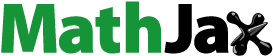
Abstract
Climate change has emerged as a pressing concern affecting nations worldwide, particularly within the African continent, including Benin. Given that maize stands as a staple cereal in Benin, this research to assess the impact and predict the effects of climate change on maize production by the year 2050. To attain this objective, an assortment of data encompassing climatic conditions, demographic factors, fertilizer application levels, and emissions of environmental pollutants has been collected and analyzed. The data analysis based on ARDL and ARIMA models has unveiled those variables such as emissions of CO2 and CH4 through food waste, peak temperatures, precipitation patterns, and rural population density exert considerable immediate influence over maize production volumes. The predictive models portend an upswing in national maize production volume, albeit accompanied by a probable decline in per capita availability. Policies aimed at controlling activities that generate high levels of air pollutants should be formulated to increase production capacities.
1. Introduction
As outlined in the Fifth Assessment Report by the Intergovernmental Panel on Climate Change, the twenty-first century is poised to witness a heightened proliferation of climate risks across numerous global regions, with a pronounced impact on developing nations and those with limited economic resources. Of note, Africa, and specifically West Africa, emerges as a heightened vulnerability hotspot to the fluctuations and change in climate. This vulnerability can be attributed to a combination of its distinct physical attributes and socio-economic dynamics, rendering the region disproportionately susceptible to the adverse consequences stemming from shifts in climatic patterns [Citation1]. Since the late 1960s, West and Central African countries have experienced worsening climate variability [Citation2]; which weakens agricultural systems that no longer respond to climate pressures [Citation3]. Kurukulasuriya et al. [Citation4] had already highlighted the great vulnerability of the continent to climate change due to the strong dependence of economies on agriculture and the limited adaptive capacities of populations. The magnitude of food-related hazards is substantial, and those at the highest risk include farmers, individuals from rural and urban impoverished communities, and others [Citation5,Citation6].
Benin, categorized as a developing nation with agriculture as its economic cornerstone, is not on the sidelines of the harmful effects of these climate changes [Citation7]. According to Ogouwalé [Citation6] and Boko [Citation8], Benin is experiencing a reduction in the length of the agricultural season, a persistence of adverse deviations from norms, escalating temperatures, and deteriorating precipitation patterns that are reshaping the landscape of agricultural production. Notably, the role of Benin's agriculture in driving economic advancement has exhibited a steady decline since 2006, when it reached 2.1%. This decline can be attributed to climatic disruptions that have significantly impeded agricultural output. Noteworthy instances include the floods of 2007 and 2010, the delayed and uneven distribution of rains in 2008 and 2009, and sporadic episodes of drought [Citation9].
Indubitably, agriculture serves as the primary income source for approximately 75% of the working population in this West African country [Citation10]. Concomitantly, the agricultural sector contributes 38% to the Gross Domestic Product (GDP), an impressive 88% to export earnings [Citation11], 15% to government revenue, and offers livelihoods to nearly 70% of the workforce [Citation12]. The landscape of this sector is predominantly shaped by crop cultivation, encompassing a diverse array of food crops. These crops constitute the foundational bedrock of the population's food and nutritional security, with cereals reigning supreme in Benin. Foremost among these is maize (Zea mays L.), cultivated extensively across the entire expanse of the nation, commanding the foremost position by occupying nearly 70% of cereal-growing areas [Citation7,Citation13]. Maize cultivation spans every region within the country, adapting to diverse conditions. Notably, maize's cultivation contributes 78.3% to the total cereal production and commands 52.6% of the overall cultivated land in 2015 [Citation12]. Thus, the maize sector emerges as the premier player in ensuring food security, holding a paramount role. Additionally, it secures the second spot by generating substantial income for rural households [Citation14]. Furthermore, previous studies have highlighted a trend of decreasing corn production (yield and surface area) over the years [Citation15]. It is recognized in previous work that many environmental factors contribute to this agricultural trend. However, most previous studies carried out in this direction have not taken into consideration the global context which involves all environmental factors including air pollutants. Thus, these studies have frequently analyzed only the influence of temperature and rainfall on agricultural production [Citation16–18]. While other studies have focused exclusively on the perception and adaptation strategies of producers in the face of climate change [Citation7,Citation19]. Furthermore, most of these studies have not used “staggered delay models” capable of taking into account temporal dynamics (adjustment periods, expectations, etc.) to elucidate the behaviour of each of these variables (series temporal).
It would therefore be more interesting to combine not only other factors such as atmospheric pollutants but also to use these models to better assess the influence of each of these parameters, including their previous dynamics, on corn production. Finally, the study attempted to predict the effects of these parameters on corn production in Benin by 2050.
2. Methodology
2.1. Choice of study area
The selection of Benin as a study area is justified by several crucial elements. First of all, the survey covers all administrative divisions of the country, thus providing a complete representation of its territory. Indeed, having agriculture as the main source of the country's economy, the country is more susceptible to the repercussions of climate change [Citation20]. This increased vulnerability of Benin to climate change makes the approach of a study focusing on the evaluation of the influence of this phenomenon on agricultural production relevant. Moreover, this is becoming more coherent, with particular emphasis on maize, recognized as the most widely cultivated crop and the predominant staple food [Citation21]. The map below provides an overview of the study's geographic scope, encompassing the entirety of Benin:
2.2. Data used
Numerous datasets were harnessed in this study, notably encompassing climatic factors such as rainfall, minimum temperature, and maximum temperature. Additionally, atmospheric pollutants, including carbon dioxide (CO2), methane (CH4), and nitrous oxide (N2O), were examined. The rationale for selecting these parameters is rooted in the imperative of addressing climate change inquiries through the lens of these multifaceted components, particularly acknowledging their roles in shaping greenhouse gases and core climatic variables. These very indicators constitute the bedrock upon which the extensive assessment conducted by the Intergovernmental Panel on Climate Change (IPCC) in their latest summary report of the sixth assessment cycle [Citation22] relies. The impacts of these changes are far-reaching, spanning areas such as agriculture, grappling with intensified temperature fluctuations, climate-linked illnesses, compromised water access, and soil acidification. These risks collectively undermine food production, leading to diminished food security and economic repercussions across sectors, with agriculture bearing a significant brunt [Citation22].
