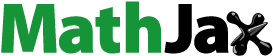
ABSTRACT
Although desert ecosystems are commonly considered to have low carbon storage owing to their sparse vegetation and limited carbon sequestration capacity, they comprise 13.5% of China's terrestrial ecosystems and cover approximately 35% of the Earth's land surface, so they still possess significant carbon storage. The carbon storage of desert ecosystems in China was estimated using MODIS-NDVI data in combination with ground survey, soil census, and literature statistical data. The results indicated that the carbon storage of desert ecosystems in China was 7.063 Pg in 2020. One-meter soil carbon storage accounted for the largest proportion (89.514%), approximately 6.322 Pg, followed by vegetation carbon at 0.741 Pg, and biological soil crust carbon at 0.002 Pg. The carbon density of desert ecosystems was 33.895 Mg.ha−1, with soil carbon density, vegetation carbon density, and biological soil crust carbon density at 30.330, 3.554, and 0.011 Mg.ha−1, respectively. This research provides important evidence for the correct understanding of the carbon storage of desert ecosystems in China.
1. Introduction
Desert ecosystems are representative of arid regions. Harsh environmental conditions, such as high temperature, low rainfall, uneven seasonal distribution of precipitation, and strong soil erosion, have led to poor soil fertility, low soil organic matter content, and low vegetation productivity in desert ecosystems. Therefore, it is generally believed that their carbon sequestration capacities and potentials are very weak. However, desert ecosystems occupy approximately 35% of Earth's land surface, and their total carbon sequestration is still considerable, playing an important role in the global carbon cycle (Liu et al. Citation2022). Similarly, it is the primary ecosystem type in northwest China, accounting for about 13.5% of terrestrial ecosystems in China. Hence, their contributions to China's carbon cycle cannot be ignored (Xu et al. Citation2017). Some studies have suggested that desert ecosystems act as carbon sources, emitting more carbon than sequester (Conant, Klopatek, and Klopatek Citation2000). However, many studies also have indicated that arid regions sequester more carbon than they release, functioning as carbon sinks (Wohlfahrt, Fenstermaker, and Arnone Citation2008; Xie et al. Citation2009; Xu et al. Citation2023).
Desert ecosystems are composed of xeromorphic and ultra-xeromorphic small trees, shrubs, semi-shrubs, and associated animals and microorganisms and are dynamic systems of material and energy flow. This unique desert environment creates extremely sensitive and fragile ecosystem that differs from forests, grasslands, wetlands, and other ecosystems. Owing to the limitations of water resources and environmental conditions, the continuous distribution of vascular plants on the surface is greatly restricted, resulting in a patchy and sparse pattern. This provides favorable conditions for the widespread growth of biological soil crusts (BSCs) (Li et al. Citation2012; Zhang and Wang Citation2010), which can dominate the surface cover in some extremely dry areas, covering more than 70% of the surface (Li Citation2012). As a result, a mosaic distribution pattern emerges between the biological soil crust and vascular plants (Eldridge and Greene Citation1994; West Citation1990). Despite their low primary productivity, desert plants possess the ability to absorb and fix atmospheric CO2 (Ma et al. Citation2021). Additionally, BSCs can also fix carbon through the photosynthesis of cryptogams, such as cyanobacteria, lichens, green algae, and mosses (Li et al. Citation2012; Su, Wu, et al. Citation2013). Soil microorganisms, an important component of BSCs, not only aid in the formation of BSCs and improve biogeochemical cycles, but also significantly enhance soil carbon storage (Liu et al. Citation2012). This makes them an important source of carbon sequestration in desert ecosystems (Elbert et al. Citation2012; Li et al. Citation2012; Petrie et al. Citation2015). In addition, the development of surface BSCs inhibits the release of soil CO2 into the atmosphere, reduces soil carbon emissions, and affects the processes of carbon exchange at the soil-atmosphere interface (Su, Wu, et al. Citation2013; Xu et al. Citation2022). Consequently, BSCs contribute to regional carbon sequestration and have a significant impact on ecosystems carbon cycling.
Accurate estimation of carbon storage in desert ecosystems is advantageous for gaining a better understanding of their carbon sequestration capability, which is vital for comprehending the role of desert ecosystems in the global carbon cycle, achieving carbon peaking and carbon neutrality (Piao, Yue, et al. Citation2022). For a long time, carbon storage in forest ecosystems and grassland ecosystems has been intensively studied, but desert ecosystems have not been studied much. Most existing studies have focused on soil carbon storage and its dynamics (Duan, Liu, and Qn Citation1996; Feng et al. Citation2005; Jia et al. Citation2019; Lal Citation2004). Studies on estimation of vegetation carbon storage have primarily focused on semi-arid and sub-humid arid regions with better vegetation growth (Xu et al. Citation2022; Yan, Wu, and Wang Citation2015; Ye et al. Citation2018; Zandler, Brenning, and Samimi Citation2015), and results differ greatly, which severely hampers our understanding of the size of the carbon pool in desert ecosystems and the characteristics of terrestrial carbon sources or sinks.
However, the integration of big data and the generation and fusion of multiple remote sensing indicators provide necessary data sources and technical support for estimating regional productivity (Cui and Li Citation2022; Li, Lu, and Jia Citation2021). The aboveground biomass of vegetation can reflect the productivity of terrestrial ecosystems and is the basis for studying the relationship between carbon sources and sinks. Remote sensing technology has become the preferred approach for large-scale estimation of vegetation biomass and carbon storage because of its extensive coverage, reliable and repeatable. However, it is challenging to accurately estimate the aboveground biomass of vegetation in desert ecosystems because of the sparse vegetation coverage, significant spatial heterogeneity, low vegetation biomass, and prevalence of dominant species with needle-like or scale-like leaves. Moreover, the extraction of information on sparse desert vegetation is easily affected by the soil background, further reducing the estimation accuracy of the remote sensing model. Although existing research can identify vegetation growth trends and distribution patterns, it has been unable to systematically evaluate vegetation productivity or quantify the size of the carbon pool in desert ecosystems. The accuracy of remote sensing technology for estimating the above-ground biomass of sparse vegetation is limited by various factors, including the quantity of ground survey data, changes in vegetation growth, and spectral resolution. These factors contribute to errors in the remote sensing data and uncertainties in the key parameters of remote sensing models, resulting in low accuracy. Considering the strong correlation between NDVI index and observed data in desertification region, this study used a large amount of ground survey data and remote sensing data to establish a remote sensing estimation model for aboveground biomass of desert ecosystem vegetation based on the vegetation index (NDVI) (Cunliffe, Brazier, and Anderson Citation2016; Mu et al. Citation2016; Sternberg et al. Citation2011; Yan, Wu, and Wang Citation2015; Ye et al. Citation2018; Citation2022; Zandler, Brenning, and Samimi Citation2015), can effectively improve the accuracy of biomass estimation for sparse vegetation and more objectively reflect the actual status of carbon storage in China's desert ecosystems.
