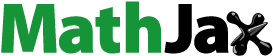
ABSTRACT
Root zone soil moisture (RZSM) is a critical parameter for drought monitoring, agriculture, and vegetation growth. In situ measurements of RZSM are scarce, and therefore, RZSM products are derived mainly through modeling approaches. This study evaluated seven RZSM products, including ERA5, MERRA2, CFSR, SMAP level 4, GLDAS-NOAH2.1, GLDAS-Catchment2.2, and SMOS CATDS Level 4, using 1153 ground sites across China. The evaluations aimed to address which products perform better, how well they capture the seasonal variations, and how they perform under different land surface and climate conditions. Results showed that MERRA2 and NOAH had the best performance, with MERRA2 having a better ability to capture surplus and deficit soil moisture conditions. The study also revealed that precipitation forcing plays a vital role in improving RZSM simulations. Conversely, ERA5 overestimates RZSM, and SMOS exhibits a dry bias. From the time series variability, all datasets except SMOS can capture the temporal dynamics of RZSM well. Moreover, the accuracy of the RZSM products varies significantly in tropical climate zones. This study provides crucial insights for improving land surface and hydrological models in estimating RZSM, which will help in drought management and agriculture production.
1. Introduction
Root zone soil moisture (RZSM) refers to the water that is available to plants. In comparison to surface soil moisture, RZSM plays a more crucial role in various applications, such as agricultural monitoring (Peng, Deng, and Di Citation2014), drought and flood forecasting (Xu et al. Citation2020), land‒atmosphere feedbacks (Tobin et al. Citation2020), and the hydrologic response of watersheds (Zohaib, Kim, and Choi Citation2017). There are three commonly used methods to obtain RZSM information.
The first method involves in-situ soil moisture sensors. However, field measurements are only representative on a small spatial scale, which restricts their applications. Microwave remote sensors have been an effective tool for measuring the distribution of soil water content globally. However, due to the limitation of microwave depth signal penetration in X-band and L-band, only surface soil moisture (SSM) in the top few centimeters (0–5 cm) can be obtained. Since SSM is closely related to RZSM through the diffusion process, some methods have been developed to obtain RZSM from SSM. For instance, the exponential filter (EF) method uses a simplified two-layer water balance approach to establish the relationship between SSM and RZSM, assuming uniform hydraulic conductivity and the absence of transpiration (Albergel et al. Citation2008; Wagner, Lemoine, and Rott Citation1999). The EF method has gained popularity in recent years due to its minimal input requirements (Grillakis et al. Citation2021; Tian et al. Citation2022; Tobin et al. Citation2017). SMOS RZSM level 4 data are developed based on this method.
Data-driven methods have also become popular in recent years, typically using SSM, meteorological data, land surface conditions, and soil properties as drivers to build machine learning models to estimate RZSM. For example, Li et al. (Citation2022) produced a 1 km daily RZSM dataset over China using a random forest model; Karthikeyan and Mishra (Citation2021) estimated multilayer high-resolution soil moisture over the United States using an extreme gradient boosting algorithm.
The third method for obtaining RZSM involves modeling approaches that combine climate models or land surface models with data assimilation techniques. RZSM reanalysis data products such as the fifth generation European Centre for Medium-Range Weather Forecasts(ECMWF) atmospheric reanalysis (ERA5), the Modern-Era Retrospective analysis for Research and Applications, Version 2 (MERRA2), and the Climate Forecast System Reanalysis (CFSR) rely on this method. Similarly, the RZSM products of Soil Moisture Active Passive (SMAP) lever4 and Global Land Data Assimilation System (GLDAS) are also model-based. However, although these RZSM products have been produced in recent years, validation and evaluation studies are limited due to the lack of observation data.
To validate RZSM products, the global in situ soil moisture database of the International Soil Moisture Network (ISMN) is an essential resource and has been widely used for this purpose (Xu et al. Citation2021). However, the ISMN measurement stations are mostly concentrated in the United States, which limits global evaluation. China, with its diverse landscapes spanning 9.6 million square km, presents an opportunity for validation. From the tropical Hainan Province to the freezing Heilongjiang Province bordering Russia, as well as the western desert and plateau regions of Xinjiang and Tibet, and the vast grasslands of Inner Mongolia, China offers a broad range of physical landscapes for validation. However, more in situ measurements are necessary to validate RZSM products in China and elsewhere globally.
