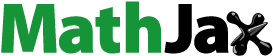
ABSTRACT
People reliant on agropastoral land systems are vulnerable to variability and changes in land function. Their vulnerability is linked to their exposure and sensitivity to various land system components and is often mediated by access to multiple livelihood capitals. We quantify aspects of that intersection with household vulnerability as measured by food insecurity, and as it is mediated by access to livelihood capitals. We use a structural equation model of household vulnerability, organized under a livelihoods framework, fitted empirically using a combination of information from 726 household surveys. These surveys were conducted in communities in Namibia, Botswana and Zambia, and are contained within a socio-ecological gradient in the Kavango-Zambezi Transfrontier Conservation Area of southern Africa. We provide evidence that adaptive capacity strongly mediates household vulnerability to food insecurity via both direct and indirect pathways that are statistically associated with land use-derived functions.
1. Introduction
1.1. Overview
Understanding how socio-environmental systems (SES) function at multiple scales is critical to advancing interdependent sustainability goals (B. Turner et al., Citation2016), global climate policy (Gupta et al., Citation2019) and land system science (B. L. Turner et al., Citation2007; Verburg et al., Citation2013). In regions where people are most directly linked to land system components, like through smallholder, rainfed agriculture and livestock production, there is a higher risk of exposure to environmental change and associated vulnerability in the context of food insecurity (Adger, Citation2006; Oluoko-Odingo, Citation2011; Williams et al., Citation2018). And while useful frameworks exist to conceptualize vulnerability within these broader system dynamics (Cutter & Finch, Citation2008; O’Brien & Leichenko, Citation2000; B. L. Turner et al., Citation2003), empirical research is often limited in its ability to quantify these dynamics in meaningful, policy-relevant ways (Liu et al., Citation2009; Morton, Citation2007; Pricope et al., Citation2020). This disconnect between concepts and quantitative data still stands as a central challenge to sustainable development, minimizing vulnerabilities and understanding and supporting local livelihoods in the context of global change (Carpenter et al., Citation2001; Fedele et al., Citation2019; Lynch et al., Citation2008).
Smallholders make adaptive decisions based on livelihood capacities and reflecting the land system and functions, or goods and services, available for use. As land use and environmental changes modify land systems, household vulnerability, as it relates to the most basic needs, can be affected (Viana et al., Citation2022). In this study, we define household vulnerability with respect to food insecurity, specifically using survey questions about household hunger, and by applying the Food Insecurity Experience Scale (FIES), an indicator adopted by the United Nations to measure Sustainable Development Goal 2 (end hunger) (Cafiero et al., Citation2018; Salerno et al., Citation2021). Capturing the dynamics between land functions and household-level food insecurity is a necessary step toward addressing interlinked Goalsets 2 (hunger) and 15 (land) of the United Nations Sustainable Development Goals (SDGs) (United Nations, Citation2015). The 2030 Agenda for Sustainable Development preamble emphasizes the need to reach those most vulnerable, globally, and in particular those in the least developed countries (United Nations, Citation2015). As such, we link interrelated concepts of sustainability and vulnerability in the context of livelihoods and land systems through an approach that quantitatively measures the components of land functions, household capacity and vulnerability as it relates to food insecurity (Gaughan et al., Citation2019).
Using multicountry household data and remotely sensed land systems information, we apply the approach in a dryland region of southern Africa. To characterize vulnerability to food insecurity in this region, we estimate an empirical model to interrogate the direct and indirect relationships linking exposure and sensitivity to environmental effects on land uses, households’ adaptive capacity to respond to systemic change and vulnerability to food insecurity. The strength and directional pathways between vulnerability components and associated indicators are presented in terms of our ability to identify different aspects within the system suitable for policy and management decision-making.
The following study includes a background review of the different salient theoretical components informing our understanding of the dryland system followed by an overview of the study region context and details of the structural equation modelling approach. We present outcomes of the model fitted to household data with a discussion that captures the geographic, statistical and hypothetical context. Finally, we provide suggestions for future research and how to enhance adaptive capacity and ultimately the sustainability of livelihoods in this critical region of southern Africa.
1.2. Conceptual framing: vulnerability, sustainability and land functions
1.2.1. Vulnerability framework and the link to land functions
Vulnerability is central to understanding interactions within socio-environmental systems where livelihoods are strongly connected to land use practices sensitive to environmental conditions (Janssen & Ostrom, Citation2006; B. L. Turner et al., Citation2003). Much work has been done to characterize vulnerability (Adger, Citation2006; Adger & Kelly, Citation1999; Luers, Citation2005), at various levels and scales (Brooks et al., Citation2005; Chaudhuri et al., Citation2002; Fraser et al., Citation2011), and often linked to concepts of exposure, sensitivity and adaptive capacity (Masson Delmotte et al., Citation2021; Smit & Wandel, Citation2006; B. L. Turner et al., Citation2003). In the context of the interrelated concepts of vulnerability, land functions and ecosystem service provisions, vulnerability is defined as the predisposition to be adversely affected by a climatic or environmental hazard/exposure and encompasses elements of sensitivity and the capacity, or lack thereof, to cope (Masson Delmotte et al., Citation2021).
We specifically conceptualize the combination of exposure and sensitivity as the land function aspect of our system. We define exposure in terms of the amount of land relied upon for services, while sensitivity is defined to be the overall amount of resources provided by the land utilized. The combination of exposure and sensitivity therefore captures both the land use and provision of associated goods and ecosystem services (land functions) (Verburg et al., Citation2009) for these primarily agropastoralist households. To establish linkages between the land function and social components of a system of interest, these components of vulnerability (exposure, sensitivity, and coping or adaptive capacity) can therefore be statistically related to empirical data (Fraser et al., Citation2011; Gaughan et al., Citation2019).
Research has shown that households and communities with established practices for risk mitigation, diversified livelihoods practices, strong social safety nets, access to financial and credit markets and robust governance structures tend to have greater adaptive capacity and therefore be less vulnerable to climate shocks and increasing climate and environmental variability (Wright et al., Citation2012). Less vulnerable communities with greater capacity to cope and adapt to changes and shocks also tend to be less reliant on a limited subset of subsistence activities (Morton, Citation2007) and be more food secure (Ali & Erenstein, Citation2017). Adaptive capacity can be measured through indicators derived from the sustainable livelihoods framework and pertaining to natural, human, social, financial or physical capitals (Scoones, Citation1998). In this study, we apply such an approach to further elucidate the household linkages between vulnerability to food insecurity, and the factors that contribute as a whole to potential features of adaptive capacity in the livelihoods framework.
