizzeri et al., Citation2019; Williams et al., Citation2017). Obtaining the optimal dose-response balance is a complicated, multi-faceted but entirely possible priority within elite sport through proactive prescription and effective recovery suited to the individual needs to the athlete. This, often data-informed process, combines measures of internal and external load to advise coaching and support staff on micro-cycle development (Impellizzeri et al., Citation2019). This depth of data rarely afforded coaching and support staff working with adolescent athletes, who often have training loads from academy training superimposed onto other physical exertions from school and recreational settings (Phibbs et al., Citation2018).
The Elite Player Performance Plan (EPPP) provides recommendations on the quantity of coaching hours that players are exposed to, which systematically increases with age (Premier League, Citation2011). Therefore, it is the responsibility of each academy to navigate within these guidelines and strive for optimal training load balance for its players to achieve long-term success. Although there is some understanding of workloads in adolescent soccer (Arazi et al., Citation2020; Wrigley et al., Citation2012), there is a relative paucity of evidence compared with adult populations. This is likely caused by logistical and environmental complexities associated with collecting, interpreting and utilizing reliable data in these environments (Salter, De Ste Croix, Hughes et al., Citation2020), further complicated by the non-linear development through maturation.
Studies exploring adolescent workloads within soccer have primarily adopted a chronological age-group approach, whereby they observe differences or similarities between chronological age-groups (Arazi et al., Citation2020; Wrigley et al., Citation2012). Granted, this approach aligns with systematic increases in coaching hours and related exposure durations (Premier League, Citation2011), however it potentially oversimplifies the magnitude of individual variability associated with physical development. It is common to see large within-age-group variations in physical characteristics such as body mass (~50%), stature (~17%), percentages of predicted adult height (PAH: 10–15%) and fat free mass (~21%; Figueiredo et al., Citation2010), which is typically severe between the ages of 13–15 years. This period aligns with the rapid changes in joint stiffness, bone density and imbalances between strength and flexibility, which contributes to “skeletal fragility” (Ford et al., Citation2010; Van der Sluis et al., Citation2013). Rommers et al. (Citation2020) identified that U13-U15 players experienced a greater rate of growth per year (6.2 vs. 5 cm) and weight change (6.4 vs. 3.4 kg) than younger players (U10-U12), which contributed to more than double the frequency of overuse injuries (93 vs. 40) and almost three-fold increase in injury burden (14.6 vs. 4.7 days/season). These rapid changes in musculoskeletal structure (triggered by the onset of PHV) and apparent lag time to adequate relative strength are individually variable based on maturity timing and tempo, which likely corresponds to a variation in readiness to perform, ability to recover and by inference vulnerability to injuries (Dudink, Citation1994; Rommers et al., Citation2020). This infers that exposing all individuals of a similar chronological age to age-specified workloads is a flawed strategy and is reasonable to expect that early- and late-maturers will experience different dose-responses (Salter, De Ste Croix, Hughes et al., Citation2020). Evidence suggests that maturation (timing and tempo) also interacts with various components of physical performance including sprint speed (Meyers et al., Citation2017), match running performance (Buchheit & Mendez-Villanueva, Citation2014), strength and muscle architecture (Radnor et al., Citation2020). These interactions indicate variable maturity-related adaptations, and it is therefore unrealistic to expect all players within a given chronological age-group to cope with prescribed loads equally.
Age-related dose-responses may have small implications for acute between-session recovery, however over prolonged periods, this broad brush approach to load prescription may well contribute to the relatively high injury incidence observed across adolescent age groups (Read et al., Citation2018; Rommers et al., Citation2020). For example, academies generally divide the on-the-pitch coaching across 2–3 evenings per week plus matches, over 40-weeks of the year (Premier League, Citation2011). Mechanistically, this confines recovery time and emphasizes the need for appropriate load management to prevent non-functional overreaching (NFOR). NFOR is defined as a detrimented or stagnated performance (weeks to months) associated with chronic fatigue and has been reported in up to 27% of male academy players (Williams et al., Citation2017) with symptoms of athletic burnout also being common (25%; Hill, Citation2013). Additionally, up to 62% of Youth Development Phase (YDP) injuries described as non-contact (Read et al., Citation2018), which may support the notion that inappropriate workloads (i.e., NFOR) during this turbulent period of development contribute to the observed spike in injury incidence.