In this study, we aim to comprehensively evaluate seven RZSM products over all of China using RZSM measurements. The products include three reanalysis products (ERA5, MERRA2, and CFSR) and four land surface model-based products (SMAP level 4, SMOS level 4, GLDAS-NOAH2.1, and GLDAS-Catchment2.2). Our study addresses several important questions: Which RZSM product performs better in simulating RZSM in China? How well do these products capture seasonal variations in RZSM? How effectively do these RZSM products detect dry and wet events? What is the performance of these products under different climate and land surface conditions? The findings of this study may contribute to improving global RZSM simulations and enhancing the accuracy of land surface, climate, and hydrological models. It should be noted that because different types of vegetation exhibit different rooting depth. To keep consistency between in-situ data and data products, soil moisture at depths of 0–100 cm is considered as an representation of root zone soil moisture in this study.
2. Data and data processing
2.1. In situ soil moisture data
Volumetric soil water content data observed at 2,417 soil moisture sites across China from 2018 to 2019 were obtained from the China Meteorological Administration (CMA). The data were measured every hour at depths from 10–100 cm. Few sites have depths reaching to 200 cm. Observation interval at depth above 50 cm is 10 cm. For deeper depth, intervals are unequal from10 cm to 30 cm. In this study, the original hourly data were arithmetically averaged to obtain daily data. To ensure the selection of high-quality in situ stations, we applied three criteria in our initial station selection. The first criterion involved the amount of missing data within a candidate station, and only sites with at least 365 concurrent daily values were kept. The second criterion considered the aerial representativeness of the stations due to the scale mismatch between the point site and the RZSM products’ resolution. We calculated the ratio of the standard deviation (SD) between three references (MERRA2, NOAH, ERA5) and in situ data and filtered out the stations with a ratio less than 0.1 or larger than 3. The third criterion was that stations with a correlation with MERRA2, NOAH, and ERA5, all smaller than 0.5, were also removed to alleviate the representativeness error issue in soil moisture validation. These latter two criteria were adopted in the study of Xu et al. (Citation2021), who evaluated eight RZSM products using ISMN measurements. In this way, 1,153 sites were selected for further analysis. The spatial distribution of the selected sites can be seen in Fig. S1.
2.2. Root zone soil moisture data products
2.2.1. SMAP soil moisture product of level 4
The SMAP Level 4 product provides surface (0–5 cm) soil moisture and root zone soil moisture (0–100 cm) data on a global grid with 9-km resolution, updated every 3 h. This product is generated using a land data assimilation system that combines L-band brightness temperature measurements, precipitation observations, and land surface modeling (Reichle et al. Citation2019). The global evaluation of the SMAP L4 product shows that there are modest regional biases of approximately 0.01 (0.03) m3 m−3 for surface (root zone) soil moisture increments (Reichle et al. Citation2017a).
2.2.2. SMOS soil moisture product of level 4
The SMOS CATDS Level 4 product provides daily root zone soil moisture data (m3 m−3) representative of the 0–100 cm depth of the soil, with a spatial resolution of 0.25 degrees. The product is obtained through assimilating surface soil moisture data into a twin-layer water budget model, which uses a modified formulation of the exponential filter, and accounts for soil properties, such as texture, to determine field capacity and hydrodynamic transfer function. The root zone soil moisture is calculated as a weighted average of the two layers’ soil moisture. Ahmad and Mahmoodi (Citation2020) provide more details on the product.
2.2.3. GLDAS-NOAH2.1 RZSM products
The study used the GLDAS NOAH2.1 product (Rodell et al. Citation2004), which has a spatiotemporal resolution of 0.25 degrees and 3 h. This product was generated using the NOAH Model 3.6 in Land Information System (LIS) Version 7, and forced with atmospheric analysis fields from the National Oceanic and Atmospheric Administration (NOAA)/Global Data Assimilation System (GDAS), precipitation fields from the disaggregated Global Precipitation Climatology Project (GPCP) V1.3 Daily Analysis, and radiation fields from the Air Force Weather Agency's AGRicultural METeorological modeling system (AGRMET). The RZSM dataset consists of four layers, but only the first three layers (0–10 cm, 10–40 cm, and 40–100 cm) were used in this study to maintain consistency with other products. The soil moisture datasets at multiple depths are weighted to construct the root-zone (0–100 cm) averaged soil moisture. The weights are ratios of the distance of two adjacent depths to the total root zone depth (100 cm). The distances of the adjacent three layers are 10, 30 and 60 cm for GLDAS-NOAH2.1, so the weights are 0.1, 0.3 and 0.6 for the three layers, respectively, and RZSM is calculated as Eq.(1). This depth weighted method was also used in other studies of Xu et al. (Citation2021) and Carranza et al. (Citation2021).
(1)
(1) where
is root zone soil moisture at depth of 0–100 cm,
is soil water content, the subscripts are the soil depths.