2. Materials and methods
2.1. Study site – KAZA, transboundary conservation, and community-based organization
Our study focused on community conservation areas (CCA) in three of five nations (Botswana, Namibia and Zambia) of the Kavango Zambezi Transfrontier Conservation Area (hereafter KAZA). The KAZA incorporates mainly dryland savannas, but also encompasses major river channels with resource-rich floodplains important for both human livelihoods and wildlife. The region typically experiences strong climate variability (Morioka et al., Citation2015; Nash, Citation2017) but is also expected to experience increased warming, decreased rainfall,and an increase in extreme climate events (C. J. Engelbrecht & Engelbrecht, Citation2016).
The decision of what candidate environmental variables to include in the model was predicated on a systemic understanding of what variables would strongly correlate to one another and provide insight on the land functions associated with selected communities in KAZA. From an ecological standpoint, there is higher mean annual rainfall and a slightly longer rainy season across the study area’s semi-arid N-S gradient (). Zambian households experience a mean rainy season ~20 days longer than those communities in Botswana (~10 days longer than the Namibia households) which is significant for maize production, whose growing season is typically on a 90-day cycle (A1).
Figure 1. The (a) Kavango-Zambezi region with study areas and mean annual precipitation (mm) in southern Africa, (b) floodplain extent and (c) mean growing season in days with village areas noted for each country area.

Each CCA is influenced by the national policy context of its country, as well as its position along the precipitation and vegetation gradient from north to south (). At the same time, CCAs across KAZA countries share similar characteristics, with sparse, remote settlements and high global value in terms of biodiversity and ecosystem services, but providing marginal livelihood opportunities. Because households in these community areas are rural, they tend to rely heavily on subsistence activities – primarily livestock-keeping, crop production and natural resource harvesting (Bailey et al., Citation2020). Among households surveyed, we estimate the prevalence of moderate-to-severe food insecurity, as measured by FIES, at 71% (Salerno et al., Citation2021). As such, their susceptibility to climate shocks is high. Climate changes that affect rainy season length and frequency and intensity of rain events (Dunning et al., Citation2018), and increased temperatures (F. Engelbrecht et al., Citation2015), all affect the land functions underpinning rural livelihoods.
2.2. Empirically modelling the KAZa socio-environmental system
2.2.1. Modelling framework
To explore linkages in the KAZA system, we incorporate data from household surveys with physical variables derived from remotely sensed and modelled data (Funk et al., Citation2015; Nardi et al., Citation2019). We take advantage of platforms such as Google Earth Engine (Gorelick et al., Citation2017) and open-access data products to explicitly incorporate spatiotemporal conditions related to key environmental components of land function that correlate with food insecurity in KAZA. We model the system using a structural equation modelling (SEM) approach (Gaughan et al., Citation2019) that estimates the covariation between both measurable and unmeasurable, latent characteristics indicated by survey items and environmental data. The hypothesized relationships between these characteristics are tested and discussed.
2.3. Linking the modelling framework to empirical data
Based on a hypothetical structural and measurement model, reflecting a version of the sustainable livelihoods framework described in (Gaughan et al., Citation2019), we developed a survey instrument that included questions on land uses, household food security and various forms of natural, social and financial capital (Gaughan et al., Citation2019; Salerno et al., Citation2020). The generalized model was constructed using the fundamental concepts of a household’s vulnerability to food insecurity being influenced by that household’s reliance on land functions measured via exposure and sensitivity, and mediated by its adaptive capacity (). As described in the original framing (Gaughan et al., Citation2019), we initially hypothesized that these various household and land function characteristics were not directly measurable, but rather latent characteristics that could be indicated by various highly correlated measures, and as such would be suitable for modelling empirically using a SEM. The realization of the model presented in this study retains the latent construct for vulnerability and adaptive capacity but incorporates the land function nexus of exposure and sensitivity as directly measured covariates () to characterize specific land functional attributes related to agropastoral land uses. While the system theorization outlined in (Gaughan et al., Citation2019) helped to guide survey design and questions (), certain data and statistical constraints made it less tenable to include latent measures for exposure and sensitivity. See Appendix 1 (Appendix 1A1) for further detail and to access open data to reproduce all analyses and results (Stevens, Citation2022).
Figure 2. Hypothesized household-level vulnerability and its components, directly and indirectly associated with land functions-related exposure and sensitivity, which are mediated by adaptive capacity (solid blue (positive) and red (negative) arrows). These components are linked in the theoretical formulation of what constitutes household vulnerability in the context of food insecurity; arrows and their thickness represent hypothetical, quantitative associations between vulnerability components as conceptualized by Gaughan et al. (Citation2019).

Table 1. Indicator variables and associated descriptions of the SEM.
2.4. Household survey design and sampling frame
A stratified and systematic random sample of 726 households across 15 villages was taken over two field seasons in 2017 and 2018. Five villages in each of the three CCAs were selected for approximately 48 households to be interviewed each. CCAs and representative villages provide a complementary comparison in terms of several key aspects that vary across KAZA. Governance and land tenure are established independently by each nation, and each CCA is constrained by a different national community-based conservation policy; biophysical conditions vary from savanna woodlands to seasonal floodplain; socio-economic characteristics are influenced by distance to market and degree of integration into the cash economy, among other factors. In Botswana, we surveyed five villages in Chobe Enclave, with a population of approximately 3,800 people. In Namibia, we chose the Mashi Conservancy and its five associated communities who together number ~2,900 residents. Finally, in Zambia, we selected the southern half of the Lower West Zambezi Game Management Area (GMA), where five villages are located, with the population of the entire area being around 10,000 (Salerno et al., Citation2021).
Our sample size of approximately 240 households per CCA was based on an equal allocation of 48 surveys per village – a village-level sample size sufficient to allow assumptions of normality of distribution and equal variance (Agresti & Finlay, Citation2009). This allowed for a robust pooled sample for the overall study (Botswana N = 240, Namibia N = 241 and Zambia N = 245, total N = 726). A full description of the survey design and approach is given in (Salerno et al., Citation2020).