Sessional ratings of perceived exertion (sRPE) are a valid and common way to monitor the psycho-physiological perceptions of intensity within soccer (Fanchini et al., Citation2016; Salter, De Ste Croix, Hughes et al., Citation2020; Wrigley et al., Citation2012). This has been modified to discriminate specific psycho-physiological mediators (Wright et al., Citation2020) in the form of differential RPE (d-RPE). These differentiated ratings of perceived exertion may distinguish between physiological and mechanical load adaptation pathways and provide insight into the individual response (Vanrenterghem et al., Citation2017). In addition to self-reported indicators of load, markers of physical performance routinely collected (e.g., countermovement jump) offer valuable insight into how players are coping with training loads. Therefore, combining subjective and objective markers can offer a robust mechanism which helps to identify within-participant variations in load-adaptation over time.
The relative paucity of evidence surrounding the role of maturation and the responses to load provide an important rationale for this longitudinal study. It is anticipated that players of different maturity status will respond the training intensity differently (e.g., less mature players will have a heightened dose-response) and that their neuromuscular performance changes over the course of the season may be maturity-specific.
Materials and methods
Participants
Fifty-five male soccer players (age 14.5 ± 1.2 years; stature 172 ± 10 cm; body mass 59.8 ± 10 kg) from an EPPP academy participated in this study during the 2018–19 season. Typically, players were required to attend three scheduled 90-minute training sessions and one competitive match each week. To maintain data fidelity but maintain statistical power, participants were excluded if they failed to provide self-reported perception of intensity for >75% of training sessions, which may be a result of injury/illness, poor adoption, exams, travel constraints or de-selection from the academy. By utilizing a 75% threshold, data would be comprised of a minimum of 90 training sessions per participant which was deemed sufficient to conduct the planned statistical analysis whilst maximizing the sample pool. Due to the position specific nuances and the likely variations in load this study excluded goalkeepers. Ethical approval in line with the declaration of Helsinki was sought and granted from the University of Gloucestershire ethics committee.
Protocol
Maturity status was expressed as a current percentage of predicted adult height (PAH%) determined by measurement of somatic markers (stature and body mass) combined with self-reported parental stature adjusted for overestimation (Epstein et al., Citation1995; Khamis & Roche, Citation1994). PAH% is a non-invasive estimation of maturation commonly applied by academies and endorsed through the EPPP player management application (PMA), likely as a result of its simplicity and accessibility (Salter, De Ste Croix, Hughes et al., Citation2020). Parr et al. (Citation2020) conducted longitudinal analysis to observe timing of PHV, and illustrated that PAH% was accurate 96% of the time. Maturation tempo was expressed as the change in stature per year (cm/year) which was measured at three time points during the study, separated by approximately 3–4 months (Malina et al., Citation2004).
Self-reported perceptions of psycho-physiological intensity were collected approximately 15-minutes after each training session for a period of 40 weeks. Players used a touch-screen tablet (Acer Iconia One 8 B1-850, Taipei, Taiwan; Acer Inc) to record their perceptions of intensity using a customized application (McLaren et al., Citation2017). The application utilized the CR100® centi-Max scale with verbal anchors to provide confidential responses free from conformation bias (McLaren et al., Citation2017; Wright et al., Citation2020). Ratings of global (sRPE) and differential (RPE-B, breathlessness; RPE-L, leg muscle; RPE-T, technical/cognitive) perceptions of exertion were provided in arbitrary units (AU) for each training session. This load monitoring procedure was introduced approximately 1-year prior to the start of the current study, allowing significant opportunity for players to become habituated. Mean weekly RPE for each participant was calculated and utilized for analysis.
Neuromuscular (NM) performance was measured using countermovement jump (CMJ), reactive strength index (RSI), absolute (ABS) and relative leg (REL) stiffness at specific time points across the season coinciding with EPPP benchmark testing dates. Participants had opportunity to familiarize themselves with the protocol after a standardized 5-minute dynamic warm-up. After sufficient rest (3–5 mins) participants completed two attempts of each protocol using the Optojump photocell system (Microgate, Bolzano, Italy) with the best result taken for analysis. CMJ and RSI were calculated from five consecutive, maximal bilateral jumps. Participants started in an upright, standing position with hands on hips and then squatted to a self-selected depth and without pausing jumped maximally five times. Jump height (cm) was calculated from the first maximal jump using flight time from the following equation (Equationequation 1(1)
(1) ; De Ste Croix et al., Citation2015). Jump height and ground contact time were averaged across the five rebound maximal bilateral jumps to calculate RSI using the following equation (Equationequation 2
(2)
(2) ).
