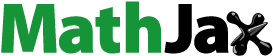
ABSTRACT
Designing efficient biomass energy systems requires a thorough understanding of the physicochemical, thermodynamic, and physical properties of biomass. One crucial parameter in assessing biomass energy potential is the higher heating value (HHV), which quantifies its energy content. Conventionally, HHV is determined through bomb calorimetry, but this method is limited by factors such as time, accessibility, and cost. To overcome these limitations, researchers have proposed a diverse range of empirical correlations and machine-learning approaches to predict the HHV of biomass based on proximate and ultimate analysis results. The novelty of this research is to explore the universal applicability of the developed empirical correlations for predicting the Higher Heating Value (HHV) of biomass. To identify the best empirical correlations, nearly 400 different biomass feedstocks were comprehensively tested with 45 different empirical correlations developed to use ultimate analysis (21 different empirical correlations), proximate analysis (16 different empirical correlations) and combined ultimate-proximate analysis (8 different empirical correlations) data of these biomass feedstocks. A quantitative and statistical analysis was conducted to assess the performance of these empirical correlations and their applicability to diverse biomass types. The results demonstrated that the empirical correlations utilizing ultimate analysis data provided more accurate predictions of HHV compared to those based on proximate analysis or combined data. Two specific empirical correlations including coefficients for each element (C, H, N) and their interactions (C*H) demonstrate the best HHV prediction with the lowest MAE (~0.49), RMSE (~0.64), and MAPE (~2.70%). Furthermore, some other empirical correlations with carbon content being the major determinant also provide good HHV prediction from a statistical point of view; MAE (~0.5–0.8), RMSE (~0.6–0.9), and MAPE (~2.8–3.8%).
Introduction
Recent decades have seen a worldwide energy and climate crisis (Erdogan and Canbazoglu Citation0000) mainly caused by the fast depletion of fossil fuel reserves and political conflicts between countries. To mitigate both crises, many countries have attempted to handle this issue by promoting the use of biomass as a source of energy production (Güleç et al. Citation2022). Biomass/bioenergy has the potential to replace fossil fuels in various applications as a sustainable and renewable energy source (Rahib et al. Citation2021). In biomass processes, organic compounds in biomass can be utilized/valorized through various processes including combustion, gasification, and pyrolysis to produce bioenergy (Aziz et al. Citation2024). Biomass has, therefore, gained substantial attention owing to its lower carbon footprint and availability in recent years (Wei, Cheng, and Shen Citation2024). The physicochemical, thermodynamic, and physical properties of biomass resources play a crucial role in the design of energy systems (Ma et al. Citation2024). A significant parameter in determining the value of biomass is the higher heating value (HHV) (Nhuchhen and Afzal Citation2017; Nhuchhen and Salam Citation2012). HHV of a biomass can be determined by a bomb calorimeter, which is an experimental method, but this method has limitations in terms of time, accessibility, and cost (Güleç, Şimşek, and Tanıker Sarı Citation2022). Moreover, the small sample size typically used in bomb calorimetry (usually 1.0 g) may not adequately represent bulky feedstocks. To obtain a homogeneous HHV value, a significantly larger number of experiments would be required, posing practical challenges. These limitations have therefore led to developing the prediction methods using the physicochemical characteristics of biomass feedstocks (Li et al. Citation2024).
In addition to HHV, the bioenergy potential of biomass can be assessed using different characterization methods. The elemental composition of biomass is analyzed by the ultimate analysis (UA) (Ozyuguran, Akturk, and Yaman Citation2018), which determines the composition of carbon (C), hydrogen (H), nitrogen (N), oxygen (O), and sulfur (S) (Lyons, Lunny, and Pollock Citation1985). Once C and H are oxidized, an exothermic reaction occurs, generating CO2 and H2O (Obernberger, Brunner, and Bärnthaler Citation2006). C and H have a positive impact on HHV since C is an essential component of solid biomass and H plays an important role in combustion (Demirbas Citation2002). It is seen that the concentration of N in biomass is an important parameter if the environmental effect of NOx is considered (Golgiyaz et al. Citation2022; Telmo, Lousada, and Moreira Citation2010). Proximate analysis (PA) reveals the contents of biomass such as volatile matter (VM), fixed carbon (FC), and ash content (Nunes, Matias, and Catalao Citation2017). The percentages of these contents have effects on the combustion of biomass. Unless nonvolatile matter is formed from noncombustible gases such as CO2 and H2O (Özyuğuran and Yaman Citation2017), the HHV increases with FC and VM (Vargas-Moreno et al. Citation2012). Biomass comprises a different amount of cellulose, hemicellulose, and lignin (Zhang, Xu, and Champagne Citation2010) in addition to lipids, proteins, simple sugars, and starches albeit in various quantities. Detecting the components through structural analysis not only helps in predicting HHV but also plays a vital role in the production of derivative fuels and chemicals, as well as in analyzing combustion (Saidur et al. Citation2011).
