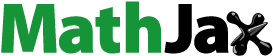
ABSTRACT
A thermal infrared spectrometer is an important sensor onboard the SDGSAT-1 dedicated to serving the 2030 Agenda for Sustainable Development of the United Nations. Field campaigns were conducted from January to December 2022 to evaluate its radiometric calibrations. A radiance-based calibration method was used to derive the spectral radiance of the lake surface around the satellite overpass using a boat-deployed TIR spectrometer or radiometer. The radiometric calibration conditions were quantified by examining the temperature bias between the satellite-based brightness temperature and the predicted at-sensor brightness temperature. Our results indicated that the average brightness temperature bias reached 0.772 K, −1.032 K, and −0.300 K, with RMSEs of 1.00, 1.10, and 0.43 K in bands 1, 2 and 3, respectively. Eighty percent of the field experiments achieved a high calibration accuracy with temperature biases of less than 1 K, and the calibration accuracy of band 3 was better than that of other bands. Atmospheric conditions and surface characterization play important roles in determining the calibration accuracy through surface observations and atmospheric transfer simulations. With the increasing need for SDGSAT-1 products, periodic field experiments are needed to monitor and improve the operational radiometric calibration of satellite instruments to create high-resolution and reasonable scientific datasets.
1. Introduction
The Sustainable Development Goals Science Satellite 1 (SDGSAT-1), developed and operated by the International Research Center of Big Data for Sustainable Development Goals (CBAS), was launched on 5 November 2021, and it is dedicated to providing extensive space observation datasets to serve the 2030 Agenda for Sustainable Development of the United Nations (Guo et al. Citation2021; Guo et al. Citation2023). To support space observation for human activities in sustainable development goals (SDGs) evaluation, three major payloads with high spatial resolution and wide swath, including a thermal infrared spectrometer, glimmer imager, and multispectral imager (), were designed and carried by the SDGSAT-1. Please refer to the SDGSAT-1 specifications available at http://www.cbas.ac.cn/en/resources/sdgsat/ for more detailed information. SDGSAT-1, orbiting the Earth at an altitude of 505 km, operates along a sun-synchronous orbit with a descending node at approximately 11:00am local time and an ascending node at approximately 10:30 pm local time. Coordinated observations were conducted among these three instruments to create a series of scientific data products on the day involving the thermal infrared spectrometer and the multispectral imager and at night using the thermal infrared spectrometer and the glimmer imager, such as the land surface temperature, nighttime lights, vegetation cover, water resources, and land cover types. These derived consistent and well-calibrated satellite measurements will become an important component of big Earth data to monitor Earth surface changes and provide key inputs to climate or ecosystem models to better understand sustainable development in different regions (Guo Citation2019; Guo et al. Citation2023).
Table 1. Spectral and spatial specifications of the SDGSAT-1 satellite.
Absolute radiometric calibration and detailed evaluation of the in-orbit operational calibration conditions of satellite-based sensors have already become the most important steps for the quantitative application of satellite scientific datasets. The thermal infrared spectrometer onboard SDGSAT-1 has significant advantages in higher spatial resolution (30 m), wide swath (300 km) and sensor sensitivity in temperature observation (NEDT<0.08 K@300 K) compared with other popular thermal infrared sensors (Hu et al. Citation2022), which can provide high-quality remote sensing products for environmental monitoring, land surface change analysis, ecosystem function assessment, and human activity detection. Thorough evaluations of the thermal infrared spectrometer onboard SDGSAT-1 are urgently needed to assess the on-orbit radiometric calibration for scientific applications. Ground-based calibration is a popular method for identifying the radiometric calibration situation of thermal infrared instruments, in addition to prelaunch calibrations in the lab and onboard calibrations involving on-orbit calibrators (Dinguirard and Slater Citation1999; Hu et al. Citation2021; Padula and Schott Citation2010). Generally, concurrent experiments across broad homogeneous water surfaces can detect the surface-emitted radiance, atmospheric conditions, and aerosol optical depth during field campaigns, which can then be adopted by atmospheric transfer codes to predict the at-sensor radiance, which can thereafter be compared to the satellite-based at-sensor radiance to evaluate the radiometric calibration conditions of thermal infrared sensors (Skoković, Sobrino, and Jiménez-Muñoz Citation2016; Tonooka et al. Citation2005; Wan et al. Citation2002). The radiance-based method based on observations retrieved from radiometers or spectrometers, such as CE312, can provide the spectral radiance of the lake surface for the prediction of the top of the atmosphere (TOA) radiance in various thermal infrared channels (Hu et al. Citation2021; Zhang et al. Citation2013). Buoy-deployed temperature sensors are another essential source used in the verification or calibration of thermal infrared sensors; however, the measured water temperatures must be converted into skin temperatures (Hook et al. Citation2007). Long-term bulk temperature records retrieved from buoys near satellite overpasses provide suitable potential for comparing skin temperature records to satellite thermal infrared sensor-derived data to monitor degradation or operational conditions (Barsi et al. Citation2014; Padula and Schott Citation2010). The conversion from the bulk temperature measured underwater to the surface skin temperature could introduce uncertainties, and it may be more suitable for the verification of temperature change trends involving TIR sensors (Hook et al. Citation2004; Hook et al. Citation2007). An automatic calibration station with high-resolution radiometers is an alternative way to improve the accuracy of vicarious radiometric calibration in many lakes to monitor the operational situation of thermal infrared instruments.
Currently, there are urgent needs and persistent challenges for big Earth data to serve sustainable development goals (SDGs) by providing quantified information for each indicator related to sustainable development progress and relative capacities among nations (Guo Citation2019). As one of the important sources of big Earth data, SDGSAT-1 can dynamically provide environmental parameters related to land, ocean, atmosphere, and human activities at the global scale (Guo et al. Citation2023). To produce high-resolution thermal infrared data for SDG evaluation, the in-orbit radiometric calibration status of the thermal infrared spectrometer aboard SDGSAT-1 must be thoroughly evaluated for comprehensive and quantitative applications. From the commissioning phase (approximately half a year after satellite launch) to the in-orbit operational phase of SDGSAT-1, we conducted a series of field campaigns at Lake Erhai and Lake Chenghai in Yunnan Province from January to December 2022 to identify the absolute radiometric calibration status of the thermal infrared spectrometer and evaluate possible uncertainties in the brightness temperature products.
