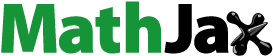
ABSTRACT
Urban expansion and development continue to increase globally, threatening rural multifunctional landscapes that provide a diversity of direct and indirect ecological benefits. However, not all landscapes are equally impacted, thus making conservation planning more difficult. We used 8 years of land use and land cover to understand this change in Greenville Co. SC, a region experiencing rapid expansion of development into rural landscapes. Regression models helped explain the relationships between development predictor variables and rural response variables. To explore where these changes were occurring, we determined which size categories of land parcels were the most developed and how this is changing over time. Developed open space was revealed to significantly impact rural character, pasture, and evergreen forest most frequently. It was also found to be most prevalent in the smallest parcels. Determining relationships between development, rural character, and multifunctionality will aid in potential solutions for the conservation of multifunctional landscapes.
Introduction
Land use and land cover change driven by increasing population density and land use intensity continues regionally and globally (Ellis et al., Citation2021). As a consequence, environmental amenities are being lost in many rural, multifunctional landscapes. For example, an increase in impervious surfaces creates non-point source pollution and more aggressive surface runoff in the absence of vegetation cover to control surficial processes (Tang et al., Citation2005). Similarly, the homogenization of landscapes results in loss of habitat for beneficial plants (Burton & Samuelson, Citation2008), wildlife (Hansen et al., Citation2005; Quinn et al., Citation2012), and functional taxonomic groups, such as pollinators (Vanbergen & the Insect Pollinators Initiative, Citation2013). Perhaps most pressing, it also leads to a loss of rural, working lands essential for the production of food, fiber, and fuel near population centers (van Vliet et al., Citation2017). The loss of these amenities to land use and specifically urban development (Brown & Quinn, Citation2018) parallels the conversations regarding loss of multifunctional landscapes and associated benefits.
Multifunctional landscapes encompass complex and diverse systems that reflect human and natural drivers (Hölting et al., Citation2019; Quinn & Wood, Citation2017). Land uses include recreation, rural spaces, energy development, and production of food, fiber, and fuel. They provide a diverse range of benefits to communities within and beyond their boundaries, including the conservation of biodiversity and the supply of multiple ecosystem services (Hölting et al., Citation2019). Forestry, ranching, agriculture, and urban centers have all demonstrated similar capabilities to supply provisioning services and to protect biodiversity (Fuhlendorf et al., Citation2006; Löf et al., Citation2016; Quinn et al., Citation2014; Ziter Citation2016). However, less is understood about the multifunctionality of populated and residential woodlands that are common at regional and global extents (Ellis et al., Citation2021). These landscapes have less of an emphasis on large-scale commodity production inherent to timber or corn production but can provide a range of values beyond housing if land is used and managed in a sustainable way.
Multifunctional landscapes and other heterogeneous spaces are threatened globally. In some cases, these landscapes are being simplified, which has occurred across the Midwestern United States through an increase in agricultural intensity (Quinn et al., Citation2017). In other cases, heterogeneity is lost when one land use is converted to another. For instance, this has transpired through the loss of forest land to farmland in multiple nations (Turubanova et al., Citation2018). A third example, and the focus of increasing concern, is the loss of multifunctional cultured landscapes to more homogeneous urban and suburban development (Brown & Quinn, Citation2018; Gibson & Quinn, Citation2017). Our work here explores this trend by determining which types of urban development most significantly impact different categories of multifunctional rural lands. Thus, this work addresses a significant gap in the literature where developmentin particular, urban development, is presented as a singular concept and threat to ecosystem sustainability in regional modeling efforts (e.g., Blaszczak et al., Citation2019; Brown & Quinn, Citation2018; Foley et al., Citation2005; Ureta et al., Citation2020)
Beyond measures of change, it is valuable to understand what policy mechanisms may or may not have an impact on ecosystem services (e.g., Conway & Lathrop, Citation2005). These mechanisms include zoning, urban growth boundaries, and minimum lot sizes. Past work has demonstrated that zoning in the region has had little effect on the flow of ecosystem services (Brown & Quinn, Citation2018). In contrast, urban growth boundaries have been successful in specific cases (e.g., Robertson, Citation2014). Minimum lot sizes have been studied for economic benefits (e.g., Zabel & Dalton, Citation2011), but little or no research has been done on environmental parameters.
