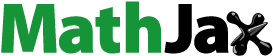
ABSTRACT
On the basis of conditional expectation of a random variable function, we present few characterization findings in this study. For a given function g, , we present necessary and sufficient conditions for characterization results in terms of a single function
. Some of these findings are completely novel, while others are expansions of previously published characterizations.
1. Introduction
Many scholars have explored probability distribution characterizations. According to [Citation1], a characterization theorem occurs in probability and statistics when a certain distribution is the only one that fits a specified property. Furthermore, a characterization, according to [Citation2], is a specific statistical or distributional attribute of a statistic or statistics that uniquely defines the associated stochastic model. Some academics suggested that before applying a probability distribution to real-world data, it should first be characterized under certain conditions. Several kinds of characterizations have been investigated for instance characterizations based on hazard functions, conditional expectations, truncated moments, order statistics and record values. Several authors, including [Citation3–8], have explored characterizations based on conditional expectations. In general, see, for example, for a survey or further information on these characterization subjects [Citation1, Citation2, Citation9–19] and references therein, to name a few, however there are numerous others.
We present some new and extended characterizations based on conditional expectations, motivated by the importance of probability distribution characterizations. The results of characterization are expressed in terms of a single function expression of X, say
.
2. Main results
In this paper, we present characterizations based on the following conditional expectations in the form where
is a mathematical expression of the function
:
(1)
(1)
(2)
(2)
(3)
(3)
(4)
(4)
(5)
(5)
(6)
(6)
such that
is a continuous and function that is differentiable on the interval
.
We are expanding certain works founded by [Citation20–22] as presented in Propositions 2.1–2.4, respectively, by using Equations (Equation1(1)
(1) )–(Equation4
(4)
(4) ). On the other side, as far as we know, the Equations (Equation5
(5)
(5) ) and (Equation6
(6)
(6) ) introduce new characterizations based on conditional expectations, as shown in the corresponding Propositions 2.5 and 2.6, respectively.
Proposition 2.1
Let be a random variable that is continuous with cdf F. Let
be function that is differentiable on
with
and
if
and
. Then,
if and only if
(7)
(7)
where
and
is the Gaussian hypergeometric function defined as
, see Equation (15.1.1) at page 556 in [Citation23].
Proof.
From Equation (Equation1(1)
(1) ), we have
Using derivatives from both sides in relation to x, we obtain
where
, see Equation (15.2.1) at page 557 in [Citation23].
From which we have
Integrating both sides from L to x,
Let
, then
(8)
(8)
where
by using Equations (1.110), (9.131.1) and (9.137.11) at pages 25, 1008 and 1010, respectively in [Citation24]. Therefore,
where
see Equation (9.131.1) at page 1008 in [Citation24]. From which we have
Let w = bv−1 then after simplification, we have
(9)
(9)
Conversely, if Equation (Equation7
(7)
(7) ) holds, then
Let
, we have
Now,
(10)
(10)
We obtain
Also,
(11)
(11)
From which we have
Moreover,
(12)
(12)
Hence,
where the Gaussian hypergeometric function defined as
.
By using Equation (Equation9
(9)
(9) ).
Remark 2.1
If r = 1 and using Equations (Equation10
(10)
(10) ) and (Equation11
(11)
(11) ) then from Equation (Equation1
(1)
(1) ) after simplification and using Equation (1.110) at page 25 in [Citation24], we obtain:
Taking, for example, r = 1,
and
then we obtain equation (44) in Proposition 10 in [Citation22].
Proposition 2.2
Let be a random variable that is continuous with cdf F. Let
be a function that is differentiable on
with
and
if 0<s<1 and
. Then,
if and only if
(13)
(13)
where
.
Proof.
If Equation (Equation2(2)
(2) ) holds, we have
Using derivatives from both sides in relation to x, we obtain
from which we have
Integrating both sides from L to x,
Let
and
, then
(14)
(14)
Conversely, if Equation (Equation13
(13)
(13) ) holds, then
Let
, we have
Using Equation (Equation14
(14)
(14) ), we get
Remark 2.2
As special cases see, for example, Proposition 2.5 page 22–23 in [Citation21].
and
and
and
Remark 2.3
Taking, for example, a = 1, r = c, s = 1−c and , we obtain Equation (1) of Proposition 2.1 in [Citation20].