2. Study area and field campaigns overview
To perform many calibration experiments during a limited period, two lakes (Lake Erhai and Lake Chenghai) in Yunnan Province were selected to conduct field campaigns based on our investigations of the satellite overpass. Lake Erhai and Lake Chenghai are located on the Yunnan-Guizhou Plateau at a distance of approximately 96 km, and the average elevations of the lake surface reach 1972 and 1460 m, respectively. Lake Erhai has an area of 251 km2 with an average depth of 11 m and a maximum depth of 21 m, whereas the area of Lake Chenghai reaches 75 km2 with an average depth of 25 m and a maximum depth of 37 m. The average width of Lake Erhai is 6.4 km, which is approximately 2 km larger than that of Lake Chenghai. Except for a slight annual temperature difference, both lakes experience a low-latitude plateau monsoon climate with a mean air temperature of approximately 15 °C and an average annual precipitation of 1028 mm (Xu and Li Citation2020). They also experienced a distinct dry season from November to April of the following year and a typical rainy season from May to October, mainly in July and August. Most of the dry season is sunny, windy, and warm, with numerous hours of sunshine. Dry, windy winter with clean air is suitable for conducting absolute radiometric calibration experiments.
We conducted ten ground-based radiometric calibration experiments at Lake Erhai and Lake Chenghai from January 8, 2022, to December 23, 2022 (). Two field instruments (a high-precision portable field 7-channel IR radiometer, CE312,and a D&P instrument model 102 hand portable Fourier transform infrared (FTIR) spectrometer, 102F) were selected to measure the lake surface radiance and examine its surface characterization. The CE312 was equipped on a solar-powered unmanned surface vehicle (USV) to collect the brightness temperature (BT) records of the water surface (). CE312 was situated on the USV at a height of 2 m above the water surface, and its three channels with center wavelengths of 9.1, 10.6 and 11.3 µm were used in the experiments due to their spectrum and bandwidths similar to those of the thermal infrared spectrometer in band 1, band 2 and band 3, respectively. USV cruised along a programed navigation route from 9:00 to 23:00 under automatic navigation mode and lower surface wind speed (), and the measured records by USV and CE312 were stored in a data logger or transferred to the real-time monitoring computer.
Figure 1. The location of the concurrent field experiments for the absolute radiometric calibration of thermal infrared spectrometer onboard SDGSAT-1, including Lake Erhai (a) and Lake Chenghai (b). CE312 and 102F were used in the field experiments to observe lake surface radiance and brightness temperature. The observation locations of 102F are shown in left figure, and the navigation routes of CE312 aboard USV on Mar 2, Dec 5, Dec 8, Dec 10, Dec 13 and Dec 23 were shown at right figures, and the marked black circles are the concurrent location of USV observations during the SDGSAT-1 overpass.

Table 2. Overview of field campaigns at Lake Erhai and Lake Chenghai for SDGSAT-1 radiometric calibration evaluation.
102F was used to collect lake surface-emitted radiation data ranging from 2–16 μm with a spectral resolution (full width at half height or FWHH) of 4 cm−1. This instrument was deployed on a boat, approximately 1 m above the lake surface. As the detector needed to be operated at a stable low temperature, the LN2 Dewar compartments were filled with liquid nitrogen to cool the internal Dewar surface to 77 K prior to each observation. The instrument was calibrated against a reference blackbody prior to each group of measurements during the field experiments. A ground-based microwave radiometer (QFW6000) located at the Dali National Meteorological Station, approximately 2 km from Lake Erhai, was used to collect atmospheric profile information (air temperature, relative humidity, pressure, and water vapor), while the atmospheric profile parameters on March 8 at Lake Chenghai were measured with a radiosonde system near the calibration site using a released balloon. Moreover, diurnal changes in aerosol optical depth (AOD) at the calibration site were detected using a Sun Sky Lunar Multispectral Photometer (CE318), which can provide aerosol and water vapor parameters. The standard atmospheric profiles at Lake Erhai were adopted in QFW6000 to derive real-time atmospheric parameters every 2 min, and the uncertainty of relative humidity and water vapor observation could be less than 12% and 0.58 g/m3, respectively. The weather station continuously monitored and provided surface atmospheric conditions, including the air temperature, relative humidity, pressure, and wind speed. Based on the measurements of surface radiance, aerosol information, and atmospheric conditions during the field campaigns, we predicted the radiance at the sensor aperture via atmospheric propagation simulations at the time of the satellite overpass to evaluate the on-orbit radiometric calibration conditions of the thermal infrared spectrometer aboard SDGSAT-1.
3. Methods
We conducted field experiments and calibration analysis to identify the calibration accuracy of the thermal infrared spectrometer onboard SDGSAT-1 and provide information for analyzing and understanding the possible uncertainties in land surface temperature products. The detailed workflow can be refer to . First, multiple concurrent field experiments were performed over the calibration sites to collect accurate parameters for identifying the lake surface radiance, surface meteorological conditions, and atmospheric profile conditions. Then, we used atmospheric radiative transfer simulation to estimate the radiance and brightness temperature at the sensor aperture to understand the in-orbit radiometric calibration conditions and operational status of the thermal infrared spectrometer.
3.1. Introduction of the thermal infrared spectrometer onboard SDGSAT-1
The entire structure of the thermal infrared spectrometer comprises a scan mirror, one-dimensional pointing mechanism, lens system, blackbody (BB), heat dissipation architecture, and temperature monitor components (). The on-orbit optical system comprises four refraction lenses with a scan mirror located at the outer front end. The system operates at approximately 195 K under the regulation of a temperature control system (Hu et al. Citation2022). As a linear array sensor with continuous observations within a scanning range of approximately ±8.65°, the thermal infrared spectrometer can provide high-resolution thermal infrared images at a spatial resolution of 30 m and swath width of 300 km. The thermal infrared spectrometer contains 2048 detectors along a pixel array comprising four 512×4×3 HgCdTe infrared detector modules. They can be divided into three bands by an integrated filter, ranging in wavelength from 8–12.5 μm (). The datasets were subjected to on-orbit calibration via a variable-temperature BB periodically operating at a constant temperature and variable temperature pattern. The changing temperature pattern adopted six fixed temperature points of 250, 260, 270, 280, 290, and 300 K during the BB warm-up and cool-down operations. These temperature points were then used to obtain the radiometric calibration coefficient for the production of scientific products. The constant-temperature pattern is mainly used to monitor the BB’s on-orbit operating situation. A set of thermistors monitored the temperature variation and stability of the BB and lens systems. To enhance the sensitivity of the thermal infrared spectrometer, deep space observations every two weeks achieved by adjusting the satellite attitude were further used to eliminate the impact of the background signal of the instrument and the dark current in the detectors on each recorded Earth view signal. Thermal infrared sensors are gradually approaching stable operation conditions according to approximately half a year of on-orbit operation and four operating mode examinations conducted via on-orbit testing.
Figure 3. The optical system structure of thermal infrared spectrometer (b) onboard SDGSAT-1 satellite (a). The thermal infrared spectrometer is a typical linear array sensor, which can receive the signals from the view of scan mirror for Earth surface, onboard blackbody and deep space.