ABS and REL stiffness were measured from contact time and flight time during 20 consecutive bilateral sub-maximal hops at a frequency of 2.5 Hz. This speed was deemed to have the highest reliability of leg stiffness measured in adolescent populations (CV 7.2%; De Ste Croix et al., Citation2015). Participants were asked to place hands on their hips to minimize upper body interference; rebound for height and land within the photocell gates; landing with legs fully extended and looking forwards. ABS stiffness was calculated using Equationequation 3(3)
(3) where Kleg refers to leg stiffness, M is total body mass, Tc refers to ground contact time and Tf is equal to flight time (Lloyd et al., Citation2009). To account for the influence of mass on leg stiffness and leg length on mechanical properties of locomotion between participants, absolute values of leg stiffness were divided by body mass and leg length to provide a dimensionless value of relative leg stiffness (De Ste Croix et al., Citation2015).
Absolute Leg Stiffness
Data analysis
Following visual inspection of raw data distribution using Q-Q plots, differential-RPE (sRPE, RPE-B, RPE-L and RPE-T) and NM performance (CMJ, RSI, ABS and REL) across maturation (status and tempo) were examined using linear mixed modelling (SPSS Statistics v.25, Armonk, NY: IBM Corp). The model investigated differences between fixed effects (Squad; PAH% or cm/year) while using a random effect for player and PAH% or cm/year (intercept; unstructured) to account for repeated-within athlete observations for each dependent variable. To protect against increased risk of a type 1 error due to multiple comparisons, Bonferroni adjusted p-values were reported. Prior to analysis PAH% was mean centred to reflect variance of the intercepts specific to the sample mean and contextualize interpretations. PAH% was also scaled to allow more meaningful interpretations, whereby the model illustrates the estimates for a 5% and 10% deviation in PAH% from the mean, as these are typical ranges observed within chronological EPPP age-groups.
To facilitate practical applications, subsequent non-clinical magnitude-based decisions (MBD) were applied (Hopkins, Citation2019). Without empirical anchors for minimum practically important differences (MPID) in differential-RPE variables, a difference of 8 AU was used as this represents the shift required to move a players rating beyond halfway to, or from, the next effort category on the scale (CR100® centi-Max. Similarly, in the absence of recognized thresholds for all variables a consistent distribution-based approach (between-player SD multiplied by 0.20) to calculating MPID, was applied to NM performance data in the absence of empirical anchors for adolescent athletes. Probabilities of effects being substantial and effect sizes were reported using standardized thresholds (Hopkins, Citation2019). Uncertainty for all estimates was expressed using 95% confidence intervals (95% CI).
Results
In accordance with our data exclusion criteria (>75% response rate), 18 participants were removed prior to analysis, resulting in 37 participants generating data from 3,996 individual training sessions with >350,000 training minutes. Descriptive data for baseline within-age-group physical characteristics, NM performance and mean weekly differential-RPE ratings are presented in .
Table 1. Descriptive statistics of anthropometric and neuromuscular characteristics (mean ± SD) according to age category at the start of the season, and mean ± SD weekly differential-RPE (AU) values over the season.
On average, within age-groups, players consistently rated training sessions as “Strong” or “Heavy” across all differential-RPE domains (). Analysis indicates there was a most likely trivial impact of maturation tempo (cm/year) on all variables across the study (). There were very likely to most likely trivial differences in maturity status for perceptions of intensity for all domains except for sRPE. Analysis indicates that for sRPE, a 5% increase in maturity status (PAH%) results in players rating sessions 6.9 AU lower and 13.9 AU lower for a 10% maturity shift ().
Figure 1. Box (25th to 75th percentile) and whisker (minimum and maximum) ratings of each differential-RPE across chronological age-groups for the full season aligned to the verbal anchors of the Borg CR-100 centi-MAX scale.

Table 2. Slope, mean difference (95% confidence interval), effect size (d) and non-clinical practical inferences (probabilities) for 5% and 10% increments in PAH.