2.5. Structural equation model specification
The original survey questions that inform the hypothetical framing were crafted based on the strong assumption that there exists a population comprising households across all three countries in the study that employ a variety of land uses, and were comparable between regions in how land functions influenced food security. As noted above, we use a SEM, an analytical technique that combines factor analysis and multiple regression to quantify relationships between one or more independent variables and one or more dependent variables (Ullman & Bentler, Citation2012). The general process of SEM is to translate theoretical constructs into modelled relationships during model specification (Asah, Citation2008; Hoyle, Citation2014), a process we define as ‘operationalizing’ our theoretical understanding of vulnerability in the KAZA socioecological system. One advantage of pre-specifying the model and fitting it using the SEM framework is that results can be discussed in the context of that theoretical framing. In KAZA, we hypothesize that those households with a higher diversification in livelihood activities (Adaptive Capacity) and subsequently less dependence on land use outcomes (Sensitivity) will be less food insecure and have a lower vulnerability measure ().
Figure 3. Proposed structure and measurement model of the KAZA socioecological system, as operationalized using latent and measurable components within a structural equation modelling framework. Hypothesized (expected) relationships between structural model components are presented as coloured, directional arrows. These are the findings expected prior to fitting our model with empirical data.

To best characterize food insecurity through the latent construct of V, we apply a set of questions directed towards identifying varying levels of food insecurity for each household. We chose to use questions that asked about food availability, food choice and preference, specific temporal aspects to experiencing hunger and other questions that were comparable to previously conducted surveys from the region in order to conduct longitudinal data analyses, A subset of these questions were used to construct the specified indicators and FIES estimates presented here () (Salerno et al., Citation2021). To indicate the realized but unmeasured ability of a household to cope or adapt to environmental conditions regarding food insecurity, we included questions based on the sustainable livelihoods framework (Fraser et al., Citation2011). Each measured indicator predicted by AC was an intentionally selected metric for identifying various financial, human, social and physical capitals of a household (Fraser et al., Citation2011). Natural capital is included through inherent aspects of the ‘Crops Harvested’ and ‘Grazers Born’ covariates, as well as conditions associated with soil and water quality indirectly captured through our agropastoral land function nexus.
Other potential data source inclusions for natural capital, such as survey data on gathered natural resources, did not associate strongly with food insecurity or agricultural land, so they were not included in the model. Together, ‘Crops Harvested’ and ‘Grazers Born’ represent the sensitivity of households exposed to environmental land use conditions related to our exposure measures of hectares cropped, rainy season length and the location of a household relative to the nearest floodplain. outlines the full set of indicators and covariates, including their data source, transformation and most basic descriptive statistics.
The specified model presented here is only one of many theoretically similar model specifications that are possible. While a model can be formulated that mirrors the one presented using latent constructs for each vulnerability component laid out by Gaughan et al. (Citation2019), we found that hypothetical indicators of exposure and sensitivity components were not correlated (e.g. gathering of natural resources of different types were unrelated to other household land use activities) and operationally did not fit a measurement model appropriate for inclusion in the final model specification (see A1). While statistically better fitting models can be found by data manipulation and model re-specification using post-hoc covariate and indicator selection, some of which are still theoretically aligned with the model presented here (A1), we choose to present the specification that, while having marginal statistical significance, best represents the a priori theoretical framework while still being estimable.
The specified model () was fit using the lavaan package (Rosseel, Citation2012) in the R Statistical Computing environment (R Core Team, Citation2020). The number of surveys across the communities from each country that contained no missing data was adequate for fitting covariance matrix-based models such as in SEM. Our total sample size (N = 726) included missing responses to capital and land use questions for 96 households, and in two cases, incorrect geocoding which complicated environmental data extraction from remotely sensed data sources. Given that these missing data were not at random, we ruled out data imputation methods and chose to use only the N = 628 complete cases for modelling. Using the described covariates () the model was fit using diagonally weighted least squares and maximum likelihood estimation with robust standard errors (i.e. mean- and variance-adjusted test statistics (Rosseel, Citation2012).
3. Results
3.1. Household survey summaries
The basic correlation structure was tested between covariates and indicators from (). These estimates show general trends that are consistent with hypothesized relationships across the hypothetical groupings of covariates. More detailed descriptions and visualizations can be found in A1 with variable transformations, model specification and fitting details (A1, ). Focusing on the clusters of variables used as indicators for the unmeasured, latent constructs of vulnerability and adaptive capacity (), we observed relatively high correlation among indicators for vulnerability and relatively low internal correlation among indicators of adaptive capacity.
Figure 4. Correlation matrix of covariates used in the specified and fitted SEM. Variable names reflect the measurement and structural components of the model followed by an underscore and the general nature of the survey data used to construct each variable (Table 1).

It should be noted that as a group the vulnerability indicators should be extremely highly correlated since they consist primarily of two questions about monthly food availability, asking respondents about the current year of their interview and an average year in general (). The composite FIES score is calculated in part from these questions () but also includes finer grained questions on nutrition and food preference (Cafiero et al., Citation2018; Salerno et al., Citation2021).
The adaptive capacity indicators correlate less strongly with each other as they represent very different forms of capital (human and financial), but as a group, tend to negatively covary with the exposure covariates and positively with sensitivity covariates. The social connection composite index (A_Social) is only weakly correlated with other adaptive capacity indicators, and shows that as a whole, there is higher covariance among those components that are more directly linked to labor, assets and financial assets.
There was significant variation between communities of different countries in overall crop yields, especially with respect to maize, the most economically important and vital subsistence crop across the study area. The total amount of maize yields per hectare in the household data is highest in Zambia ( 603.4 kg/Ha, SD = 782.8 kg/Ha) compared to Namibia (
254.7 kg/Ha, SD = 766.9 kg/Ha) or Botswana (
402.0 kg/Ha, SD = 880.8 kg/Ha), and yet Botswanan households overall have the highest cropped area reported (