Indeed, the negative influences of temperature and precipitation on agricultural output, specifically maize volume, have been underscored by researchers in previous studies [Citation23]. Similarly, other scholars have highlighted the adverse relationship between atmospheric pollutants like CO2 and CH4 and the production of agricultural commodities, mediated through alterations in soil acidity [Citation24]. These premises rationalize the incorporation of these variables within the present study. Beyond the climatic markers, additional parameters, especially social indicators such as population density in both rural and urban areas, have been integrated. Demography emerges as another pivotal determinant of the developmental trajectory of agriculture (land productivity) in developing nations [Citation25].
Furthermore, as explanatory elements influencing the production volume under consideration, fertilizers (specifically Nitrogen, potassium K2O, and phosphate fertilizers) were incorporated. The selection of these fertilizers stems from the understanding that, alongside the climate-related challenges discussed earlier, the diminished productivity of maize is significantly linked to declining soil fertility [Citation26]. Certain studies emphasize that the provision of essential mineral elements, particularly nitrogen, phosphate, and potassium fertilizers, augments maize yield and consequentially its production volume [Citation27].
Regarding metrics gauging maize production levels, both the average annual cultivated areas across the nation and the total annual production volumes (measured in tons) were considered. The rationale for including cultivated area stems from its established positive correlation with production volume within the literature [Citation28]. Meanwhile, the production volume (total tons) stands as a fundamental indicator signifying the significance of crop production during a specific timeframe for a given crop within distinct regions [Citation29].
Precipitation, maximum and minimum temperature data were procured from the Benin Agency for Air Navigation Safety (ASECNA), whereas data pertaining to other parameters (air pollutants, population density, fertilizers, and maize production indicators) were collected over a 30-year span (1990-2020) from the Food and Agriculture Organization of the United Nations (FAO) website.
2.3. Analysis method
The chronological evolution of parameters was assessed using time series, statistical analysis tools that track the trajectory of variables over time, providing meaningful predictions [Citation30]. Unlike traditional statistical approaches, this study opted for dynamic lag (DL) models due to their ability to take into account temporal dynamics, thereby improving the accuracy of predictions on the behaviour of variables [Citation31]. These models, similar to ARDLs, encompass both short-term effects and long-term dynamics. Furthermore, the difference between short-term and long-term effects is marked by “step lags”, reflecting the different scales of response of the dependent variable to the variation of the independent variable.
The particularity of distributed lag (DL) models is linked to the fact that these models are divided into two categories: autoregressive (AR) models, which integrate past values of the dependent variable () among the explanatory variables, and staggered lag models (DL), which use the past values of the independent variable (
). To do this, autoregressive distributed lag (ARDL) models merge these characteristics by including both past values of the dependent variable and the independent variable in the explanatory variables [Citation31,Citation32].
If we consider the dependent variable “” and the independent variable “
”, the general form of these different distributed lag (DL) models is as follows [Citation31, Citation33]:
However, ARDL models often face challenges related to error autocorrelation, especially when they include the lagged endogenous variable as an explanatory element [Citation32]. To address this issue, the DL method was chosen to mitigate error autocorrelation, especially near the dependent variable. The general equation of the model applied in this study is then formulated as follows:
With:
: designating the error term;
;
, … … … ,
reflecting the short-term effect of each explanatory variable in year t on the dependent variable in the same year t (
); then
;
; … … … ;
translating the short-term effect of each explanatory variable shifted to the year
on the dependent variable to the year t
The description of the variables has been given in Table .
Table 1. Summary table of variables used for the model.
Considering the following long-run or equilibrium relation: “”, the long-run effect
on
(or “
”) is determined as follows:
[Citation31,Citation32].
The use of the models depends on the cointegration of the time series, implying that they have the same order of integration [Citation31]. If levels of differentiation vary between series, an error correction model (ECM) is recommended [Citation31]. To assess stationarity, the Augmented Dickey-Fuller (ADF) test, Kwiatkowski-Phillips-Schmidt-Shin (KPSS) test, and Phillips-Perron (PP) test were used, followed by successive differentiations to make the series stationary. An ECM model was then applied to present the results of the short- and long-term distributed lag (DL) models.
2.4. Production volume prediction
Predicting production volume remains a challenge in distributed lag and autoregressive models, often absent from previous studies [Citation31–33]. In this research, the ARIMA approach was adopted to predict the explanatory values, influencing the volume of corn production. These forecasts were then used to directly estimate the production volume results in the distributed lag model, thus introducing a novelty in this study. Consider the ARIMA time series of an explanatory variable
written in the form of a linear transfer function of a series of noises:
To obtain a forecast
(L being any positive integer denoting a next year or time frame) of the explanatory variable
, the following equation was used [Citation30]:
The calculation of the conditional mathematical expectation of
knowing its past to date
is given by:
with:
[Citation30].
To identify the parameters (p, q), characterizing the order of the autoregressive component (p) and that of the moving average component (q), the partial autocorrelation functions (PAFC) and that of simple autocorrelation (AFC) were respectively used as observed in the literature [Citation30]. The choice of the parameters p and q was made following the observation of the correlograms of these functions.
To conduct each of the different analyzes developed, the R version 4.3.0 software was used, and Excel was used to enter the data obtained from our two sources (ASECNA and FAO). (Figures and )
Figure 1. Map of the study area (Benin). Source: [Citation20].