All aspects of NM performance were influenced by maturity status and identified likely to most likely differences, except for relative stiffness (unclear), but no such influence was observed for maturation tempo (). Both 5% and 10% changes in maturity status (PAH%) most likely resulted in higher CMJ, with likely to very likely differences observed for RSI and ABS. In line with probability distribution interpretations, REL data was unclear and therefore deemed not influenced by maturation (status or tempo).
Discussion
The aim of this single cohort study was to establish whether maturation (status and/or tempo) influenced perceived psycho-physiological response and NM performance response to typical training loads. Primary findings are a) overall session intensity (sRPE) was substantially influenced by maturity status, but not tempo; and b) maturity status (not tempo) substantially influences NM performance over the course of a season. Whilst the latter is less surprising, the former is novel and may provide practical insight for managing training load prescription in academy settings.
Mean pooled sRPE ratings were 49.2 AU which compares to previous studies involving players of similar age groups that have used either the CR-100 (Wright et al., Citation2020) or CR-10 scale which can be subsequently converted to facilitate CR-100 comparisons (Clemente et al., Citation2019). illustrates a progressive negative trend in mean sRPE values across age-groups, which is likely explained by an increased distribution of more biologically advanced players reporting lower sRPE values in older age-groups. Analysis indicates that a 5% increase in PAH%, resulted in a reduction of ~7AU per session, with a ~ 14AU difference for a 10% difference in PAH% (). On face value, these findings may appear trivial as within-session inter-individual differences of 7–14AU are common (McLaren, Citation2017). However, these values represent perceived intensity across the full season, comprising of approximately three sessions each week. Therefore, less mature players consistently perceive training more intense than more biologically advanced teammates of a similar age. When accrued, these small inter-individual differences may generate a substantial variation in training load over prolonged periods (e.g., week, month, term).
This is a noteworthy finding based on evidence suggesting chronological age-groups within this range are comprised of players varying in PAH% by 10–15% (Figueiredo et al., Citation2010) with injury incidence also peaking at the same time (Rommers et al., Citation2020). Although very much depicted as a “worst case scenario”, forecasts the potential annual self-reported load differences between players of different maturity status. The general consensus from training load research is that doing too much, or too little, is associated with increased injury risk (Kalkhoven et al., Citation2021). The omission of injury data in the current study prevents interpretations into which of the extremes depicted are more “at risk”, but it clearly illustrates that not all players are experiencing the same dose-response.
Figure 2. Forecasted accumulated training load differences in sRPE over a typical season for players of varied maturation.

No such substantial differences were observed when independent psycho-physiological mediators were observed using differential-RPE variables (). There is a paucity of research exploring the use of differential-RPE within adolescent soccer with only one study showing moderate to large (r. .59-.69) associations between sRPE and differential mediators (Wright et al., Citation2020). It is possible that this differentiated approach may have over-complicated the task for the adolescent population within current study. This population are considered to have the cognitive ability to understand and accurately rate sRPE, however the relationship between sRPE and the specific internal constructs (i.e. heart rate) is less pronounced at this age and somewhat influenced by the training mode (Groslambert & Mahon, Citation2006) which may confound differential-RPE sensitivity. Therefore, authors are satisfied that the substantial differences observed in sRPE across maturation in this academy, result in meaningful findings for the applied practitioner, who in most cases adopt sRPE rather than differential-RPE to monitor training loads in academy settings (Salter, De Ste Croix, Hughes et al., Citation2020; Wrigley et al., Citation2012).
A 5% increase in PAH% would likely improve CMJ performance by approximately 4.3 cm with similar relative magnitudes of performance improvement for RSI and ABS (). Inferences for REL were unclear, however this equation accounts for changes in body dimension by including mass and leg length, therefore this finding is not surprising. This progressive improvement in NM performance aligned to maturity status is not novel and has been highlighted in previous work (Lloyd et al., Citation2015) and is thought to result in the development of musculotendinous properties that enhance the stretch-shortening cycle and utilization of elastic energy (Radnor et al., Citation2020). Previous work had suggested that changes in leg stiffness were individualized (De Ste Croix et al., Citation2019), though the strength of relationship observed here would indicate this is more predictable than previously inferred. A notable result from the current study indicates that maturation tempo was not substantially associated with NM performance. There is a paucity of research that observes rate of growth and its interaction with performance characteristics, but findings here would indicate that maturity status may be a more useful marker than tempo to analyse performance progression. Admittedly, these are secondary findings from the current study and longitudinal work from diverse populations would be required to confirm this assertion.