Proposition 2.3
Let be a random variable that is continuous with cdf F. Let
be a function that is differentiable on
with
. Then, for
and
implies
(15)
(15)
where
.
Proof.
For r>1 and from Equation (Equation3(3)
(3) ), we have
Using derivatives from both sides in relation to x, we obtain
from which we have
Integrating both sides from L to x,
Let
, then for
(16)
(16)
For r = 1,
Let
, then
Let
, then
Therefore,
(17)
(17)
where
.
For r>1 and from Equation (Equation16(16)
(16) ),
(18)
(18)
It is difficult to obtain
analytically in closed form from Equation (Equation18
(18)
(18) ) but we will get it numerically as follows:
and
Therefore, from Equation (Equation18
(18)
(18) ), we have
. Now, let
By using the Newton-Raphson method as follows:
We obtain the root z such that
, i.e.
.
For r = 1 and from Equation (Equation17(17)
(17) ),
where
is the Lambert function. Hence,
Remark 2.4
Taking, for example, , r = 1, s = c and
, we obtain Equation (7) of Proposition 2.3 in [Citation20].
Remark 2.5
Taking, for example, , r = 1, s = 1−c and
, we obtain Equation (11) of Proposition 2.5 in [Citation20].
Proposition 2.4
Let be a random variable that is continuous with cdf F. Let
be a function that is differentiable on
with
and
. Then, for
implies
(19)
(19)
where
.
Proof.
From Equation (Equation4(4)
(4) ), we have
Using derivatives from both sides in relation to x, we obtain
from which we have
Integrating both sides from L to x,
Let
and
, then
where
is the Lambert function. Therefore,
Hence,
Remark 2.6
Taking, for example, a = 2, r = 1 and , we obtain Equation (15) of Proposition 2.7 in [Citation20].
Proposition 2.5
Let be a random variable that is continuous with cdf F. Let
be a function that is differentiable on
with
and
. Then, for
implies
(20)
(20)
where
and
is the Lambert function.
Proof.
From Equation (Equation5(5)
(5) ), we have
Using derivatives from both sides in relation to x, we obtain
from which we have
Integrating both sides from L to x,
Let
and
, then
Let
Then,
where
is the Lambert function. Hence,
Proposition 2.6
Let be a random variable that is continuous with cdf F. Let
be a function that is differentiable on
with
and
. Then, for
implies
(21)
(21)
where
and
is the Lambert function.
Proof.
From Equation (Equation6(6)
(6) ), we have
Using derivatives from both sides in relation to x, we obtain
from which we have
Integrating both sides from L to x,
Let
, then
Let
Then,
where
is the Lambert function. Hence,
3. Applications
As illustrations and without loss of generality, we apply three of these characterizations as follows:
Using Proposition 2.1 where
and
, we have
If
is the cdf of TL-G distribution (see [Citation25]) given by
then Proposition 2.1 (using Equation (Equation7
(7)
(7) )) gives a characterization of TL-G distribution as follows:
If
is the cdf of AGT-G distribution (see [Citation22]) given by
then Proposition 2.1 (using Equation (Equation7
(7)
(7) )) gives a characterization of AGT-G distribution as follows:
Using Proposition 2.3 where
and
, we get
If s = 1−c and
where
and
then
If s = c and
where
and
then
If s = c and
where
and
then
If s = 1−c and
where
and
then
Using Proposition 2.4 where
and
, we get
If
where
and
then
If
where
and
then
For other Propositions, they may be treated in a similar fashion.
4. Conclusions
The question of the characterization of a distribution is important in many fields and has recently aroused the interest of many researchers. As a result, several characterization results have been published in the literature. The purpose of this study is to present several characterizations of the distribution in their generality in hoping they would be beneficial to researchers wishing to know whether their model meets the requirements of a certain underlying distribution.
Disclosure statement
No potential conflict of interest was reported by the author.