The objectives of our analysis were to determine (1) which aspects of rural, multifunctional lands were most vulnerable to land use development, (2) which development types are associated with the greatest decline in these multifunctional land use types, and (3) if land-use policy had the capability to be utilized as a potential solution to this trend.
Methods
Study site
We studied changing land use and land cover (LULC) in Greenville county in northern South Carolina, USA (). The size of this county is 2035.5 square kilometers (US Census Bureau, Citation2021), and it is located primarily in the Piedmont ecoregion, with a small portion of the northernmost parts in the Blue Ridge ecoregion. The county is a heterogeneous mix of forests, farmland, and urban development that has seen a rapid increase in development in the last decade (Brown & Quinn, Citation2018). The areas of development are primarily located in the middle of the county along the I-85 corridor, but are spreading north and south from the corridor. The city of Greenville is the largest settlement within the county, and it is centered within the majority of Greenville county’s urban development. The human population in the county grew from 451,225 in 2010 to 525,534 in 2020, constituting a 16.5% increase, with many projections suggesting that this rate will continue or even increase until 2060. Furthermore, the city of Greenville’s population grew by a greater rate of 21%, from 58,409 in 2010 to 70,720 in 2020 (US Census Bureau, Citation2021).
Data collection
We obtained LULC raster layers from CropScape (USDA National Agricultural Statistics Service Cropland Data Layer, Citation2020), which is a geospatial data service provided by the USDA that provides crop-specific LULC data from satellite imagery for the continental United States. We used the data given for Greenville County, South Carolina, for the years 2008 to 2018. For our analysis, we chose to focus on the following attributes: developed high intensity, developed medium intensity, developed low intensity, developed open space, evergreen forest, cultivated cropland, and grass pasture/hay. These development types are defined by the Multi-Resolution Land Characteristics (MRLC) Consortium in the USGS National Land Cover Database (Wickham et al., Citation2014) as areas with increasing amounts of constructed infrastructure and impervious surfaces, where developed open space is mostly lawn grasses with some constructed materials, developed low and medium intensity are the intermediary categories, and developed high intensity is almost entirely impervious surfaces. Since South Carolina does not contain much land specifically dedicated to cropland, we reclassified all cropland types into one category that was labeled cultivated cropland. Evergreen forest was distinguished from other forest types because it is considered an agricultural commodity in South Carolina. We also calculated a unique constructed indicator of total rural character defined as all variables that constitute the rural aesthetic of the county: here a combination of the evergreen forest, cultivated cropland, grass pasture/hay, and fallow idle cropland.
We also obtained vector layers for Hydrologic Unit Code 12 (HUC12) watersheds (USGS Water Resources of the United States, Citation2020), zoning (Greenville County Geographic Information Systems, Citation2020a), and tax parcel boundaries (Greenville County Geographic Information Systems, Citation2020b). As our unit of analysis, we quantified the proportion of each LULC for individual watersheds and what proportion of each watershed was zoned. To elucidate the impact of any political zoning on LULC changes, we did not distinguish between the different zoning types and instead only identified the proportion of watersheds that were zoned versus unzoned. We summarized the proportion of the LULCs in each tax parcel within Greenville County for the years 2010, 2013, 2016, and 2018 to better understand if there were patterns of development associated with lot or parcel size. Parcel sizes were discretized into four categories: zero to two acre parcels, two to five acre parcels, five to ten acre parcels, and ten or more acre parcels.
As evidence of the change in benefits from natural and managed ecosystems in the county, we calculated the change in four ecosystem services. Building on our past work (Brown & Quinn, Citation2018) we derived nutrient movement (nitrogen and phosphorus), sediment export, and carbon storage with InVEST (Integrated Valuation of Ecosystem Services and Tradeoffs) software, version 3.9.0. The description, inputs, and outputs of each InVEST model used can be found in of Brown and Quinn (Citation2018) and the included appendix 1. We compared carbon storage and exports of nitrogen, phosphorus, and sediment in 2011 and 2016 in Greenville County. This was done for HUC12 watersheds, including watersheds that extend beyond the county boundary, but was not done for Greenville’s tax parcels as we were less interested in the scale of change in ecosystem services spatially, but rather the relative rate of change overtime.
Table 1. Results from beta mixed-effect regression models.