Limitations
It is acknowledged that this study utilized an “estimation” of somatic maturity, rather than “gold-standard” measurements of skeletal age. If measured accurately the PAH% equation is reported to predict the adult stature to within 2.2 and 5.3 cm for the 50th and 90th percentile respectively (Malina et al., Citation2019). Objectively measuring parent stature was logistically difficult and therefore the equation often used self-reported parent stature and was therefore corrected for overestimation (Epstein et al., Citation1995). Although informative, it is accepted that the single cohort nature of this study comprised of data from one academy limits its wider application. To some degree, the relatively large number of training sessions and data fidelity obtained by removing players with limited exposure negate this, but maturation and load monitoring strategies vary between organizations. Additionally, including competitive match and injury data, recorded in a manner sensitive to overuse type injuries would help complete the picture outlined in this study. It is acknowledged that a large proportion of players (n = 18) were excluded from the final analysis due to participating in less than 75% of sessions. This has reduced the overall sample and power of the analyses, but the decision to complete a (>75%) case analysis was made a priori to protect against bias.
Conclusion
Findings illustrate that less mature individuals consistently perceive training to be substantially more intense than more mature peers. In the short term this should at least be a concern for practitioners involved with training prescription. However, over extended periods this has the potential to undermine the developmental pathway, as the assumption that players of a similar chronological age are experiencing similar load-responses is precarious. Failure to act, by adopting more mature sensitive ways of working for example, will result in a “survival of the fittest” environment, rather than the systematic, considered, and individualized approach to optimal loading proposed in policy documents and literature. It is this exact scenario that injury incidence, non-functional overreaching and burnout research has been critical of for several years and without adapting our practice will continue to impact practice for years to come. To do this, practitioners should monitor and utilize maturity status opposed to maturation tempo to inform training prescription.
Author contributions
JS was lead researcher and conducted the data collection and manuscript draft; RJ and SM assisted with the development of the statistical approach and edited interpretations/manuscript in line with this; AH was responsible for facilitating data collection and collating data; JH and MDSC were involved with research design, manuscript editing and practical applications. All authors have seen the complete manuscript and approved this for submission.
Acknowledgments
The authors would like to thank the staff and players at Hull City FC Academy for their support and assistance during the data collection of this research study, and Dr Shaun McLaren for his statistical insight.
Disclosure statement
No potential conflict of interest was reported by the author(s).
Additional information
Funding
References
- Arazi, H., Asadi, A., Khalkhali, F., Boullosa, D., Hackney, A. C., Granacher, U., & Zouhal, H. (2020). Association between the acute to chronic workload ratio and injury occurrence in young male team soccer players: A preliminary study. Frontiers in Physiology, 11, 11. https://doi.org/10.3389/fphys.2020.00608
- Buchheit, M., & Mendez-Villanueva, A. (2014). Effects of age, maturity and body dimensions on match running performance in highly trained under-15 soccer players. Journal of Sports Sciences, 32(13), 1271–1278. https://doi.org/10.1080/02640414.2014.884721
- Clemente, F. M., Rabbani, A., & Araújo, J. P. (2019). Ratings of perceived recovery and exertion in elite youth soccer players: Interchangeability of 10-point and 100-point scales. Physiology & Behavior, 210, 112641. https://doi.org/10.1016/j.physbeh.2019.112641