The HHV value of biomass can be estimated using either PA or UA alone or together. Based on only UA analysis, several studies have been conducted to estimate the HHV of biomass (Huang and Lo Citation2020; Qian et al. Citation2021). Empirical equations have demonstrated how the rate of C, H, N, O, and S in biomass elementally affect HHV value, depending on the type of biomass. The major determinant of HHV in all empirical correlations based on UA is C content of biomass. A few empirical correlations consider only C (Channiwala and Parikh Citation2002) as the variable predicting HHV, while others take into account C, H, O, and N together (Demirbas et al. Citation1997). In addition to elemental composition, some studies have been conducted to predict the HHV level of biomass utilizing the results of the PA (Dashti et al. Citation2019). The empirical equations obtained with PA include one (Kathiravale et al. Citation2003), two (Callejón-Ferre et al. Citation2011), or all (Ahmaruzzaman Citation2008) of the proximate analysis results of FC, VM, and ash with various correlations. In addition to estimating HHV using the UA and PA analyses separately, there are also studies in which the estimation of HHV is carried out by using these two analyses together (Nhuchhen and Afzal Citation2017). It is the rapidly developing field of machine learning that provides a variety of approaches for optimizing biomass HHV predictions. Artificial neural networks decision trees, random forests, support vector machines, and deep learning are among these approaches. However, the limitations of machine learning are its inability to design proper models due to its lack of interpretability, the risk of overfitting or underfitting the data, the challenge of determining the optimal number of training epochs, the need for high-quality and diverse training data, systematic errors and outliers in the data, and the need for curated datasets that are accurate and accessible to machine learning (Dobbelaere et al. Citation2021). Despite the existence of a wide range of empirical correlations developed to predict the HHV of different types of fuels, there is a limitation on their applicability to a wide range of biomass feedstocks. Moreover, the existing literature does not sufficiently show a comprehensive understanding of how parameters derived from both ultimate and proximate analyses impact HHV prediction. Consequently, there is a need for a thorough investigation that explores the universal applicability and reliability of these empirical correlations across an extensive array of biomass feedstocks.
This study provides a comprehensive understanding of the generalizability of the developed empirical correlations to predict the HHV for two different type of biomass feedstocks. The groundbreaking aspect of this research resides in investigating the diverse application possibility of an empirical correlation for forecasting biomass HHV. This research focuses on three main objectives: (i) discerning the paramount empirical correlation for forecasting the HHV of biomass, (ii) probing the applicability of these correlations across varied biomass feedstocks, and (iii) isolating the superior empirical correlation that can uniformly predict the HHV across these two groups of biomass feedstocks. This research covers the comparative quantitative and statistical analysis of 45 different HHV prediction equations using approximately 400 different biomass feedstocks categorized as woods (category-1), herbaceous and agricultural biomasses (category-2) using their characteristics of PA and UA. A quantitative and statistical analysis is conducted to assess the performance of these empirical correlations and their applicability to diverse biomass types. The main objective of this work is to present this analysis more clearly and concisely, emphasizing its significance in advancing our understanding of biomass HHV prediction.
Materials and methods
Dataset collection and analysis
As a data set, 391 different biomass feedstock data of proximate analysis, ultimate analysis, and experimental HHV were collected from the literature (Appendix A, Table A1) and used to investigate the generalizability of 45 different empirical correlations developed for the predicting of HHV. The data set consists of two main biomass feedstocks; 1st category is woody biomass consisting of soft and hardwoods with 137 different feedstocks, 2nd category is herbaceous and agricultural biomass feedstocks with 254 different feedstocks. The biomass feedstocks are categorized as presented in . Additionally, the data distribution of proximate analysis, ultimate analysis, and HHV for the woody biomass data and herbaceous-agricultural biomass data are presented in .
Figure 1. Data distributions of a) woody biomass feedstocks (137 data) and b) herbaceous and agricultural biomass feedstocks (254 data) with proximate analysis, ultimate analysis, and experimental HHV. “db” represents dry basis.
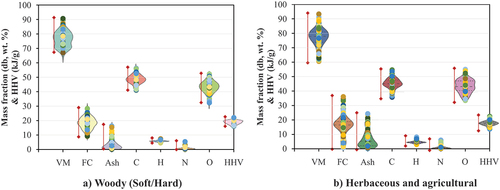
Table 1. Biomass types and categorized collected for the generalizability of the developed equations to predict the HHV.
In this research, we distinguished between “woody biomass” and “herbaceous and agricultural biomass” based on their botanical characteristics and typical usage patterns. Woody biomass, comprising softwood and hardwood, is primarily derived from trees and forests. Although it can be considered a part of agricultural biomass in a broader sense, for the purposes of our study, it is categorized it separately due to its distinct physical and chemical properties, which are notably different from those of herbaceous and agricultural biomass. The latter category is typically associated with non-wood plants, including grasses, crops, and residues from agricultural activities. This distinction is crucial for our HHV prediction model as these two categories exhibit different combustion and energy properties.
Empirical correlations for the HHV prediction
In order to identify the best empirical correlations, about 400 different biomass feedstocks were comprehensively tested with 45 different empirical correlations developed to use ultimate analysis (21 different empirical correlations, ), combined ultimate-proximate analysis (8 different empirical correlations ) and proximate analysis (16 different empirical correlations ) data of these biomass feedstocks.
Table 2. Empirical correlations for the prediction of biomass HHV using UA data.
Table 3. Empirical correlations for the prediction of biomass HHV using combined PA and UA data.
Table 4. Empirical correlations for the prediction of biomass HHV using PA data.