In this study, we utilized MODIS-NDVI data, combined with 485 valid field survey quadrat data, 45 soil type data, and a large amount of literature data to estimate the carbon storage and its constituent components in China's desert ecosystems. The objective of this study was to provide a scientific basis for accurately understanding carbon storage in China's desert ecosystems and accurately assessing the role of desert ecosystem carbon pools in addressing climate change.
2. Study area
Desert ecosystems in China are primarily located in inland basins and plateaus between 75–106°E and 35–50°N, from the western end of the Tarim Basin to Helan Mountain. They cover a total area of 2.08 × 106 km2. Desert ecosystems are mainly distributed in Xinjiang, Qinghai, Gansu, Inner Mongolia, Ningxia, and other regions (Liu et al. Citation2020; Zulkar, Zhao, and Jiang Citation2021). There are eight major deserts, and a large area of Gobi, including Taklamakan Desert, Gurbantunggut Desert, Kumtag Desert, Qaidam Basin Desert, Badain Jaran Desert, Tengger Desert, Ulan Buh Desert, and Hobq Desert.
This region is located deep in the Eurasian continent and is less affected by the East Asian summer monsoon. Moist air from the ocean is blocked by mountains, resulting in a typical temperate dry arid desert climate. The area has an annual precipitation below 200 mm and is categorized as arid and extremely arid regions (Lu et al. Citation2022). Precipitation is unevenly distributed throughout the year, with the majority occurring between June and August (summer) and accounting for more than half of the total annual precipitation.
3. Data sources and methods
3.1. Method for estimating vegetation carbon storage aboveground biomass
3.1.1. Ground survey
Considering the distribution area of desert ecosystems and the difficulty of data collection, we carefully selected specific representative areas to collect ground vegetation data. These areas covered both arid regions and extremely arid regions, including the northeast region of the Ulan Buh Desert, the southeast region of the Tengger Desert, the surrounding areas of the Kumtag Desert, and the Hami Depression (). The dominant vegetation in these areas consists of shrubs and semi-shrubs such as Nitraria tangutorum, and Reaumuria songarica. Additionally, herbaceous plants such as Agriophyllum squarrosum, Grubovia dasyphylla, Salsola laricifolia are also present in these regions.
A quadrat (30 m × 30 m) sample plot method was used for the field investigation. The investigation included topographical features, soil characteristics, elevation, GPS coordinates of the central point, and vegetation growth status. Additionally, the base diameter (D), crown length axis (C1), short axis (C2), and height (H) of each shrub within the sample plot were measured and recorded. We selected various healthy plants of different sizes and species from the study area. The stem diameter, height, crown length (axis), and short axis were recorded. Whole plants were clipped to the ground, weighed, and transported to the laboratory for drying in a ventilated oven at 80°C for 48 h. Based on ground sample plot survey data, the individual aboveground volume of each shrub was calculated using the basal diameter (D), canopy area (A = πC1×C2/4), and plant height (H) as predictor variables to construct an allometric equation between aboveground volume and biomass dry weight for each shrub species. The coefficient of determination (R2) and mean absolute error (MAE) were used to compare and evaluate the accuracy of the allometric equation, and an optimal allometric equation was obtained. The allometric equation was used to derive the unit area aboveground biomass of shrubs from the survey data of each sample plot (Yao et al. Citation2021; Ye et al. Citation2018; Citation2022). A total of 628 plots of ground vegetation were investigated in arid and extremely arid areas from August to September 2007 to 2020.
For plots with herbaceous plants, three 1 m × 1 m subplots were randomly placed along a diagonal line, and the aboveground biomass was measured using the harvesting method.
3.1.2. Preparation of remote sensing data
MOD13Q1 data derived from MODIS satellite imagery with a spatial resolution of 250 m and a temporal resolution of 16 days were used in this study. The annual vegetation information from 2007 to 2020 was extracted, representing the growing season from the 145th to 289th day (May to September) of each year. MRT software was used to extract NDVI within a confidence band after image stitching and mask processing. There were ten MOD13Q1 images taken during the growing season every year, and the highest value for each pixel was selected as the indicator of the vegetation index data of the year, which was expressed as MODIS-NDVI to estimate the aboveground biomass of desert vegetation. The acquisition time of all the remote sensing data was matched with the vegetation survey time.
3.1.3. Model construction and accuracy verification
The GPS coordinates of all the ground vegetation quadrats were superimposed on the MODIS-NDVI images in the same year as the survey time of the ground quadrats and compared with Google Earth images. Quadrats containing land cover types, such as cultivated land, water bodies, or towns were excluded from the pixels of the ground quadrats. Sample plots located in pixels containing land cover types, such as croplands, water bodies, or urban areas, were excluded after comparing them with Google Earth images. After image screening, 485 effective quadrats were finally determined from 628 ground vegetation quadrats, among which 259 were from 311 quadrats in the arid zone and 226 were from 317 quadrats in extremely arid zone. Data from 70% of the valid ground survey sample plots were randomly selected using regression analysis to establish a correlation between the vegetation index and the measured aboveground biomass. The estimation models of aboveground biomass for both arid and extremely arid regions were obtained (), and the remaining 30% of the valid ground survey sample plots were used to evaluate the accuracy of the estimation results. The logarithmic model of MODIS-NDVI (p < 0.001, R2 = 0.70) was determined to be the most suitable for estimating aboveground biomass in arid regions ((b)), while the linear model of MODIS-NDVI (p < 0.1, R2 = 0.67) was found to be optimal for extremely arid regions ((a)).
