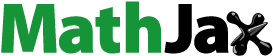
Abstract
New pyrrolidine derivatives with more than 50% structural similarity with captopril were designed to get new captopril mimics with superior potential to act on both peripheral and central ACE. Further optimization was carried out through pharmacophoric mapping, then pharmacokinetics of these compounds were analyzed, 42 derivatives were selected for further study, as they exhibited potential to pass through BBB. Molecular docking on ACE using captopril and lisinopril as reference drugs was performed, and Compound 28 (2-Pyrrolidin-2-ylidene-N-thiomorpholin-4-ylmethyl-malonamic acid ethyl ester) showed the best docking scores, proving its superiority over captopril and comparability to lisinopril. Further molecular dynamics simulations and energy calculations demonstrated binding stability and close mimicry to both drugs. The results indicate that Compound 28 is a promising candidate for further investigations as a potential drug to act centrally and peripherally. Compound 28 can be synthesized by reacting Cyano-pyrrolidin-2-ylidene-acetic acid ethyl ester through Mannich reaction with thiomorpholine and formaldehyde.
1. Introduction
The burden of cardiovascular disorders (ischemic heart disease and stroke) on health is evident; WHO (world health organization) has considered these disorders as common causes of death worldwide, with an estimated 17.8 million deaths annually [Citation1]. The mortality rates over the past decade leading to 2017 present an alarming 21% increase. The burden of cardiovascular diseases is not exclusively derived from the fatalities they cause; morbidity and disability associated with cardiovascular diseases are major aspects of the illness's epidemiology [Citation2].
Generally, management and treatment of such cases begin with clinical manifestations. However, the problem in this manifestation is often sudden death [Citation3]. As a result, the focus is shifted towards the underlying risk factors such as hypertension which affects one-third of the adult world population [Citation4]. As in gestational hypertension, several conditions cause secondary hypertension, which is reversible and resolves eventually after the cause passes [Citation5,Citation6]. Most cases suffer from primary or essential hypertension, which is irreversible and multifactorial [Citation7]. The management of this menace includes; (1) non-pharmacological lifestyle intervention such as weight loss, stress reduction, and physical activity [Citation4]. (2) pharmacological drug therapy, including Ca channel blockers, diuretics, and renin-angiotensin system inhibitors (angiotensin-II receptor blockers and angiotensin-I converting enzyme blockers) [Citation8].
Captopril is the first orally active peptide inhibitor of the angiotensin-I converting enzyme (ACE) used to manage hypertension [Citation9]. It is characterized by rapid oral absorption and an exaggerated fall in blood pressure following the initial dose [Citation10]. Additionally, research has shown an intriguing property resulting from the captopril-induced ACE inhibition in the brain [Citation11]. These data indicated that captopril and other ACE inhibitors possess neuron effects, resulting in the retardation of signs of neurodegenerative disorders such as Alzheimer's disease [Citation12,Citation13]. These features made captopril an intriguing prospect for derivatization to attain more potent hypotensive agents with prospective neuroprotective function, significantly benefiting Alzheimer's disease and other disorders. However, one of the limiting factors of its development is the side effects attributed to its mercapto group, which caused allergic reactions, taste disturbance and indirect weight loss, and the extensive metabolism of the inactive disulphide derivative [Citation14–16].
Our design maintained the pyrrole moiety of captopril as the cornerstone. The acidic COOH was substituted with other moieties, like the nitrile group. Substituents were distributed to either the pyrrole nitrogen atom or in the place of the nitrile group adjacent to the pyrrole, as shown in . Furthermore, the compounds were designed without the mercapto group to minimize any possible side effects owing to the group. These compounds were then analyzed through various in silico studies.
2. Methods
2.1. Design of captopril mimics
The compounds were designed using captopril substructure, and a total of one hundred and two analogues were obtained. These newly designed mimics will be subjected to several CADD techniques, such as pharmacophore mapping, virtual screening, molecular docking and molecular dynamics to get the best hits to be synthesized.