2.2.4. ERA5 RZSM products
ERA5 is the fifth generation of atmospheric reanalysis to be produced at the European Centre for Medium-Range Weather Forecasts (ECMWF) and currently covers the period from 1950 to the present (Bell et al, Citation2021). ERA5 provides hourly estimates of RZSM at a resolution of 0.1° and at depths of 0–7 cm, 7–28 cm, and 28–100 cm. It is vertically interpolated to a depth of 0–100 cm with depth weighted averaging, as shown in Eq.(1). ERA5 is based on 4D-Var data assimilation using Cycle 41r2 of the Integrated Forecasting System (IFS). Assimilation data include many recent instruments, including the Advanced Scatterometer (ASCAT), the Tropical Rainfall Measuring Mission’s (TRMM) Microwave Imager (TMI), the Advanced Microwave Scanning Radiometer 2 (AMSR-2), the Global Precipitation Measurement (GPM) Microwave Imager (GMI) and other satellite sensors. The core of ERA5-Land is the Tiled ECMWF Scheme for Surface Exchanges over Land incorporating land surface hydrology (H-TESSEL) (Munoz-Sabater et al. Citation2021).
2.2.5. MERRA2 RZSM products
The Modern-Era Retrospective analysis for Research and Applications, version 2 (MERRA-2) is a global atmospheric reanalysis produced by the NASA Global Modeling and Assimilation Office (GMAO). It spans the satellite observing era from 1980 to the present. Modern hyperspectral radiance and microwave observations, along with GPS-Radio Occultation and NASA ozone datasets, are assimilated in MERRA-2. Land reanalysis is generated using coupled atmosphere‒land systems but with observation-corrected precipitation (Reichle et al. Citation2017b). Furthermore, it uses both fully coupled and semicoupled systems to make first-guess simulations and land reanalysis. The RZSM dataset in MERRA-2 includes two layers (0–5 cm and 10–100 cm), with a spatial resolution of approximately 0.5°×0.625°. RZSM at depths of 0–100 cm was also obtained by the depth weighted averaging method.
2.2.6. CFSR RZSM products
CFSR is a global, high-resolution, coupled atmosphere-ocean-land surface-sea ice system designed to provide the best estimate of the state of these coupled domains. It was developed at the Environmental Modeling Center at NCEP. It is superior to previous NCEP reanalyses with respect to an improved model, finer resolution, advanced assimilation schemes, and atmosphere‒land-ocean-sea ice coupling, assimilates satellite radiances rather than retrievals and accounts for changing CO2 and other trace gasses, aerosols and solar variations (Saha et al. Citation2014). The RZSM dataset in CFSR includes three layers (0–10 cm, 10–40 cm, 40–100 cm and 100–200 cm), with a spatial resolution of approximately 0.205°×0.204°. RZSM in the first three layers was used in the study and was aggregated into 0–100 cm by the depth weighted averaging method.
2.2.7. GLDAS-catchment2.2 RZSM products
We used GLDAS2.2 daily catchment model simulations (Rodell et al. Citation2004) in the study. This simulation was forced with the meteorological analysis fields from the ECMWF Integrated Forecasting System. The total terrestrial water anomaly observations from the Gravity Recovery and Climate Experiment (GRACE) were assimilated. The daily catchment model simulations use the UMD land cover scheme from the AVHRR land cover map. Root zone soil moisture (0–100 cm) is provided at a spatial resolution of 0.25°.
To make these RZSM datasets comparable, they are all resampled to 0.25°×0.25°, and the subdaily data from GLDAS-NOAH2.1, ERA-5, MERRA-2, SMAP and CFSR are arithmetically averaged into daily data. RZSM at depths of 0–100 cm were calculated for in-situ data and each product by using depth weighted method like the example of Eq.(1). summarizes the characteristics of the above seven RZSM datasets.
Table 1. Overview of the RZSM datasets used in this study.
2.3. Auxiliary datasets
Some auxiliary datasets were used to evaluate the accuracy of the RZSM products under different climate and land surface conditions. The Köppen-Geiger climate classification was used to characterize the performance of the RZSM products under different climate conditions. The CNLUCC land cover map was used to evaluate different land cover types. The CNLUCC data for 2018 were developed using 30 m Landsat TM images covering China (Liu et al. Citation2014) and can be downloaded from the Resource and Environment Science and Data Center (http://www.resdc.cn/). MODIS products of land surface temperature (LST) and Normalized Difference Vegetation Index (NDVI) were used to evaluate the RZSM products in a variety of contexts. We also obtained precipitation forcing data of NOAH, ERA5, CFSR, and MERRA2 and compared it with the CN05.1 precipitation data, developed by Wu and Gao (Citation2013) based on 2416 meteorological sites in China, to investigate the potential causes of RZSM simulation errors. Data on soil organic matter and soil texture, developed by Shangguan et al. (Citation2013), were also used to compared with the Harmonized World Soil Database (HWSD) which is commonly used in land surface models as soil parameters.