Figure 4. The arrangement of linear array detector of the thermal infrared spectrometer, and it included four detector modules on the focal plane (M1, M2, M3 and M4). Pixel size in each module is 30 µm, and detector modules are spliced on a substrate with the overlap of 25 detector elements between every two adjacent modules.

3.2. Radiance-based calibration method
Surface radiance is usually measured using thermal infrared radiometers deployed above the lake surface, and the radiance-based method can be used to calculate the at-sensor radiance propagating from the surface to the satellite aperture. The at-sensor thermal infrared radiance comprises three components: the thermal radiance emitted from the water surface and attenuated by the atmosphere, upward atmospheric thermal radiance, and radiance reflected by the water surface attenuated by the atmosphere (Tonooka et al. Citation2005; Zhang et al. Citation2013).
Owing to the relatively consistent spectral emissivity of a water body, the spectral radiance at the thermal infrared sensor aperture can be expressed as (Skoković, Sobrino, and Jiménez-Muñoz Citation2016; Tonooka et al. Citation2005):
(1)
(1) Where λ is the wavelength,
is the atmospheric transmittance,
is the Planck function for blackbody spectral radiance,
is the path radiance, and
is the atmospheric downwelling radiance.
The at-sensor radiance was convolved with spectral response functions () to obtain the equivalent radiance (Req) of a specific sensor channel, which can be expressed as
(2)
(2)
3.3. Lake surface radiance measurement
Field experiments were conducted to measure lake surface radiance using CE312 and 102F (). Here, CE312 deployed on the USV can continuously record the variation of lake surface radiance at 10-second intervals along a programed navigation route. According to the change in weather conditions (mainly wind speed), the navigation route was modified to maintain its observation stability and safety during field experiments, such as the navigation route on December 5, December 8, and December 23 (). To eliminate possible uncertainties from a single observation, we selected observation records within 20 min around the satellite overpass to calculate the averaged lake surface radiance. This information is then used to calculate the surface brightness temperature and predict the at-sensor radiance.
Approximately 20 groups of records collected from the 102F spectrometer around the SDGSAT-1 overpass were used to obtain the average radiance of the lake surface. A pair of observations encompassing a cold BB (5 °C) and a warm BB (40 °C) must be acquired before each data collection operation, which can generate a calibration function with a certain slope and offset at each wavelength. Then, the sample values of subsequent observations over the lake surface can be converted into sample radiance (W/m2·sr·μm) using the calibration coefficient retrieved from these relative BB views. We could also derive the water emissivity by adding a parameter for the downward calibrated radiation, which could supply an emissivity of 0.03 by observing the gold diffuse plate. Due to the bands of SDGSAT-1/TIS covering 8–12.5 μm, we mainly selected 102F observation records ranging from 8–14 μm to examine the radiance of the water surface of Lake Erhai and Lake Chenghai (). The temperature/emissivity separation method was used to examine the surface radiance:
(3)
(3) where λ is the wavelength, B(λ,T) is the radiance of the black body at temperature T, τ(λ) is the transmittance,
is the downward radiance, and
is the upward radiance. The uncertainty of 102F observations under stable conditions could be less than 0.5 K (Gao et al. Citation2021). Due to the high spectral resolution of thermal infrared instruments (4 cm−1), 102F records can be directly convoluted with the spectral response function of each TIS band of SDGSAT-1 to derive the equivalent radiance. This may reduce the influence of the spectral matching process on the accuracy of the radiometric calibration (Hu et al. Citation2021).
3.4. Brightness temperature derivation using the calibration coefficient
L1A and L4A level products collected from the CBAS SDGSAT-1 Open Data System (http://www.sdgsat.ac.cn) were used to derive the at-sensor radiance and perform concurrent ground-based calibration experiments around the satellite overpass. The L1A images mainly provided raw digital values captured by the thermal infrared detector. Each L1A image comprises a pixel matrix with 10000×1987 pixels arranged in sequence as rows 1–500, 501–975, 976–1475, and 1476–1987 for the detector modules M1, M2, M3, and M4, respectively. The onboard calibrator of the thermal infrared spectrometer mainly contains a deep-space view and changing-temperature BB observations. The background signals from the deep-space view were removed from the digital number (DN) values to create the corrected DN value, and the spectral radiance was calculated at each temperature-changing point according to the relative spectral response and Planck equation. Then, the linear regression for DN values and relative spectral radiance at each temperature point can be used to create the radiometric calibration coefficient for thermal infrared channels (Hu et al. Citation2022).
Here, the digital numbers (DNs) of thermal infrared images of SDGSAT-1 L1A products can be converted into at-sensor radiance using calibration coefficients created by onboard calibration (), and the calibration equation for L1A images is as follows:
(4)
(4) where L is the at-sensor radiance (W/m2·sr·μm), DN is the digital number value of the L1A images from the thermal infrared spectrometer of SDGSAT-1, and BG is the calibration coefficient of the background retrieved from a deep space view. K and B are the gain and bias of the calibration coefficient, respectively.
Table 3. The calibration coefficient for the L1A product of the thermal infrared spectrometer onboard SDGSAT-1.
Then, the at-sensor radiance can be converted into brightness temperature according to the inverse Planck equation:
(5)
(5) where T is the at-sensor brightness temperature (K), h is the Planck constant (6.626×10−34 J·s), c is the speed of light (2.9979×108 m/s), k is the Boltzmann constant (1.3806×10−23 J/K), λ is the center wavelength of each band, and L is the at-sensor radiance. Here, the equivalent center wavelengths of bands 1, 2, and 3 of the thermal infrared spectrometer of SDGSAT-1 are 9.35, 10.73, and 11.72 μm, respectively.
According to the updated information about metadata of SDGSAT-1 in July 2022 (http://124.16.184.48:6008/home), the calibration coefficients from multiple models of the thermal infrared spectrometer were unified to create a single calibration coefficient for each channel (). We use the updated calibration coefficients to convert DN values of the L4A images into at-sensor radiance, and the calibration equation for L4A products is as follows:
(6)
(6) Then, the brightness temperature can be derived from the at-sensor radiance, L, using the inverse Planck equation.
Table 4. The calibration coefficient for the L4A product of the thermal infrared spectrometer onboard SDGSAT-1.