2.2. E-pharmacophore generation and screening
The protein containing angiotensin-1 convertingenzyme (ACE) in complex with captopril was obtained from the protein data bank (PDB ID: 1UZF) containing captopril and ACE at a resolution of 2 Å [Citation17]. The protein was preprocessed, solvent molecules beyond 5 Å of heteroatoms were removed, and missing hydrogens and loops were rectified. After minimization using OPLS3e, the structure was imported into “Develop pharmacophore from protein–ligand complex” for the e-pharmacophore generation in Schrodinger Maestro [Citation18], and the pharmacophore was mapped. The validation step was then performed using two data sets; the first set contained eight known ACE inhibitors (captopril, enalapril, lisinopril, fosinopril, ramipril, quinapril, benazepril, and trandolapril). While the second had four hundred structurally similar inactive dummies generated by the DUD-E website (www.dude.docking.org).
2.3. ADMET descriptors
Multiple descriptors of ADMET properties (absorption, distribution, metabolism, excretion and toxicity) of the compounds were calculated using the “Schrodinger Maestro” suite. “QikProp” protocol utilizes the 3D structure of the compounds to predict relevant properties such as log S (aqueous solubility), log Khsa (human serum binding albumin), SASA (total solvent-accessible area), log HERG (predicted IC50 for HERG K+ channel blockage), pKa (Supp. Data, Table 1S) and many other pharmacokinetic features. It provides an intrinsic scoring function (#stars) that compares each calculated descriptor with its optimal range for 95% of known drugs [Citation19–22].
2.4. Molecular docking
The file containing the human angiotensin-converting enzyme in complex with lisinopril was downloaded from the protein data bank (PDB ID: 1O86) [Citation23]. The screened compounds were docked via MOE 2019 software [Citation24]. The software's default approach for structural optimization was used to create and optimize both receptors and compounds. After creating a library of chemicals, the software's usual structure optimization methodology was used to generate both receptors and ligands. Using the AMBER12: EHT force field, energy minimization was carried out, and the active site was assigned to the pocket enclosing the co-crystallized ligands. The “Induced-fit protocol” of MOE DOCKTITE Wizard's induced fit protocol, which uses the GBVI/WSA dG scoring function, was used to carry out the docking. Re-docking lisinopril into the binding pocket and calculating the root mean square deviation (RMSD) between poses were both used to accomplish validation [Citation25–29].
2.5. Molecular dynamics simulations and molecular mechanics-Poisson Boltzmann surface area (MM-PBSA) calculations
Molecular dynamics simulations were performed using GROningen MAchine for Chemical Simulations (GROMACS 2022.3) software [Citation30,Citation31]. Enzyme chains containing active sites were isolated. The enzyme and the ligand topologies were generated and joined using the standard published protocol under the Amber 99SB-ILDN force field [Citation32]. Ligands topology files were successfully generated using the ACPYPE-AnteChamber PYthon Parser interface using General Amber Force Field (GAFF) [Citation33]. The typical workflow of GROMACS enzyme-ligand simulations was applied in which systems were solvated using a TIP3P (three-site transferrable intermolecular potential) water model in a dodecahedron box Na+/Cl− ions were sufficiently added for neutralization [Citation34]. The energy minimization (1000.0 kJ/mol/nm) was performed using the steepest descent method, followed by a canonical ensemble (NVT at 300 K) and isothermal-isobaric (NPT at 1 bar) to pre-balance the system successively. All systems were simulated with 50 ns MD simulation. After the MD simulation, the GROMACS package built-in tools were deployed to evaluate the trajectories in terms of RMSD, the radius of gyration (Rg), and root mean square fluctuation (RMSF) after residues numbers were corrected [Citation20,Citation35]. While “gmx covar” and “gmx anaeig” functions were used for the implementation of Principle component analysis (PCA). Gibbs free energy landscapes (FEL) were calculated through “g_sham” using the predicted distribution probability of the top two principal components (PCs) [Citation36].
The MM-PBSA approach calculates the interaction free energy of protein–ligand complexes (potential, polar-solvation and non-polar solvation energies) for molecular dynamics simulation trajectories. The gmx_MMPBSA (1.5.7) tool was employed for the last 2ns of the simulation processes (48–50 ns) with two frame intervals [Citation37]. The trajectories were prepared by removing PBC conditions, and calculations performed using Amberff19SB and GAFF forcefields were used for proteins and ligands under the dielectric model (ipb = 2) [Citation38]. Binding free energies were then calculated using either of the following two equations:
(ΔH corresponds to the enthalpy of binding and −TΔS to the conformational entropy after ligand binding)
In which to calculate each term of the above equation is calculated individually through the application of several procedures to get the energy terms for complex, receptor and ligand as described in the literature [Citation39].