Data analysis
To analyze rates and drivers of land use change, we utilized beta mixed-effect regression models with a random intercept for HUC12 watershed. We selected this model for two reasons. First, the response variable of our analyses are rates between 0 and 1. Second, repeated measurements over time were made for each watershed. We tested if and how well the proportion per watershed of developed open space, developed low intensity, developed medium intensity, developed high intensity, and zoning predicted our selected response variables, which were evergreen forest, cultivated cropland, grass pasture/hay, and total rural character. These models were run using the glmmTMB (Brooks et al., Citation2017) package in R (R Core Team, Citation2021). We also fit a linear model to these data to discern whether parcel size or year were causing significant changes in the proportion of developed open space per parcel. For this model, we utilized the linear model function in R with parcel size and year as our predictor variables and developed open space as our response. We defined significance in all models by a p-value of less than 5% significance level.
Results
In 2010, there were 270,330 acres of rural land (inclusive of evergreen forest, cultivated cropland, grass pasture/hay, and fallow idle cropland) in Greenville Co, SC. This included 4,878 acres of cropland and 66,506 acres of timber. By 2018, the total acreage decreased by 25% to only 203,758 acres of rural land, with 3,637 acres of cropland and 55,760 acres of timber. Conversely, there was a 41% increase in developed open space during this time, from 74,882 acres in 2010 to 105,812 in 2018, and a 32% increase across development categories, from 141,064 acres in 2010 to 186,041 in 2018.
From our beta regression models, developed open space had a significant negative impact on the extent of grass pasture/hay (Estimate ,
, ), evergreen forest (Estimate
,
, ), and total rural character (Estimate
,
, ). Developed medium intensity (Estimate
,
, ) and developed low intensity (Estimate
,
, ) had an even larger and significant negative effect on the extent of the total rural area. However, these development categories did not show an effect on individual land use types. Cultivated cropland was not significantly impacted by any of the five predictor variables in our model. Cropland was positively associated with developed open space, though uncertainty persists (Estimate
,
, ). However, it should be noted that cropland only comprised 1.317% of all rural land in both 2008 and 2019. Zoning practices could have moderated the negative impacts of rural change. Zoned watersheds showed a positive association with pasture (Estimate
,
, ) and total rural character (Estimate
,
, ). From our linear regression model, size was important in explaining the expansion of developed open space with parcel sizes of 0.0–2.0, 2.0–5.0, and 5.0–10.0 acres (
, , ) as evidenced by the total sum of all open space development being highest within the 0.0–2.0 acre category and increased from 2010 to 2018. However, year was also significant (
, , ), as the amount of developed open space substantially increased from 2010 to 2018.
Table 2. Results from multiple linear regression model.
Parallel to the land use change, there was a clear loss of multiple ecosystem services. In the county, phosphorus exports increased from 2011 to 2016 in most watersheds, sediment exports decreased in most watersheds, and nitrogen exports were unaffected by landscape changes (). Watersheds that had high carbon storage in 2011 had lower carbon storage in 2016, and watersheds with moderate carbon storage in 2011 saw a slight increase for 2016.
Figure 3. Shifts in mean ecosystem service delivery from 2011 to 2016 by watershed. Mean values for (a) nitrogen export, (b) phosphorus export, (c) sediment export, and (d) carbon storage for each watershed in Greenville County graphed for 2011 and 2016 in comparison to a y = x linear relationship. This line represents no change between 2011 and 2016 values, while watersheds depicted above or below the line have seen an increase or decrease, respectively, in the ecosystem service over the five years.

Discussion
With this project, we present an innovative approach to analyze drivers of the change in a rural landscape. We show that over the past decade, the loss of rural character, pasture, and evergreen forests was primarily associated with development. Specifically, the loss of these multifunctional landscapes was associated most consistently with open space development, which is classified as turf grasses with some built infrastructure like buildings or pavement (Dewitz & U.S. Geological Survey, Citation2021). Low and medium intensity development were only associated with the loss of total rural character, suggesting that open space development is the most impactful development type within this county. High-intensity development was not significantly associated with any of the response variables. This implies that rural land is not directly being converted into highly developed areas with dense apartment complexes or other spaces where people reside or work in large capacities. Cultivated cropland, what is commonly thought of when farmland is studied, was not significantly impacted by development, likely because it is such a small portion of the county’s rural landscape. More broadly, these data clearly suggest that oversimplification of development as a singular driver of change as used in past research (e.g., Blaszczak et al., Citation2019; Brown & Quinn, Citation2018; Ureta et al., Citation2020) may misrepresent drivers of the loss of multifunctional landscapes.