3. Evaluation metrics
To assess the products’ accuracy, four statistical indicators were used, including the Pearson correlation coefficient (R), root mean square error (RMSE), bias and unbiased RMSE (ubRMSE). Their equations are listed in the following:
(2)
(2)
(3)
(3)
(4)
(4)
(5)
(5) where
is the RZSM product,
is the in situ RZSM observation, and the overbar indicates the temporal mean operator. n denotes the sample size.
4. Results
4.1. Overall performances of the seven RZSM products
presents a comparison of seven RZSM products against in situ RZSM measurements. Among the six products with comparable R values, ERA5, SMAP, Catchment, NOAH, and MERRA2 have relatively higher R medians, ranging from 0.62–0.64. In contrast, SMOS has a significantly lower R median of 0.31. When considering RMSE or ubRMSE, MERRA2, SMAP, NOAH, and Catchment outperform ERA5, SMOS, and CFSR. For bias, MERRA2 and NOAH exhibit negligible biases. SMAP and SMOS have negative biases, with medians of −0.022 m3 m−3 and −0.084 m3 m−3, respectively, while ERA5, CFSR, and Catchment display positive biases ranging from 0.005 m3 m−3–0.041 m3 m−3. Overall, the results suggest that MERRA2 and NOAH perform slightly better than Catchment, SMAP, and ERA5 in RZSM estimations, while SMOS performs the worst. The error bars in represent the standard deviations around the median metrics.
4.2. Evaluation on land covers
To assess how land cover affects the performance of RZSM estimation, gives the evaluations of the seven RZSM products on land covers of forest, grassland, wetland, build-up, irrigated cropland and rainfed cropland. Because only 6 stations belong to the bare soil type, their evaluation results were removed. The R results are generally acceptable, ranging from 0.4–0.7. It is clear that SMOS does not perform well in all metrics. In terms of R, the six RZSM products (ERA5, NOAH, MERRA2, SMAP, Catchment, CFSR) performed better in forest, built-up land and wetland than in croplands and grassland. According to the bias, positive biases were obtained in forest and grassland for all six RZSM datasets. ERA5 overestimated RZSM the most, reaching approximately 0.08 m3 m−3. For the other land types (two types of croplands, wetland and built-up), NOAH, MERRA2 and CFSR exhibit negative biases. However, Catchment and ERA5 still have positive biases, which means they have overall overestimation in RZSM simulations. This can also be seen in . On average, the RMSE in rainfed cropland and in built-up areas is lower than that in the other types. It is the largest for wetland. In addition, it is noted that the performance of the rain-fed cropland is generally better than the irrigated cropland, which probably is because it is difficult to get accurate irrigation information for global models.
4.3. Evaluation on spatial scale
Climate factors are important drivers for RZSM simulation. This means that the performance of the RZSM estimation in different climate zones may be different. Here, we provide validations of the geographical distribution of the seven RZSM products based on 1153 in situ sites, as shown in Fig. S1.
The evaluation metrics in different Köppen climate zones are given in . Overall, the R values in tropical and temperate zones are higher than those in arid and cold zones for all products except SMOS. R median for SMOS is very small (less than 0.1). All products overestimated RZSM in tropical and arid areas except SMOS which has negative bias in arid, temperate and cold zones. It is also noted that ERA5 displays much higher RMSE and positive bias in tropical areas and it overestimated RZSM in all four climate zones. Small biases for MERRA2, NOAH, CFSR and Catchment in temperate and cold zones can be found. In terms of RMSE, it is slightly lower in tropical and arid areas compared with in temperate and cold zones.
4.4. Evaluation in seasonal cycle
The temporal series of the seven RZSM products in different Köppen climate zones are depicted in . On the whole, RZSM dynamics are generally captured by these products except SMOS. However, the change in RZSM from wet to dry from October to March in the arid zone was not reflected very well. Comparatively, SMOS gives the best performance in this case. The results in are consistent with the error metrics shown in that in tropical and arid areas, all products except SMOS overestimated RZSM. ERA5 overestimated RZSM in all four climate zones.
Figure 4. Time series of the seven RZSM products and in situ observations in the four Köppen climate zones during 2018–2019.