2.6. Density functional theory (DFT) analysis
The Spartan ‘14 programme was used to perform quantum chemistry calculations using the DFT method. Spartan ‘14 was used to display all the data files. The density functional theory (DFT) at 6-311G++(d,p) basis set/B3LYP approach was utilized to optimize the organic chemical structure of the compounds under investigation, and Chem3D 15.0 software was used to create the original chemical structure [Citation40].
3. Results and discussion
3.1. Design of captopril mimics
These new mimics (1-102) shown in were designed to resemble the captopril 2D structure, keeping the pyrrolidine moiety as the main scaffold. Several computational techniques will explore these new mimics to find the most hopeful candidates.
3.2. E-pharmacophore generation and screening
The generated pharmacophore model showed three features in the form of a triangle with features around 3.00–3.48 Å apart from each other. When superposed on captopril, features were distributed as follows, hydrophobic feature in the pyrrolidine residue, negative ionic feature in the carboxylic region, and acceptor feature in the amide carbonyl group. The area under the accumulated curve (AUAC) and receiver operating characteristic (ROC) were calculated for the hypothesis and yielded 0.74 for both. The library was screened, and all designed compounds fitted the designed pharmacophore model; represents the fitted pharmacophoric features for the target compounds and illustrates that the designed mimics share more than 70% of captopril features.
3.3. Drug-likeness study
Lipinski's rule of five measures the “Drug likeness” of compounds through four properties (molecular weight, lipophilicity, hydrogen bond donors, and hydrogen bond acceptors) [Citation41]. All the designed compounds entirely adhered to the rule of five (Table 1S), and no violations were observed. The # stars count provides a comprehensive overview of the inclination of the compounds toward attaining favourable pharmacokinetic and metabolic profiles. The highest portion of our compounds, about 92.16% (ninety-four compounds), achieved high similarity of the calculated properties to the standard ranges of known drugs. At the same time, 5.88% (six compounds) had one property outside the acceptable 95% range of known drugs. Only 1.96% (two compounds) had a higher violation score of 2. Additionally, the “Rule of three”, determining the probability of compounds’ oral bioavailability when complying with certain predetermined factors, was enforced. Only 77.45% (seventy-nine compounds) conformed to the rule of three, while the other 22.55% (twenty-three compounds) had one descriptor, only violating the rule ().
The blood–brain barrier permeability of the library revealed the ability of almost half of the designed compounds to reach CNS 45.10% (forty-six compounds). Most of the compounds showed good metabolic steps (<8), with three metabolic steps being the highest frequent metabolic order. The compounds showed high safety profiles with no predicted blockage of HERG K+, which can cause QT prolongation, ventricular arrhythmias, and sudden death [Citation42]. Moreover, 98% (one hundred compounds) showed no plasma protein binding, adding to the safety profile of the design as well as access to biological targets.
All the above rules guided us to select the most promising drug-like compounds for further analysis (). This resulted in forty-two compounds being promoted for molecular modelling study.
3.4. Molecular docking
The study was performed on a human angiotensin-converting enzyme (ACE) obtained from the protein databank (PDB ID: 1O86). It contained the enzyme complexed with Zinc ion (Zn701) and lisinopril. Lisinopril, a known inhibitor of this metalloenzyme, was used as a reference for docking validation and for comparative analysis of the activity of our compounds in addition to captopril. After the compounds and the receptor were prepared appropriately for docking through MOE. The first step analyzed the validity of our docking protocol by calculating RMSD between the co-crystallized and docked pose of lisinopril, which resulted in 0.73 Å, indicating successful validation (). Afterwards, the compounds’ docking commenced, and the results are presented in . Although some research highlighted the beneficial outcomes of setting restraints during molecular docking [Citation43], our study was performed without such restraints encouraged by other research studies with similar practical aspects [Citation44] and precision of validation. All compounds achieved favourable binding to ACE with negative scores (Kcal/mole). The two drugs used for comparison, namely captopril and lisinopril, scored −6.54 and −12.47 Kcal/mole, respectively. Nearly all compounds (34 compounds, 80%) were able to produce superior scores than captopril, while the remaining eight compounds produced close submarginal binding scores. The top-scoring compounds, 28 and 18, achieved close results to lisinopril, with one even out-performing scoring −12.69 and −11.81 Kcal/mole, respectively.