Statistical analysis of the empirical models
To assess the performance of developed empirical correlations and their applicability to diverse biomass types (specially the defined two categories), a quantitative and statistical analysis was conducted using the statistical methods including mean absolute error (MAE), mean absolute percentage error (MAPE), correlation coefficient (R2), and root mean square error (RMSE). The statistical analysis of the results obtained from 45 distinct models holds great significance and shows the suitability of these models in the field. MAE, EquationEquation (1)(46)
(46) , is a widely used standard statistical method to obtain model performance (Chai and Draxler Citation2014). It can be summarized the average value of sum of absolute errors (Boztepe et al. Citation2021). It is used to show the distance of the predicted data from the true value. RMSE, EquationEquation (2)
(47)
(47) , is the most widely used statistical method to analyze the prediction performance (Chai and Draxler Citation2014). The RMSE specifies the square root of the mean of the squares of the differences between the predicted and actual values. Furthermore, the MAPE as defined by EquationEquation (3)
(48)
(48) and the correlation coefficient (R2) were also employed (Boztepe, Daskin, and Erdogan Citation2022).
Here, is the mean value of the sample, z is the value of the confidence level, s is the standard deviation of the sample, n is the sample size, and i is the sample index. yi and xi are the predicted and measured HHVs for the ith sample.
Results and discussions
Analysis with a mixed biomass feedstock
presents the HHV prediction results of mixed biomass feedstocks using the empirical correlations based on ultimate analysis data (Eqs. (1)-(21)). summarize the results of the statistical analysis aimed at assessing the performance of the developed empirical correlations for HHV prediction of biomass feedstocks. To derive these values, the MAE, RMSE, MAPE, and R2 were applied as primary statistical tools. Each of these statistical methods offers a different perspective on the accuracy and reliability of our predictive models:
Figure 2. The HHV prediction results of mixed biomass feedstocks (391 data in total) using the empirical correlations based on ultimate analysis data (eqs. (1)-(21)).
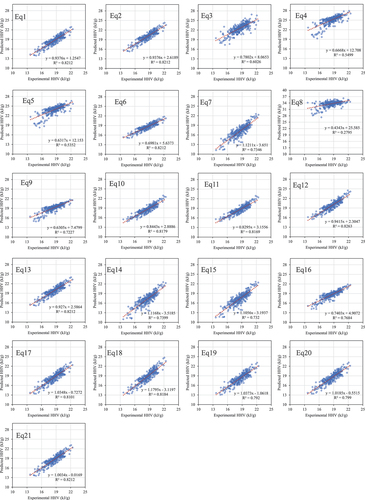
MAE (EquationEquation (46)
(46)
(46) ): This was calculated as the average of the absolute differences between the predicted and measured HHVs. It provides a straightforward measure of prediction accuracy without considering the direction of errors.
RMSE (EquationEquation (47)
(47)
(47) ): This metric was computed as the square root of the average of the squared differences between the predicted and actual HHVs. RMSE is sensitive to larger errors, making it a valuable tool for understanding the variability in prediction performance.
MAPE (EquationEquation (48)
(48)
(48) ): We calculated MAPE as the average of the absolute percentage errors. This metric is particularly useful in contexts where it is important to understand the error relative to the magnitude of the values being predicted.
Correlation Coefficient (R2): This statistical measure was used to determine the strength of the linear relationship between the predicted and actual HHVs.
Table 5. Statistical analysis for the prediction of HHV of mixed biomass feedstocks using empirical correlations (eqs. (1)-(21)) based on ultimate analysis.
Table 6. Statistical analysis for the prediction of HHV of mixed biomass feedstocks using empirical correlations (eqs. (22)-(29)) based on both proximate and ultimate analyses.
Table 7. Statistical analysis for the prediction of HHV of mixed biomass feedstocks using empirical correlations (eqs. (30)-(45)) based on proximate analysis.
Table 8. Statistical analysis of the best two empirical correlations for the prediction of HHV of herbaceous and agricultural biomass feedstocks (254 data set) and woody biomass feedstocks (137 data set) using either ultimate analysis or combined ultimate-proximate analyses data.
The data for these calculations were derived from our extensive dataset of biomass feedstocks. For each model, it was applied the aforementioned statistical methods to the entire dataset to evaluate the model’s performance across different types of biomass. This comprehensive approach ensured that the results reflected the general applicability of the models to diverse biomass types. The values presented in are the culmination of these calculations, reflecting a thorough statistical analysis of the model’s performance. This methodology not only demonstrates the robustness of our approach but also provides a clear and quantifiable way to compare different models.
Among these 21 different empirical correlations (provided in ), Eq. (10) and Equation (11) demonstrate the lowest MAE (~0.49), RMSE (~0.64), and MAPE (~2.70%) (). Both these empirical correlations include coefficients for each element (C, H, N) and their interactions (CH) that potentially reflect the energy of the corresponding chemical bonds in the biomass. The empirical correlation Eq. (10) includes a power function, which reflects the non-linear relationship between the HHV and the elemental composition of the biomass. Since the non-linearity may limit the accuracy of the equation for some types of biomasses, especially those with unusual chemical compositions. Equation (11) exhibits distinct leading coefficients for each element (C, H, N, and C*H) as well as constants within the empirical correlations. Similarly in this study, Eq. (10) has been tested and validated for various types of biomass, including agricultural residues, energy crops, woody biomass, and biochar (torrefied) and has shown reasonable accuracy in predicting their HHV (Friedl et al. Citation2005; Nhuchhen and Afzal Citation2017).