3.74 Ha, SD = 7.47, Namibia:
1.90 Ha, SD = 2.14, Zambia:
2.02 Ha, SD = 2.76).
3.2. Structural model development
The fitted measurement model, the estimated latent constructs and the specified structural components are presented in the attached Appendix (A1, ), alongside their robust standard errors, z-scores and associated p-values at the ɑ = 0.05 level. All coefficient and variance estimates are presented there in unstandardized form, with units reflecting those specified in . The overall model fit, by the most commonly reported fit indices (), should be considered statistically marginal and indicates that the model, while interpretable, may be improved by re-specification. We present these results without such re-specification since we pre-described the framework with a priori hypothetical relationships, and fit it with minimal adjustments, considering only the appropriateness of the components included in the measurement model (A1). The fitted model produces an estimated variance-covariance matrix which is compared statistically against the observed covariances of the empirical data included. The overall, robust χ2 (723.37, d.f. = 56, p < 0.01) should be considered a ‘badness-of-fit,’ and a statistically significant result indicates that the overall covariances do not statistically agree. This metric is, however, very sensitive to sample sizes. We therefore also present the Comparative Fit Index (CFI) (>0.90 is considered ‘well-fitting’), the Tucker-Lewis Index (TLI) (>0.80 is ‘well-fitting’) and the Root Mean Square Error of Approximation (RMSEA) (<0.12 is ‘well-fitting’). All of these fit measures indicate a marginal model fit with statistical properties that leave room for improvement in both the measurement and structural model. The Goodness-of-Fit Index (GFI) and Adjusted Goodness-of-Fit Indices (AGFI) (>0.9 is ‘well-fitting’) can be interpreted as the proportion of variance accounted for by the estimated population covariance, analogous to R2 in a linear regression context, and both indicate a significant portion of the overall variance is explained by the hypothesized relationships; however, room remains for model improvement (~10%) (Kline, Citation2011).
Figure 5. Fitted SEM with visualized standardized coefficient values and strengths of associations. Arrows for structural relationships are color coded red (negative) and blue (positive), with measurement model loadings and coefficient weights scaled to relative widths.

The measurement model and the relationships of the indicators with their latent constructs of vulnerability and adaptive capacity are presented in standardized form in . The strength of the correlations among indicators for each construct () is reflected in the relative strength of each indicator and its ‘prediction’ by the unobserved construct. The covariance of the first indicator is fixed to one for each of the constructs of adaptive capacity and vulnerability, respectively, while the standardized values presented in reflect the relative strength of the correlation with the estimated factor scores of each latent variable. In this case, the ‘Durable Assets Index’ and ‘Highest Education of Any Household Member’ variables are most strongly predicted by the resulting latent construct of adaptive capacity, while the ‘FIES Score’ and number of months of food insecurity experienced in the last 12 months are most strongly associated with the vulnerability latent variable.
3.3. Exploration of factor scores and covariates
Another SEM output that lends itself to interrogate hypothetical relationships is the factor scores estimated for latent constructs. For these latent variables, the factor scores themselves represent an estimate for each household that is predictive of each individual indicator variable used in the measurement model. We visualize the relationship between factor scores estimated for each household (), and categorized by the household’s country location. These illustrate the structural association, with an estimated direct coefficient of −2.70 (−0.47 standardized) (p-value <0.01), and the combined indirect pathways that result in the observed traced effect of adaptive capacity on vulnerability (−0.67) (). The breakdown by household countries illustrates important country-level differences that are indirectly accounted for in the model specification and partially controlled for by environmental covariates.
4. Discussion
Our findings from the estimated model suggest that variation in vulnerability to food insecurity is most strongly associated with variation in households’ adaptive capacity (). This linkage is via both direct and indirect pathways mediated through land use, specifically grazing productivity (). The combined effects of the agropastoral land function nexus (via exposure and sensitivity), while statistically significant, are less informative than adaptive capacity for understanding the variability in household vulnerability in the studied communities. Components that we hypothesized would indicate household adaptive capacity significantly covary, but the measured forms of social capital were less strongly associated with the latent construct than physical and other aspects of human capital. These results may reflect a more nuanced influence of how social relationships in our study area communities might impact food security or how social ties vary differently in their effect on vulnerability between the cultural contexts of villages, conservancies and countries represented.
Despite being less influential than adaptive capacity in explaining variation in vulnerability, another important aspect highlighted in our results is the relationship between environmental covariates (mean rainfall season length and distance of household to floodplain) and variation in land use activities. These form the basis for further discussion regarding how environmental and ecological conditions affect agropastoral land functions, and how these pathways exacerbate or mediate household vulnerability to food insecurity. As such, we present KAZA-specific insights relevant to our contextualization of this dryland system (6.1 to 6.4) and then focus on generalizable results for how this research contributes to the broader conversation of sustainable development, household vulnerability and rural land systems (6.5).
4.1. Land function reliance and vulnerability
We found that KAZA households that engage in more extensive cropping also produce on average more crops but also have higher vulnerability to food insecurity. This at first may seem counterintuitive but aligns with the conceptual framing that while controlling for other forms of capital, like those represented in adaptive capacity, a reliance on increased levels of cropping is significantly associated with higher levels of vulnerability. Furthermore, adaptive capacity is not a significant predictor of crop productivity, which is a rationale supporting the conceptual framing that agriculture represents a different type of component contributing to vulnerability than adaptive capacity. Therefore, for agricultural land functions, we would argue that cropping and grazing do align with a conceptual framing around exposure and sensitivity.
Grazing and cattle production in the KAZA context, like other areas of sub-Saharan Africa, may be less aligned with our conceptual framing of the exposure-sensitivity and land function nexus. We found that adaptive capacity is statistically and significantly positively associated with cattle production, and that higher cattle production is associated with lower levels of vulnerability (). In this context, cattle ownership and their yield may empirically act more as a buffer to food insecurity-related vulnerability, and that despite grazing land uses that support cattle production, and the land function that represents being statistically important, it does not fit our hypothesized positive relationship between exposure and sensitivity components and vulnerability (Gaughan et al., Citation2019, ).
4.2. Environmental and geographic specificity
With regard to components that interact with land uses (exposure) and their outcomes (sensitivity), the model shows a statistically significant, positive association with the mean rainy season length and the amount of crops harvested, meaning that the shorter the rainy season length, the less total harvested crops. However, the opposite statistical relationship is found with grazers born, indicating that a shorter rainy season length associates with more livestock born. The productivity in crops and grazers cannot be disentangled completely from other environmental (e.g. crop and livestock depredation, flooding) and geographic (e.g. cultural variability, accessibility to communal herding and grazing resources, etc.) factors, and further research will focus on such complexity.