While there are few cases of formal evaluations in the literature of the policy decisions on land use change and ecosystem services (as compared to other social or economic factors, e.g., Zabel & Dalton, Citation2011), there is an emerging literature suggesting the policy impacts on both land use and ecosystem services are evident but heterogeneous and perhaps location specific (Debbage & Shepherd, Citation2018; Hamel et al., Citation2021). The results here from our beta regression models show that zoning did have a positive association with grassland cover and total rural character, suggesting that it could potentially moderate the loss of these lands. But this was not the case with other specific land use types. This is an interesting contrast to past work where we saw zoning did not moderate any ecosystem services (Brown & Quinn, Citation2018). Future work could build on this analysis by determining the relationship between types of zoning and LULC changes in the county. These results would be useful in decision-making for future zoning policies aimed towards the conservation of multifunctional land.
There was not a clear minimum lot size that could be established based on our analysis of developed open space within Greenville’s tax parcels. As seen in , there were clear differences in the amount of open space development between the different size categories of the parcels, where the 0 to 2 acre parcels contained the most and the 5 to 10 acre parcels contained the least. However, the parcels that were 10 acres or greater contained the second greatest amount of open space development. Time was also a significant driver in the change of open space development. This is true for both the total amount of open space development (), as well as the proportion of open space development per parcel (). It is of note, though, that the total area substantially increased from 2010 to 2016 but increased at a much slower rate from 2016 to 2018, and it even decreased from 2016 to 2018 in the parcels that were 10 acres or greater.
The InVEST models provide further evidence that aligns with past work (Brown & Quinn, Citation2018; Gibson & Quinn, Citation2017; Ureta et al., Citation2020) that the replacement of rural character with developed open space decreases ecosystem services in the landscape. The loss of these land uses results in a loss of multiple ecological benefits. For example, pasture reduces runoff, sequesters carbon, and provides wildlife habitat. These manicured lands of open space development require large inputs of synthetic fertilizers, increasing phosphorus exports into local waterways. In contrast, timberland and other forests provide diverse value to the local economy. Indeed, the direct economic impact of forestry in South Carolina is estimated at $21 billion annually as of 2018 (Adams et al., Citation2019). Additionally, the tourism industry is in part driven by views of forests from our public lands and quiet spaces on private lands. At the same time, timberland provides a diversity of indirect values including stormwater retention, habitat for wildlife and pollinators, carbon storage, and erosion prevention.
It would be valuable for future work to look more specifically at the interactions between land use types and ecosystem services. In the export models, we did see increased phosphorus export. But is this driven by urbanization and not other land use types? It is clear that land use change affects and is affected by ecosystem processes beyond political boundaries (Bolund & Hunhammar, Citation1999; Jiang et al., Citation2008; McDonald et al., Citation2016).
There are a few limitations that should be considered for this study. Our beta regression models revealed significant relationships between our predictor and response variables over time, which provided insight to positive and negative associations between variables. However, we did not track the change of LULC in each ownership parcel of land over time to determine exactly how these land cover types are changing.
We also did not consider factors other than the four development types for changes in our agricultural variables. As we only considered the watersheds within the boundary of Greenville county, it would also be beneficial to continue this work in other areas with similar rapid development and to develop models that could make predictions for future changes.
Conclusion
This study examined the relationship between development and multifunctional landscapes. We analyzed LULC changes in Greenville County, South Carolina from 2010 to 2018 to determine which aspects of multifunctional landscapes were vulnerable to development and which urban and suburban development types were driving this change. Open space development, which has little ecological function, was found to be most strongly associated with the loss of each multifunctional landscape, and we determined that cultivated cropland did not appear to be at risk of development in this county. We found that zoning could potentially be used to mitigate some of this land conversion, which is contrary to previous findings for Greenville County. Further analysis is needed to determine which types of zoning would be most effective here. Lastly, a minimum lot size could not be established based on our analysis of open space development within the tax parcels of Greenville County. Application of the estimates presented here to regional planning efforts could improve how future development should be moderated to allow for growth with the least amount of impact on the landscape.
Disclosure statement
The authors confirm that there are no relevant financial or non-financial competing interests to report.
References
- Adams, T.O., Danskin, S.D., & Aries, E.F. (2019). The wave of wood: Forestry’s economic contribution to South Carolina’s economy in 2018. South Carolina Forestry Commission.