In addition to these two equations, eight different empirical correlations (Eq. (1), Equation (6), Eqs. (16)-(21)) also show relatively low MAE (~0.5–0.8), RMSE (~0.6–0.9), and MAPE (~2.8–3.8%) (). Unlike the best two empirical correlations (Eqs. (10) and (11)), these eight empirical correlations (Eq. (1), Equation (6), Eqs. (16)-(21)) focus on the content of C and H, not N in the biomass. Considering the low N content of biomass resources, the impact of nitrogen in the HHV was evaluated much lower compared to C and H. On the other hand, the empirical correlations include the oxygen (O) content in addition to C, H, and N, which shows higher error for the prediction of biomass HHV. The ultimate analysis of HHV prediction correlations i.e., Eq. (10) and Equation (11), presented in can be a useful tool for predicting the HHV of wood, herbaceous, and agricultural solely on their elemental composition. In addition to ultimate analysis, the predictions with Eq. (1) and Equation (6) appear to be reasonably accurate HHV predictions for wood, herbaceous, and agricultural biomasses using only carbon (C) content.
presents the HHV prediction results of mixed biomass feedstocks using the empirical correlations based on combined ultimate and proximate analyses data (Eqs. (22)-(29)). also shows the statistical error analysis for the prediction of HHV of mixed biomass feedstocks using combined ultimate-proximate analyses based on empirical correlations. Among these 8 different empirical correlations (provided in ), Eq. (22) is a simple linear regression model that includes variables for C, H, and Ash. It does not include N or VM content. Furthermore, Eqs. (23)-(25) include terms for C, H, Ash, and/or N content, as well as additional terms such as VM or Ash squared. These equations demonstrate higher errors; MAE (~0.81–1.82), RMSE (~0.99–2.17), and MAPE (~4.3–9.7%), in the prediction of HHV. The complexity of these equations may be more prone to overfitting and not generalize as well to new data. On the other hand, Eqs. (26)-(29) include terms for multiple variables, such as Ash, C, H, N, and/or VM content, and appear to be more complex than Equation (22) but less complex than Eqs. (23)-(25). These equations show a good balance between complexity and generalizability and provide better HHV prediction with lower errors. Eq. (26) shows the best prediction with the lowest MAE (~0.65), RMSE (~0.83), and MAPE (~3.5%). However, the correlation developed for the combined ultimate-proximate analyses demonstrated higher errors () for the prediction of HHV compared to the eight different empirical correlations developed for ultimate analysis data sets ().
Figure 3. The HHV prediction results of mixed biomass feedstocks (391 data in total) using the empirical correlations based on combined proximate-ultimate analyses data (eqs. (22)-(29)).

shows the experimental and predicted HHV of mixed biomass feedstocks using the empirical correlations developed for proximate analyses data (Eqs. (30)-(45)). The statistical errors are shown in for the prediction of HHV of mixed biomass feedstocks using proximate analyses based on empirical correlations. Among these 16 empirical correlations, none of them shows better prediction (lower errors) compared to the promising empirical correlations developed for ultimate analysis and combined ultimate analysis. The lowest errors (MAE ~0.96, RMSE ~ 1.27, and MAPE ~ 5.27%) were observed with Eq. (32) (), while these errors are much higher than those of many other correlations developed for ultimate analysis () and combined ultimate-proximate analyses (). Although these empirical show better predictions in (Sheng and Azevedo Citation2005) and (Callejón-Ferre et al. Citation2011), they are not well-suited to the specific data being used in this study and do not capture the full range of variability in the data.
Figure 4. The best four empirical correlations based on average confidence intervals to predict the HHV of mixed biomass feedstocks using proximate analysis data; a)Eq. (32), b) equation (33), c) equation (42), d)Equation (45).
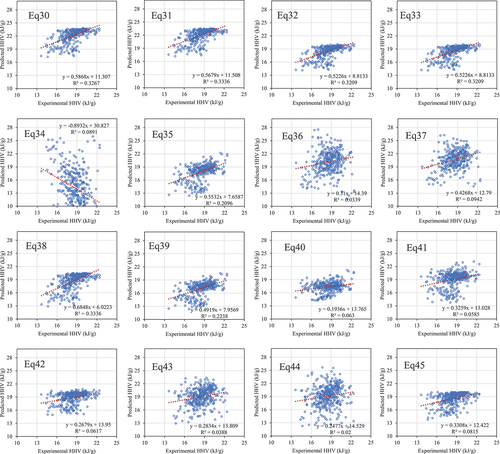
Considering these 45 different empirical correlations, the empirical correlations based on ultimate analysis and combined ultimate-proximate analysis can be useful tools for predicting the HHV of woody, herbaceous and agricultural biomass feedstocks. However, the predictive accuracy may be limited for some other types of biomasses with unusual chemical compositions. Therefore, the next section “3.2 Analysis with categorized biomass feedstocks” will provide the impact of these two groups on different types of biomass feedstocks with the herbaceous and agricultural biomass feedstocks (254 data set) and woody biomass feedstocks (137 data set).
Analysis with categorised biomass feedstocks
In this section dataset was divided into two biomass groups; herbaceous and agricultural biomass feedstocks and woody biomass feedstocks to evaluate the performance of the empirical correlations. Since it is significantly important to understand whether the empirical correlations are good for some specific type of biomass feedstocks or generalizable for any type of biomass feedstocks. and show the best two empirical correlation results for the prediction of HHV of herbaceous and agricultural biomass feedstocks and woody biomass feedstocks using ultimate analysis data and combined ultimate-proximate analyses data, respectively. The HHV prediction results with the correlation developed for only proximate analysis show relatively low prediction as in the previous section () and the results are presented in the Appendix B. In addition, and 9 presented the statistical analysis results (MAE, MAPE, STDEV, RMSE, and R2) of the best empirical correlations for predicting the HHV of herbaceous and agricultural biomass feedstocks and woody biomass feedstocks with the correlations developed by either ultimate analysis or combination of proximate and ultimate analysis.