Indeed, distance to the floodplain is positively associated with crops harvested, suggesting that the farther away an area is from the river, the higher the crop production. The increased land area for cropping in Botswana may indicate a need to have more area for farming when molapo (floodplain) fields are flooded. However, the large range of variability in these estimates means that caution should be taken when interpreting environmental variables. Furthermore, more household data were available to facilitate a more complex model, other potentially important distance-based and regionally varying covariates such as accessibility to forest vs. open woodland, for example, may also be useful to include and help differentiate between household reliance on natural resource gathering and cropping land uses.
4.3. Institutional and country-specific considerations
Although for the SEM we treated all households as being from the same population, it is clear that there are country-level differences in both adaptive capacity and vulnerability (), with households in Botswana better off, and those in Zambia worse off with respect to food insecurity (). These broad trends parallel differences at the national level: Botswana, closely followed by Namibia, is reported to have more effective governance than Zambia (Mo Ibrahim Foundation, Citation2020); the same pattern exists for human development indices (Roser, Citation2014) and per capita GDP (WorldBank, Citation2022). At the same time, there is clearly considerable within-CCA variation. At a coarse level, our data would support suggestions that Zambia was in greater need of interventions; however, the SEM is able to identify households within each CCA that have less adaptive capacity, as well as the leverage points that could best increase this so that such interventions could be more specifically targeted.
Figure 8. Vulnerability factor scores by country vs. the amount of collected, non-firewood resources across study households.

Given the spatial focus on CCAs, something further must be said about the state of development of community conservation in each country. While both Botswana and Namibia have well-supported CBNRM programs and associated healthy wildlife populations, with Botswana in particular seeing high income at community level from wildlife-related tourism activities, Zambia’s program currently exists in name only, with few – if any – activities or projects on the ground within the communities of our study, and a relative paucity of wildlife to the Namibian and Botswanan contexts. When considering the intention of CCAs to protect biodiversity and ecosystem services, in Zambia there does appear to be a higher reliance on at least provisioning services, whereas that purpose may be more externalized in Botswana and Namibia (Bailey et al., Citation2020). This is reflected in the constrained nature of which land functions are represented in the specified model presented here.
4.4. Natural resource capital
Having only agropastoral land uses and aspects of their yield captured in the exposure and sensitivity components was limiting to addressing land function more broadly in the KAZA context. We initially considered natural sources of capital beyond simply cropping and grazing as being integral to households’ exposure and sensitivity. However, natural resources, both edible and non-edible, were nearly ubiquitously gathered and relied upon by households regardless of context, in addition to there being country-specific differences in the availability of woody biomass for fuel and the uses of those resources for burning directly (in Namibia and Botswana) vs. charcoal production (Zambia). These facts challenged assumptions about the common population across countries and how variability in access to natural resources might help explain variations in household food insecurity ().
What is clear is that there is much variability in the numbers of resources collected and the amounts of those resources, particularly gathered edible foods (A1). Understanding the role of harvested natural resources in rural livelihoods requires substantial unpacking, such as which households are relying on such resources primarily for subsistence use, and which might be harvesting commercially, potentially as part of a suite of livelihood activities. There is also much to understand about what proportion of a total livelihood basket that natural resources might contribute for different households. The expected importance of the resources is there, and future research will look at these aspects.
4.5. Applications to sustainable development metrics
In the 2030 Agenda for Sustainable Development, food and agriculture are key components for addressing various interrelated SDGs. Rural and agricultural developments, especially in subsistence communities, are the cornerstone for ending poverty and staving off hunger (SDG 2) while concurrently contributing to sustainable development, generally (Del Arco et al., Citation2021). Our study illustrates one potential use of the internationally comparable FIES score (the survey module for SDG indicator 2.1.2) as a tool in constructing a quantitative measurement scale of the severity of the food insecurity conditions in rural, subsistence-based communities (Cafiero et al., Citation2018). When we integrate food insecurity metrics (FIES, number of months food insecure in an average or previous year) with data on livelihoods composition as proxies for adaptive capacity and land function proxies, we unveil important dimensions in food insecurity-related vulnerability, both among and across households from communities in three socio-economically and politically differing countries. Thus, by integrating measures of vulnerability, sensitivity, exposure and adaptive capacity in a quantitative model, we can contribute to identifying actionable outcomes for policy interventions and argue that this need for integration is more urgent in semiarid, marginally productive landscapes. Where land use pressures overlap with already marginal environments characterized by poor soils and high climatic variability, household-level vulnerability can further influence household decisions and land use outcomes (Tsegaye et al., Citation2010). Households that diversify their livelihoods as an adaptation strategy will have lower overall vulnerability, greater adaptability (Cassidy & Barnes, Citation2012; Shackleton & Shackleton, Citation2006) and face less food insecurity (Richardson et al., Citation2018).
That said, a key empirical result from our study is the significant in-country variation in vulnerability and adaptive capacity which suggests that targeting broad interventions is not enough to reach the most vulnerable. The diversity of livelihood activities within KAZA varies, especially for livestock and crop production. Since these are not coupled land activities, either distinct interventions need to be applied or it may be necessary to untangle livestock–wealth relationships, meaning to what degree people rely on livestock for subsistence versus commercial use. Our model indicates that when adaptive capacity scores are relatively higher and associated vulnerability scores relatively lower, while controlling for that standardized scores of exposure and sensitivity components, households reflect lower reliance on agropastoral land functions overall. One potential piece of support for this finding may lie in the broad social services, as evidenced in Botswana, for farming or resource gathering households where productivity is severely limited. Using the FIES as a component in household vulnerability modelling, we 1) provide a tractable way to extrapolate findings in comparison with larger rural lands context and 2) provide additional building blocks for insight on the importance of rural land functions and livelihoods at local scales in achieving SDGs and particularly outcome 2.2.1 for reducing hunger globally.