- Blaszczak, J.R., Delesantro, J.M., Zhong, Y., Urban, D.L., & Bernhardt, E.S. (2019). Watershed urban development controls on urban streamwater chemistry variability. Biogeochemistry, 144(1), 61–84. https://doi.org/10.1007/s10533-019-00572-7
- Bolund, P., & Hunhammar, S. (1999). Ecosystem services in urban areas. Ecological Economics, 29(2), 293–301. https://doi.org/10.1016/S0921-8009(99)00013-0
- Brooks, M.E., Kristensen, K., Van Benthem, K.J., Magnusson, A., Berg, C.W., Nielsen, A., Skaug, H.J., Machler, M., & Bolker, B.M. (2017). glmmTMB balances speed and flexibility among packages for zero-inflated generalized linear mixed modeling. The R Journal, 9(2), 378–400. https://doi.org/10.32614/RJ-2017-066
- Brown, M.G., & Quinn, J.E. (2018). Zoning does not improve the availability of ecosystem services in urban watersheds. A case study from Upstate South Carolina, USA. Ecosystem Services, 34, 254–265. https://doi.org/10.1016/j.ecoser.2018.04.009
- Burton, M.L. and Samuelson, L.J. (2008). Influence of urbanization on riparian forest diversity and structure in the Georgia Piedmont, US. Plant Ecology, 195(1), 99–115. https://doi.org/10.1007/s11258-007-9305-x
- Conway, T.M., & Lathrop, R.G. (2005). Modeling the ecological consequences of land-use policies in an urbanizing region. Environmental Management, 35(3), 278–291. https://doi.org/10.1007/s00267-004-4067-x
- Debbage, N., & Shepherd, J.M. (2018). The influence of urban development patterns on streamflow characteristics in the Charlanta Megaregion. Water Resources Research, 54(5), 3728–3747. https://doi.org/10.1029/2017WR021594
- Dewitz, J., & U.S. Geological Survey. (2021). National Land Cover Database (NLCD) 2019 products (ver. 2.0, June 2021). U.S. Geological Survey data release. https://doi.org/10.5066/P9KZCM54.
- Ellis, E.C., Gauthier, N., Klein Goldewijk, K., Bird, R.B., Boivin, N., Díaz, S., Fuller, D.Q., Gill, J.L., Kaplan, J.O., Kingston, N., Locke, H., McMichael, C.N.H., Ranco, D., Rick, T.C., Shaw, M.R., Stephens, L., Svenning, J.-C., & Watson, J.E.M. (2021). People have shaped most of terrestrial nature for at least 12,000 years. Proceedings of the National Academy of Sciences, 118, e2023483118. https://doi.org/10.1073/pnas.2023483118
- Foley, J.A., DeFries, R., Asner, G.P., Barford, C., Bonan, G., Carpenter, S.R., Chapin, F.S., Coe, M.T., Daily, G.C., Gibbs, H.K., Helkowski, J.H., Holloway, T., Howard, E.A., Kucharik, C.J., Monfreda, C., Patz, J.A., Prentice, I.C., Ramankutty, N., & Snyder, P.K. (2005). Global consequences of land use. Science: Advanced Materials and Devices, 309(5734), 570–574. https://doi.org/10.1126/science.1111772
- Fuhlendorf, S.D., Harrell, W.C., Engle, D.M., Hamilton, R.G., Davis, C.A., & Leslie, D.M., Jr. (2006). Should heterogeneity be the basis for conservation? Grassland bird response to fire and grazing. Ecological Applications, 16(5), 1706–1716. https://doi.org/10.1890/1051-0761(2006)016[1706:SHBTBF]2.0.CO;2
- Gibson, D.M., & Quinn, J.E. (2017). Application of anthromes to frame scenario planning for landscape-scale conservation decision making. Land, 6(2), 33. https://doi.org/10.3390/land6020033
- Greenville County Geographic Information Systems. (2020a). Zoning [Data set]. Greenville County. https://www.gcgis.org/AccessDistribution.html#
- Greenville County Geographic Information Systems. (2020b). Tax parcels & ownership information [data set]. Greenville County. https://www.gcgis.org/Access.Distribution.html#
- Hamel, P., Guerry, A.D., Polasky, S., Han, B., Douglass, J.A., Hamann, M., Janke, B., Kuiper, J.J., Levrel, H., Liu, H., Lonsdorf, E., McDonald, R.I., Nootenboom, C., Ouyang, Z., Remme, R.P., Sharp, R.P., Tardieu, L., Viguié, V. … Zheng, H. (2021). Mapping the benefits of nature in cities with the InVEST software. Npj Urban Sustainability, 1(1), 25. https://doi.org/10.1038/s42949-021-00027-9
- Hansen, A.J., Knight, R.L., Marzluff, J.M., Powell, S., Brown, K., Gude, P.H., & Jones, K. (2005). Effects of exurban development on biodiversity: Patterns, mechanisms, and research needs. Ecological Applications, 15(6), 1893–1905. https://doi.org/10.1890/05-5221
- Hölting, L., Jacobs, S., Felipe-Lucia, M.R., Maes, J., Norström, A.V., Plieninger, T., & Cord, A.F. (2019). Measuring ecosystem multifunctionality across scales. Environmental Research Letters, 14(12), 124083. https://doi.org/10.1088/1748-9326/ab5ccb
- Jiang, L., Young, M.H., & Hardee, K. (2008). Population, urbanization and the environment. World Watch, 5, 34–39.