- De Ste Croix, M. B. A., Priestley, A. M., Lloyd, R. S., & Oliver, J. L. (2015). ACL injury risk in elite female youth soccer: Changes in neuromuscular control of the knee following soccer-specific fatigue: Female youth soccer and fatigue. Scandinavian Journal of Medicine & Science in Sports, 25(5), e531–e538. https://doi.org/10.1111/sms.12355
- De Ste Croix, M. B. A., Lehnert, M., Maixnerova, E., Zaatar, A., Svoboda, Z., Botek, M., Varekova, R., & Stastny, P. (2019). Does maturation influence neuromuscular performance and muscle damage after competitive match-play in youth male soccer players? European Journal of Sport Science, 19(8), 1130–1139. https://doi.org/10.1080/17461391.2019.1575913
- Dudink, A. (1994). Birth date and sporting success. Nature, 368(6472), 592. https://doi.org/10.1038/368592a0
- Epstein, L. H., Valoski, A. M., Kalarchian, M. A., & McCurley, J. (1995). Do children lose and maintain weight easier than adults: A comparison of child and parent weight changes from six months to ten years. Obesity Research, 3(5), 411–417. https://doi.org/10.1002/j.1550-8528.1995.tb00170.x
- Fanchini, M., Ferraresi, I., Modena, R., Schena, F., Coutts, A. J., & Impellizzeri, F. M. (2016). Use of the CR100 scale for session rating of perceived exertion in soccer and its interchangeability with the CR10. International Journal of Sports Physiology and Performance, 11(3), 388–392. https://doi.org/10.1123/ijspp.2015-0273
- Figueiredo, A. J., Silva, M. J. C. E., Cumming, S. P., & Malina, R. M. (2010). Size and maturity mismatch in youth soccer players 11- to 14-years-old. Pediatric Exercise Science, 22(4), 596–612. https://doi.org/10.1123/pes.22.4.596
- Ford, K., Myer, G., & Hewett, T. E. (2010). Longitudinal effects of maturation on lower extremity joint stiffness in adolescent athletes. The American Journal of Sports Medicine, 38(9), 1829–1837. https://doi.org/10.1177/0363546510367425
- Groslambert, A., & Mahon, A. D. (2006). Perceived exertion: Influence of age and cognitive development. Sports Medicine, 36(11), 911–928. https://doi.org/10.2165/00007256-200636110-00001
- Hill, A. (2013). Perfectionism and Burnout in junior soccer players: A test of the 2 × 2 model of dispositional perfectionism. Journal of Sport & Exercise Psychology, 35(1), 18–29. https://doi.org/10.1123/jsep.35.1.18
- Hopkins, W. (2019). Magnitude-based decisions. http://sportsci.org/2019/index.html
- Impellizzeri, F. M., Marcora, S. M., & Coutts, A. J. (2019). Internal and external training load: 15 years on. International Journal of Sports Physiology and Performance, 14(2), 270–273. https://doi.org/10.1123/ijspp.2018-0935
- Kalkhoven, J. T., Watsford, M. L., Coutts, A. J., Edwards, W. B., & Impellizzeri, F. M. (2021). Training load and injury: Causal pathways and future directions. Sports Medicine, 51(6), 1137–1150. https://doi.org/10.1007/s40279-020-01413-6
- Khamis, H. J., & Roche, A. F. (1994). Predicting adult stature without using skeletal age: The Khamis-Roche method. Pediatrics, 94(4), 504–507.
- Lloyd, R. S., Oliver, J. L., Hughes, M. G., & Williams, C. A. (2009). Reliability and validity of field-based measures of leg stiffness and reactive strength index in youths. Journal of Sports Sciences, 27(14), 1565–1573. https://doi.org/10.1080/02640410903311572
- Lloyd, R. S., Oliver, J. L., Radnor, J. M., Rhodes, B. C., Faigenbaum, A., & Myer, G. (2015). Relationships between functional movement screen scores, maturation and physical performance in young soccer players. Journal of Sports Sciences, 33(1), 11–19. https://doi.org/10.1080/02640414.2014.918642
- Malina, R. M., Bouchard, C., & Bar-Or, O. (2004). Growth, maturation, and physical activity. Human Kinetics.
- Malina, R. M., Cumming, S. P., Rogol, A. D., Coelho-e-Silva, M. J., Figueiredo, A. J., Konarski, J. M., & Kozieł, S. M. (2019). Bio-Banding in youth sports: Background, concept, and application. Sports Medicine, 49(11), 1671–1685. https://doi.org/10.1007/s40279-019-01166-x
- McLaren, S. J. (2017). The application of differential ratings of perceived exertion to training monitoring in team sports. Teesside University.