![Figure 1. Map of the study area (Benin). Source: [Citation20].](/cms/asset/12af0886-64c5-4abb-9fc0-8bbc4e02d6bd/tusc_a_2316361_f0001_oc.jpg)
3. Results
3.1. Time series of climatic parameters and volume of maize production in Benin during the period 1990 to 2020
3.1.1. Evolution of climatic parameters
The assessment of rainfall evolution was conducted based on the three distinct seasons delineated by the climatic attributes defined by DGEC [Citation20]: the extended wet season spanning June, July, September, and October, the extensive dry season encompassing November to March, and the transitional period comprising April, May, and August. In this transitional phase, the northern-central region experiences a dry spell (April and May) succeeded by a rainy spell (August), while the southern region undergoes a wet phase (April and May) followed by a dry spell (August). A chronological analysis of total national rainfall between 1990 and 2020 (refer to Figure ) demonstrates irregular oscillations in both the prolonged wet season and the dry season, even during the transitional phase. Nonetheless, the overall national rainfall remains higher during the extended wet season and the transitional period combined.
The analysis of the progression of maximum and minimum temperatures in Benin was conducted in alignment with the previously outlined three seasons (Figure ). Naturally, there is a discernible pattern wherein temperatures, both at their peak and lowest, are elevated during the extended dry season, prevailing from November to March across the entire national expanse. Subsequently, the transitional period and the ensuing wet season manifest cooler temperatures. This anticipated pattern is inherently logical – during the dry season, the sun's dominance results in daily warmth, whereas the alternating and rainy seasons exhibit the converse phenomenon.
These findings align with those from reputable sources such as the Intergovernmental Panel on Climate Change [Citation22] and the National Aeronautics and Space Administration [Citation34], underscoring the mounting global temperature increases that serve as a definitive hallmark of climate change. These increments are situated within the broader context of worldwide global warming [Citation35]. Analogous outcomes are apparent concerning minimum temperatures, which exhibit an upward trajectory during the rainy season, particularly in the alternating phase. However, the assessment of minimum temperature data during the dry season delineates a downward trend. In 2020, the average minimum temperature registered around 19°C, diverging from the 21°C plus range witnessed from 1990 to 2015. This might be attributed to sporadic instances of rainfall observed during the prolonged dry season, indicating the evolving seasonal dynamics encountered by agricultural producers. Similar observations have been made by other researchers like Djenontin [Citation36], Dedjan [Citation37], and Yegbemey et al. [Citation38], who noted that local communities, especially in northern Benin, have perceived shifts in their regional climate. These shifts are manifested through uneven rain distribution and occasional alterations in the onset of the rainy season. (Figures and )
3.1.2. Evolution of atmospheric pollutants
The subsequent visuals illustrate the chronological trajectory of atmospheric contaminants spanning time, specifically focusing on methane (CH4), carbon dioxide (CO2), and nitrous oxide (N2O). This temporal assessment unveils the volumes of each pollutant, categorized by their originating emission sources – most notably, emissions stemming from agricultural activities (AS) (including incineration, synthetic fertilizer usage, bushfires, manure utilization, and crop residue), emissions linked to household food waste (FW) (encompassing household consumption, commercial products, and food processing), and emissions arising from energy and transportation practices.
Turning to the CO2 emission series, it unveils an expanding and notable production attributed primarily to food waste and, more significantly, to other origins such as energy production, electricity generation, and transportation. The CO2 emission volume from agricultural practices in 2020 is almost negligible, whereas emissions originating from household food waste, energy consumption, and transportation persistently ascend. These findings can be elucidated by the shared sources of methane and nitrous oxide production, notably agricultural practices. Within agriculture, the utilization of chemical fertilizers exacerbates nitrogen release into the environment. Similarly, processes like manure decomposition and rice production are tangible agricultural sources of methane emissions [Citation39]. Notably, in Benin, rice occupies a pivotal role among essential food crops and stands as the second most consumed cereal in households, following maize [Citation40,Citation41]. Its production has notably doubled in recent years, surpassing 220,000 tons in 2015 [Citation40], underscoring the substantial production intensity of this crop and thereby the potential for pollutant emission risks, such as methane. The surge in CO2 emissions, on the other hand, is primarily attributed to electricity and heat generation [Citation42], as well as energy consumption, often involving coal and electricity [Citation43].
3.1.3. Evolution of population density
The graph presented below illustrates the chronological progression of the population in Benin over time. Both the rural and urban populations demonstrate a consistent upward trajectory, thereby reflecting the overall growth evident in the total population. Notably, the rural population exhibits a more pronounced upward trend compared to the urban population, and currently, the urban population is also converging with the rural population. These increases reaffirm the rapid pace of population expansion experienced by nations worldwide, particularly those within sub-Saharan Africa. This region has emerged as a potential hub for demographic surge, largely attributed to elevated fertility rates, notably in rural areas where household sizes tend to be larger [Citation44]. This phenomenon accounts for the substantial inclination of the rural population as opposed to the urban counterpart.
3.1.4. Volume of production, area sown and quantities of fertilizers used
Figure summarize the national evolution of production volume (in tonnes), total agricultural area (in hectares) and fertilizer quantities (in tonnes) over time. A major observation is the continued growth in production volume, particularly for corn. From 400,000 tonnes and less than 500 hectares in the 1990s, corn production increased considerably to exceed 1,600,000 tonnes on almost 1,267,159 hectaresThese observations can be attributed to several factors. Firstly, the strategic inclusion of maize in Benin's Agricultural Sector Development Plan has played a pivotal role in promoting this crop and ensuring household food security, along with poverty alleviation efforts [Citation45]. Secondly, the exponential population growth observed both in rural and urban areas significantly contributes to increased food demand [Citation44]. Consequently, for a staple like maize, which holds the status of the most consumed food item [Citation21,Citation41], the rapid population increase markedly elevates the necessity for this commodity.