5. Conclusions
Our work underscores the context-dependent nature of measuring and modelling vulnerability and highlights the need to account for both environmental and socio-economic variables at scales relevant for decision-making at the household level. The capacity of rural, subsistence-based households to adapt to, react and prepare for climatic and environmental variability and changes can influence SES resilience at the community level (Selm et al., Citation2018). Dimensions and aggregated indices of social, physical, human, financial and natural capitals that support livelihoods can be used effectively to approximate measures of adaptive capacity among different households, and when appropriately analysed across political and environmental gradients, can point to leverage points where effective interventions may be suggested. In modelling these responses and associations in a transboundary, mixed-land use, subsistence context, limitations pertaining to data comparability, survey response standardization, data granularity and ability to statistically link environmental and socio-economic variables in a sustainable livelihoods framework persist and future work is needed to address these limitations. We acknowledge that complex relationships among the various livelihood capitals may confound our ability to measure financial, physical and human capitals separately and hence to identify leverage points to effectively influence household vulnerability. At the same time, our model shows that while the details of local livelihood capital assemblages may be both location-specific and complex, the approach of linking such indicator-based capitals to the latent characteristics of vulnerability and adaptive capacity through a structural equation model allows for generalization of the relationships to other social and geographical contexts. As such, we posit that strategies to increase household adaptive capacity and decrease vulnerability to food insecurity in the KAZA gradient landscape might include crop diversification as a measure of increasing food security, which is associated with a decrease in total cropped area devoted solely to maize. In addition, investment in durable assets, educational and health outcomes of workers and creation of income-generating opportunities that do not rely on welfare may be critical leverage points. All of these are important indicators in our model of overall adaptive capacity, which empirically has the strongest net association with lower food insecurity-related vulnerability for households in KAZA.
Thank you to the communities of Zambezi Region, Namibia and Chobe Enclave in Botswana. Without their willingness to engage in the research study, this type of work would not be possible. We also thank the KAZA-TFCA Secretariat, the University of Namibia, the Integrated Rural Development and Nature Conservation, the Department of National Parks and Wildlife in Zambia, and Dr Patricia Mupeta-Muyamwa for their insight, collaboration and support of our work. We also thank the external reviewers for the thoughtful and useful critiques for improving the final version of this paper. Research protocols followed recognized codes of conduct and were approved by community leadership, Traditional Authorities, national research councils and the University of Colorado Institutional Review Board (#16-0126).
Supplemental Material
Download MS Word (672 KB)Disclosure statement
No potential conflict of interest was reported by the author(s).
Supplementary material
Supplemental data for this article can be accessed online at https://doi.org/10.1080/1747423X.2023.2226140
Additional information
Funding
References
- Adger, W.N. (2006). Vulnerability. Global Environmental Change, 16(3), 268–281. https://doi.org/10.1016/j.gloenvcha.2006.02.006
- Adger, W.N., & Kelly, P.M. (1999). Social vulnerability to climate change and the architecture of entitlements. Mitigation and Adaptation Strategies for Global Change, 4(3), 253–266. https://doi.org/10.1023/A:1009601904210
- Agresti, A., & Finlay, B. (2009). Statistical Methods for the Social Sciences. Pearson. https://www.pearson.com/content/one-dot-com/one-dot-com/us/en/higher-education/product.html
- Ali, A., & Erenstein, O. (2017). Assessing farmer use of climate change adaptation practices and impacts on food security and poverty in Pakistan. Climate Risk Management, 16, 183–194. https://doi.org/10.1016/j.crm.2016.12.001
- Asah, S.T. (2008). Empirical social-ecological system analysis: From theoretical framework to latent variable structural equation model. Environmental Management, 42(6), 1077–1090. https://doi.org/10.1007/s00267-008-9172-9
- Bailey, K.M., Drake, M.D., Salerno, J., Cassidy, L., Gaughan, A.E., Stevens, F.R., Pricope, N.G., Woodward, K.D., Luwaya, H.M., & Hartter, J. (2020). Mapping natural resource collection areas from household survey data in Southern Africa. Applied Geography, 125, 102326. https://doi.org/10.1016/j.apgeog.2020.102326
- Brooks, N., Neil Adger, W., & Mick Kelly, P. (2005). The determinants of vulnerability and adaptive capacity at the national level and the implications for adaptation. Global Environmental Change, 15(2), 151–163. https://doi.org/10.1016/j.gloenvcha.2004.12.006
- Cafiero, C., Viviani, S., & Nord, M. (2018). Food security measurement in a global context: The food insecurity experience scale. Measurement, 116, 146–152. https://doi.org/10.1016/j.measurement.2017.10.065
- Carpenter, S., Walker, B., Anderies, J.M., & Abel, N. (2001). From metaphor to measurement: Resilience of what to what? Ecosystems (New York), 4(8), 765–781. https://doi.org/10.1007/s10021-001-0045-9
- Cassidy, L., & Barnes, G.D. (2012). Understanding household connectivity and resilience in marginal rural communities through social network analysis in the village of habu, Botswana. Ecology and Society, 17 4 https://www.jstor.org/stable/26269205. https://doi.org/10.5751/ES-04963-170411
- Chaudhuri, S., Jalan, J., & Suryahadi, A. (2002). Assessing household vulnerability to poverty from cross-sectional data: A methodology and estimates from Indonesia. Columbia University Department of Economics Discussion Papers. 0102–52. https://doi.org/10.7916/D85149GF
- Cutter, S.L., & Finch, C. (2008). Temporal and spatial changes in social vulnerability to natural hazards. Proceedings of the National Academy of Sciences, 105(7), 2301–2306. https://doi.org/10.1073/pnas.0710375105
- Del Arco, I., Ramos-Pla, A., Zsembinszki, G., de Gracia, A., & Cabeza, L.F. Implementing sdgs to a sustainable rural village development from community empowerment: Linking energy, education, innovation, and research. (2021). Sustainability, 13(23), 12946. Article 23. https://doi.org/10.3390/su132312946
- Douxchamps, S., Van Wijk, M.T., Silvestri, S., Moussa, A.S., Quiros, C., Ndour, N.Y.B., Buah, S., Somé, L., Herrero, M., Kristjanson, P., Ouedraogo, M., Thornton, P.K., Van Asten, P., Zougmoré, R., & Rufino, M.C. (2016). Linking agricultural adaptation strategies, food security and vulnerability: Evidence from West Africa. Regional Environmental Change, 16(5), 1305–1317. https://doi.org/10.1007/s10113-015-0838-6
- Dunning, C.M., Black, E., & Allan, R.P. (2018). Later wet seasons with more intense rainfall over Africa under future climate change. Journal of Climate, 31(23), 9719–9738. https://doi.org/10.1175/JCLI-D-18-0102.1
- Engelbrecht, F., Adegoke, J., Bopape, M.-J., Naidoo, M., Garland, R., Thatcher, M., McGregor, J., Katzfey, J., Werner, M., Ichoku, C., & Gatebe, C. (2015). Projections of rapidly rising surface temperatures over Africa under low mitigation. Environmental Research Letters, 10(8), 085004. https://doi.org/10.1088/1748-9326/10/8/085004
- Engelbrecht, C.J., & Engelbrecht, F.A. (2016). Shifts in Köppen-Geiger climate zones over southern Africa in relation to key global temperature goals. Theoretical and Applied Climatology, 123(1), 247–261. https://doi.org/10.1007/s00704-014-1354-1
- Fedele, G., Donatti, C.I., Harvey, C.A., Hannah, L., & Hole, D.G. (2019). Transformative adaptation to climate change for sustainable social-ecological systems. Environmental Science & Policy, 101, 116–125. https://doi.org/10.1016/j.envsci.2019.07.001
- Fraser, E.D.G., Dougill, A., Hubacek, K., Quinn, C., Sendzimir, J., & Termansen, M. (2011 3). Assessing vulnerability to climate change in dryland livelihood systems: Conceptual challenges and interdisciplinary solutions. Ecology and Society, 16. https://doi.org/10.5751/ES-03402-160303
- Funk, C., Peterson, P., Landsfeld, M., Pedreros, D., Verdin, J., Shukla, S., Husak, G., Rowland, J., Harrison, L., Hoell, A., & Michaelsen, J. (2015). The climate hazards infrared precipitation with stations—A new environmental record for monitoring extremes. Scientific Data, 2(1). Article 1. https://doi.org/10.1038/sdata.2015.66.