- Löf, M., Brunet, J., Filyushkina, A., Lindbladh, M., Skovsgaard, J.P., & Felton, A. (2016). Management of oak forests: Striking a balance between timber production, biodiversity and cultural services. International Journal of Biodiversity Science, Ecosystem Services & Management, 12(1–2), 59–73. https://doi.org/10.1080/21513732.2015.1120780
- McDonald, R.I., Weber, K.F., Padowski, J., Boucher, T., & Shemie, D. (2016). Estimating watershed degradation over the last century and its impact on water-treatment costs for the world’s large cities. Proceedings of the National Academy of Sciences, 113(32), 9117–9122. https://doi.org/10.1073/pnas.1605354113
- Quinn, J.E., Awada, T., Trindade, F., Fulginiti, L., & Perrin, R. (2017). Combining habitat loss and agricultural intensification improves our understanding of drivers of change in avian abundance in a North American cropland anthrome. Ecology and Evolution, 7(3), 803–814. https://doi.org/10.1002/ece3.2670
- Quinn, J.E., Brandle, J.R., & Johnson, R.J. (2012). The effects of land sparing and wildlife-friendly practices on grassland bird abundance within organic farmlands. Agriculture, Ecosystems & Environment, 161, 10–16. https://doi.org/10.1016/j.agee.2012.07.021
- Quinn, J.E., Johnson, R.J., & Brandle, J.R. (2014). Identifying opportunities for conservation embedded in cropland anthromes. Landscape Ecology, 29(10), 1811–1819. https://doi.org/10.1007/s10980-014-0098-8
- Quinn, J.E., & Wood, J.M. (2017). Application of a coupled human natural system framework to organize and frame challenges and opportunities for biodiversity conservation on private lands. Ecology and Society, 22(1). https://doi.org/10.5751/ES-09132-220139
- R Core Team. (2021). R: A language and environment for statistical computing. R Foundation for Statistical Computing. https://www.R-project.org/
- Robertson, M. (2014). Sustainability Principles and Practice. Routledge.