Figure 6. Validation of docking protocol showing RMSD of 0.73 Å. (Green is the co-crystallized pose, and pink is the re-docked pose).

Table 1. Docking scores of compounds against human ACE in Kcal/mole.
Analysis of their binding interactions () illustrated the binding of both drugs to Zn701 and the formation of several hydrogen bonds to HIS353 and TYR520. Additionally, the extended nature of lisinopril offered extra binding ionically with GLU162, ASP377, and LYS511. As well as several hydrophobic interactions with HIS383 and VAL518.
Table 2. Binding interactions of lisinopril, captopril and compounds 28 and 18.
The highest-scoring compound, 28, interacted with Zn701 through the nitrogen atom of its thiomorpholine ring. Furthermore, its double carboxylic groups acted as hydrogen bond acceptors to imidazole rings of HIS353 and HIS513 and the phenolic hydroxyl group of TYR520. On the other hand, Although 18 possessed similar moieties, the placement of the acetamido group didn't allow for the formation of hydrogen bonds with TYR520, thus decreasing its score, as evident in .
The interesting properties of 28 encouraged further exploration and analysis of its binding to ACE using subsequent molecular dynamic simulation and energy calculations.
3.5. Molecular dynamic simulations and molecular mechanics-Poisson Boltzmann surface area (MM-PBSA) calculations
The computerized approach known as molecular dynamics (MD) is used to investigate the dynamic behaviour of complex systems where atoms and molecules interact over time, thus, generally increasing the effectiveness of drug discovery. In contrast to standard molecular docking techniques, MD simulations consider the targets’ flexibility. When coupled with estimations of binding energies, a more precise prediction of potential inhibitors can be made [Citation45]. ACE (PDB ID: 1O86) in complexes with captopril, lisinopril and 28 were simulated under normal physiological conditions for 50 ns each. Afterwards, stability, dynamic behaviour, and compactness of protein-drug complexes were assessed using structural characteristics such as RMSD, RMSF, and Rg.
3.5.1. Root mean square deviation (RMSD)
To determine whether or not ACE is stable in complex with the chosen compounds, we measured their RMSD of the protein backbone. The average RMSD of captopril, lisinopril and 28 complexes produced close values of 0.14±0.01, 0.16±0.01 and 0.16±0.03 nm, respectively. As shown in , the similarity in the behaviour of ACE across the three simulations illustrates the similarity of 28 in terms of effects on the protein to both reference drugs. Furthermore, each compound's RMSD was calculated throughout the simulation in which values of 0.10±0.02, 0.10±0.02 and 0.17±0.03 nm were observed for captopril, lisinopril and 28, respectively (). These homogenous data provide evidence of the stability of the three ACE complexes.
3.5.2. Root mean square fluctuations (RMSF)
The RMSF analysis was performed, and the values were plotted against the residue number to compute the residual mobility and behavioural similarity of 28 to the two reference drugs. Overall, the RMSF shows consistent trends in all three simulations, with residues involved in interactions mentioned before showing low fluctuations, such as HIS513 and TYR520 ().
3.5.3. Radius of gyration (Rg) and solvent accessible surface area (SASA)
The radius of gyration (Rg) and solvent-accessible surface area (SASA) are useful tools for gaining insight into the folding and compactness of protein–ligand complexes. The ability of a medication to cause conformational changes in proteins can be evaluated using Rg and SASA. The Rg value indicates how loosely packed the molecules are within a protein, while SASA predicts the extent of conformational changes that occur during binding. When simulating a stable ligand–protein interaction, the Rg and SASA values plateau [Citation36]. Figures and show that the simulated structures of all complexes are relatively conservative and stable at sizes of around 2.41 nm and ∼245 nm2 for Rg and SASA, respectively.
3.5.4. Principal component analysis (PCA)
Throughout the simulation, PCA was used to evaluate the linked motions. Both eigenvalues and eigenvectors were determined as projections of the proteins’ Cα atoms. Eigenvectors define motion orientation, whereas eigenvalues represent motion magnitude. The projected motions of ACE when complexed with 28 express high similarity to when the metalloenzyme is complexed with either reference drug, as shown by the overlapping in eigenvectors in . These overlaps suggest that when attached to 28 and reference inhibitors, the proteins act similarly, creating persistent clusters and occupying the same subspace, indicating the stability of the created complexes. Individual examination of the correlated motion of the first ten eigenvectors (which account for 80% of the variations) reveals that 28 displayed moderate relative motion with residues lower than captopril and slightly greater than lisinopril ().