3.2. Methods for estimating soil carbon storage
Based on the Second Soil Resources Census in China, soil organic matter content, gravel (particle size > 2 mm) content, and bulk density data of soil profiles at different depths were collected in accurately located desert areas (Office National Soil Survey Citation1998). The soil thickness as the weighting coefficient, average thickness (with a maximum calculated thickness of 1 m), average organic matter, and average bulk density of all soil types and subtypes were obtained by the weighted average. After obtaining the corresponding parameters, the total organic carbon storage of each soil type was calculated using the following formula:
where j represents the soil type, Cj refers to the carbon storage (t), Sj represents the total area (hm2), Hj is the average thickness (cm), Oj is the average organic matter content (%), Wj represents the average bulk density (g.cm−3), and 0.58 is the conversion coefficient between soil organic matter and organic carbon. The area (Sj) for each soil type in the arid and extremely arid regions was obtained from the 1:1,000,000 soil-type distribution map of China from the Chinese Resource and Environmental Database.
3.3. Methods for estimating of biological soil crusts carbon storage
The estimation of biological soil crust carbon storage follows the same methodology as soil carbon storage. The carbon density of the biological soil crusts was based on published data. If thickness and bulk density data were unavailable from the literature, average values for algae, lichens, and mosses were used as substitutes. Previous studies have shown that biological soil crusts account for 40%−70% of fixed and semi-fixed sand dunes in desert ecosystems (Li Citation2012). In view of the lack of accurate statistical data on the crust coverage of the entire desert ecosystems, the distribution area of biological soil crusts of desert ecosystems in China was estimated using 40% of the area of fixed and semi-fixed sand dunes as a baseline, as provided in the Atlas of Chinese Deserts (Administration State Forestry Citation2018).
4. Results and analysis
4.1. Vegetation biomass
The aboveground biomass of desert vegetation was estimated separately in arid and extremely arid regions using the established remote sensing estimation model based on MODIS-NDVI data from 2020. The estimated aboveground biomass of desert vegetation in China is approximately 0.228 Pg, with approximately 0.203 Pg in arid regions and 0.025 Pg in extremely arid regions.
Huang (Huang et al. Citation2006) simulated the spatial distribution patterns of total vegetation biomass, above- and below-ground biomass, and root-to-shoot ratio in China using the Atmospheric Vegetation Interaction Model (AVIM2). Based on the ratio of roots, stems and leaves in different desert communities in China, the average root-shoot ratio of desert vegetation was determined to be 5.5 (Huang et al. Citation2006), which was used to estimate below-ground biomass in our study. In 2020, the total biomass of desert ecosystems in China was approximately 1.481 Pg, with arid areas contributing 88.86% ().
4.2. Soil organic carbon storage
Organic carbon density for each soil type was calculated in arid and extremely arid regions using data from The Second Soil Resources Census. The soil organic carbon storage of desert ecosystems in China was approximately 6.320 Pg.
4.3. Biological soil crusts carbon storage
The carbon density of biological soil crust in desert ecosystems exhibits significant spatial heterogeneity, primarily influenced by topography and vegetation. The carbon density was higher in inter-dune areas and areas with vegetation cover, especially in fenced areas where artificial sand-fixing vegetation was planted (). In order to reflect more accurately the average carbon storage in biological soil crust, we collected crusts data from literature in desert ecosystems worldwide and calculated carbon density. Globally, biological soil crust carbon density ranged from 3.05 g.m−2 to 181.58 g.m−2, with a median value of 49.26 g.m−2 and an average value of 61.66 g.m−2. Desert ecosystems in China, the biological soil crust carbon density ranged from 20.88 g.m−2 to 181.58 g.m−2, with a median value of 60.65 g.m−2 and an average value of 74.60 g.m−2 (). A higher carbon density of biological soil crust appeared in vegetation areas of the artificially stabilized dune in arid regions, ranging from 46.95 g.m−2 to 181.58 g.m−2. Based on the average carbon density of global biological soil crust and 40% of the fixed and semifixed dune areas in the Atlas of Sandy Deserts in China (2.083 × 106 km2) (Administration State Forestry Citation2018; Li Citation2012; Li, Hui, and Zhao Citation2016), the carbon storage of biological soil crusts of desert ecosystems in China was approximately 0.002 Pg.
Table 1. The carbon density of biological soil crusts in desert ecosystems all over the world.
4.4. Carbon storage in desert ecosystems
After applying a conversion coefficient of 0.50 to the total vegetation biomass, the vegetation carbon storage of desert ecosystems in China was estimated to be approximately 0.741 Pg by 2020. Additionally, the soil organic carbon storage was 6.320 Pg, while the biological soil crust carbon storage was 0.002 Pg. The total carbon storage of desert ecosystems in China was 7.063 Pg. Based on the total area of 2.083 × 106 km2 of desert ecosystems in China, the carbon density of desert ecosystems was 33.895 Mg ha−1. The carbon densities of vegetation, soil, and biological soil crusts were 3.554 Mg ha−1, 30.330 Mg ha−1, and 0.011 Mg ha−1 respectively.
5. Discussion
China's terrestrial ecosystems have served and will continue to serve as CO2 sinks for a long time in the future (Piao, He, et al. Citation2022), but the contributions of different ecosystems vary greatly (Yang et al. Citation2022). At present, the resource inventory method, a classical method for estimating terrestrial ecosystems carbon storage, is widely used because it can separate the contribution of vegetation and soil to the sources and sinks of ecosystems in a certain period. However, the coverage density of ground survey data is a crucial limiting factor for the accuracy of this method (Piao, Yue, et al. Citation2022). By integrating extensive ground survey, literature, and national soil census data, the carbon pools of desert ecosystems in China were accurately estimated in this study. The total carbon pool (7.063 Pg) in this study was significantly higher than that estimated using empirical models for sparse herbaceous deserts (3.450 Pg), which accounts for approximately 7%−8% of the carbon pool in China's terrestrial ecosystems (Wang et al. Citation2021). The soil carbon pool in this study was higher (6.320 Pg) than sparse herbaceous desert (2.480 Pg) and the vegetation carbon pool (0.741 Pg) was lower than sparse herbaceous desert (0.970 Pg). In addition to differences in vegetation type, vegetation coverage, and productivity, environmental conditions such as high temperature, low precipitation, and low soil moisture are important constraints in slowing down the decomposition process of soil organic matter in desert ecosystems (Yang et al. Citation2022). Although the carbon pool of desert ecosystems in China is significantly lower than that of forests and grasslands (Yang et al. Citation2022), it is not lower than shrubland ecosystems (6.700 Pg) (Tang et al. Citation2018) or wetland ecosystems (5.4∼7.3 Pg) (Zheng et al. Citation2013). Further analysis revealed that the desert ecosystem vegetation carbon pool (0.741 Pg) was higher than that in wetlands (0.22 Pg) and shrubland (0.4∼0.7 Pg) estimated by the inventory method in China. Even though over 90% of the carbon in desert ecosystems is stored in the soil, a relatively smaller value is the primary reason for their low contribution to the global terrestrial ecosystem carbon pool.