- Tang, Z., Engel, B.A., Pijanowski, B.C., & Lim, K.J. (2005). Forecasting land use change and its environmental impact at a watershed scale. Journal of Environmental Management, 76(1), 35–45. https://doi.org/10.1016/j.jenvman.2005.01.006
- Turubanova, S., Potapov, P.V., Tyukavina, A., & Hansen, M.C. (2018). Ongoing primary forest loss in Brazil, democratic republic of the Congo, and Indonesia. Environmental Research Letters, 13(7), 074028. https://doi.org/10.1088/1748-9326/aacd1c
- Ureta, J.C., Clay, L., Motallebi, M., & Ureta, J. (2020). Quantifying the landscape’s ecological benefits—an analysis of the effect of land cover change on ecosystem services. Land, 10(1), 21. https://doi.org/10.3390/land10010021
- US Census Bureau. (2021) Retrieved from https://www.census.gov/quickfacts/greenvillecountysouthcarolina
- USDA National Agricultural Statistics Service Cropland Data Layer. 2020. Published crop-specific data layer. USDA-NASS. Retrieved June, 2020; Verified June, 2020 [Online]. Available at https://nassgeodata.gmu.edu/CropScape/
- USGS Water Resources of the U.S. (2020). The watershed boundary dataset [data set]. USGS. https://water.usgs.gov/GIS/huc.html
- Vanbergen, A.J., & the Insect Pollinators Initiative. (2013). Threats to an ecosystem service: Pressures on pollinators. Frontiers in Ecology and the Environment, 11(5), 251–259. https://doi.org/10.1890/120126
- van Vliet, J., Eitelberg, D.A., & Verburg, P.H. (2017). A global analysis of land take in cropland areas and production displacement from urbanization. Global Environmental Change, 43, 107–115. https://doi.org/10.1016/j.gloenvcha.2017.02.001
- Wickham, J., Homer, C., Vogelmann, J., McKerrow, A., Mueller, R., Herold, N., & Coulston, J. (2014). The multi-resolution land characteristics (MRLC) consortium—20 years of development and integration of USA national land cover data. Remote Sensing, 6(8), 7424–7441. https://doi.org/10.3390/rs6087424
- Zabel, J., & Dalton, M. (2011). The impact of minimum lot size regulations on house prices in Eastern Massachusetts. Regional Science and Urban Economics, 41(6), 571–583. https://doi.org/10.1016/j.regsciurbeco.2011.06.002
- Ziter, C. (2016). The biodiversity–ecosystem service relationship in urban areas: A quantitative review. Oikos, 125(6), 761–768. https://doi.org/10.1111/oik.02883
Appendix 1.
Data sources for modeling ecosystem services
Nutrient Delivery Ratio model
1. Digital elevation model
Source: https://gdg.sc.egov.usda.gov/GDGHome.aspx
Resolution: 30 m
Notes:
Data from USGS via SCDNR database
Projection changed toNAD 1983 UTM 17N
Filled
Clipped to watersheds that are at least partially in Greenville and Spartanburg counties
2. Nutrient runoff proxy – using annual precipitation
Source: https://gdg.sc.egov.usda.gov/GDGHome.aspx
Notes:
Projection is NAD 1983 UTM 17N
Units were originally inches, changed to mm
Clipped to watersheds that are at least partially in Greenville and Spartanburg
Average annual precipitation 1981–2010
3. Watersheds
Source: https://gdg.sc.egov.usda.gov/GDGHome.aspx
Notes:
Twelve digit watersheds
Added ws_id field
Projection is NAD 1983 UTM 17N
Selected watersheds at least partially Greenville and Spartanburg counties to create new shapefile of watersheds contributing to those counties. Used this shapefile to clip other layers.
Sediment Delivery Model
1. DEM
Source: https://gdg.sc.egov.usda.gov/GDGHome.aspx
Resolution: 30 m
Notes:
Data from USGS via SCDNR database
Projection changed to NAD 1983 UTM 17N
Filled
Clippedto watersheds that are at least partially in Greenville and Spartanburgcounties
2. Rainfall erosivity index (R)
Source: Digitized from USDA map
Notes:
Projection is NAD 1983 UTM 17 M
Digitized from USGS map
Clipped to watersheds at least partially in Greenville and Spartanburg Counties
Multiplied by 17.02 to get correct units.
3. Soil erodibility (K)
Source: https://gdg.sc.egov.usda.gov/GDGHome.aspx
Notes:
Changed to UTM17N
Clipped to watersheds
Integer raster to float
Divided values by 100 to reverse changes made by ESRI
Multiplied by 0.127 to get to correct units.
4. Watersheds
Source: https://gdg.sc.egov.usda.gov/GDGOrder.aspx
Notes:
Twelve digit watersheds
Added ws_id field
Projection is NAD 1983 UTM 17N
Selected watersheds at least partially Greenville and Spartanburg counties to create new shapefile of watersheds contributing to those counties. Used this shapefile to clip other layers.
5. Biophysical Table
Carbon Model
1. Carbon pools
Source:
Andersen, C.B., Donovan, R.K., Quinn, J.E. 2015. Human Appropriation of Net Primary Production (HANPP) in an agriculturally-dominated watershed, southeastern U.S.A.. Land. 4(2), 513–540; doi:10.3390/land4020513
Gibson, D.M., Quinn, J.E. 2017 Application of anthromes to frame scenario planning for landscape-scale conservation decision making. Land. 6(2): 33