The proteins’ conformational space was analyzed in terms of energy and time using principle component (PC) analysis. PC1 and PC2 were then utilized to determine Gibbs's free energy in each case, and the Free Energy 3D Landscape (FEL) was plotted. These energy graphs depict high and low energies in red and blue colours. Evaluation spatial positioning of the of atoms in the system using Gibbs FEL demonstrates that complexes formed metastable formations that cross multiple energy barriers indicating high stability. Compound 28, captopril and lisinopril all have comparable energy surfaces with canonical-shaped energy minima indicating the presence of stable minima ().
Molecular Mechanics Poisson-Boltzmann Surface Area (MM-PBSA) is one of the most often used methods for calculating binding free energy. The lower the predicted binding free energy of a ligand–protein complex, the more stable that complex is expected to be and the higher the ligand's activity and potency. The energy values of captopril and lisinopril produced consistent values of −5.36±2.75 and −5.04±1.64 kcal/mole, respectively. While the complex with compound 28 resulted in a slightly lower value of −6.39±1.50 Kcal/mole. Consistent with previous results, the values obtained exhibit relatively similar behaviour in both cases ().
Table 3. MMPBSA calculations of ACE in complex with captopril, lisinopril and 28.
3.6. DFT analysis
The DFT/B3LYP approach was used in the current work to perform quantum chemical computations to optimize the selected structures. The DFT (B3LYP) method with 6-311G++(d,p) basis set was applied in this test. The optimized structure and its HOMO and LUMO values are represented in and . The HOMO energy expresses the ability of the compound to give electrons as an electron donor. It is localized mainly on the thiomorpholine moiety of compound 5 and the mercaptomethylpropanoyl residue of captopril.
Table 4. The molecular properties of compounds 28 and captopril after DFT calculations.
On the other hand, the LUMO energy displayed by a site that has the ability to act as an electron attractive, i.e. electron acceptors due to vacant orbitals and localized at L-proline residues of compound 28 and captopril, respectively. The electrostatic potential maps of the compounds showed areas with electron localization throughout the molecules, with red and blue colours representing electron-rich (negative) and deficient (positive) localization, respectively. Finally, the DFT calculations revealed favourable energetic parameters for the selected compound (5), .
4. Conclusion
Our journey started with a search for suitable ACE inhibitors that can act peripherally and centrally; a library of one hundred and two captopril analogues was designed. The library was then subjected to several investigations through pharmacophoric screening, drug-likeness, molecular docking, and molecular dynamic simulation. Most of the designed compounds conformed to the generated pharmacophore. The subsequent drug-likeness study resulted in the most promising forty-two compounds afterwards. Molecular docking illustrated the compounds that can occupy the same space as captopril and showed their ability to interact with the metalloenzyme to various extents. The most promising analogue was compound 28, which out-performed captopril and produced comparable results to lisinopril while attaining higher CNS permeability, thus, a higher potential to act centrally. The molecular dynamic simulation was performed for 50 ns to assess the stability of the complexes. All calculations, such as RMSD, RMSF, SASA and Rg, supported the stability of binding and the close mimicry of 28 to both reference drugs. Additionally, MM-PBSA calculations, PCA and FEL also supported our postulations. Finally, DFT expanded on its favourable energetic parameters as well. Thus, the potential of thiomorpholine pyrrolidine derivative (compound 28) to target both central and peripheral ACE encourages further testing and analysis for approving this hopeful captopril mimic as a future antihypertensive drug.
Supplemental Material
Download MS Word (31.3 KB)Acknowledgments
The authors extend their appreciation to the Deputyship for Research & Innovation, Ministry of Education in Saudi Arabia for funding this research work through the project number: IFP22UQU4290565DSR235.
Disclosure statement
No potential conflict of interest was reported by the author(s).
Data availability statement
All data are incorporated in the manuscript and supplementary data files.
Additional information
Funding
References
- Roth GA, Abate D, Abate KH, et al. Global, regional, and national age-sex-specific mortality for 282 causes of death in 195 countries and territories, 1980–2017: a systematic analysis for the Global Burden of Disease Study 2017. Lancet. 2018;392:1736–1788.