Vegetation carbon contributed 10.49% of the total carbon pool in desert ecosystems in China, which was 6.09% higher than the vegetation carbon contribution (4.40%) in global desert ecosystems, whereas the corresponding contribution of soil carbon storage was lower by 6.12% (Carvalhais et al. Citation2014). There are two reasons for this finding. First, a uniform soil depth of one meter was used to estimate global ecosystem carbon storage (Carvalhais et al. Citation2014), whereas this study considered different soil depths due to soil types. Affected by geomorphic and geological processes, desert soils in China are mostly less than 100 cm thick, with sierozem having the highest thickness (104.4 cm) and chisley soil the lowest (11.7 cm). Second, arid regions are the key area of ecological engineering construction in China, especially windbreak forests, sand-fixation forests, and farmland shelterbelts, which not only improve and protect the ecological environment but also play an important role in improving regional vegetation coverage and productivity (Lu et al. Citation2018). Vegetation restoration and ecological restoration carried out can not only increase vegetation carbon pool, but also improve soil texture to increase soil carbon pool, while the increase rate of soil carbon pool is much slower than that of vegetation. Arid regions with better climate conditions contributed 88.86% of the vegetation carbon storage to desert ecosystems in China. Therefore, the protection and restoration of desert ecosystems have a great impact on improving regional environment, increasing carbon sink capacity and enhancing the role of desert ecosystems in carbon peaking and carbon neutrality (Piao, Yue, et al. Citation2022; Lu et al. Citation2018; Yang et al. Citation2022). Future climate change scenarios, such as precipitation increase and CO2 fertilization, will boost vegetation coverage and productivity in desert areas (Brandt et al. Citation2019; Donohue et al. Citation2013; Li et al. Citation2015). This will enhance the contribution of desert ecosystems to the carbon sources and sinks of terrestrial ecosystems, particularly because of their high sensitivity to extreme climate or significant changes in inter-annual precipitation (Deng et al. Citation2021; Poulter et al. Citation2014).
Biological soil crusts, as a main component of desert ecosystems, inlay vascular plants, forming a stable state through competition for limited resources. It has been widely proven that biological soil crusts play a significant role in carbon sequestration (Elbert et al. Citation2012; Li et al. Citation2012; Liu et al. Citation2020). At regional scales, the carbon density of biological soil crusts exhibited high spatial heterogeneity under different climatic conditions (). Topography and vegetation are the main influencing factors at a small scale (Li et al. Citation2010). The stabilized land surface, dust capture, precipitation redistribution, and organic matter input all contribute to the development of biological soil crust resulting in a relatively higher carbon density in the artificial sand-fixing vegetation areas (). In desert ecosystems, the biological crust is discontinuous, mostly distributed under shrubs, and black or gray under drought conditions. Remote sensing analysis is easily affected by the shrub canopy and soil background values. Large-scale remote sensing monitoring of biological soil crust coverage is relatively lacking, and there have been only exploration attempts in the Mu Us Sandland and Gurbantunggut Desert (Bu et al. Citation2016; Wang et al. Citation2022; Zan Citation2012). It is challenging to quantitatively estimate the carbon storage of biological soil crusts, despite their importance in desert ecosystem carbon cycling cannot be ignored. The carbon storage of biological soil crusts in desert ecosystems is approximately 0.002 Pg, accounting for less than 1/1000 of the entire desert ecosystem’s carbon pool (Tang et al. Citation2018). Their relatively low contribution is due to the low carbon density resulting from their thin layer (Li, Fu et al. Citation2021; Li, Hui, and Zhao Citation2016; Weber, Büdel, and Belnap Citation2016). Nevertheless, the thin layer plays an essential role in stabilizing the land surface, enhancing nutrient content, reducing erosion, and sequestering CO2 (Li, Hui, and Zhao Citation2016; Weber, Büdel, and Belnap Citation2016), particularly in increasing soil carbon storage. It cannot be denied that biological soil crust is a key component of soil organic carbon storage in extreme arid areas with very minimal vascular plants cover and soil organic carbon content (Monus et al. Citation2023). Even a small change in biological soil crusts can affect carbon exchange between vegetation and soil and atmospheric interference by affecting its ecological functions, leading to changes in the carbon or sink capacity of the ecosystem. Therefore, it would not be appropriate to ignore biological soil crusts carbon storage contribution in the calculation of carbon budgets for desert ecosystems.
6. Conclusion
The carbon storage capacity of desert ecosystems in China was 7.063 Pg in 2020, with a carbon density of 33.895 Mg.ha−1. Soil carbon storage accounted for the majority of the total, accounting for 89.48%, with a carbon storage of 6.320 Pg and a carbon density of 30.330 Mg.ha−1. Vegetation was the second largest contributor, with a carbon storage of approximately 0.741 Pg and a carbon density of 3.554 Mg.ha−1. Biological soil crusts had the smallest contribution, with a carbon storage of 0.002 Pg and carbon density of 0.011 Mg.ha−1. Although desert ecosystems have sparse vegetation and a relatively low carbon density, their vast areas make their total carbon storage significant. Therefore, an accurate estimation of carbon storage is crucial for achieving China's carbon peak and carbon neutrality goals. Despite some uncertainties in the estimation results, they can still provide valuable insights into the carbon storage of desert ecosystems to a certain degree.