3.5. Spectral matching
shows that there are mismatches in the relative spectral response (RSR) of the thermal infrared channels between the thermal infrared spectrometer and CE312, and calibration errors may be introduced by the RSR mismatch in the derived surface temperatures for the same observed target. Therefore, spectral matching must be conducted to eliminate the influence of these disagreements on radiometric calibration (Yan et al. Citation2021). A previous investigation suggested that the spectral matching method for RSR disagreements could be used to efficiently eliminate the uncertainties used in radiometric calibration evaluation (Hu et al. Citation2021). Therefore, a series of numerical experiments were conducted using the MODTRAN simulation to derive the coefficient of spectral matching between the thermal infrared spectrometer and CE312. Numerous simulation combinations were adopted to generate their relative TOA spectral radiances, including nine different targets (cloud cover, desert, dry grass, field, forest, fresh snow, maple, ocean, and wet grass), two satellite view angles (0° and 10°), six built-in atmospheric profiles of MODTRAN (tropical, midlatitude summer/winter, sub-Arctic summer/winter, U.S. Standard), and nine boundary temperatures (278, 283, 288, 293, 298, 303, 208, 212, and 320 K).
Figure 6. The difference of relative spectral response (RSR) between field instrument (CE312) and satellite thermal infrared sensor.

These top-of-atmosphere radiances were convolved with the spectral response functions of the selected thermal infrared channel of the thermal infrared spectrometer and CE312 to derive the unified channel equivalent radiance according to Equations (7) and (8):
(7)
(7)
(8)
(8) where RCE and RTIS are the unified equivalent spectral radiances for CE312 and the thermal infrared spectrometer, Lmod,λ is the top-of-atmosphere spectral radiance simulated by MODTRAN, and fCE,λ and fTIS,λ are the spectral response functions of the selected thermal infrared bands. Finally, the spectral matching factors for CE312 and the thermal infrared spectrometer were derived from 972 pairs of unified channel equivalent radiances using linear regression analysis. The equivalent center wavelength of band1, band2, band3 of the thermal infrared spectrometer of SDGSAT-1 are 9.35μm, 10.73μm, 11.72μm, while the center wavelength of band4, band3 and band1 of CE312 are 9.38μm, 10.6μm, 11.3μm, respectively. shows the derived coefficients of spectral matching between SDGSAT-1 and CE312, and the predicted at-sensor radiance of CE312 can be converted to a reasonable radiance value to evaluate the absolute radiometric calibration status of SDGSAT-1/TIS.
3.6. At-sensor radiance prediction by the atmospheric transfer model
The radiance-based method was used to quantify the influence of atmospheric conditions on at-sensor radiance. During the propagation process from the lake surface to the satellite aperture, atmospheric absorption and scattering effects can be estimated regarding thermal radiation via atmospheric transfer model simulations. Atmospheric profile records were collected using a QFW6000 instrument around the satellite overpass at Lake Erhai. The QFW6000 can detect atmospheric parameters at altitudes ranging from the land surface to 10 km every 2 min. A radiosonde system was used at Lake Chenghai to release a balloon for collecting atmospheric parameters at different pressure levels. To obtain atmospheric profiles covering the entire tropospheric layer for atmospheric transfer model simulations, atmospheric parameters at five pressure levels (100, 70, 50, 30, 20, and 10 hPa) were collected from National Centers for Environmental Prediction (NCEP)-Department of Energy (DOE) reanalysis 2 datasets (https://psl.noaa.gov/data/gridded/data.ncep.reanalysis2.html) to provide upper-air meteorological information (). As the key parameter used in atmospheric transfer simulation, the water vapor accuracy of the NCEP reanalysis dataset is less than 0.5 mm with a standard deviation of less than 0.6 mm compared with GPS network observation records from 2001–2004 (Jade and Vijayan Citation2008). A previous investigation suggested that the combined atmospheric profiles from the radiosonde balloon and NCEP atmospheric profiles could provide more reliable results than single atmospheric profile from radiosonde in examining surface temperature variations (Li et al. Citation2013).
Figure 8. Atmospheric temperature and relative humidity profiles measured at calibration site of Lake Erhai and Lake Chenghai during the field campaigns from Jan 2022 to Dec 2022. The parameters of atmospheric profiles in upper layer (>12 km) were collected from NECP reanalysis dataset.

The atmospheric aerosols were characterized using an automatic sun tracking photometer (CE318) at the calibration site, and the predicted optical depth at 550 nm was used to estimate the visibility parameters needed for atmospheric transfer simulations. These parameters were then adopted in the Moderate Resolution Atmospheric Transmission Model (MODTRAN 5.2) to derive the path radiance and spectral transmittance during the propagation process from the lake surface to the satellite aperture. The simulation results were convolved with the spectral response functions of the thermal infrared spectrometer to obtain the band-averaged values of the atmospheric parameters and further predict the equivalent at-sensor radiance.
4. Results and discussion
4.1. Brightness temperature around the calibration site derived from the images of the thermal infrared spectrometer onboard SDGSAT-1
According to the detector locations arranged in the thermal infrared spectrometer, calibration sites were identified in the L1A and L4A images to select the appropriate parameters to convert the radiance into brightness temperature, including the calibration coefficient and center wavelength of each band ( and ). Each relative spectral response (RSR) record can be used to generate the equivalent center wavelength to calculate the brightness temperature. We investigated the brightness temperature variation during the day and night around the calibration site of Lake Erhai during field campaigns to examine the temperature stability of the lake surface (). A 6-km transect at Lake Erhai along 25.8° north was used to identify brightness temperature changes from the center to the shore of the lake. We found less brightness temperature variation at night along the transect, with average brightness temperatures of 274.54±0.16 K and 278.19±0.21 K on February 27 and December 10, respectively, while the average brightness temperatures during the day were 276.79±0.19 K and 276.84±0.13 K on March 2 and December 23, respectively. Generally, the brightness temperature at the lake center was less than that near the lake shore by 0.1 K at Lake Erhai. Our investigation at Lake Chenghai also suggested that it has a relatively stable lake temperature, with a temperature around its center of 285.12±0.08 K and a temperature near the shore of 285.42±0.07. Therefore, the lake temperatures around the calibration site achieved satisfactory homogeneity compared to those at traditional thermal infrared calibration sites in Lake Titicaca (<0.5 K) and Lake Qinghai (<1 K) and Lake Qinghai (<1 K) (Wan et al. Citation2002; Zhang et al. Citation2013).
Figure 9. Brightness temperature derived from SDGSAT-1/TIS band1 around calibration site of Lake Erhai on day (Mar 2 and Dec 23) and night (Feb 27 and Dec 10), respectively. A transect, marked by the dotted line, across the lake was used to analyze temperature change from the center to the shore of the lake.