- Townsend N, Kazakiewicz D, Lucy Wright F, et al. Epidemiology of cardiovascular disease in Europe. Nat Rev Cardiol. 2022;19:133–143.
- Whelton PK. Epidemiology of hypertension. Lancet. 1994;344:101–106.
- Valenzuela PL, Carrera-Bastos P, Gálvez BG, et al. Lifestyle interventions for the prevention and treatment of hypertension. Nat Rev Cardiol. 2021;18:251–275.
- Sibai B. Diagnosis and management of gestational hypertension and preeclampsia. Obstet Gynecol. 2003;102:181–192.
- Chiong JR, Aronow WS, Khan IA, et al. Secondary hypertension: current diagnosis and treatment. Int J Cardiol. 2008;124:6–21.
- Brouwers S, Sudano I, Kokubo Y, et al. Arterial hypertension. Lancet. 2021;398:249–261.
- Umemura S, Arima H, Arima S, et al. The Japanese Society of Hypertension guidelines for the management of hypertension (JSH 2019). Hypertens Res. 2019;42:1235–1481.
- Koch-Weser J, Vidt DG, Bravo EL, et al. Captopril. N Engl J Med. 1982;306:214–219.
- Atkinson A, Robertson JI. Captopril in the treatment of clinical hypertension and cardiac failure. Lancet. 1979;314:836–839.
- Unger T, Kaufmann-Bühler I, Schölkens B, et al. Brain converting enzyme inhibition: a possible mechanism for the antihypertensive action of captopril in spontaneously hypertensive rats. Eur J Pharmacol. 1981;70:467–478.
- AbdAlla S, Langer A, Fu X, et al. ACE inhibition with captopril retards the development of signs of neurodegeneration in an animal model of Alzheimer’s disease. Int J Mol Sci. 2013;14:16917–16942.
- O’Caoimh R, Healy L, Gao Y, et al. Effects of centrally acting angiotensin converting enzyme inhibitors on functional decline in patients with Alzheimer’s disease. J Alzheimer’s Dis. 2014;40:595–603.
- Alsharif NZ. Medicinal chemistry and therapeutic relevance of angiotensin-converting enzyme inhibitors. Am J Pharm Educ. 2007;71:123.
- Martin MFR, Mckenna F, Bird HA, et al. Captopril: a new treatment for rheumatoid arthritis? Lancet. 1984;323:1325–1328.
- Waeber B, Gavras I, Brunner HR, et al. Safety and efficacy of chronic therapy with captopril in hypertensive patients: an update. J Clin Pharmacol. 1981;21:508–516.
- Natesh R, Schwager SLU, Evans HR, et al. Structural details on the binding of antihypertensive drugs captopril and enalaprilat to human testicular angiotensin I-converting enzyme. Biochemistry. 2004;43:8718–8724.
- Schrödinger LLC. Schrödinger Release 2021-3: Maestro. Schrödinger LLC; 2021.
- Ntie-Kang F. An in silico evaluation of the ADMET profile of the StreptomeDB database. Springerplus. 2013;2:353.
- Sun X, Belal A, Elanany MA, et al. Identification of some promising heterocycles useful in treatment of allergic rhinitis: virtual screening, pharmacophore mapping, molecular docking, and molecular dynamics. Russ J Bioorganic Chem. 2022;48:438–456.
- Kamel MS, Belal A, Aboelez MO, et al. Microwave-assisted synthesis, biological activity evaluation, molecular docking, and ADMET studies of some novel pyrrolo [2,3-b] pyrrole derivatives. Molecules. 2022;27:2061.
- Elsayed MMA, Aboelez MO, Elsadek BEM, et al. Tolmetin sodium fast dissolving tablets for rheumatoid arthritis treatment: preparation and optimization using box-behnken design and response surface methodology. Pharmaceutics. 2022;14:880.
- Natesh R, Schwager SLU, Sturrock ED, et al. Crystal structure of the human angiotensin-converting enzyme–lisinopril complex. Nature. 2003;421:551–554.
- ULC, C.C.G. Molecular Operating Environment (MOE), 2019.01; 2020.
- Bhimaneni S, Kumar A. Abscisic acid and aloe-emodin against NS2B-NS3A protease of Japanese encephalitis virus. Environ Sci Pollut Res. 2022;29:8759–8766.