- Gaughan, A.E., Stevens, F.R., Pricope, N.G., Hartter, J., Cassidy, L., & Salerno, J. Operationalizing vulnerability: land system dynamics in a transfrontier conservation area. (2019). Land, 8(7), 111. Article 7. https://doi.org/10.3390/land8070111
- Gorelick, N., Hancher, M., Dixon, M., Ilyushchenko, S., Thau, D., & Moore, R. (2017). Google earth engine: Planetary-scale geospatial analysis for everyone. Remote Sensing of Environment, 202, 18–27. https://doi.org/10.1016/j.rse.2017.06.031
- Gupta, A.K., Negi, M., Nandy, S., Alatalo, J.M., Singh, V., & Pandey, R. (2019). Assessing the vulnerability of socio-environmental systems to climate change along an altitude gradient in the Indian Himalayas. Ecological Indicators, 106, 105512. https://doi.org/10.1016/j.ecolind.2019.105512
- Hoyle, R.H. (2014). Handbook of Structural Equation Modeling. Gulford Press. https://www.guilford.com/books/Handbook-of-Structural-Equation-Modeling/Rick-Hoyle/9781462516797
- Ibrahim Foundation, M. (2020). Ibrahim Index of African Governance.
- Janssen, M., & Ostrom, E. (2006). Empirically Based, Agent-based models. Ecology and Society, 11(2). https://doi.org/10.5751/ES-01861-110237
- Kline, R.B. (2011). Principles and Practice of Structural Equation Modeling (3rd ed. ; Eds., D. A. Kenny & T. D. Little.). The Guilford Press.
- Liu, X., Wang, Y., & Peng, J. (2009). Progress in vulnerability analysis of coupled human-environment system. Advances in Earth Science, 24(8), 917. https://doi.org/10.11867/j.issn.1001-8166.2009.08.0917
- Luers, A.L. (2005). The surface of vulnerability: An analytical framework for examining environmental change. Global Environmental Change, 15(3), 214–223. https://doi.org/10.1016/j.gloenvcha.2005.04.003
- Lynch, A.H., Tryhorn, L., & Abramson, R. (2008). Working at the boundary: Facilitating interdisciplinarity in climate change adaptation research. Bulletin of the American Meteorological Society, 89(2), 169–179. https://doi.org/10.1175/BAMS-89-2-169
- Masson Delmotte, V.P., Zhai, P., Pirani, A., Connors, S.L., Pean, C., Berger, S., Caud, N., Chen, L., Goldfarb, M.I., Gomis, M., Huang, K., Leitzell, E., Lonnoy, J.B.R., Matthews, T.K., Maycock, T.K., Waterfield, T., Yelekei, R., Yu, R., & Zhou, B. (2021). The physical science basis. contribution of working group i to the sixth assessment report of the intergovernmental panel on climate change. In Climate Change. IPCC, 2021: Summary for Policymakers IPCC, 2021: Summary for Policymakers (pp. 3–32). Cambridge University Press. https://doi.org/10.1017/9781009157896.001
- Morioka, Y., Engelbrecht, F., & Behera, S.K. (2015). Potential sources of decadal climate variability over southern africa. Journal of Climate, 28(22), 8695–8709. https://doi.org/10.1175/JCLI-D-15-0201.1
- Morton, J.F. (2007). The impact of climate change on smallholder and subsistence agriculture. Proceedings of the National Academy of Sciences, 104(50), 19680–19685. https://doi.org/10.1073/pnas.0701855104
- Nardi, F., Annis, A., DiBaldassarre, G., Vivoni, E.R., & Grimaldi, S. (2019). Gfplain250m, a global high-resolution dataset of Earth’s floodplains. Scientific Data, 6(1). Article 1. https://doi.org/10.1038/sdata.2018.309.