Disclosure statement
No potential conflict of interest was reported by the author(s).
Data availability statement
The data supporting the findings of this study are available upon request from the corresponding author.
Additional information
Funding
References
- Administration State Forestry. 2018. Atlas of Sandy Deserts in China. Beijing: Science Press.
- Beraldi-campesi, Hugo, Hilairy Hartnett, Ariel Anbar, Gwyneth Gordon, and Ferran Garcia-Pichel. 2009. “Effect of Biological Soil Crusts on Soil Elemental Concentrations: Implications for Biogeochemistry and as Traceable Biosignatures of Ancient Life on Land.” Geobiology 7 (3): 348–359. https://doi.org/10.1111/j.1472-4669.2009.00204.x.
- Brandt, Martin, Pierre Hiernaux, Kjeld Rasmussen, Compton J. Tucker, Jean-Pierre Wigneron, Abdoul Aziz Diouf, Stefanie M. Herrmann, et al. 2019. “Changes in Rainfall Distribution Promote Woody Foliage Production in the Sahel.” Communications Biology 2 (1). https://doi.org/10.1038/s42003-019-0383-9.
- Bu, Chongfeng, Peng Zhang, Chun Wang, Yongsheng Yang, Hongbo Shao, and Shufang Wu. 2016. “Spatial Distribution of Biological Soil Crusts on the Slope of the Chinese Loess Plateau Based on Canonical Correspondence Analysis.” Catena 137:373–381. https://doi.org/10.1016/j.catena.2015.10.016.
- Cantón, Yolanda, Jose Raúl Román, Sonia Chamizo, Emilio Rodríguez-Caballero, and María José Moro. 2014. “Dynamics of Organic Carbon Losses by Water Erosion after Biocrust Removal.” Journal of Hydrology and Hydromechanics 62 (4): 258–268. https://doi.org/10.2478/johh-2014-0033.
- Carvalhais, Nuno, Matthias Forkel, Myroslava Khomik, Jessica Bellarby, Martin Jung, Mirco Migliavacca, Mingquan Μu, et al. 2014. “Global Covariation of Carbon Turnover Times with Climate in Terrestrial Ecosystems.” Nature 514 (7521): 213–217. https://doi.org/10.1038/nature13731.
- Chamizo, Sonia, Yolanda Cantón, Isabel Miralles, and Francisco Domingo. 2012. “Biological Soil Crust Development Affects Physicochemical Characteristics of Soil Surface in Semiarid Ecosystems.” Soil Biology and Biochemistry 49:96–105. https://doi.org/10.1016/j.soilbio.2012.02.017.
- Conant, Richard T., Jeffrey M. Klopatek, and Carole C. Klopatek. 2000. “Environmental Factors Controlling Soil Respiration in Three Semiarid Ecosystems.” Soil Science Society of America Journal 64 (1): 383–390. https://doi.org/10.2136/sssaj2000.641383x.
- Cui, Yuran, and Xiaosong Li. 2022. “A New Global Land Productivity Dynamic Product Based on the Consistency of Various Vegetation Biophysical Indicators.” Big Earth Data 6 (1): 36–53. https://doi.org/10.1080/20964471.2021.2018789.
- Cunliffe, Andrew M., Richard E. Brazier, and Karen Anderson. 2016. “Ultra-fine Grain Landscape-scale Quantification of Dryland Vegetation Structure with Drone-acquired Structure-from-motion Photogrammetry.” Remote Sensing of Environment 183:129–143. https://doi.org/10.1016/j.rse.2016.05.019.
- Deng, Ying, Xuhui Wang, Kai Wang, Philippe Ciais, Shuchang Tang, Lei Jin, Lili Li, and Shilong Piao. 2021. “Responses of Vegetation Greenness and Carbon Cycle to Extreme Droughts in China.” Agricultural and Forest Meteorology 298–299:108307. https://doi.org/10.1016/j.agrformet.2020.108307.
- Donohue, Randall J., Michael L. Roderick, Tim R. McVicar, and Graham D. Farquhar. 2013. “CO2 Fertilisation has Increased Maximum Foliage Cover Across the Globe's Warm, Arid Environments.” Geophysical Research Letters 40 (12): 3031–3035. https://doi.org/10.1002/grl.50563.
- Drahorad, S., P. Felix-Henningsen, K. U. Eckhardt, and P. Leinweber. 2013. “Spatial Carbon and Nitrogen Distribution and Organic Matter Characteristics of Biological Soil Crusts in the Negev Desert (Israel) Along a Rainfall Gradient.” Journal of Arid Environments 94:18–26. https://doi.org/10.1016/j.jaridenv.2013.02.006.
- Du, Jun, Yixuan Li, Xiaoxia Yang, Yunfei Li, and Xiaojun Ma. 2018. “Effects of Biological Soil Crusts Types on Soil Physicochemical Properties in the Southeast Fringe of the Tengger Desert.” Journal of Desert Research 38 (1): 111–116. https://doi.org/10.7522/j.issn.1000-694X.2017.00073.
- Duan, Zhenghu, Xinming Liu, and Jianjun Qn. 1996. “Atmospheric CO2 Content Affected By Desertification in China.” Journal of Arid Land Resources and Environment 10 (2). CNKI:SUN:GHZH.0.1996-02-012.
- Elbert, Wolfgang, Bettina Weber, Susannah Burrows, Jörg Steinkamp, Burkhard Büdel, Meinrat O. Andreae, and Ulrich Pöschl. 2012. “Contribution of Cryptogamic Covers to the Global Cycles of Carbon and Nitrogen.” Nature Geoscience 5 (7): 459–462. https://doi.org/10.1038/ngeo1486.
- Eldridge, D. J., and R. S. B. Greene. 1994. “Microbiotic Soil Crusts: A Review of their Roles in Soil and Ecological Processes in the Rangelands of Australia.” Australian Journal of Soil Research 32 (3): 389–415. https://doi.org/10.1071/SR9940389.
- Feng, Qi, Yonghong Su, Wei Liu, Jianhua Shi, Zongqiang Chang, and Yanwu Zhang. 2005. “Carbon Storage in the Desertified Land: A Case Study in North China.” Paper Presented at the 3rd International Nitrogen Conference: Contributed Papers, Lanzhou.