The subsequent graph indicates a parallel rise in the utilization of phosphate, nitrogen, and potassium (K2O) fertilizers, particularly from the year 2015 onward. The intensive adoption of these chemical fertilizers, often in the form of NPK blends, is primarily attributed to the escalating employment of adaptive strategies by producers to enhance soil fertility levels. This becomes crucial due to the progressive soil degradation witnessed year after year [Citation26]. These mineral fertilizers, enhancing maize yield, consequently amplify its production volume [Citation27].
3.1.5. Significance of time series for maize production volume
Considered as the variable of interest for the present study, it is essential to understand the factors exerting a significant influence on the evolution of the volume of production. Furthermore, it is important to first verify the temporal effect at the level of this variable before exploring the other determining factors. Indeed, the analysis of the significance thresholds of the simple autocorrelation plots (ACF) shows several significant delays. These results confirm that the residual values of these variables are not independent. In other words, this suggests that the observations at time t-1 and those of the following years are linked. (Figures and )
Furthermore, the partial autocorrelation graph shows a single significant delay, thus reflecting the possibility of the existence of other determining factors in its evolution.
3.2. Impact of climatic parameters on the volume of maize production in Benin between 1990 and 2020
3.2.1. Analysis of the determinants of the volume of maize production
The application of the ARDL method is preceded by a careful evaluation of the stationarity of the explanatory variables taken into account in the model. To achieve this, three tests have been employed to scrutinize the stationarity of each distinct series corresponding to the various parameters (refer to Table ). These tests encompass the Augmented Dickey-Fuller (ADF) test, the Kwiatkowski-Phillips-Schmidt-Shin (KPSS) test, and the Phillips-Perron (PP) test.
Table 2. Stationarity test of the variables.
The ADF test, known as the Augmented Dickey-Fuller test, evaluates the presence of a unit root in a time series, indicating non-stationarity [Citation31]. Analysis of the results reveals that none of the explanatory variables demonstrate stationarity (p > 0.05). Similarly, the outcomes of the KPSS test align with the aforementioned results for most variables, with the exception of the data pertaining to fertilizer quantities. Specifically, the KPSS test indicates that the variable associated with fertilizer quantity is stationary, contrary to the first test (p > 0.05). However, results from the Phillips-Perron test, akin to the ADF test, indicate that the majority of variables are non-stationary (p > 0.05), except for the production volume (dependent variable), rural population density, and maximum temperature. These variables exhibit stationary characteristics (p < 0.05) according to this particular test.
To recap, a minimum of two tests concur that all series exhibit non-stationarity. This circumstance amply justifies proceeding with differentiation, as certain studies have similarly undertaken only one or two tests to assess stationarity [Citation46].
3.2.2. Short-term effects of climatic and social factors through the error correction model (ECM)
The selection of the optimal lagged model was guided by the Schwarz Information Criterion (SIC), ensuring the attainment of statistically significant outcomes (refer to Table ). The chosen model encompasses several factors, including the quantity of CO2 derived from food waste (CO2_Food_waste), the amount of methane resulting from food waste (CH4_Food_waste), along with methane sourced from electricity and transportation (CH4_Others). Additionally, the model considers nitrogen fertilizer quantity (Nitrogen_fertilizer_N), maximum temperature (Temperature_max), rainfall (Rainfall), and the densities of rural (rural_population) and urban (urban_population) populations. Lag periods were established based on an analysis of the autocorrelation functions for each explanatory parameter.
Table 3. Estimation of short-term effects.
Evaluation of the outcomes from the lagged model indicates that several variables – CO2_Food_waste, CH4_Food_waste, maximum temperature, rainfall, and rural population density – hold significant short-term sway over maize production volume. The table reveals a significant negative correlation in the short term between atmospheric pollutants like CO2 from food waste and methane from other emission sources such as energy and transportation. The coefficients signify that a one-unit increase (1 kiloton) in their levels in the environment two years prior leads to a subsequent reduction of several tons (41,590 tons) in maize production volume two years later. Notably, while the inverse relationship is well-discussed, the converse also holds true. Crops and forests are profoundly affected by climate change and atmospheric pollutants like CO2 and methane, given that ecosystem functioning is intrinsically linked to factors like radiation, temperature, water presence, and precipitation. These factors, which are significantly affected by air pollution, can impact ecosystems both positively (e.g. atmospheric deposition and carbon storage) and negatively, including through dysfunctions like acidification. Moreover, pH variations strongly correlate with waste decomposition, a process that facilitates the release of methane and CO2 into the environment [Citation47].
In addition to these variables, the table underscores the adverse impact of rising maximum temperatures and increased rainfall on production volume. These findings align with Adjovi et al. [Citation48], who also observed a negative influence of these climatic parameters on maize production. They specified that maize is more susceptible to climatic variability compared to other crops like sorghum or soybeans, which adapt better to warmer conditions.
Furthermore, the results reveal a significant negative association in the short term between rural population density and maize production volume at a lag period. The coefficient signifies that an increase of one individual in rural areas during the preceding year corresponds to a decrease of approximately six (06) tons of maize in the subsequent year. Although these results counter the anticipated positive effect of this factor, they can be attributed to the growing phenomenon of rural-to-urban migration, which is increasingly prevalent on a national scale. This trend reduces the agricultural population's intensity and subsequently the production volume, while amplifying the demand for agricultural products in urban areas. This aligns with the DGEC [Citation20] report, emphasizing human mobility and urban migration as climate change adaptation strategies increasingly embraced by the rural population.
For the other explanatory variables, no statistically significant effect on maize production volume was observed. This might be attributed to these factors not yet reaching a threshold of substantial influence within this country's context.
Below are the estimation results of the optimal ARDL model retained:
In the long term, as indicated in Table , only the increased utilization of nitrogenous fertilizers demonstrates a significant influence at the 10% significance threshold on maize production volume. In contrast, the remaining climatic parameters exhibit a lack of significant long-term impact on production volume. This observation might be attributed to the escalating adoption of new improved crop varieties available in the market, renowned for their heightened resilience to climatic adversities like limited rainfall, elevated temperatures, and emerging diseases from diverse sources [Citation29]. Indeed, an array of adaptation strategies is increasingly being embraced by producers to navigate the challenges posed by climate change. Among these strategies, besides deploying climate-resistant crop varieties, producers also employ adjustments to cropping calendars (such as altering sowing dates or modifying the frequency of weeding) to capitalize on the initial rainfall signals marking the onset of the rainy seasons [Citation20].