Figure 5. The best two empirical correlations for the prediction of HHV of herbaceous and agricultural biomass feedstocks (254 data set) using ultimate analysis data a) equation (10) and b) equation (11) and combined ultimate-proximate analyses data; c) equation (26) and d) equation (29).

Figure 6. The best two empirical correlations for the prediction of HHV of woody biomass feedstocks (137 data set) using ultimate analysis data a) equation (10) and b) equation (11), and combine ultimate-proximate analyses data; c) equation (26) and d) equation (29).

The characteristics of these two biomass types (herbaceous and agricultural biomass feedstocks and woody biomass feedstock) show some similarities in terms of ultimate analysis and proximate analysis characteristic distribution (). That’s why the results seem to be close to each other. However, herbaceous and agricultural biomass feedstocks biomass have slightly wider characteristic ranges in terms of contents (C, H, O, N, VM, FC, Ash) compared to woody biomass feedstock. Therefore, there is a slight difference in terms of statistical results since woody biomass feedstocks show slightly better performance in terms of prediction using the same empirical correlations.
Both biomass feedstocks (herbaceous and agricultural, woody) demonstrate that the effect of C content is of the utmost importance in the prediction of HHV. Both biomass feedstocks groups provide the same empirical correlations (Eq. (10), Equation (11), Eq. (26), and Equation (29)) as the best candidates for the predicting of HHV. The only differences are from a statistical point of view, i.e., MAE was determined to be approximately 0.5 for Herbaceous and Agricultural biomass feedstocks and approximately 0.46–0.47 for Woody biomass feedstocks using Eqs. (10) and (11).
Starting with the best two empirical correlations based on ultimate analysis, Eqs. (10) and (11) have very similar performance across all metrics for both herbaceous and agricultural biomass feedstocks and woody biomass feedstocks. shows that Eq. (10) and Equation (11) could potentially be the most reliable correlations for predicting HHV based on ultimate analysis data, with the lowest error and highest accuracy. On the other hand, Eq. (26) and Equation (29) which are obtained from combined proximate analysis, also show good performance for both biomass types with higher error rates compared to Eqs. (10) and (11).
It is possible to achieve better results by using ultimate analysis-based empirical correlations for both biomass feedstocks. Considering the close error rates and the order of the equations that did not change in both groups, the four mentioned equations are applicable to the process of obtaining the HHV. Overall, only ultimate analysis data is sufficient to obtain the best HHV prediction process, even if the dataset includes both proximate and ultimate analysis results.
Sensitivity analysis
Based on the prediction results for Eqs. 10 and 11, a single variable sensitivity plot was presented to evaluate the relationships between all the independent variables and predicted HHV. The plots can be found in the supplementary materials (Appendix C, Figure C1 and C2). It should be noted that the single variable plots display similar trends across all equations. Furthermore, the C, FC, VM and N contents of biomass shows progressive increase with HHV. A slight increase in C content of biomass from 14 wt.% to 24 wt.% led to an elevation in the HHV from 36 kJ/g to 56 kJ/g. Also, as the FC content of biomass rises from 17 wt.% to 24 wt.% the HHV values also increase up to a value of 40 kJ/g. Other parameters such as ASH and H content did not display a visible trend with HHV values while the O content displays a negative trend. HHV values increase with a decline in O contents. It should be mentioned that the HHV value increases with C content due to the role of carbon in combustion processes (Nhuchhen and Afzal Citation2017). Carbon atoms react with oxygen to form carbon dioxide, releasing significant amount of energy. The higher the carbon content in the biomass, the more carbon atoms are available to undergo this reaction, thus generating more heat (Maksimuk et al. Citation2021). Additionally, carbon-rich biomass tends to have lower moisture and oxygen content, which further enhances its combustibility and energy yield. On the contrary, biomass with lower carbon content often has higher proportions of noncombustible elements like oxygen and nitrogen, leading to lower energy release during combustion.
Conclusions
This study aims to investigate the generalizability of empirical correlations for predicting the HHV of biomass, which is an essential parameter for assessing biomass energy potential. The study comprehensively analyzed 391 different biomass feedstocks, including wood, herbaceous, and agricultural materials, using 45 different empirical correlations. The goal was to identify the best empirical correlations for predicting HHV and assess their performance across diverse biomass types. For this reason, some statistics analysis has been applied using Matlab to real and estimated data to determine the best equation/equations for predicting the HHV of different biomass types.
The results indicated that
Empirical correlations utilizing ultimate analysis data provided more accurate predictions of HHV (lowest MAE:0.49 and MAPE:2.70% - Equation-10) compared to those based on proximate analysis (lowest MAE:0.96 and MAPE:5.27% - Equation-32) or combined data (lowest MAE:0.65 and MAPE:3.55% - Equation-26).
Two empirical correlations (Eqs.. (10) and (11)), which consider coefficients for each element (C, H, N) and their interactions (C*H), showed the best HHV prediction with the lowest mean absolute error (MAE) of approximately 0.49, root mean square error (RMSE) of around 0.64, and mean absolute percentage error (MAPE) of approximately 2.70%.