- Huang, Mei, JinJun Ji, MingKui Cao, and KeRang Li. 2006. “Modeling Study of Vegetation Shoot and Root Biomass in China.” Acta Ecologica Sinica 26 (12): 4156–4163.
- Jia, Xiao Hong, Yuan Shou Li, Wu Bo, Lu Qi, and Li Xin Rong. 2019. “Plant Restoration Leads to Divergent Sequestration of Soil Carbon and Nitrogen in Different Fractions in an Arid Desert Region.” Land Degradation and Development 30 (18): 2197–2220. https://doi.org/10.1002/ldr.3416.
- Lal, Rattan. 2004. “Carbon Sequestration in Dryland Ecosystems.” Environmental Management 33 (4): 528–544. https://doi.org/10.1007/s00267-003-9110-9.
- Li, Xinrong. 2012. Eco-hydrology of Biological Soil Crusts in Desert Regions of China. Beijing: Higher Education Press.
- Li, Zhi, Yaning Chen, Weihong Li, Haijun Deng, and Gonghuan Fang. 2015. “Potential Impacts of Climate Change on Vegetation Dynamics in Central Asia.” Journal of Geophysical Research: Atmospheres 120 (24): 12345–12356. https://doi.org/10.1002/2015JD023618.
- Li, Changjia, Bojie Fu, Shuai Wang, Lindsay C. Stringer, Yaping Wang, Zidong Li, Yanxu Liu, and Wenxin Zhou. 2021. “Drivers and Impacts of Changes in China's Drylands.” Nature Reviews Earth & Environment 2:858–873. https://doi.org/10.1038/s43017-021-00226-z.
- Li, Xinrong, Minzhu He, Stefan Zerbe, Xiaojun Li, and Lichao Liu. 2010. “Micro-geomorphology Determines Community Structure of Biological Soil Crusts at Small Scales.” Earth Surface Processes and Landforms 35 (8): 932–940. https://doi.org/10.1002/esp.1963.
- Li, Xinrong, Rong Hui, and Yang Zhao. 2016. Eco-physiology of Biological Soil Crusts in Desert Regions of China. Beijing: Higher Education Press.
- Li, Xiaosong, Qi Lu, and Xiaoxia Jia. 2021. “Harnessing Big Earth Data to Facilitate Land Degradation Neutrality Goals—Practices and Prospects.” Bulletin of Chinese Academy of Sciences 36:896–903. https://doi.org/10.16418/j.issn.1000-3045.20210705002.
- Li, Xinrong, Xinping Wang, Tao Li, and Jingguang Zhang. 2002. “Microbiotic Soil Crust and its Effect on Vegetation and Habitat on Artificially Stabilized Desert Dunes in Tengger Desert, North China.” Biology and Fertility of Soils 35 (3): 147–154. https://doi.org/10.1007/s00374-002-0453-9.
- Li, Bin, Zhifang Wu, Ye Tao, Xiaobing Zhou, and Bingchang Zhang. 2021. “Effects of Biological Soil Crust Type on Herbaceous Diversity in the Gurbantunggut Desert.” Arid Zone Research 38 (2): 438–449. https://doi.org/10.13866/j.azr.2021.02.15.
- Li, Xinrong, Peng Zhang, Yangui Su, and Rongliang Jia. 2012. “Carbon Fixation by Biological Soil Crusts Following Revegetation of Sand Dunes in Arid Desert Regions of China: A Four-year Field Study.” Catena 97:119–126. https://doi.org/10.1016/j.catena.2012.05.009.
- Liu, Yanmei, Xinrong Li, Mingzhu He, Rongliang Jia, Li Xiaojun, and Zhang Zhishan. 2012. “Effect of Biological Soil Crusts on Soil Microbial Biomass Carbon Content.” Journal of Desert Research 32 (3): 669–673. http://ir.casnw.net/handle/362004/11553.
- Liu, Zhen, Yanfei Sun, Yuqing Zhang, Wei Feng, Zongrui Lai, and Shugao Qin. 2020. “Soil Microbes Transform Inorganic Carbon into Organic Carbon by Dark Fixation Pathways in Desert Soil.” Journal of Geophysical Research-biogeosciences. https://doi.org/10.1002/essoar.10504121.1.
- Liu, Hongyan, Chongyang Xu, Craig D. Allen, Henrik Hartmann, Xiaohua Wei, Dan Yakir, Xiuchen Wu, and Pengtao Yu. 2022. “Nature-based Framework for Sustainable Afforestation in Global Drylands under Changing Climate.” Global Change Biology 28 (7): 2202–2220. https://doi.org/10.1111/gcb.16059.
- Lu, Fei, Huifeng Hu, Wenjuan Sun, Jiaojun Zhu, Guobin Liu, Wangming Zhou, Quanfa Zhang, et al. 2018. “Effects of National Ecological Restoration Projects on Carbon Sequestration in China from 2001 to 2010.” Proceedings of the National Academy of Sciences 115 (16): 4039–4044. https://doi.org/10.1073/pnas.1700294115.
- Lu, Chang, Long Ma, Tingxi Liu, and Xing Huang. 2022. “Temporal and Spatial Variations of Annual Precipitation and Meteorological Drought in China during 1951–2018.” Chinese Journal of Applied Ecology 33. https://doi.org/10.13287/j.1001-9332.202206.022.
- Ma, Quanlin, Xinyou Wang, Fang Chen, Linyuan Wei, Dekui Zhang, and Hujia Jin. 2021. “Carbon Sequestration of Sand-fixing Plantation of Haloxylon Ammodendron in Shiyang River Basin: Storage, Rate and Potential.” Global Ecology and Conservation 28:1–19. https://doi.org/10.1016/j.gecco.2021.e01607.
- Miralles, Isabel, Francisco Domingo, Yolanda Cantón, Carmen Trasar-Cepeda, M. Carmen Leirós, and Fernando Gil-Sotres. 2012. “Hydrolase Enzyme Activities in a Successional Gradient of Biological Soil Crusts in Arid and Semi-arid Zones.” Soil Biology and Biochemistry 53:124–132. https://doi.org/10.1016/j.soilbio.2012.05.016.