4.2. BT bias evaluation of the thermal infrared spectrometer
Ten concurrent field experiments were conducted from January to December 2022 to evaluate the on-orbit radiometric calibration status of the thermal infrared spectrometer aboard SDGSAT-1, including nine experiments in Lake Erhai and one experiment in Lake Chenghai (). Convolution calculations of the observed surface radiance, upward spectral transmittance, atmospheric path radiance, and RSR function of the thermal infrared spectrometer were used to derive the at-sensor radiance in each experiment. Then, the at-sensor radiance was converted into brightness temperature according to the inverse Planck equation, which was used for comparing with the brightness temperature derived from satellite images to examine the BT bias. shows the daytime and nighttime BT biases calculated using the predicted and satellite-derived brightness temperatures at the satellite aperture during field experiments, and the results suggested that the averaged bias temperatures for bands 1, 2, and 3 were 0.772, −1.032, and −0.300 K, with RMSEs of 1.00, 1.10, and 0.43 K, respectively. Approximately 80% of the BT bias records satisfy the requirements of in-flight calibration accuracy (≤1 K) of the thermal infrared spectrometer, and the overall calibration accuracy at band 3 is better than that at bands 1 and 2. We further examined the temporal change in BT bias and found that the averaged BT bias in March 2022 reached 0.622 K, −0.987 K, and −0.338 K with RSME values of 0.717, 1.059, and 0.429 K, respectively, while the averaged BT bias in December reached 1.212 K, −0.908 K, −0.318 K with RSME values of 1.157, 0.849, and 0.453 K, respectively. The BT bias results suggested that the thermal infrared spectrometer may be approaching stable in-orbit operational conditions, except for band 1, with its averaged BT bias in December greater than that in March by 0.59 K. The thermal infrared sensor may be approaching stability with decreased uncertainties induced by the change in sensor operational conditions, and this phenomenon could also be found for other satellite-based thermal infrared bands, such as the approximately half-year period for TIR bands of the Moderate Resolution Imaging Spectrometer (MODIS) to achieve relatively stable in-orbit conditions (Xiong and Barnes Citation2006). Daytime radiometric calibration during our field campaigns was slightly better than that at nighttime, with a BT bias lower than night BT bias by 0.861, 0.688, and 0.032 K at bands 1, 2, and 3, respectively. This phenomenon may be influenced by the disturbance of wind speed on surface radiance observations because of the relatively strong prevailing wind at night caused by the lake-land thermal contrast and the pressure difference between the western land, mountains, and lake surface (). The wind speed at the lake surface gradually increased to 3.5 m/s from 13:00 to 23:00, and a relatively stronger wind speed appeared around the overpass time of SDGSAT-1 (approximately 22:30). In contrast, the daytime overpass time of SDGSAT-1 (approximately 11:00) usually has a relatively static lake surface, which is more suitable for surface radiance observations (). Our investigation also suggested that the thermal infrared spectrometer onboard SDGSAT-1 attained a similar calibration accuracy to that of other satellite-based TIR instruments, such as values of approximately 0.44 K for MODIS in Lake Titicaca, 0.61 K for the FengYun-4A (FY4A)/Advanced Geosynchronous Radiation Imager (AGRI) in Lake Qinghai, and 1.67 K for the Landsat 8/Thermal Infrared Sensor (TIRS) in Lake Tahoe and Salton Sea (Barsi et al. Citation2014; Hu et al. Citation2021; Skoković, Sobrino, and Jiménez-Muñoz Citation2016; Zhang et al. Citation2013). We also noted that there are some fluctuations in the BT bias change during field campaigns. In the future, the radiometric calibration evaluations and reasonable satellite status monitoring also require more in-orbit tests and field experiments, including investigations to quantify the uncertainties related to surface temperature measurements, detector noise, and variations in atmospheric profiles.
Figure 10. The temperature difference between the predicted at-sensor BT and satellite-derived at-senor BT (BT bias) derived from nighttime field campaigns (a) and daytime field campaigns (b). BT bias is calculated by using satellite-derived at-sensor BT minus predicted at-sensor BT. Field experiments using 102F and CE312 are simultaneously conducted on Mar 2, and their results are marked using 2-Mar(1) and 2-Mar(2).

4.3. Uncertainties analysis
Due to the possible limitations related to field measurements, reference instrument accuracy, and atmospheric transfer simulation during vicarious radiometric calibration, the uncertainties need to be identified to understand the radiometric conditions of the thermal infrared spectrometer onboard SDGSAT-1. The uncertainties of the absolute radiometric calibration mainly comprise four parts: atmospheric characterization, surface characterization, atmospheric transfer simulation, and spectral matching (Hu et al. Citation2021; Thome et al. Citation2008). Here, we mainly evaluated uncertainties from the measurement of surface temperature and the calculation of atmospheric radiative transfer using the root mean square (RMS) (Slater et al. Citation1996). Each part of the uncertainty results is combined to calculate the overall uncertainty of the thermal infrared channels by using the root sum of squares of the RMSs from different factors (Tong et al. Citation2009).
(9)
(9)
The uncertainties were analyzed as follows:
The uncertainty of surface temperature measurements by CE312 in Lake Erhai is less than 0.3 K (Sicard et al. Citation1999; Tong et al. Citation2009), while the uncertainty of measurements of surface temperature by 102F is less than 0.5 K (Gao et al. Citation2021). The calibration analysis indicated that the uncertainty induced by surface temperature measurements by CE312 reached 0.225, 0.224, and 0.181 K for bands 1, 2 and 3, respectively, while the uncertainties induced by surface temperature measurements by 102F reached 0.375, 0.374, and 0.302 K for bands 1, 2 and 3, respectively.
Longwave radiation absorption by water vapor is the key factor influencing the accuracy of radiometric calibration for thermal infrared channels. Therefore, multiple groups of atmospheric transfer simulations with different relative humidity levels were used to identify uncertainties. The comparison of atmospheric profiles of NCEP reanalysis data and the atmospheric profiles measured by QFW6000 or radiosonde balloon for each experiment was used in the uncertainty analysis, and the results indicated that relative humidity change would cause uncertainty with RMSEs of 0.455, 0.646, and 0.248 K for bands 1, 2, and 3, respectively.
Disagreements in the relative spectral response (RSR) were found for the thermal channels between SDGSAT-1 and CE312 (), which may cause errors in the derived surface temperatures for the same observed target. To identify the uncertainties of radiometric calibration results by spectral mismatch of RSR, we analyzed the difference between the original calibration BT and the BT after spectral matching to examine the uncertainties. The results indicated that the spectral mismatch may cause uncertainty by 0.791, 2.184, and 3.412 K for bands 1, 2, and 3, respectively.
Generally, the total estimated uncertainty of the thermal infrared spectrometer onboard SDGSAT-1, excluding spectral matching, is 0.631, 0.779, and 0.431 K for bands 1, 2, and 3, respectively. The average uncertainty of the calibration experiment for the three channels reached 0.614 K, while it increased to 2.256 K if spectral matching was included in the uncertainty analysis. This phenomenon also suggests that spectral matching is the key step in conducting a reasonable analysis and maintaining high calibration accuracy in vicarious calibration experiments.