Table 4. Long-term effects of climatic factors on production volume.
3.2.3. Post-estimate diagnostics of the estimated error correction model (ECM)
Regarding the diagnostic tests employed to assess the validity of the estimated short-term model, it is noteworthy that the results from the Durbin-Watson (DW) and Breusch–Godfrey tests (refer to Table ) reveal an absence of errors autocorrelation. These test choices align with the methodology outlined by Kuma [Citation31]. Additionally, these findings are corroborated by the graphical analysis of residuals. Furthermore, there is no presence of heteroscedasticity, and the errors conform to a normal distribution. Consequently, the model aligns well with the specifications stipulated by the Ramsey test (Fisher), effectively elucidating the interplay between explanatory variables and the dependent variable, without necessitating the incorporation of nonlinear terms.
Table 5. Results of short-term model diagnostic tests.
Given the acceptance of the null hypothesis across all these tests, it can be deduced that this model maintains its statistical integrity. Therefore, it stands as an acceptable representation from a statistical standpoint.
3.3. Prediction of maize production volume under the influence of climatic parameters
The endeavour to forecast maize production volume, influenced by significant explanatory variables, is illustrated through the subsequent graph, presenting a reasonably apt prediction. It is worth noting that the ARDL methodology, including distributed delay models, doesn't inherently facilitate forecasting of future values for the dependent variable. This characteristic is evident in several studies that have employed this analysis method, including works by Kuma [Citation31], Bensbahou and Seyagh [Citation32], Mestiri [Citation33], Demirhan [Citation49], and Tagwi [Citation50].
However, for the purpose of forecasting maize production volume in upcoming years, this study employed an endogenous modeling approach, specifically the Auto Regressive Integrated Moving Average (ARIMA) type, coupled with the outcomes from the realized dynamic model (DL). This strategy aligns with the methodology utilized by Sawadogo et al. [Citation51], who employed a similar approach for predicting grain prices in Burkina Faso. While ARIMA models forecast based on previous parameter data, they don't account for the impact of exogenous variable shocks on the considered parameter [Citation51]. This limitation is addressed effectively by dynamic models such as the stepped-delay models utilized in this study.
To achieve this, ARIMA was employed to predict the values of influential explanatory variables that significantly impact maize production volume. These variables include CO2 quantity from food waste, CH4 quantity from electricity and transport sectors, maximum temperature, rainfall, and rural population density-effects that are observed prominently in the produced dynamic model. These predicted values were then utilized to directly forecast the production volume, based on the short-term effects identified in the model estimation results.
As depicted in the prediction graph for the forthcoming years, there is an exponential growth trend in national-level production volume, even under the influence of key determining factors such as CO2 quantity from food waste, CH4 quantity from electricity and transport sectors, maximum temperature, rainfall, and rural population density. The forecasted values of explanatory variables derived from the ARIMA models indicate a gradual evolution of the various evaluated parameters. Analysis of the prediction graphs suggests that by 2030, CO2 quantity could surpass 200 kt and potentially exceed 400 kt by 2050 in Benin. Similarly, methane is projected to surpass 200 kt by 2030, with a potential quantity approaching 400 kt by 2050. Population size could reach seven million inhabitants by 2030, aligning with forecasts from the DGEC [Citation20], which predicts a population increase of approximately 2.7% annually for Benin.
Regarding rainfall, a slight upward trend is evident compared to previous years, with a potential increase of at least 1000 mm by 2050 compared to the 1990s (Figure ). Nonetheless, the trend in rainfall evolution appears less pronounced than the other parameters, suggesting the possibility of stable rainfall over the next decade. This outlook is echoed by Benin's Ministry of the Environment, Housing and Urban Planning, which states in its 2021 report on rainfall forecasts that there will likely be relatively consistent annual rainfall from south to north of the country until 2100, compared to the reference period of 1971 to 2000.
Lastly, maximum temperature is anticipated to experience a gradual evolution over time, consistently remaining within the range of 38°C. This temperature rise aligns with predictions made by the Ministry of the Environment [Citation52], which anticipates temperature increases throughout all regions of Benin by 2100, with the most significant thermal rise estimated at 3.27°C compared to the reference period of 1971-2000. This temperature increase, in turn, contributes to heightened potential evapotranspiration (ETP) and a scenario of water scarcity, ultimately impacting overall agricultural production, including maize, as highlighted by the Ministry of Environment [Citation52]. (Figure )
Despite the anticipated escalation of various climatic parameters and atmospheric pollutants, along with an increase in maximum temperature according to ARIMA forecasts, there will still be a substantial rise in maize production volume over time. The results of the predictions strongly suggest a production volume surpassing threefold (4 million tons of maize) the amount produced in 2020 (approximately 1.6 million tons of maize). This noteworthy production surge is primarily attributed to the projected rise in the agricultural population (rural population) in the forthcoming years, as outlined by the forecasts concerning explanatory variables, particularly the evolution of the rural population in relation to weather conditions. Consequently, despite the impact of climatic factors like atmospheric pollutants, rainfall, and maximum temperature, the maize production volume could experience a considerable increase by 2030. These findings align with certain predictions put forth in the Second National Communication regarding maize production yields in specific zones of Benin by 2025, including the extreme North-Benin zone, northern cotton-growing zone, North-Donga zone, and West-Atacora zone [Citation52]. However, in other agro-ecological zones such as the southern food crop zone, barre land zone, depression zone, and fisheries zone, forecasts by MEHU [Citation52] indicate a considerable decline in maize yields by 2025. Nevertheless, when considering the entire national territory, a general upswing in production levels by 2025 is expected, and these predictions concur with the outcomes of the present study.