- Monus, Brittney D., Elise N. Nghalipo, Vimbai J. Marufu, Ferran Garcia-Pichel, and Heather L. Throop. 2023. “Contributions of Hypolithic Communities to Surface Soil Organic Carbon Across a Hyperarid-to-arid Climate Gradient.” GEODERMA 433:116428. https://doi.org/10.1016/j.geoderma.2023.116428.
- Mu, Qier, Zhihai Gao, Yuhai Bao, Bengyu Wang, and Lina Bai. 2016. “Estimation of Sparse Vegetation Biomass Based on Grey-level Co-occurrence Matrix of Vegetation Indices.” Remote Sensing Information 31 (1): 58–63. https://doi.org/10.3969/j.issn.1000-3177.2016.01.009.
- Niu, Jiping, Kai Yang, Zejun Tang, and Yitong Wang. 2017. “Relationships between Soil Crust Development and Soil Properties in the Desert Region of North China.” Sustainability 9 (5): 725. https://doi.org/10.3390/su9050725.
- Office National Soil Survey. 1998. Chinese Soil. Beijing: China Agricultural Press.
- Petrie, M. D., S. L. Collins, A. M. Swann, P. L. Ford, and M. E. Litvak. 2015. “Grassland to Shrubland State Transitions Enhance Carbon Sequestration in the Northern Chihuahuan Desert.” Global Change Biology 21 (3): 1226–1235. https://doi.org/10.1111/gcb.12743.
- Piao, Shilong, Yue He, Xuhui Wang, and Fahu Chen. 2022. “Estimation of China's Terrestrial Ecosystem Carbon Sink: Methods, Progress and Prospects.” Scientia Sinica(Terrae) 52 (6): 1010–1020. https://doi.org/10.1007/s11430-021-9892-6.
- Piao, Shilong, Chao Yue, Jinzhi Ding, and Zhengtang Guo. 2022. “Role of Terrestrial Ecosystem Carbon Sequestration in ‘Carbon Neutralization’ Target.” Scientia Sinica(Terrae) 52 (7): 1419–1426. https://doi.org/10.1007/s11430-022-9926-6.
- Poulter, Benjamin, David Frank, Philippe Ciais, Ranga B. Myneni, Niels Andela, Jian Bi, Gregoire Broquet, et al. 2014. “Contribution of Semi-arid Ecosystems to Interannual Variability of the Global Carbon Cycle.” Nature 509 (7502): 600–603. https://doi.org/10.1038/nature13376.
- Ram, Almog, and Yair Aaron. 2007. “Negative and Positive Effects of Topsoil Biological Crusts on Water Availability Along a Rainfall Gradient in a Sandy Arid Area.” Catena 70 (3): 437–442. https://doi.org/10.1016/j.catena.2006.11.012.
- Sternberg, Troy, Renchin Tsolmon, Nicholas Middleton, and David Thomas. 2011. “Tracking Desertification on the Mongolian Steppe Through NDVI and Field-survey Data.” International Journal of Digital Earth 4 (1): 50–64. https://doi.org/10.1080/17538940903506006.
- Su, Yangui, Xinrong Li, Yingwu Chen, Zhishan Zhang, and Yan Lu. 2013. “Carbon Fixation of Cyanobacterial-Algal Crusts After Desert Fixation and its Implication to Soil Organic Carbon Accumulation in Desert.” Land Degradation & Development 24 (4): 342–349. https://doi.org/10.1002/ldr.1131.
- Su, Yangui, Lin Wu, and Yuanming Zhang. 2012. “Characteristics of Carbon Flux in two Biologically Crusted Soils in the Gurbantunggut Desert, Northwestern China.” Catena 96:41–48. https://doi.org/10.1016/j.catena.2012.04.003.
- Su, Yangui, Lin Wu, Zhibin Zhou, Yaobin Liu, and Yuanming Zhang. 2013. “Carbon Flux in Deserts Depends on Soil Cover Type: A Case Study in the Gurbantunggute Desert, North China.” Soil Biology and Biochemistry 58:332–340. https://doi.org/10.1016/j.soilbio.2012.12.006.
- Tang, Xuli, Xia Zhao, Yongfei Bai, Zhiyao Tang, Wantong Wang, Yongcun Zhao, Hongwei Wan, et al. 2018. “Carbon Pools in China’s Terrestrial Ecosystems: New Estimates Based on an Intensive Field Survey.” Proceedings of the National Academy of Sciences 115 (16): 4021–4026. https://doi.org/10.1073/pnas.1700291115.
- Wang, Li Xia, Ji Xi Gao, Wen Ming Shen, Yuan Li Shi, and Hong Wei Zhang. 2021. “Carbon Storage in Vegetation and Soil in Chinese Ecosystems Estimated by Carbon Transfer Rate Method.” Ecosphere (Washington, D.C) 12 (1). https://doi.org/10.1002/ecs2.3341.
- Wang, Zhengdong, Bingfang Wu, Miao Zhang, Hongwei Zeng, Leidong Yang, Fuyou Tian, Zonghan Ma, and Hantian Wu. 2022. “Indices Enhance Biological Soil Crust Mapping in Sandy and Desert Lands.” Remote Sensing of Environment 278:113078. https://doi.org/10.1016/j.rse.2022.113078.
- Weber, Bettina, Burkhard Büdel, and Jayne Belnap. 2016. Biological Soil Crusts: An Organizin Principle in Drylands. Swizerland: Springer International Publishing.
- West, Neil E. 1990. “Structure and Function of Microphytic Soil Crusts in Wildland Ecosystems of Arid to Semi-arid Regions.” 179–223.
- Wohlfahrt, Georg, Lynn F. Fenstermaker, and John A. Arnone III. 2008. “Large Annual net Ecosystem CO₂ Uptake of a Mojave Desert Ecosystem.” Global Change Biology 14 (7): 1475–1487. https://doi.org/10.1111/j.1365-2486.2008.01593.x.
- Xie, Ting, Yunfei Li, and Xiaojun Li. 2021. “Organic Carbon Mineralization of Biological Soil Crusts and Subsoils in the Revegetated Areas of the Southeast Fringe of the Tengger Desert.” Acta Ecologica Sinica 41 (6): 2339–2348. https://doi.org/10.5846/stxb202001040027.