To achieve better calibration accuracy, we must select a stable and relatively homogeneous lake surface around the calibration site. Clear and dry atmospheric conditions are also important for conducting a reasonable calibration analysis and reducing possible uncertainties. Some concurrent experiments may be influenced by unstable weather conditions due to the experiment date being close to the rainy day, and the water vapor content would cause uncertainties in the calibration analysis, such as field experiments on January 9. Water vapor is the most important source for the absorption of thermal infrared radiation, such as the approximately 1.0 K at-sensor brightness temperature bias for FY3D induced by the 1.0 g/m3 variation in water vapor at Lake Qinghai (Yan et al. Citation2021), while atmospheric transfer simulations suggested that this value increased to 2.6 K for FY2B with doubled relative humidity (Tong et al. Citation2010). The air temperature and relative humidity of atmospheric profiles above 10 km were adopted from the NCEP reanalysis multilevel data, which accounted for less than 5% of the total water vapor content and consequently slightly affected longwave radiation absorption. Local weather conditions in the lake may also influence field experiments, such as wind speed, water waves, and cloud cover (Hook et al. Citation2004; Slater et al. Citation1996; Wan et al. Citation1999), and they could cause uncertainty in the radiometric calibration evaluation of thermal infrared sensors in ground-based experiments. For example, a large clear sky area at the calibration site is needed to reduce the influence of the cloud edge on the brightness temperature derivation. A previous investigation found that cloud edges usually result in a notable decrease in brightness temperature when the selected pixels are close to clouds (Hu et al. Citation2021). The efficient elimination of atmospheric influences is essential and critical for evaluating the on-orbit status of thermal infrared sensors, and less atmospheric impacts have been observed during field campaigns conducted over large and homogeneous lakes at higher altitudes in dry and clean atmospheres (Schott et al. Citation2012; Tonooka et al. Citation2005; Wan et al. Citation2002; Zhang et al. Citation2013).
4.4. The linearity evaluation of calibration coefficients
Onboard calibration coefficients of the thermal infrared spectrometer of SDGSAT-1 were created by six fixed temperature points during the BB warm-up operation, which were used to derive at-sensor radiance from satellite images. Our vicarious calibration experiment in Lake Erhai can also create vicarious calibration coefficients according to two-point radiance information calculated from deep space observations and land surface observations. Here, the equivalent temperature records of deep space observations, approximately 4 K, were usually used in the calibration coefficient derivation (Hu et al. Citation2022). Therefore, vicarious calibration coefficients theoretically cover a wider temperature range, which can be used to validate or verify the operational calibration coefficient by comparing their derived brightness temperature records (Schneider and Mauser Citation1996). Therefore, two-point vicarious calibration coefficients for the thermal infrared spectrometer were created in each concurrent calibration experiment according to the deep-space view and the predicted at-sensor radiance. Then, the linear regression analysis may explain the linearity of the calibrated brightness temperature derived from the onboard calibration coefficient and vicarious calibration coefficient, particularly for lower-temperature surface objects. Similar gains were found with the values of the vicarious coefficient at bands 1, 2, and 3 by 0.00438, 0.00491, and 0.00730, respectively (). shows the at-sensor radiance change using the vicarious calibration coefficient and onboard calibration coefficient when DN values range from 200–4000, which indicates that the linearity of the calibration coefficient can be better maintained at band 3 than at bands 1 and 2. We further selected a region (20 km×20 km) near the calibration site of Lake Erhai and Lake Chenghai to investigate the at-sensor radiance difference, and a linear relationship was found for the at-sensor radiance variation under different land surface types. Generally, the range of vicarious calibration coefficients is larger than that of onboard calibration coefficients because of the possible uncertainties from concurrent field experiments (Thome et al. Citation1998; Xiong and Barnes Citation2006). The average difference in at-sensor radiances derived from onboard calibration coefficients and vicarious calibration coefficients reached 0.34±0.09 W/m2·sr·μm, −0.19±0.15 W/m2·sr·μm, and −0.26±0.08 W/m2·sr·μm for bands 1, 2 and 3, respectively. The BT differences (RMSE) derived from the vicarious calibration coefficient and onboard calibration coefficient in the selected region indicated that the averaged BT differences were less than 1 K, with RMSEs of 0.885, 0.638, and 0.300 K for bands 1, 2, and 3, respectively.
Figure 12. The at-sensor radiance (OC-Radiance: radiance derived by onboard calibration coefficient, VC-Radiance: radiance derived from vicarious calibration coefficient, W/m2·sr·μm) comparison calculated by vicarious calibration coefficients and onboard calibration coefficients when DN values ranged from 200-4000.

4.5. Emissivity variation in lake water
Water emissivity is usually affected by the water quality conditions and lake water constituents, which can result in uncertainties in the observed surface temperature (Liu et al. Citation1987; Schneider and Mauser Citation1996). A simulation investigation suggested that a 0.005 change in surface emissivity could cause a 0.4 K deviation in land surface temperature derivation in midlatitude regions (Sobrino, Coll, and Caselles Citation1991). A negative relationship was found between water emissivity and the concentration of suspended particulates (Liu et al. Citation1987; Wei et al. Citation2017). A large, clean, and homogeneous water surface is considered an ideal calibration site for the absolute radiometric calibration of thermal infrared sensors (Wan et al. Citation2002). To identify the influence of surface emissivity, we investigated the variation in the spectral radiance and water emissivity covering the thermal infrared regions (8–14 μm) in Lake Erhai on January 9 and Lake Chenghai on March 8 using 102F data (). In contrast to Lake Chenghai, Lake Erhai exhibited better water quality because its emissivity change profile was more similar to that of standard water (Carbonneau and Piegay Citation2012). Local government records also suggested that the water quality of Lake Erhai and Lake Chenghai reached the 2nd and 4th categories according to the Environmental Quality Standards for Surface Water released by the Chinese government in 2002. In fact, the main difference in water emissivity between Lake Erhai and Lake Chenghai occurred over the spectral range of 13–14 μm, and a slight difference could be found from our investigation of the emissivity profiles with respect to the wavelength specifications of the thermal infrared spectrometer, such as 0.980, 0.989, and 0.968 for bands 1, 2, and 3, respectively, at Lake Erhai and 0.974, 0.989, and 0.962 for bands 1, 2, and 3 at Lake Chenghai. This phenomenon indicates that water emissivity did not significantly affect the observed surface temperature. Surface observations by 102F attained a relatively consistent accuracy for the brightness temperature due to its internal calibration coefficient obtained by BB observations.