To evaluate the ability of these RZSM products to characterize both surplus and deficit soil moisture conditions, we further divided the time series into two terciles, for which the 67%−100% tercile is high soil moisture and the 0%−33% tercile is low soil moisture. When RZSM simulation at the high tercile was also observed, it was considered a hit for wet events. Likewise, when RZSM simulation at the low tercile was also observed, it was considered a hit for dry events. The indicator of hit ratio (the ratio of hits to the dry/wet events observed) was used to assess the ability of these products to capture surplus and deficit soil moisture conditions.
shows the hit rate statistics for every RZSM product in the four climate zones. We can see that MERRA2 exhibits a generally better performance than the other products in capturing surplus and deficit soil moisture conditions overall. 40%−60% wet/dry events can be captured by it. The performance of CFSR is best in the tropical zone but is worst in the arid zone. Similar wet/dry hit rates are obtained between the seven RZSM products for the temperate zone. In general, 30%−60% wet/dry events were characterized by these RZSM products in the tropical zone. 40%−50%, 20%−50% and 30%−50% are characterized for the temperate zone, arid zone and cold zone, respectively.
4.5. Evaluation with dynamic conditions
4.5.1. Soil moisture
presents the evaluation results of the RZSM accuracy with soil moisture in different climate zones. It is obvious that the biases in these four zones commonly decrease from positive to negative with increasing soil moisture. In the case of R, no obvious trends are found. The tropical zone presents highly fluctuating patterns, and the differences between different RZSM products are obvious. R of the seven products in the arid, temperate and cold zones displays larger diversity when RZSM >0.45 m3 m−3. The changes in RMSE with increasing RZSM show a decreasing trend in the tropical zone but show convex shapes for the arid, temperate and cold zones, for which stationary points with minimum RMSE values are approximately 0.2 m3 m−3 in the arid and cold zones and approximately 0.3 m3 m−3 in the temperate zone. Stable ubRMSE changes with increasing RZSM are observed in the tropical, arid and cold zones. The temperate zone exhibits a slight decreasing trend.
4.5.2. Vegetation index
presents the evaluation results of the RZSM accuracy with NDVI (Normalized Difference Vegetation Index) in different climate zones. With the increase in NDVI, the bias decreased from positive to negative in the tropical and arid zones, whereas for the temperate zone, the bias increased from negative to positive. R significantly fluctuates in the tropical and arid zones. Comparatively, it is much more stable in the temperate and cold zones, for which the differences between the seven products are much smaller. In terms of RMSE and ubRMSE, no significant trends with NDVI variability are found. There are larger differences between different products for the tropical zone than for other zones, which is also observed in .
4.5.3. Land surface temperature
presents the evaluation results of the RZSM accuracy with LST (land surface temperature) in different climate zones. The bias likely increases with increasing LST in the tropical zone. Conversely, it decreases with increasing LST in the cold zone. As shown in and , R also presents a fluctuating pattern. According to RMSE and ubRMSE, no obvious change with LST variability is observed. Only a slight increase in RMSE is obtained in the tropical zone. Lower ubRMSE occurs in low and high LST conditions for the arid zone.
5. Discussion
The errors in RZSM simulations by land surface models can stem from various sources, such as data forcing, uncertainties in soil parameters, and physical parameterizations of the model. Of these, precipitation is one of the most influential forcings in RZSM estimation. Different precipitation data used by the seven RZSM products can lead to significant differences in RZSM simulations. To investigate this cause further, we compared the precipitation forcings of NOAH, ERA5, CFSR, and MERRA2 with CN05.1, which was produced using over 2400 meteorological stations and is considered to have higher accuracy. The time series of these precipitation data during 2018–2019 for the Köppen climate zones are shown in Fig.S2. The Nash-Sutcliffe Efficiency (NSE), RMSE, and R between CN05.1 and other precipitation data are given in . MERRA2 shows a much closer relationship with CN05.1 in all four climate zones, with the highest NSE and R and the smallest RMSE. The averages across the four climate zones are 0.88, 0.94, and 1.6 mm/day for NSE, R, and RMSE, respectively. The higher accuracy in precipitation is likely a significant reason for its better RZSM simulations than other RZSM products. ERA5 exhibits a good relationship with CN05.1 in tropical and temperate areas compared with CFSR and NOAH, but a negative NSE is found in arid areas. This is also reflected in Fig. S2, where significant discrepancies between CN05.1 and ERA5 exist, especially in 2018.03–06 and 2019.11. The performance of NOAH precipitation is better than that of CFSR in tropical, arid, and temperate climate zones, but CFSR performs better in cold areas.
Table 2. The relationships between CN05.1 precipitation data and the precipitation forcings of CFSR, ERA5, MERRA2 and NOAH.