- Belal A, Elkady H, Al-Karmalawy AA, et al. Discovery of some heterocyclic molecules as bone morphogenetic protein 2 (BMP-2)-inducible kinase inhibitors: virtual screening, ADME properties, and molecular docking simulations. Molecules. 2022;27:5571.
- Elanany MA, Osman EEA, Gedawy EM, et al. Design and synthesis of novel cytotoxic fluoroquinolone analogs through topoisomerase inhibition, cell cycle arrest, and apoptosis. Sci Rep. 2023;13:4144.
- Belal A, Elanany MA, Santali EY, et al. Screening a panel of topical ophthalmic medications against MMP-2 and MMP-9 to investigate their potential in keratoconus management. Molecules. 2022;27:3584.
- Belal A, Elanany MA, Raafat M, et al. Calendula officinalis phytochemicals for the treatment of wounds through matrix metalloproteinases-8 and 9 (MMP-8 and MMP-9): in silico approach. Nat Prod Commun. 2022;17:1934578X2210988.
- Bauer P, Hess B, Lindahl E. GROMACS 2022.4 Source code; 2022.
- Van Der Spoel D, Lindahl E, Hess B, et al. GROMACS: fast, flexible, and free. J Comput Chem. 2005;26:1701–1718.
- Maier JA, Martinez C, Kasavajhala K, et al. ff14SB: improving the accuracy of protein side chain and backbone parameters from ff99SB. J Chem Theory Comput. 2015;11:3696–3713.
- Sousa da Silva AW, Vranken WF. ACPYPE - AnteChamber PYthon Parser interfacE. BMC Res Notes. 2012;5:367.
- Mark P, Nilsson L. Structure and dynamics of the TIP3P, SPC, and SPC/E water models at 298 K. J Phys Chem A. 2001;105:9954–9960.
- Belal A, Elsayed A, Gharib AF, et al. Toward the discovery of SARS-CoV-2 main protease inhibitors: exploring therapeutic potentials of evodiamine and its derivatives, virtual screening, molecular docking, and molecular dynamic studies. Nat Prod Commun. 2022;17:1934578X-2211430.
- Nada H, Elkamhawy A, Lee K. Identification of 1H-purine-2,6-dione derivative as a potential SARS-CoV-2 main protease inhibitor: molecular docking, dynamic simulations, and energy calculations. PeerJ. 2022;10:e14120.
- Miller BR, McGee TD, Swails JM, et al. MMPBSA.py: an efficient program for end-state free energy calculations. J Chem Theory Comput. 2012;8:3314–3321.
- Wang J, Cai Q, Xiang Y, et al. Reducing grid dependence in finite-difference Poisson–Boltzmann calculations. J Chem Theory Comput. 2012;8:2741–2751.
- Valdés-Tresanco MS, Valdés-Tresanco ME, Valiente PA, et al. Gmx_MMPBSA: a new tool to perform end-state free energy calculations with GROMACS. J Chem Theory Comput. 2021;17:6281–6291.
- Legler CR, Brown NR, Dunbar RA, et al. Scaled Quantum Mechanical scale factors for vibrational calculations using alternate polarized and augmented basis sets with the B3LYP density functional calculation model. Spectrochim Acta Part A Mol Biomol Spectrosc. 2015;145:15–24.
- Lipinski CA, Lombardo F, Dominy BW, et al. Experimental and computational approaches to estimate solubility and permeability in drug discovery and development settings 1PII of original article: S0169-409X(96)00423-1. The article was originally published in Advanced Drug Delivery Reviews 23 (1997). Adv Drug Deliv Rev. 2001;46:3–26.
- Vandenberg JI, Perry MD, Perrin MJ, et al. hERG K + channels: structure, function, and clinical significance. Physiol Rev. 2012;92:1393–1478.
- Caballero J. Considerations for docking of selective angiotensin-converting enzyme inhibitors. Molecules. 2020;25:295.
- Ke Z, Su Z, Zhang X, et al. Discovery of a potent angiotensin converting enzyme inhibitor via virtual screening. Bioorg Med Chem Lett. 2017;27:3688–3692.
- Liu X, Shi D, Zhou S, et al. Molecular dynamics simulations and novel drug discovery. Expert Opin Drug Discov. 2018;13:23–37.