5. Conclusion
This study investigated and evaluated the absolute radiometric calibration status of a thermal infrared spectrometer onboard SDGSAT-1 using concurrent field experiments at Lake Erhai and Lake Chenghai from January 2022 to December 2022. A TIR spectrometer (102F) and TIR radiometer (CE312) were used to derive the brightness temperature of the lake surface and further evaluate the radiometric calibration condition of the thermal infrared spectrometer by comparing the satellite-based at-sensor brightness temperature with the predicted at-sensor brightness temperature. Generally, the calibration status of thermal infrared sensor aboard SDGSAT-1 is approaching stable from January to December, except for a slightly larger BT bias in band 1 in December. The BT bias of band3 was lower than that of bands 1 and 2 during the field campaigns, which indicates that it is more suitable for creating land surface temperature products. The overall estimated uncertainties of our thermal infrared calibration analysis are 0.631, 0.779, and 0.431 K for bands 1, 2, and 3, respectively, and the spectral matching processes play important roles in maintaining reasonable calibration accuracy. More field experiments or intercalibration studies are needed to monitor the operational status of satellite instruments and further improve the accuracy of the absolute radiometric calibration of the thermal infrared spectrometer. Our study also suggests that thermal infrared images of SDGSAT-1 are qualified for producing high-level scientific products, and they will be greatly helpful in facilitating a global approach to the realization of the SDGs and contributing to the attainment and measurement progress toward the attainment of the SDGs.
Acknowledgments
The authors thank Prof. Huazhong Ren of Peking University, Prof. Yong Zhang of the National Satellite Meteorological Center, and Miss Haiyan Rao of the Chenghai Administrative Bureau for their help during the field campaigns. The authors appreciate the help of the USV group from the Key Laboratory of Middle Atmosphere and Global Environment Observation, Institute of Atmospheric Physics, Chinese Academy of Sciences. The authors also thank Dr. Hailiang Gao, Dr. Wenyong Ma, Dr. Hanlin Ye, Mr. Hongwei Zhang, Miss Min Zhu, Mr. Hao Zhang, and Mr. Chenglong Zhang for their help with the data collection and valuable comments on the manuscript.
Disclosure statement
No potential conflict of interest was reported by the author(s).
Data availability statement
The data supporting the findings of this study are available from the corresponding author upon reasonable request.
Additional information
Funding
References
- Barsi, J. A., J. R. Schott, S. J. Hook, N. G. Raqueno, B. L. Markham, and R. G. Radocinski. 2014. “Landsat-8 Thermal Infrared Sensor (TIRS) Vicarious Radiometric Calibration.” Remote Sensing 6 (11): 11607–11626. https://doi.org/10.3390/rs61111607.
- Carbonneau, P., and H. Piegay. 2012. Fluvial Remote Sensing for Science and Management. Hoboken: Wiley-Blackwell. ISBN:9780470714270. DOI:10.1002/9781119940791.
- Dinguirard, M., and P. N. Slater. 1999. “Calibration of Space-Multispectral Imaging Sensors: A Review.” Remote Sensing of Environment 68 (3): 194–205. https://doi.org/10.1016/S0034-4257(98)00111-4.
- Gao, L. Y. Z., L. Q. Cao, Y. F. Zhong, and Z. Y. Jia. 2021. “Field-Based High-Quality Emissivity Spectra Measurement Using a Fourier Transform Thermal Infrared Hyperspectral Imager.” Remote Sensing 13 (21), https://doi.org/10.3390/rs13214453.
- Guo, H. D. 2019. “From Digital Earth to Big Earth Data: Accelerating Scientific Discovery and Supporting Global Sustainable Development.” International Journal of Digital Earth 12 (1): 1–1. https://doi.org/10.1080/17538947.2018.1559481.
- Guo, H. D., C. Y. Dou, H. Y. Chen, J. B. Liu, B. H. Fu, X. M. Li, Z. M. Zou, and D. Liang. 2023. “SDGSAT-1: The World's First Scientific Satellite for Sustainable Development Goals.” Science Bulletin 68 (1): 34–38. https://doi.org/10.1016/j.scib.2022.12.014.
- Guo, H. D., D. Liang, F. Chen, and Z. Shirazi. 2021. “Innovative Approaches to The Sustainable Development Goals Using Big Earth Data.” Big Earth Data 5 (3): 263–276. https://doi.org/10.1080/20964471.2021.1939989.
- Hook, S. J., G. Chander, J. A. Barsi, R. E. Alley, A. Abtahi, F. D. Palluconi, B. L. Markham, R. C. Richards, S. G. Schladow, and D. L. Helder. 2004. “In-Flight Validation and Recovery of Water Surface Temperature with Landsat-5 Thermal Infrared Data Using an Automated High-Altitude Lake Validation Site at Lake Tahoe.” IEEE Transactions on Geoscience and Remote Sensing 42 (12): 2767–2776. https://doi.org/10.1109/Tgrs.2004.839092.
- Hook, S. J., R. G. Vaughan, H. Tonooka, and S. G. Schladow. 2007. “Absolute Radiometric In-Flight Validation of Mid Infrared and Thermal Infrared Data from ASTER and MODIS on The Terra Spacecraft Using the Lake Tahoe, CA/NV, USA, Automated Validation Site.” IEEE Transactions on Geoscience and Remote Sensing 45 (6): 1798–1807. https://doi.org/10.1109/Tgrs.2007.894564.
- Hu, Y. H., Y. Zhang, L. Yan, X. M. Li, C. Y. Dou, G. S. Jia, Y. D. Si, and L. J. Zhang. 2021. “Evaluation of the Radiometric Calibration of FY4A-AGRI Thermal Infrared Data Using Lake Qinghai.” IEEE Transactions on Geoscience and Remote Sensing 59 (9): 8040–8050. https://doi.org/10.1109/Tgrs.2020.3037828.
- Hu, Z. Y., M. Zhu, Q. Y. Wang, X. F. Su, and F. S. Chen. 2022. “SDGSAT-1 TIS Prelaunch Radiometric Calibration and Performance.” Remote Sensing 14 (18): 4543. doi:ARTN454310.3390rs14184543.
- Jade, S., and M. S. M. Vijayan. 2008. “GPS-Based Atmospheric Precipitable Water Vapor Estimation Using Meteorological Parameters Interpolated from NCEP Global Reanalysis Data.” Journal of Geophysical Research-Atmospheres 113 (D3). doi:ArtnD03106/10.10292007jd008758.
- Li, H., Q. H. Liu, Y. M. Du, J. X. Jiang, and H. S. Wang. 2013. “Evaluation of the NCEP and MODIS Atmospheric Products for Single Channel Land Surface Temperature Retrieval With Ground Measurements: A Case Study of HJ-1B IRS Data.” IEEE Journal of Selected Topics in Applied Earth Observations and Remote Sensing 6 (3): 1399–1408. https://doi.org/10.1109/Jstars.2013.2255118.