Other empirical correlations that primarily relied on carbon content as the major determinant also provided good HHV prediction from a statistical perspective, with MAE ranging from approximately 0.5 to 0.8, RMSE ranging from around 0.6 to 0.9, and MAPE ranging from approximately 2.8% to 3.8%.
The advantages of these two empirical correlations are offering high accuracy in predicting HHVs for specific biomass types using their ultimate analysis results (C, H, N), as evidenced by their low MAE, RMSE, and MAPE. They are straightforward to use, requiring only the elemental composition for calculation, making them suitable for quick assessments to predict the HHV in practical applications. However, these models may have limited generalizability beyond the specific types of biomasses tested in this study. Their accuracy heavily depends on precise elemental analysis, and they might oversimplify the complex processes governing HHV. There’s also a risk of overfitting to the dataset they were developed from, potentially reducing their applicability to other biomass types or conditions.
Future research endeavors exploring the HHV of various biomass feedstocks should notably focus on the expansion of datasets to improve the robustness and applicability of empirical correlations across a wide array of biomass types. A pivot toward developing novel empirical correlations specifically tailored for unique and outlier biomass types will enhance predictive precision, especially when synthesized with advanced machine learning and deep learning models. This hybrid approach, intertwining empirical and computational methodologies, promises to bolster prediction accuracy by seamlessly navigating through complex, multidimensional biomass characteristic spaces. Lastly, an in-depth study into technological and combustion dynamics will facilitate a practical transition, ensuring that theoretical HHV predictions effectively translate into optimized combustion and sustainable energy generation in real-world contexts. Consequently, these focused strands of exploration stand to fortify the interlink between theoretical predictability and pragmatic energy production efficacy in bioenergy research.
CRediT authorship contribution statement
Mahmut Daskin: Formal analysis, Validation, Funding, Project administration, Visualization, Investigation, Writing – original draft, Writing – review & editing. Ahmet Erdoğan: Project administration, Formal analysis, Visualization, Investigation, Writing – original draft, Writing – review & editing. Fatih Güleç: Data collection, Project administration, Validation, Funding, Formal analysis, Visualization, Investigation, Writing – original draft, Writing – review & editing. Jude A. Okolie: Validation, Formal analysis, Visualization, Writing – review & editing.
Supplemental Material
Download MS Word (539.8 KB)Disclosure statement
No potential conflict of interest was reported by the author(s).
Supplementary material
Supplemental data for this article can be accessed online at https://doi.org/10.1080/15567036.2024.2332472
Additional information
Funding
Notes on contributors
Mahmut Daskin
Dr Mahmut Daskin is a visiting academic at Cranfield University and an Assistant Professor in the Department of Mechanical Engineering at İnönü University. He obtained his Ph.D. in Mechanical Engineering from İnönü University in 2019. His areas of interest include Control Theory, Fuels and Combustion, System Modelling, CO2 Capture, Hydrogen Detection.
Ahmet Erdoğan
Dr Ahmet Erdoğan is a visiting academic at the University of Nottingham and an Assistant Professor in the Department of Mechanical Engineering at İnönü University. He obtained his Ph.D. in Mechanical Engineering from İnönü University in 2017. His areas of interest include Computational Fluid Dynamics, Refrigeration and Air Conditioning, and CO2 capture.
Fatih Güleç
Dr Fatih Gulec is an Assistant Professor in the Department of Chemical and Environmental Engineering at the University of Nottingham with expertise in energy and industrial decarbonisation. Dr gulec’s research encompasses (i) the synthesis and characterisation of advanced nanocomposites and their applications in chemical/calcium looping technologies, CO2 capture, negative emissions, energy storage and catalytic reactions and (ii) process integration/ intensification based on waste/biomass-to-energy via thermal conversion.
Jude A. Okolie
Dr. Jude A. Okolie is currently an Assistant Professor at the College of Engineering, University of Oklahoma and the co-founder of VComics. Dr. Okolie's research focuses on the thermochemical conversion of waste materials to green fuels and the subsequent utilization of hydrochar/biochars for environmental remediation. In addition, his research includes the application of process simulation and artificial intelligence/machine learning to address climate change, environmental pollution, and sustainable agriculture challenges. Dr. Okolie has been recognized for making significant contributions to the field of biomass to energy, reflected by his outstanding publication track record and peer recognition. He has been granted several prestigious local and international awards, including the George Ira Hanson Energy Award for his work on thermochemical hydrogen production and the University of Oklahoma, Alternative textbook grant. Dr. Okolie is the author of Biofuels book and also an editorial board member of the societal impact journal. He is a strong believer that anyone could be an engineer and thrive with the right support system.
References
- Ahmaruzzaman, M. 2008. Proximate analyses and predicting HHV of chars obtained from cocracking of petroleum vacuum residue with coal, plastics and biomass. Bioresource Technology 99 (11):5043–5050. doi:10.1016/j.biortech.2007.09.021.
- Aziz, N., H. Mohamed, D. Kania, H. C. Ong, B. S. Zainal, H. Junoh, P. J. Ker, and A. S. Silitonga. 2024. Bioenergy production by integrated microwave-assisted torrefaction and pyrolysis. Renewable and Sustainable Energy Reviews 191:114097. doi:10.1016/j.rser.2023.114097.
- Boztepe, C., M. Daskin, and A. Erdogan. 2022. Synthesis of magnetic responsive poly (NIPAAm-co-VSA)/Fe3O4 IPN ferrogels and modeling their deswelling and heating behaviors under AMF by using artificial neural networks. Reactive and Functional Polymers 173:105219. doi:10.1016/j.reactfunctpolym.2022.105219.