RZSM estimation strongly relies on soil properties such as soil organic matter (SOM) and soil texture. Most RZSM products in this study used soil data from HWSD, which has been questioned over the Tibetan Plateau (Bi et al. Citation2016). Shangguan et al. (Citation2013) published soil data that have a more reasonable spatial pattern and magnitude than HWSD, as shown in . To compare the two datasets on a whole-China scale, we compared the data of SOM and sand fraction, which have a close relationship with soil water storage conditions. Results indicate that the SOM of Shangguan et al. (Citation2013) is obviously higher than that of HWSD in most areas, except for desert areas. The sand fraction distribution also shows large differences between the two datasets, with HWSD being lower in western and northern China and Shangguan et al. (Citation2013) being lower in northeast and south China. The averages of SOM and sand fraction over China are 0.59 and 37.1 g/100 g for HWSD, and 1.53 and 42.5 g/100 g for Shangguan et al. (Citation2013), respectively. The limited accuracy of HWSD is likely due to the dataset's minimal use of data from China's national soil surveys in the 1950s and later (Bi et al. Citation2016).
Figure 9. Distributions of soil organic matter and sand fraction over China for datasets of HWSD and Shangguan et al. (Citation2013).

As historical in situ measurements of RZSM are extremely scarce and spatially uneven, evaluations of RZSM products across China are lacking. However, recent studies have assessed the performance of various RZSM products in different regions of China. For instance, the study of Xing et al. (Citation2021) evaluated the RZSM products of SMAPL4, NOAH, and ERA5 over the Qinghai-Tibet Plateau and found that SMAPL4 and NOAH performed better than ERA5. In contrast, another study conducted in the same region by Yang et al. (Citation2022) indicated that ERA5 outperformed NOAH and Catchment, while NOAH outperformed Catchment. The different conclusions related to ERA5 and NOAH in these two studies may be attributed to the use of different soil moisture observation stations.
Furthermore, Fan et al. (Citation2022) evaluated RZSM products using observations from 91 sites distributed in croplands of Jiangsu Province, China, and found that ERA5 and SMAPL4 generally performed better than NOAH, with a higher correlation coefficient (R). Consistent with these findings, our results also showed a higher R of 0.64 for ERA5 () than for other RZSM products. However, in terms of bias, root mean square error (RMSE), and unbiased root mean square error (ubRMSE), ERA5 performed less well than MERRA2, SMAP, and NOAH.
Overall, these studies confirm that RZSM products can capture the temporal evolution of RZSM well. However, the performance of these products varies across different regions and is influenced by factors such as data forcing, soil properties, and model parameterizations.
6. Conclusions
This study conducted a comprehensive validation of seven RZSM datasets, including three reanalysis products (ERA5, MERRA2 and CFSR) and four datasets (SMAP level 4, GLDAS_NOAH2.1, GLDAS_Catchment2.2 and SMOS CATDS Level 4), based on 1153 soil moisture measurement sites across China. The accuracy of the seven datasets was assessed in different Köppen climate zones and on different land cover types. The following key findings were obtained:
All datasets except SMOS achieved Pearson correlation coefficient (R) values of 0.61–0.64 when compared to in situ RZSM measurements. ERA5 exhibited the best performance overall, with the highest R value of 0.64. MERRA2 and NOAH showed almost zero bias, while SMAP and SMOS had negative bias, especially SMOS with a median of −0.084 m3 m−3. ERA5 displayed positive bias of 0.041 m3 m−3. In terms of RMSE or ubRMSE, MERRA2, SMAP, NOAH and Catchment performed better than ERA5, SMOS and CFSR. Overall, MERRA2 and NOAH exhibited better performance.
The accuracy of the datasets was better in forest, built-up land and wetland compared to cropland and grassland, as determined by R. Positive biases were found in forest and grassland for all products except SMOS. ERA5 and Catchment overestimated RZSM simulations in all land cover types, especially ERA5.
At the seasonal scale, all datasets except SMOS were able to capture the temporal dynamics of RZSM well. MERRA2 also showed a better ability to capture surplus and deficit soil moisture conditions, likely due to higher accuracy in precipitation forcing.
The accuracy of RZSM estimates varied with changes in land surface conditions such as soil moisture, NDVI and LST. Biases decreased from positive to negative with increasing soil moisture. No clear patterns were observed in relation to NDVI and LST. The largest differences in accuracy between products were observed in tropical climate zones.
These findings provide valuable information for improving land surface and hydrological models in RZSM estimations, as well as for better monitoring of vegetation growth, agricultural drought and improved agricultural water management.
Disclosure statement
No potential conflict of interest was reported by the author(s).
Data availability
Data available on request due to privacy/ethical restrictions.
Additional information
Funding
References
- Ahmad, Al Bitar, and A. Mahmoodi. 2020. “Algorithm Theoretical Basis Document (ATBD) for the SMOS Level 4 Root Zone Soil Moisture (Version v30_01).” Zenodo, https://doi.org/10.5281/zenodo.4298572.