- Liu, W. Y., R. T. Field, R. G. Gantt, and V. Klemas. 1987. “Measurement of the Surface Emissivity of Turbid Waters.” Remote Sensing of Environment 21: 97–109. https://doi.org/10.1016/0034-4257(87)90009-5.
- Padula, F. P., and J. R. Schott. 2010. “Historic Calibration of the Thermal Infrared Band of Landsat-5 TM.” Photogrammetric Engineering and Remote Sensing 76 (11): 1225–1238. https://doi.org/10.14358/Pers.76.11.1225.
- Schneider, K., and W. Mauser. 1996. “Processing and Accuracy of Landsat Thematic Mapper Data for Lake Surface Temperature Measurement.” International Journal of Remote Sensing 17 (11): 2027–2041. https://doi.org/10.1080/01431169608948757.
- Schott, J. R., S. J. Hook, J. A. Barsi, B. L. Markham, J. Miller, F. P. Padula, and N. G. Raqueno. 2012. “Thermal Infrared Radiometric Calibration of The Entire Landsat 4, 5, and 7 Archive (1982–2010).” Remote Sensing of Environment 122: 41–49. https://doi.org/10.1016/j.rse.2011.07.022.
- Sicard, M., P. R. Spyak, G. Brogniez, M. Legrand, N. K. Abuhassan, C. Pietras, and J. Buis. 1999. “Thermal-Infrared Field Radiometer for Vicarious Cross-Calibration: Characterization and Comparisons with Other Field Instruments.” Optical Engineering 38 (2): 345–356. https://doi.org/10.1117/1.602094.
- Skoković, D., J. A. Sobrino, and J. C. Jiménez-Muñoz. 2016. “Vicarious Calibration of the Landsat 7 Thermal Infrared Band and LST Algorithm Validation of the ETM Plus Instrument Using Three Global Atmospheric Profiles.” IEEE Transactions on Geoscience and Remote Sensing 55 (3): 1804–1811. https://doi.org/10.1109/Tgrs.2016.2633810.
- Slater, P. N., S. F. Biggar, K. J. Thome, D. I. Gellman, and P. R. Spyak. 1996. “Vicarious Radiometric Calibrations of EOS Sensors.” Journal of Atmospheric and Oceanic Technology 13 (2): 349–359. https://doi.org/10.1175/1520-0426(1996)013<0349:vrcoes>2.0.co;2.
- Sobrino, J. A., C. Coll, and V. Caselles. 1991. “Atmospheric Correction for Land Surface Temperature Using NOAA-11 AVHRR Channels 4 and 5.” Remote Sensing of Environment 38 (1): 19–34. https://doi.org/10.1016/0034-4257(91)90069-i.
- Thome, K., K. Arai, S. Hook, H. Kieffer, H. Lang, T. Matsunaga, A. Ono, F. Palluconi, H. Sakuma, and P. Slater. 1998. “ASTER Preflight and Inflight Calibration and the Validation of Level 2 Products.” IEEE Transactions on Geoscience and Remote Sensing 36 (4): 1161–1172. https://doi.org/10.1109/36.701023.
- Thome, K. J., K. Arai, S. Tsuchida, and S. F. Biggar. 2008. “Vicarious Calibration of ASTER via the Reflectance-Based Approach.” IEEE Transactions on Geoscience and Remote Sensing 46 (10): 3285–3295. https://doi.org/10.1109/tgrs.2008.928730.
- Tong, J. J., S. J. Dery, Y. Chen, and B. Hu. 2010. “An Alternative Method for In-Flight Absolute Radiometric Calibration of Thermal Infrared Channels of Chinese Geostationary Meteorological Satellites.” International Journal of Remote Sensing 31 (3): 791–803. https://doi.org/10.1080/01431160902897841.
- Tong, J. J., S. J. Dery, B. Hu, Y. Chen, C. J. Yang, and Z. G. Rong. 2009. “Onboard Real-Time Absolute Radiometric Calibration for Thermal Infrared Channels of Chinese Geostationary Meteorological Satellites.” Journal of Atmospheric and Oceanic Technology 26 (2): 281–289. https://doi.org/10.1175/2008jtecha1139.1.
- Tonooka, H., F. D. Palluconi, S. J. Hook, and T. Matsunaga. 2005. “Vicarious Calibration of ASTER Thermal Infrared Bands.” IEEE Transactions on Geoscience and Remote Sensing 43 (12): 2733–2746. https://doi.org/10.1109/Tgrs.2005.857885.
- Wan, Z. M., Y. L. Zhang, Z. L. Li, R. B. Wang, V. V. Salomonson, A. Yves, R. Bosseno, and J. F. Hanocq. 2002. “Preliminary Estimate of Calibration of the Moderate Resolution Imaging Spectroradiometer Thermal Infrared Data Using Lake Titicaca.” Remote Sensing of Environment 80 (3): 497–515. https://doi.org/10.1016/s0034-4257(01)00327-3.
- Wan, Z. M., Y. L. Zhang, X. L. Ma, M. D. King, J. S. Myers, and X. W. Li. 1999. “Vicarious Calibration of the Moderate-Resolution Imaging Spectroradiometer Airborne Simulator Thermal-Infrared Channels.” Applied Optics 38 (30): 6294–6306. https://doi.org/10.1364/ao.38.006294.
- Wei, J. A., D. F. Wang, F. Gong, X. Q. He, and Y. Bai. 2017. “The Influence of Increasing Water Turbidity on Sea Surface Emissivity.” IEEE Transactions on Geoscience and Remote Sensing 55 (6): 3501–3515. https://doi.org/10.1109/Tgrs.2017.2675623.
- Xiong, X. X., and W. Barnes. 2006. “An Overview of MODIS Radiometric Calibration and Characterization.” Advances in Atmospheric Sciences 23 (1): 69–79. https://doi.org/10.1007/s00376-006-0008-3.
- Xu, A. L., and J. Li. 2020. “An Overview of the Integrated Meteorological Observations in Complex Terrain Region at Dali National Climate Observatory, China.” Atmosphere 11 (3): 279. https://doi.org/10.3390/atmos11030279.
- Yan, L., Y. H. Hu, Y. Zhang, X. M. Li, C. Y. Dou, J. Li, Y. D. Si, and L. J. Zhang. 2021. “Radiometric Calibration Evaluation for FY3D MERSI-II Thermal Infrared Channels at Lake Qinghai.” Remote Sensing 13 (3): 466. doi:ARTN466/10.3390rs13030466.
- Zhang, Y., Z. J. Zheng, X. Q. Hu, Z. G. Rong, and L. J. Zhang. 2013. “Lake Qinghai: Chinese Site for Radiometric Calibration of Satellite Infrared Remote Sensors.” Remote Sensing Letters 4 (4): 315–324. https://doi.org/10.1080/2150704x.2012.728298.