- Boztepe, C., M. Daskin, A. Erdogan, and T. Sarici. 2021. Preparation of poly (acrylamide‐co‐2‐acrylamido‐2‐methylpropan sulfonic acid)‐g‐Carboxymethyl cellulose/Titanium dioxide hydrogels and modeling of their swelling capacity and mechanic strength behaviors by response surface method technique. Polymer Engineering & Science 61 (7):2083–96. doi:10.1002/pen.25736.
- Callejón-Ferre, A., B. Velázquez-Martí, J. A. López-Martínez, and F. Manzano-Agugliaro. 2011. Greenhouse crop residues: Energy potential and models for the prediction of their higher heating value. Renewable and Sustainable Energy Reviews 15 (2):948–55. doi:10.1016/j.rser.2010.11.012.
- Chai, T., and R. R. Draxler. 2014. Root mean square error (RMSE) or mean absolute error (MAE)?–arguments against avoiding RMSE in the literature. geoscientific Model Development 7 (3):1247–50. doi:10.5194/gmd-7-1247-2014.
- Channiwala, S., and P. Parikh. 2002. A unified correlation for estimating HHV of solid, liquid and gaseous fuels. Fuel 81 (8):1051–63. doi:10.1016/S0016-2361(01)00131-4.
- Cordero, T., F. Marquez, J. Rodriguez-Mirasol, and J. J. Rodriguez. 2001. Predicting heating values of lignocellulosics and carbonaceous materials from proximate analysis. Fuel 80 (11):1567–71. doi:10.1016/S0016-2361(01)00034-5.
- Dashti, A., A. S. Noushabadi, M. Raji, A. Razmi, S. Ceylan, and A. H. Mohammadi. 2019. Estimation of biomass higher heating value (HHV) based on the proximate analysis: Smart modeling and correlation. Fuel 257:115931. doi:10.1016/j.fuel.2019.115931.
- Demirbas, A. 2002. Relationships between heating value and lignin, moisture, ash and extractive contents of biomass fuels. Energy exploration & exploitation 20 (1):105–111. doi:10.1260/014459802760170420.
- Demirbas, A. 2004. Combustion characteristics of different biomass fuels. Progress in Energy and Combustion Science 30 (2):219–230. doi:10.1016/j.pecs.2003.10.004.
- Demirbaş, A. 1997. Calculation of higher heating values of biomass fuels. Fuel 76 (5):431–34. doi:10.1016/S0016-2361(97)85520-2.
- Demirbas, A., D. Gullu, A. Çaglar, and F. Akdeniz. 1997. Estimation of calorific values of fuels from lignocellulosics. Energy Sources 19 (8):765–70. doi:10.1080/00908319708908888.
- Dobbelaere, M. R., P. P. Plehiers, R. Van de Vijver, C. V. Stevens, and K. M. Van Geem. 2021. Machine learning in chemical engineering: Strengths, weaknesses, opportunities, and threats. Engineering 7 (9):1201–11. doi:10.1016/j.eng.2021.03.019.
- Erdogan, A., and S. Canbazoglu. 2016. An investigation on energy consumption and 424 environmental effects of different architectural cases. Journal of Bartin University 425:51–60 .
- Friedl, A., E. Padouvas, H. Rotter, and K. Varmuza. 2005. Prediction of heating values of biomass fuel from elemental composition. Analytica Chimica Acta 544 (1–2):191–98. doi:10.1016/j.aca.2005.01.041.
- Golgiyaz, S., M. Daşkin, C. ONAT, and M. F. TALU. 2022. An artificial intelligence regression model for prediction of NOx emission from flame image. Journal of Soft Computing and Artificial Intelligence 3 (2):93–101. doi:10.55195/jscai.1213863.
- Güleç, F., A. Samson, O. Williams, E. T. Kostas, and E. Lester. 2022. Biofuel characteristics of chars produced from rapeseed, whitewood, and seaweed via thermal conversion technologies – impacts of feedstocks and process conditions. Fuel Processing Technology 239:107492. doi:10.1016/j.fuproc.2022.107492.
- Güleç, F., E. H. Şimşek, and H. Tanıker Sarı. 2022. Prediction of biomass pyrolysis mechanisms and kinetics: Application of the Kalman filter. Chemical Engineering & Technology 45 (1):167–177. doi:10.1002/ceat.202100229.
- Huang, Y.-F., and S.-L. Lo. 2020. Predicting heating value of lignocellulosic biomass based on elemental analysis. Energy 191:116501. doi:10.1016/j.energy.2019.116501.
- Jiménez, L., and F. González. 1991. Study of the physical and chemical properties of lignocellulosic residues with a view to the production of fuels. Fuel 70 (8):947–50. doi:10.1016/0016-2361(91)90049-G.
- Kathiravale, S., M. N. M. Yunus, K. Sopian, A. H. Samsuddin, and R. A. Rahman. 2003. Modeling the heating value of municipal solid waste☆. Fuel 82(9):1119–25. doi:10.1016/S0016-2361(03)00009-7.
- Li, X., Z. Huang, S. Shao, and Y. Cai. 2024. Machine learning prediction of physical properties and nitrogen content of porous carbon from agricultural wastes: Effects of activation and doping process. Fuel 356:129623. doi:10.1016/j.fuel.2023.129623.
- Lyons, G. J., F. Lunny, and H. P. Pollock. 1985. A procedure for estimating the value of forest fuels. Biomass 8 (4):283–300. doi:10.1016/0144-4565(85)90061-7.