References
- Koudou AE, Ley C. Characterizations of GIG laws: A survey. Probab Surv. 2014;11:161–176. DOI:10.1214/13-PS227
- Nagaraja H, Characterizations of probability distributions. In: Springer handbook of engineering statistics. 2006. p. 79–95. London: Springer. DOI:10.1007/978-1-84628-288-1_4
- Dimaki C, Xekalaki E. Towards a unification of certain characterizations by conditional expectations. Ann Inst Stat Math. 1996;48:157–168. DOI:10.1007/BF00049296
- Ghitany M, Gupta R, Wang S. Some characterization results by conditional expectations and their applications to lindley-type distributions. Int J Probab Stat. 2017;7:86. DOI:10.5539/ijsp.v7n1p86
- Khan A, Athar H, Yaqub M. Characterization of probability distributions through conditional expectation of function of two order statistics. Calcutta Stat Assoc Bull. 2001;51:259–266. DOI:10.1177/0008068320010309
- Ruiz JM, Navarro J. Characterizations based on conditional expectations of the doubled truncated distribution. Ann Inst Stat Math. 1996;48:563–572. DOI:10.1007/BF00050855
- Su JC, Huang WJ. Characterizations based on conditional expectations. Stat Pap. 2000;41:423. DOI:10.1007/BF02925761
- Zoroa P, Ruiz J, Marín J. A characterization based on conditional expectations. Commun Stat Theory Methods. 1990;19:3127–3135. DOI:10.1080/03610929008830368
- Ahsanullah M, Shakil M, Kibria BMG. Characterizations of folded student's t distribution. J Stat Distrib Appl. 2015;2:15. DOI:10.1186/s40488-015-0037-5
- Ahsanullah M, Shakil M, Kibria BG. On a generalized raised cosine distribution: some properties, characterizations and applications. Moroc J Pure Appl Anal. 2019;5:63–85. DOI:10.2478/mjpaa-2019-0006
- Ahsanullah M. Characterizations of univariate continuous distributions. Paris: Springer; 2017. DOI:10.2991/978-94-6239-139-0
- Ahsanullah M, Shakil M. A note on the characterizations of pareto distribution by upper record values. Commun Korean Math Soc. 2012;27:835–842. DOI:10.4134/CKMS.2012.27.4.835
- Ahsanullah M, Shakil M. Characterizations of continuous probability distributions occurring in physics and allied sciences by truncated moment. Int J Adv Stat Probab. 2015;3:100–114.
- Ahsanullah M, Shakil M, Kibria BMG. Characterizations of continuous distributions by truncated moment. J Mod Appl Stat Methods. 2016;15:17. DOI:10.22237/jmasm/1462076160
- Galambos J, Kotz S. Characterizations of probability distributions: a unified approach with an emphasis on exponential and related models. Vol. 675. Berlin: Springer; 2006. DOI:10.1007/BFb0069530
- Hamedani G. Characterizations of recently introduced univariate continuous distributions II. Nova Science Publishers; 2019. (Mathematics research developments). Available from: https://books.google.com.sa/books?id=rtAywAEACAAJ.
- Khan MI. Characterization of some continuous distributions by truncated moment. J Stat Manag Syst. 2021;0:1–10. DOI:10.1080/09720510.2021.1933709
- Kotz S, Shanbhag DN. Some new approaches to probability distributions. Adv Appl Probab. 1980;12:903–921. DOI:10.2307/1426748
- Shakil M, Ahsanullah M, MG Kibria B. Some characterizations and applications of a size-biased weighted distribution useful in lifetime modelling. J Stat Appl Probab. 2021;10:607–624. DOI:10.18576/jsap/100301
- Hamedani G. A few characterizations of the univariate continuous distributions. JIRSS. 2016;15:63–71. Available from: https://iranjournals.nlai.ir/bitstream/handle/123456789/600330/C1AEA206AD3A27B2CEAF61EFEA8CB376.pdf?sequence=-1
- Hamedani G, Mameli V. Characterizations of the generalized beta-generated family of distributions. J Stat Theory Appl. 2017;16:18–25. DOI:10.2991/jsta.2017.16.1.2
- Merovci F, Alizadeh M, Hamedani GG. Another generalized transmuted family of distributions:properties and applications. Austrian J Stat. 2016;45:71–93. Available from: https://www.ajs.or.at/index.php/ajs/article/view/doi
- Abramowitz M. Handbook of mathematical functions: with formulas, graphs, and mathematical tables. New York: Dover Publications; 1970. Available from: https://books.google.com.sa/books?id=4j2hwwEACAAJ.
- Gradshteyn IS, Ryzhik IM. Table of integrals, series, and products. New York: Academic Press; 2007.
- Al-Shomrani A, Arif O, Shawky A, et al. Topp–Leone family of distributions: some properties and application. Pakistan J Stat Oper Res. 2016;12:443–451. DOI:10.18187/pjsor.v12i3.1458