4. Discussion
The results demonstrate a steady decline in national precipitation, especially during the rainy season, from around 5,000 mm in 1990 to less than 3,500 mm in 2020. This trend is consistent with observations by other researchers, including Atchadé et al. [Citation53], highlighting changes in rainfall regimes since 1970, characterized by late or early onsets of rainfall and interruptions during peaks, with an overall decrease in annual rainfall [Citation53]. Djenontin [Citation36], Dedjan [Citation37], and Yegbemey et al. [Citation38] also found irregular rain sequences and premature seasons influencing the perception of climate change by farmers in northern Benin.
Unlike total precipitation, maximum and minimum temperatures are increasing steadily each year, a trend aligned with global observations from the IPCC and NASA that identify this rise as a major sign of climate change [Citation22]. These temperature increases are strongly linked to the broader context of global warming [Citation35]. Farmers report intensifying heat, even in shaded areas under trees, highlighting the warming trend [Citation54].
Concerning air pollutant emissions, analyzes show that methane and nitrous oxide emissions mainly come from food waste and agricultural practices, while CO2 is mainly emitted by food waste, energy consumption (especially electricity) and transport. These results support previous research that associates CO2 emissions with electricity and heat production, as well as energy consumption (coal and electricity), and attributes methane and nitrous oxide emissions to practices agriculture [Citation39,Citation42,Citation43]. With population growth, pollution is expected to increase alongside the expansion of production and areas of maize, the dominant crop in Benin (around 85%), playing a crucial role in the diet compared to rice and sorghum [Citation21].
In addition to the production volume of previous years, the analysis of the autocorrelation function highlights a significant association between the production volume at time t-1 and the following year, resulting from the accumulation of agricultural experience and the adoption of ameliorative strategies over time [Citation55]. The ECM model confirms the significant impact of temperature and precipitation on the volume of corn production, in agreement with the findings of Adjovi et al. [Citation48] on the influence of climate indicators on corn, sorghum and soybean yields. Furthermore, a negative correlation between atmospheric pollutants (CO2 and methane) and the volume of corn production is noted, supported by the work of Chandio et al. [Citation46] on the impact of CO2 on wheat production, and supported by Casemir and Diaw [Citation24] as well as Cellier et al. highlighting the change in soil acidity and its negative impact on production yield.
The Step Lag (DL) model indicates a significant negative impact of rural population density on the volume of corn production, contradicting the positive observations of other researchers [Citation28,Citation56]. These researchers suggest that rapid population growth in rural areas may lead to an expansion of cultivated land at the expense of grazing areas and peripheral vegetation [Citation56], although the expansion of cultivated areas is considered essential to the growth of agricultural production [Citation28].
Forecasts indicate continued expansion in the volume of corn production over the next ten to thirty years, despite the influence of methane and the exponential growth of the urban population. These results confirm MEHU [Citation52] forecasts for an increase in maize production yields by 2025 in various agro-ecological zones of Benin. This growing projection of corn production volume may also be linked to the gradual increase in rural population density, often considered the most productive segment [Citation57,Citation58].
5. Conclusion
The results of this study indeed show an upward trend in climatic parameters such as rainfall, maximum and minimum temperature as well as the emission of atmospheric pollutants such as CH4, CO2 and N2O which are released into the atmosphere (from food waste, agricultural practices and other sources such as electricity and the transport sector). These results clearly indicate that Benin is not on the sidelines of climate change characterized mainly by global warming of the planet. Faced with this situation, agricultural producers are greatly increasing the areas sown each year for maize production to keep the total volume of production high despite situations of climate instability. Similarly, several climatic factors including temperature, rainfall and atmospheric pollutants (CO2, CH4) as well as the density of the rural population are the main parameters having a strong short-term influence on the volume of production. The predictive models carried out under the influence of temperature, which significantly influences the volume of production, predict an increase in maize production over time. However, curtailing activities that contribute to the release of atmospheric pollutants into the environment holds the potential to enhance national-level production volume by mitigating the repercussions of climatic fluctuations.
Author contributions
YEM, SA, KFD: Writing – review & editing; SA: Project administration, Funding acquisition; YEM, KFD: Investigation, Data curation; KFD: Sampling & investigation; YEM: Project Supervision.
Acknowledgments
The authors would like to sincerely thank the BAP of Çukurova University (Project no: FDK-2023-15492) for their participation. The authors also thank Prof. Dr. Haydar ŞENGÜL and Prof. Dr. Handan Vuruş AKÇAÖZ for their support.
Disclosure statement
No potential conflict of interest was reported by the author(s).
Data availability statement
Data supporting reported results is available as supplementary files and anything provided at reasonable request.
Additional information
Funding
References
- Niasse M, Afouda A, Amani A. Réduire la Vulnérabilité de l’Afrique de l’Ouest aux impacts du climat sur les ressources en eau, les zones humides et la désertification. Eléments de stratégie régionale de préparation. UICN, Gland & Cambridge; 2004; 59 p.
- Adjahossou VN. Stratégies d’adaptation des paysans du plateau d’Allada (Bénin) aux changements climatiques). XXVIIe Colloque de l’Association Internationale de Climatologie; Dijon (France); 2014. p. 255–259.
- Kinhoegbe J, Ouinsou E. Analyse économique de la prise en compte des changements climatiques dans la production du riz: Cas de la Vallée de l’Ouémé, Mémoire de Licence en Planification et Gestion des Projets, École Nationale d’Économie Appliquée et de Management; 2016. 69p.
- Kurukulasuriya P, Mendelsohn R, Hassan R, et al. Will African agriculture survive climate change? World Bank Econ Rev. 2006;20:367–388.
- FAO. Food insecurity: when people must live with hunger and fear starvation. The state of food insecurity in the world 2002. Rome (Italy): FAO; 2002; 214 p.