- Majumder, A., R. Jain, P. Banerjee, and J. Barnwal. 2008. Development of a new proximate analysis based correlation to predict calorific value of coal. Fuel 87 (13–14):3077–81. doi:10.1016/j.fuel.2008.04.008.
- Maksimuk, Y., Z. Antonava, V. Krouk, A. Korsakova, and V. Kursevich. 2021. Prediction of higher heating value (HHV) based on the structural composition for biomass. Fuel 299:1–7. doi:10.1016/j.fuel.2021.120860.
- Ma, Y., W. Wang, H. Miao, S. Han, Y. Fu, Y. Chen, and J. Hao. 2024. Physicochemical synergistic effect of microwave-assisted Co-pyrolysis of biomass and waste plastics by thermal degradation, thermodynamics, numerical simulation, kinetics, and products analysis. Renewable Energy 223:120026. doi:10.1016/j.renene.2024.120026.
- Nhuchhen, D. R., and M. T. Afzal. 2017. HHV predicting correlations for torrefied biomass using proximate and ultimate analyses. Bioengineering 4 (1):7. doi:10.3390/bioengineering4010007.
- Nhuchhen, D. R., and P. A. Salam. 2012. Estimation of higher heating value of biomass from proximate analysis: A new approach. Fuel 99:55–63. doi:10.1016/j.fuel.2012.04.015.
- Nunes, L. J., J. C. D. O. Matias, and J. P. D. S. Catalao. 2017. Torrefaction of biomass for energy applications: From fundamentals to industrial scale. London,United Kingdom: Academic Press.
- Obernberger, I., T. Brunner, and G. Bärnthaler. 2006. Chemical properties of solid biofuels—significance and impact. Biomass and Bioenergy 30 (11):973–982. doi:10.1016/j.biombioe.2006.06.011.
- Ozyuguran, A., A. Akturk, and S. Yaman. 2018. Optimal use of condensed parameters of ultimate analysis to predict the calorific value of biomass. Fuel 214:640–646. doi:10.1016/j.fuel.2017.10.082.
- Özyuğuran, A., and S. Yaman. 2017. Prediction of calorific value of biomass from proximate analysis. Energy Procedia 107:130–136. doi:10.1016/j.egypro.2016.12.149.
- Parikh, J., S. Channiwala, and G. Ghosal. 2005. A correlation for calculating HHV from proximate analysis of solid fuels. Fuel 84 (5):487–494. doi:10.1016/j.fuel.2004.10.010.
- Qian, X., J. Xue, Y. Yang, and S. W. Lee. 2021. Thermal properties and combustion-related problems prediction of agricultural crop residues. Energies 14 (15):4619. doi:10.3390/en14154619.
- Rahib, Y., B. Sarh, J. Chaoufi, S. Bonnamy, and A. Elorf. 2021. Physicochemical and thermal analysis of argan fruit residues (AFRs) as a new local biomass for bioenergy production. Journal of Thermal Analysis and Calorimetry 145 (5):2405–16. doi:10.1007/s10973-020-09804-7.
- Saidur, R., E. A. Abdelaziz, A. Demirbas, M. S. Hossain, and S. Mekhilef. 2011. A review on biomass as a fuel for boilers. Renewable and Sustainable Energy Reviews 15 (5):2262–89. doi:10.1016/j.rser.2011.02.015.
- Sheng, C., and J. Azevedo. 2005. Estimating the higher heating value of biomass fuels from basic analysis data. Biomass and Bioenergy 28 (5):499–507. doi:10.1016/j.biombioe.2004.11.008.
- Telmo, C., J. Lousada, and N. Moreira. 2010. Proximate analysis, backwards stepwise regression between gross calorific value, ultimate and chemical analysis of wood. Bioresource Technology 101 (11):3808–15. doi:10.1016/j.biortech.2010.01.021.
- Thipkhunthod, P., V. Meeyoo, P. Rangsunvigit, B. Kitiyanan, K. Siemanond, and T. Rirksomboon. 2005. Predicting the heating value of sewage sludges in Thailand from proximate and ultimate analyses. Fuel 84 (7–8):849–57. doi:10.1016/j.fuel.2005.01.003.
- Vargas-Moreno, J., A. J. Callejón-Ferre, J. Pérez-Alonso, and B. Velázquez-Martí. 2012. A review of the mathematical models for predicting the heating value of biomass materials. Renewable and Sustainable Energy Reviews 16 (5):3065–83. doi:10.1016/j.rser.2012.02.054.
- Wei, Z., Z. Cheng, and Y. Shen. 2024. Recent development in production of pellet fuels from biomass and polyethylene (PE) wastes. Fuel 358:130222. doi:10.1016/j.fuel.2023.130222.
- Yin, C.-Y. 2011. Prediction of higher heating values of biomass from proximate and ultimate analyses. Fuel 90 (3):1128–32. doi:10.1016/j.fuel.2010.11.031.
- Zanzi, R., K. Sjöström, and E. Björnbom. 2002. Rapid pyrolysis of agricultural residues at high temperature. Biomass and Bioenergy 23 (5):357–366. doi:10.1016/S0961-9534(02)00061-2.
- Zhang, L., C. C. Xu, and P. Champagne. 2010. Overview of recent advances in thermo-chemical conversion of biomass. Energy Conversion and Management 51 (5):969–82. doi:10.1016/j.enconman.2009.11.038.