- McLaren, S. J., Smith, A., Spears, I. R., & Weston, M. (2017). A detailed quantification of differential ratings of perceived exertion during team-sport training. Journal of Science and Medicine in Sport, 20(3), 290–295. https://doi.org/10.1016/j.jsams.2016.06.011
- Meyers, R. W., Oliver, J. L., Hughes, M. G., Lloyd, R. S., & Cronin, J. B. (2017). Influence of age, maturity, and body size on the spatiotemporal determinants of maximal sprint speed in boys. Journal of Strength and Conditioning Research, 31(4), 1009–1016. https://doi.org/10.1519/JSC.0000000000001310
- Parr, J., Winwood, K., Hodson-Tole, E., Deconinck, F. J. A., Parry, L., Hill, J. P., Malina, R. M., & Cumming, S. P. (2020). Predicting the timing of the peak of the pubertal growth spurt in elite youth soccer players: Evaluation of methods. Annals of Human Biology, 47(ja), 1–23. https://doi.org/10.1080/03014460.2020.1782989
- Phibbs, P. J., Jones, B., Roe, G., Read, D., Darrall-Jones, J., Weakley, J., Rock, A., & Till, K. (2018). The organised chaos of English adolescent rugby union: Influence of weekly match frequency on the variability of match and training loads. European Journal of Sport Science, 18(3), 341–348. https://doi.org/10.1080/17461391.2017.1418026
- Premier League. (2011) . The Elite Player Performance Plan.
- Radnor, J. M., Oliver, J. L., Waugh, C. M., Myer, G., & Lloyd, R. S. (2020). The influence of maturity status on muscle architecture in school-aged boys. Pediatric Exercise Science, 32(2), 89–96. https://doi.org/10.1123/pes.2019-0201
- Read, P., Oliver, J. L., De Ste Croix, M. B. A., Myer, G., & Lloyd, R. S. (2018). An audit of injuries in six English professional soccer academies. Journal of Sports Sciences, 36(13), 1542–1548. https://doi.org/10.1080/02640414.2017.1402535
- Rommers, N., Rössler, R., Goossens, L., Vaeyens, R., Lenoir, M., Witvrouw, E., & D’Hondt, E. (2020). Risk of acute and overuse injuries in youth elite soccer players: Body size and growth matter. Journal of Science and Medicine in Sport, 23(3), 246–251. https://doi.org/10.1016/j.jsams.2019.10.001
- Salter, J., De Ste Croix, M. B. A., & Hughes, J. D. (2020). The moderating impact of maturation on acute neuromuscular and psycho-physiological responses to simulated soccer activity in academy soccer players. European Journal of Sport Science, In press, 17. 21(12), 1637–1647. https://doi.org/10.1080/17461391.2020.1851775
- Salter, J., De Ste Croix, M. B. A., Hughes, J. D., Weston, M., & Towlson, C. (2020). Monitoring practices of training load and biological maturity in UK soccer academies. International Journal of Sports Physiology and Performance, 16(1), 28. https://doi.org/10.1123/ijspp.2019-0683
- van der Sluis, A., Elferink-Gemser, M., Coelho-e-Silva, M., Nijboer, J., Brink, M., & Visscher, C. (2013). Sport injuries aligned to peak height velocity in talented pubertal soccer players. International Journal of Sports Medicine, 35(4), 351–355. https://doi.org/10.1055/s-0033-1349874
- Vanrenterghem, J., Nedergaard, N. J., Robinson, M. A., & Drust, B. (2017). Training load monitoring in team sports: A novel framework separating physiological and biomechanical load-adaptation pathways. Sports Medicine, 47(11), 2135–2142. https://doi.org/10.1007/s40279-017-0714-2
- Williams, C., Winsley, R. J., Pinho, G., De Ste Croix, M. B. A., Lloyd, R. S., & Oliver, J. L. (2017). Prevalence of non-functional overreaching in elite male and female youth academy football players. Science and Medicine in Football, 1(3), 222–228. https://doi.org/10.1080/24733938.2017.1336282
- Wright, M., Songane, F., Emmonds, S., Chesterton, P., Weston, M., & Mclaren, S. J. (2020). Differential ratings of perceived match and training exertion in girls’ soccer. International Journal of Sports Physiology and Performance, 1(aop), 1–9. https://doi.org/10.1123/ijspp.2019-0595
- Wrigley, R., Drust, B., Stratton, G., Scott, M., & Gregson, W. (2012). Quantification of the typical weekly in-season training load in elite junior soccer players. Journal of Sports Sciences, 30(15), 1573–1580. https://doi.org/10.1080/02640414.2012.709265