- Ogouwalé E. Changements climatiques et sécurité alimentaire dans le Bénin méridional. Mémoire de DEA, UAC/EDP/FLASH; 2004; 119p.
- Behanzin PC, Brisso D, Sainou J, et al. Climate change and production of corn: perceptions and effects on the municipality of Allada in Benin. Environ Water Sci Public Health Territ Intel J. 2018;2(4):106–118.
- Boko M. Climats et communautés rurales du Bénin: rythmes climatiques et rythmes de développement. Thèse de Doctorat ès d’Etat. Dijon, France: Université de Bourgogne; 1988; 601 p.
- BIPEN. Implications économiques des changements climatiques dans le secteur agricole; 2012; 115p.
- MAEP. Politiques agricoles à travers le monde quelques exemples. Rapport de performance secteur agricole. Ministère de l’Agriculture, de l’Élevage et de la Pêche, Bénin; 2015; 5 p.
- Gologo H. Place à l’adaptation, le rôle des paysans mieux formés au Bénin. IRDC-DFID; 2007; 4p.
- MAEP. Plan Stratégique de Développement du Secteur Agricole (PSDSA) 2025 et Plan National d’Investissements Agricoles et de Sécurité Alimentaire et Nutritionnelle PNIASAN 2017–2021; 2017; 139p.
- MAEP. Rapport de performance secteur agricole. Direction de la Programmation et de la Prospective). Ministère de l’Agriculture, de l’Élevage et de la Pêche; Cotonou, Bénin; 2010; 20 p.
- Yabi AJ, Bachabi FX, Labiyi AI, et al. Déterminants socio-économiques de l’adoption des pratiques culturales de gestion de la fertilité des sols utilisées dans la commune de Ouaké au Nord-Ouest du Bénin. Int J Biol Chem Sci. 2016;10(2):779–792.
- Djohy GL, Edja AH, Nouatin GS. Variation climatique et production vivrière: la culture du maïs dans le système agricole péri-urbain de la commune de Parakou au Nord-Benin. Afrique Sci. 2015;11(6):183–194.
- Yai ED, Ahodode BG, Biaou FC. Incidence du changement climatique sur les productions agricoles: cas de la commune de Banikoara. Outlook Agric. 2014;35(1):25–31.
- Kabore NP, Ouedraogo A, Some L, et al. Les facteurs determinants de la production de cereales seches en zone soudano-sahelienne du burkina faso [the driving factors of dry cereals production in the sudano-sahelian zone of burkina faso]. Int J Innov Appl Stud. 2015;11(1):214.
- PANA. Convention-cadre des Nations Unies sur les changements climatiques). Programme d’Action National d’Adaptation aux changements climatiques du Bénin (PANA- Bénin), MEPN; Cotonou, Bénin; 2008; 81 p.
- Savi MMOM, Yabi JA, Sodjinou E. Perception des variabilités climatiques des producteurs de maïs en relation avec les tendances climatiques au Nord-Bénin. Int J Biol Chem Sci. 2022;16(5):2174–2188.
- DGEC. Plan national d’adaptation aux changements climatiques du Bénin (PNA-Bénin); 2022; 175p.
- Akpo CY. Impact des semences améliorées sur la productivité du maïs au Bénin. African Economic Research Consortium, A final report Submitted to AERC, Biannual Research Workshop; 2020; p. 1–26.
- IPCC. Summary for Policymakers. Climate Change 2021: The Physical Science Basis. Contribution of Working Group I to the Sixth Assessment Report of the Intergovernmental Panel on Climate Change; Cambridge University Press, Cambridge, United Kingdom and New York, NY, USA; 2021; p. 3–32, doi:10.1017/9781009157896.001.
- Dossa FK, Miassi YE. Exploring the nexus of climate variability, population dynamics, and maize production in Togo: implications for global warming and food security. Farming Syst. 2023;1(100053):1–12.
- Casemir BH, Diaw A. Analysis of climate change effect on agricultural production in Benin. Asian J Agric Ext Economics Sociol. 2018;24:1–12.
- Miassi YE, Dossa KF. Forecasting animal protein supply in Asia and Europe in light of climate change, population growth and land pressure. Trop. Plant. 2023;2(22):1–15.
- Azontondé HA, Igué AM, Dagbénonbakin G. Carte de fertilité des sols du Bénin par zone agro-écologique du Bénin. Document validé à l’INFOSEC Cotonou le 10 août 2010). Rapport final, LSSEE/CRA-Agonkanmey/INRAB/Bénin; 2010; 128 p.
- Maliki R, Bernard M, Padonou E, et al. Effet combiné de NPK et de trois différents mulch d’origine végétale sur la production maïsicole et la fertilité des sols au Sud-Bénin. Bulletin de la Recherche Agronomique du Bénin (BRAB). 2017;1025-2355:1–17.
- Cesaro JD. Transformation des agricultures en Asie du Sud-Est: la paysannerie face aux défis de la mondialisation. Géoconfluences, dossier L'Asie du Sud-Est, carrefours et confins; 2020; 26p.
- FAO. Variétés de Semences Appropriées pour les Agriculteurs à Petite Échelle; 2014; 44p.
- Sakli. Modélisation et évaluation des vulnérabilités et des risques dans les chaînes logistiques. Thèse de Doctorat. Université d’Aix Marseille, École Doctorale mathématiques-informatique UFR Sciences. Laboratoire des sciences de l’information et des systèmes, UMR CNRS. 2016;729:1–214.
- Kuma J. Modélisation ARDL, Test de cointégration aux bornes et Approche de Toda-Yamamoto: Eléments de théorie et pratiques sur Logiciel, cel-01766214. Guide théorique et pratique; 2018; 55p.
- Bensbahou A, Seyagh I. L’émission de CO2 et la croissance économique: cas du Maroc. Revue Alternatives Managériales et Économiques. 2020;2(4):505–526.