- Albergel, C., C. Rudiger, T. Pellarin, J. C. Calvet, N. Fritz, F. Froissard, D. Suquia, A. Petitpa, B. Piguet, and E. Martin. 2008. “From Near-Surface to Root-Zone Soil Moisture Using an Exponential Filter: An Assessment of the Method Based on in-Situ Observations and Model Simulations.” Hydrology and Earth System Sciences 12 (6): 1323–1337. https://doi.org/10.5194/hess-12-1323-2008.
- Bell, B., H. Hersbach, A. Simmons, P. Berrisford, P. Dahlgren, A. Horanyi, J. Munoz-Sabater, et al. 2021. “The ERA5 Global Reanalysis: Preliminary Extension to 1950.” Quarterly Journal of the Royal Meteorological Society 147 (741): 4186–4227. https://doi.org/10.1002/qj.4174.
- Bi, H. Y., J. W. Ma, W. J. Zheng, and J. Y. Zeng. 2016. “Comparison of Soil Moisture in GLDAS Model Simulations and in Situ Observations Over the Tibetan Plateau.” Journal of Geophysical Research-Atmospheres 121 (6): 2658–2678. https://doi.org/10.1002/2015jd024131.
- Carranza, C., C. Nolet, M. Pezij, and M. van der Ploeg. 2021. “Root Zone Soil Moisture Estimation with Random Forest.” Journal of Hydrology 593. https://doi.org/10.1016/j.jhydrol.2020.125840.
- Fan, L., Z. P. Xing, G. De Lannoy, F. Frappart, J. Peng, J. Y. Zeng, X. J. Li, et al. 2022. “Evaluation of Satellite and Reanalysis Estimates of Surface and Root-Zone Soil Moisture in Croplands of Jiangsu Province, China.” Remote Sensing of Environment 282. https://doi.org/10.1016/j.rse.2022.113283.
- Grillakis, M. G., A. G. Koutroulis, D. D. Alexakis, C. Polykretis, and I. N. Daliakopoulos. 2021. “Regionalizing Root-Zone Soil Moisture Estimates from ESA CCI Soil Water Index Using Machine Learning and Information on Soil, Vegetation, and Climate.” Water Resources Research 57 (5). https://doi.org/10.1029/2020WR029249.
- Karthikeyan, L., and A. K. Mishra. 2021. “Multi-layer High-Resolution Soil Moisture Estimation Using Machine Learning Over the United States.” Remote Sensing of Environment 266. https://doi.org/10.1016/j.rse.2021.112706.
- Li, Q. L., G. S. Shi, W. Shangguan, V. Nourani, J. D. Li, L. Li, F. N. Huang, et al. 2022. “A 1 km Daily Soil Moisture Dataset Over China Using in Situ Measurement and Machine Learning.” Earth System Science Data 14 (12): 5267–5286. https://doi.org/10.5194/essd-14-5267-2022.
- Liu, J. Y., W. H. Kuang, Z. X. Zhang, X. L. Xu, Y. W. Qin, J. Ning, W. C. Zhou, et al. 2014. “Spatiotemporal Characteristics, Patterns, and Causes of Land-use Changes in China Since the Late 1980s.” Journal of Geographical Sciences 24 (2): 195–210. https://doi.org/10.1007/s11442-014-1082-6.
- Munoz-Sabater, J., E. Dutra, A. Agusti-Panareda, C. Albergel, G. Arduini, G. Balsamo, S. Boussetta, et al. 2021. “ERA5-Land: A State-of-the-art Global Reanalysis Dataset for Land Applications.” Earth System Science Data 13 (9): 4349–4383. https://doi.org/10.5194/essd-13-4349-2021.
- Peng, C. M., M. X. Deng, and L. P. Di. 2014. “Relationships Between Remote-Sensing-Based Agricultural Drought Indicators and Root Zone Soil Moisture: A Comparative Study of Iowa.” Ieee Journal of Selected Topics in Applied Earth Observations and Remote Sensing 7 (11): 4572–4580. https://doi.org/10.1109/Jstars.2014.2344115.
- Reichle, R. H., G. J. M. De Lannoy, Q. Liu, J. V. Ardizzone, A. Colliander, A. Conaty, W. Crow, et al. 2017a. “Assessment of the SMAP Level-4 Surface and Root-Zone Soil Moisture Product Using In Situ Measurements.” Journal of Hydrometeorology 18 (10): 2621–2645. https://doi.org/10.1175/Jhm-D-17-0063.1.
- Reichle, R. H., C. S. Draper, Q. Liu, M. Girotto, S. P. P. Mahanama, R. D. Koster, and G. J. M. De Lannoy. 2017b. “Assessment of MERRA-2 Land Surface Hydrology Estimates.” Journal of Climate 30 (8): 2937–2960. https://doi.org/10.1175/Jcli-D-16-0720.1.