- Xie, Jingxia, Yan Li, Cuixia Zhai, Chenhua Li, and Zhongdong Lan. 2009. “CO2 Absorption by Alkaline Soils and its Implication to the Global Carbon Cycle.” Environmental Geology 56 (5): 953–961. https://doi.org/10.1007/s00254-008-1197-0.
- Xu, Xinliang, Jiyuan Liu, Zengxiang Zhang, Wancun Zhow, Shuwen Zhang, rendong Li, Changzhen Yan, Shixin Wu, and Xuezheng Shi. 2017. “A Time Series Land Ecosystem Classification Dataset of China in Five-year Increments (1990–2010).” Journal of Global Change Data & Discovery 1 (1): 52–59. https://doi.org/10.3974/geodp.2017.01.08.
- Xu, Xiaotian, Bo Wu, Fang Bao, Ying Gao, Xinle Li, Yanli Cao, Qi Lu, Junliang Gao, Zhiming Xin, and Minghu Liu. 2023. “Different Responses of Growing Season Ecosystem CO2 Fluxes to Rain Addition in a Desert Ecosystem.” Plants 12 (5): 1158. https://doi.org/10.3390/plants12051158.
- Xu, Hengkang, Yingjun Zhang, Xinqing Shao, and Nan Liu. 2022. “Soil Nitrogen and Climate Drive the Positive Effect of Biological Soil Crusts on Soil Organic Carbon Sequestration in Drylands: A Meta-Analysis.” Science of the Total Environment 803:150030. https://doi.org/10.1016/j.scitotenv.2021.150030.
- Yan, Feng, Bo Wu, and Yanjiao Wang. 2015. “Estimating Spatiotemporal Patterns of Aboveground Biomass Using Landsat TM and MODIS Images in the Mu Us Sandy Land, China.” Agricultural and Forest Meteorology 200:119–128. https://doi.org/10.1016/j.agrformet.2014.09.010.
- Yang, Yuanhe, Yue Shi, Wenjuan Sun, Jinfeng Chang, Jianxiao Zhu, Leiyi Chen, Xin Wang, et al. 2022. “Terrestrial Carbon Sinks in China and Around the World and their Contribution to Carbon Neutrality.” Scientia Sinica(Vitae) 52 (4): 534–574. https://doi.org/10.1360/ssv-2021-0362.
- Yao, Xueling, Guojing Yang, Bo Wu, Lina Jiang, and Feng Wang. 2021. “Biomass Estimation Models for Six Shrub Species in Hunshandake Sandy Land in Inner Mongolia, Northern China.” Forests 12 (2): 167. https://doi.org/10.3390/f12020167.
- Ye, Jingyun, Bo Wu, Xiaohong Jia, Bingqiang Fei, Junliang Gao, Long Cheng, Yingjun Pang, Bin Yao, and Deyong Kong. 2022. “Estimation of Aboveground Biomass of Sparse Desert Vegetation Based on Remote Sensing Techniques in Hyper-arid Area.” Arid Land Geography 2 (45): 478–487. https://doi.org/10.12118/j.issn.1000–6060.2021.177.
- Ye, Jingyun, Bo Wu, Minghu Liu, Ying Gao, Junliang Gao, and Yuancai Lei. 2018. “Estimation of Aboveground Biomass of Vegetation in the Desert-oasis Ecotone on the Northeastern Edge of the Ulan Buh Desert.” Acta Ecologica Sinica 38 (4): 1216–1225. https://doi.org/10.5846/stxb201612312719.
- Zan, Guosheng. 2012. “Relationship of Biological Soil Crusts and Land Desertification in Mu Us Desert Lands.” Bulletin of Soil and Water Conservation 32 (4): 27–31. Cnki:Sun:Sttb.0.2012-04-008.
- Zandler, H., A. Brenning, and C. Samimi. 2015. “Quantifying Dwarf Shrub Biomass in an Arid Environment: Comparing Empirical Methods in a High Dimensional Setting.” Remote Sensing Of Environment 158:140–155. https://doi.org/10.1016/j.rse.2014.11.007.
- Zhang, Bingchang, Renhui Li, Peng Xiao, Yangui Su, and Yuanming Zhang. 2016. “Cyanobacterial Composition and Spatial Distribution Based on Pyrosequencing Data in the Gurbantunggut Desert, Northwestern China.” Journal Of Basic Microbiology 56 (3): 308–320. https://doi.org/10.1002/jobm.201500226.
- Zhang, Yuanming, and Xueqin Wang. 2010. “Summary on Formation and Developmental Characteristics of Biological Soil Crusts in Desert Areas.” Acta Ecologica Sinica 30 (16): 4484–4492. Cnki:Sun:Stxb.0.2010-16-027.
- Zhang, Bingchang, Yuanming Zhang, Jiancheng Zhao, Nan Wu, Rongyi Chen, and Jing Zhang. 2009. “Microalgal Species Variation at Different Successional Stages in Biological Soil Crusts of the Gurbantunggut Desert, Northwestern China.” Biology and Fertility of Soils 45 (5): 539–547. https://doi.org/10.1007/s00374-009-0364-0.
- Zhang, BingChang, XiaoBing Zhou, and YuanMing Zhang. 2015. “Responses of Microbial Activities and Soil Physical-chemical Properties to the Successional Process of Biological Soil Crusts in the Gurbantunggut Desert, Xinjiang.” Journal of Arid Land 7 (1): 101–109. https://doi.org/10.1007/s40333-014-0035-3.
- Zheng, YaoMin, ZhenGuo Niu, Gong Peng, Dai YongJiu, and Shang Guanwei. 2013. “Preliminary Estimation of the Organic Carbon Pool in China’s Wetlands.” Chinese Science Buiieth 58 (6): 662–670. https://doi.org/10.1007/s11434-012-5529-9.
- Zulkar, H. A. I. L. I. L., Tingning Zhao, and Qun Ou Jiang. 2021. “Boundary Scope and Change of Arid Desert Area in Northwest China.” Arid Land Geography 44 (6): 1635–1643. https://doi.org/10.12118/j.issn.1000–6060.2021.06.12.