- Mestiri S. La modélisation ARLD en utilisant logiciel R. Unité de recherche EAS-Mahdia Faculté des sciences économiques et de gestion de Mahdia). Conférence FSGE Mahdia; 2022; 18p.
- NASA. Le réchauffement climatique constitue désormais une menace existentielle de notre temps. Consulté le 15 Mai 2023; 2023.
- Badameli A, Dubreuil V. Diagnostic du changement climatique au Togo à travers l’évolution de la température entre 1961 et 2010. XXVIIIe Colloque de l’Association Internationale de Climatologie; Liège; 2015; p. 421–426.
- Djenontin SNI. Vulnérabilité des ressources en eau face aux changements climatiques et stratégies endogènes de gestion développées dans le secteur agricole: cas des communes de Banikoara et Malanville (Bénin). Thèse pour l'obtention du diplôme d'ingénieur agronome, Université de Parakou, Bénin; 2010.
- Dedjan YJ. Changements Climatiques et évolution des périodes de semis des principales cultures dans l'Alibori: cas des communes de Malanville et de Banikoara. Thèse pour l'obtention du diplôme d'ingénieur agronome, Université de Parakou, Bénin; 2010.
- Yegbemey RN, Yabi JA, Aïhounton GB, et al. Modélisation simultanée de la perception et de l'adaptation au changement climatique: cas des producteurs de maïs du Nord Bénin (Afrique de l'Ouest). Cah Agric. 2014;23(3):177–187.
- Miassi YE, Akdemir S, Dossa KF, et al. Technical efficiency and constraints related to rice production in West Africa: the case of Benin Republic. Cogent Food Agric. 2023;9(1):1–20.
- Avandé FL, Aboubacary IL, Hinnou LC. Stratégies d’adaptation des riziculteurs aux effets du changement climatique dans la commune de Malanville au Bénin. Agron Afr. 2022;34(3):387–402.
- PSDSA. Plan Stratégique de Développement du Secteur Agricole, Orientations stratégiques 2025, Plan National d’Investissements Agricoles et de Sécurité Alimentaire et Nutritionnelle PNIASAN, 2017–2021, Version finale; 2017; 132p.
- Salahuddin, M., Ali, M. I., Vink, N. et Gow, J., 2018. The effects of urbanization and globalization on CO2 emissions: evidence from the Sub-Saharan Africa (SSA) countries. Environ Sci Pollut Res, 2699–2709: 26.
- Balogan K.A., 2021. Effets de la consommation d'energie dans les transports, de l'urbanisation, des IDE et la croissance sur l’émission de CO2 dans l’UEMOA. Revues Économie, Gestion et Société, (1): 1-21.
- Edoun G. et Mongbo R., 2020. Les formes socioculturelles de la malnutrition des enfants de moins de cinq ans dans la commune de Karimama au Nord du Bénin. Eur Sci J. 16: 73–97.
- Dossa FK, Todota CT, Miassi YES, et al. Analyse comparée de la performance économique des cultures de coton et de maïs au Nord-Bénin: cas de la commune de Kandi. Int J Cur Innov Adv Res. 2018;1(6):118–130.
- Chandio AA, Gokmenoglu KK, Ahmad F. Addressing the long- and short-run effects of climate change on major food crops production in Turkey. Environ Sci Pollut Res. 2021;28:51657–51673.
- Kelthoum FM, Kelthoum O. Effet du traitement chimique et aérobie sur le rendement de PBM (Potentiel Biochimique Méthanogène) des déchets organiques du restaurant universitaire. Mémoire de master; 2017; 82 p.
- Adjovi NR, Agboton A, Quenum F, et al. Variation climatique et production vivrière au Sud-Bénin: cas de la commune de Bohicon. Afrique Sci. 2019;15(2):32–43.
- Demirhan H. dLagM: An R package for distributed lag models and ARDL bounds testing. PLoS One. 2020;15(2):e0228812.
- Tagwi A. The Impacts of Climate Change, Carbon Dioxide Emissions (CO2) and Renewable Energy Consumption on Agricultural Economic Growth in South Africa: ARDL Approach. Sustainability. 2022: 14. doi:10.3390/su142416468
- Sawadogo I, Kabore M, Koursangama A, et al. Dynamique à court terme et prévision des prix des céréales au Burkina-Faso: approche par la cointégration. En ligne]. In: Lankoande, Dr Damien, Quelle agriculture pour un développement durable de l’Afrique. 2010. p. 657–667.
- Ministère de l’Environnement, de l’Habitat et de l’Urbanisme Direction Générale de l’Environnement. Deuxième communication nationale de la république du Bénin sur les changements climatiques; 2021; 168p.
- Atchadé GA, Vissin EW, Gbenou P, et al. Cultures de contre-saison dans la commune d’adjohoun au Bénin: entre atouts et contrainte. Revue Ivoirienne de Géographie des Savanes. 2017;No 2:172–184.
- Dimon R. Adaptation aux changements climatiques: perceptions, savoirs locaux et stratégies d’adaptation développées par les producteurs des communes de Kandi et de Banikoara au Nord du Bénin. Thèse pour l'obtention du diplôme d'ingénieur agronome, Faculté des Sciences Agronomiques. Calavi, Bénin: Université d’Abomey; 2008; 132 p.
- Ibitola OR, Fasakin IJ, Popoola OO, et al. Determinants of maize farmers’ productivity among smallholder farmers in Oyo State, Nigeria. Greener J Agric Sci. 2019;9(2):189–198.
- Jouve P. Transition agraire: la croissance démographique, une opportunité ou une contrainte? Caire Info. 2006;No 217:43–54.
- Requier-Desjardins D. Démographie, agriculture familiale et développement rural en Amérique latine. Éléments pour un débat. Mondes en développement. 2018;182(2):87–114.
- Acloque D, Adam M, Ageron P, et al. Déprise. Géoconfluences. Ressources de géographie pour les enseignants; 2017.