- Nash, D. (2017, February 27). Changes in precipitation over southern Africa during recent centuries. Oxford Research Encyclopedia of Climate Science. https://doi.org/10.1093/acrefore/9780190228620.013.539
- O’Brien, K.L., & Leichenko, R.M. (2000). Double exposure: Assessing the impacts of climate change within the context of economic globalization. Global Environmental Change, 10(3), 221–232. https://doi.org/10.1016/S0959-3780(00)00021-2
- Oluoko-Odingo, A.A. (2011). Vulnerability and adaptation to food insecurity and poverty in kenya. Annals of the Association of American Geographers, 101(1), 1–20. https://doi.org/10.1080/00045608.2010.532739
- Pricope, N.G., Cassidy, L., Gaughan, A.E., Salerno, J.D., Stevens, F.R., Hartter, J., Drake, M., & Mupeta-Muyamwa, P. (2020). Addressing integration challenges of interdisciplinary research in social-ecological systems. Society & Natural Resources, 33(3), 418–431. https://doi.org/10.1080/08941920.2019.1680783
- R Core Team. (2020). R: A Language and Environment for Statistical Computing. R Foundation for Statistical Computing. https://www.R-project.org/
- Richardson, K.J., Lewis, K.H., Krishnamurthy, P.K., Kent, C., Wiltshire, A.J., & Hanlon, H.M. (2018). Food security outcomes under a changing climate: Impacts of mitigation and adaptation on vulnerability to food insecurity. Climatic Change, 147(1), 327–341. https://doi.org/10.1007/s10584-018-2137-y
- Roser, M. (2014). Human Development Index (HDI). Our World in Data. https://ourworldindata.org/human-development-index
- Rosseel, Y. (2012). Lavaan: An R package for structural equation modeling. Journal of Statistical Software, 48(2), 1–36. https://doi.org/10.18637/jss.v048.i02
- Salerno, J., Bailey, K., Gaughan, A.E., Stevens, F.R., Hilton, T., Cassidy, L., Drake, M.D., Pricope, N.G., & Hartter, J. (2020). Wildlife impacts and vulnerable livelihoods in a transfrontier conservation landscape. Conservation Biology, 34(4), 891–902. https://doi.org/10.1111/cobi.13480
- Salerno, J., Stevens, F.R., Gaughan, A.E., Hilton, T., Bailey, K., Bowles, T., Cassidy, L., Mupeta-Muyamwa, P., Biggs, D., Pricope, N., Mosimane, A.W., Henry, L.M., Drake, M., Weaver, A., Kosmas, S., Woodward, K., Kolarik, N., & Hartter, J. (2021). Wildlife impacts and changing climate pose compounding threats to human food security. Current Biology, 31(22), 5077–5085.e6. https://doi.org/10.1016/j.cub.2021.08.074
- Scoones, I. (1998). Sustainable Rural Livelihoods: A Framework for Analysis. https://opendocs.ids.ac.uk/opendocs/handle/20.500.12413/3390
- Selm, K.R., Hess, G.R., Peterson, M.N., Beck, S.M., & McHale, M.R. (2018). Developing an Instrument to measure autonomous adaptive capacity to climate change among urban households. Frontiers in Ecology and Evolution, 6, 6. doi 10.3389/fevo.2018.00013
- Shackleton, C.M., & Shackleton, S.E. (2006). Household wealth status and natural resource use in the Kat River valley, South Africa. Ecological Economics, 57(2), 306–317. https://doi.org/10.1016/j.ecolecon.2005.04.011
- Smit, B., & Wandel, J. (2006). Adaptation, adaptive capacity and vulnerability. Global Environmental Change, 16(3), 282–292. https://doi.org/10.1016/j.gloenvcha.2006.03.008
- Stevens, F.R. (2022). KAZAVA household survey data and processing for vulnerability modelling. [Data set]. figshare. https://doi.org/10.6084/m9.figshare.20459805.v1
- Tsegaye, D., Moe, S.R., Vedeld, P., & Aynekulu, E. (2010). Land-use/cover dynamics in northern afar rangelands, ethiopia. Agriculture, Ecosystems & Environment, 139(1), 174–180. https://doi.org/10.1016/j.agee.2010.07.017
- Turner, B., II, Esler, K.J., Bridgewater, P., Tewksbury, J., Sitas, N., Abrahams, B., Chapin, F.S., Chowdhury, R.R., Christie, P., Diaz, S., Firth, P., Knapp, C.N., Kramer, J., Leemans, R., Palmer, M., Pietri, D., Pittman, J., Sarukhán, J., Shackleton, R. … Mooney, H. (2016). Socio-Environmental Systems (SES) Research: What have we learned and how can we use this information in future research programs. Current Opinion in Environmental Sustainability, 19, 160–168. https://doi.org/10.1016/j.cosust.2016.04.001
- Turner, B.L., Kasperson, R.E., Matson, P.A., McCarthy, J.J., Corell, R.W., Christensen, L., Eckley, N., Kasperson, J.X., Luers, A., Martello, M.L., Polsky, C., Pulsipher, A., & Schiller, A. (2003). A framework for vulnerability analysis in sustainability science. Proceedings of the National Academy of Sciences, 100(14), 8074–8079. https://doi.org/10.1073/pnas.1231335100
- Turner, B.L., Lambin, E.F., & Reenberg, A. (2007). The emergence of land change science for global environmental change and sustainability. Proceedings of the National Academy of Sciences, 104(52), 20666–20671. https://doi.org/10.1073/pnas.0704119104
- Ullman, J.B., & Bentler, P.M. (2012). Structural Equation Modeling. Handbook of Psychology (Second Edition ed.). John Wiley & Sons, Ltd. https://doi.org/10.1002/9781118133880.hop202023
- United Nations. (2015). Transforming our world: The 2030 agenda for sustainable development | department of economic and social affairs. https://sdgs.un.org/2030agenda
- Verburg, P.H., Erb, K.-H., Mertz, O., & Espindola, G. (2013). Land System Science: Between global challenges and local realities. Current Opinion in Environmental Sustainability, 5(5), 433–437. https://doi.org/10.1016/j.cosust.2013.08.001
- Verburg, P.H., van de Steeg, J., Veldkamp, A., & Willemen, L. (2009). From land cover change to land function dynamics: A major challenge to improve land characterization. Journal of Environmental Management, 90(3), 1327–1335. https://doi.org/10.1016/j.jenvman.2008.08.005
- Viana, C.M., Freire, D., Abrantes, P., Rocha, J., & Pereira, P. (2022). Agricultural land systems importance for supporting food security and sustainable development goals: A systematic review. Science of the Total Environment, 806, 150718. https://doi.org/10.1016/j.scitotenv.2021.150718
- Williams, P.A., Crespo, O., Abu, M., & Simpson, N.P. (2018). A systematic review of how vulnerability of smallholder agricultural systems to changing climate is assessed in Africa. Environmental Research Letters, 13(10), 103004. https://doi.org/10.1088/1748-9326/aae026
- WorldBank. (2022). GDP per capita (current US$) | Data. https://data.worldbank.org/indicator/NY.GDP.PCAP.CD
- Wright, H., Kristjanson, P.M., & Bhatta, G.D. (2012). Understanding adaptive capacity: Sustainable livelihoods and food security in coastal bangladesh [Working Paper]. CGIAR Research Program on Climate Change, Agriculture and Food Security. https://cgspace.cgiar.org/handle/10568/24794