- Reichle, R. H., Q. Liu, R. D. Koster, W. Crow, G. J. M. De Lannoy, J. S. Kimball, J. V. Ardizzone, et al. 2019. “Version 4 of the SMAP Level-4 Soil Moisture Algorithm and Data Product.” Journal of Advances in Modeling Earth Systems 11 (10): 3106–3130. https://doi.org/10.1029/2019ms001729.
- Rodell, M., P. R. Houser, U. Jambor, J. Gottschalck, K. Mitchell, C. J. Meng, K. Arsenault, et al. 2004. “The Global Land Data Assimilation System.” Bulletin of the American Meteorological Society 85 (3): 381–394. https://doi.org/10.1175/Bams-85-3-381.
- Saha, S., S. Moorthi, X. R. Wu, J. Wang, S. Nadiga, P. Tripp, D. Behringer, et al. 2014. “The NCEP Climate Forecast System Version 2.” Journal of Climate 27 (6): 2185–2208. https://doi.org/10.1175/Jcli-D-12-00823.1.
- Shangguan, W., Y. J. Dai, B. Y. Liu, A. X. Zhu, Q. Y. Duan, L. Z. Wu, D. Y. Ji, et al. 2013. “A China Data set of Soil Properties for Land Surface Modeling.” Journal of Advances in Modeling Earth Systems 5 (2): 212–224. https://doi.org/10.1002/jame.20026.
- Tian, J., Y. Q. Zhang, J. P. Guo, X. Z. Zhang, N. Ma, H. S. Wei, and Z. X. Tang. 2022. “Predicting Root Zone Soil Moisture Using Observations at 2121 Sites Across China.” Science of The Total Environment 847. https://doi.org/10.1016/j.scitotenv.2022.157425.
- Tobin, K. J., R. Torres, M. E. Bennett, J. Z. Dong, and W. T. Crow. 2020. “Long-Term Trends in Root-Zone Soil Moisture Across CONUS Connected to ENSO.” Remote Sensing 12 (12). https://doi.org/10.3390/rs12122037.
- Tobin, K. J., R. Torres, W. T. Crow, and M. E. Bennett. 2017. “Multi-decadal Analysis of Root-Zone Soil Moisture Applying the Exponential Filter Across CONUS.” Hydrology and Earth System Sciences 21 (9): 4403–4417. https://doi.org/10.5194/hess-21-4403-2017.
- Wagner, W., G. Lemoine, and H. Rott. 1999. “A Method for Estimating Soil Moisture from ERS Scatterometer and Soil Data.” Remote Sensing of Environment 70 (2): 191–207. https://doi.org/10.1016/S0034-4257(99)00036-X.
- Wu, J., and X. J. Gao. 2013. “A Gridded Daily Observation Dataset Over China Region and Comparison with the Other Datasets.” Chinese Journal of Geophysics-Chinese Edition 56 (4): 1102–1111. https://doi.org/10.6038/cjg20130406.
- Xing, Z. P., L. Fan, L. Zhao, G. De Lannoy, F. Frappart, J. Peng, X. J. Li, et al. 2021. “A First Assessment of Satellite and Reanalysis Estimates of Surface and Root-Zone Soil Moisture Over the Permafrost Region of Qinghai-Tibet Plateau.” Remote Sensing of Environment 265. https://doi.org/10.1016/j.rse.2021.112666.
- Xu, L., P. Abbaszadeh, H. Moradkhani, N. C. Chen, and X. Zhang. 2020. “Continental Drought Monitoring Using Satellite Soil Moisture, Data Assimilation and an Integrated Drought Index.” Remote Sensing of Environment 250. https://doi.org/10.1016/j.rse.2020.112028.
- Xu, L., N. C. Chen, X. Zhang, H. Moradkhani, C. Zhang, and C. L. Hu. 2021. “In-situ and Triple-Collocation Based Evaluations of Eight Global Root Zone Soil Moisture Products.” Remote Sensing of Environment 254. https://doi.org/10.1016/j.rse.2020.112248.
- Yang, S. Q., J. Y. Zeng, W. J. Fan, and Y. K. Cui. 2022. “Evaluating Root-Zone Soil Moisture Products from GLEAM, GLDAS, and ERA5 Based on In Situ Observations and Triple Collocation Method Over the Tibetan Plateau.” Journal of Hydrometeorology 23 (12): 1861–1878. https://doi.org/10.1175/Jhm-D-22-0016.1.
- Zohaib, M., H. Kim, and M. Choi. 2017. “Evaluating the Patterns of Spatiotemporal Trends of Root Zone Soil Moisture in Major Climate Regions in East Asia.” Journal of Geophysical Research-Atmospheres 122 (15): 7705–7722. https://doi.org/10.1002/2016jd026379.