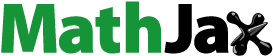
ABSTRACT
Ice, Cloud, and land Elevation Satellite-2 (ICESat-2) provides effective photon-counting light detection and ranging (LiDAR) data for estimating forest height across extensive geographical areas. Although prior studies have illustrated canopy conditions during leaf-on and leaf-off phases may influence ICESat-2 derived forest heights, a comprehensive understanding of this effect remains incomplete. This study seeks to comprehensively assess how varying canopy conditions (leaf-on/leaf-off) affect ICESat-2 forest height retrieval and modelling. First, the accuracies of ICESat-2 terrain and canopy heights under leaf-on and leaf-off conditions were validated. Second, random forest algorithm was utilized to model forest height by integrating ICESat-2, Sentinel-2, and other ancillary datasets. Finally, we evaluated the influence of leaf-on and leaf-off conditions on forest height retrieval and modelling. Results reveal higher consistency between ICESat-2 and airborne LiDAR-derived terrain heights compared to the agreement between two canopy height datasets. Accuracies of ICESat-2 terrain and canopy heights are higher under leaf-off conditions in contrast to leaf-on conditions. Notably, the accuracies of ICESat-2 terrain and canopy heights under various conditions are closely linked to canopy cover. Furthermore, the accuracy of forest height modelling can be enhanced by combining ICESat-2 data collected during both leaf-on and leaf-off seasons with further eliminating low-quality samples.
1. Introduction
The growing concern over ecological and environmental challenges resulting from global climate change has put a spotlight on the importance of forest ecosystems in mitigating these impacts. Comprehensive and large-scale observations of forest ecosystems have become indispensable for understanding and addressing these challenges (Silva et al. Citation2021). Accurately quantifying vertical structural parameters within forests, such as forest height, is crucial not only for reducing uncertainties in carbon stock estimations but also for advancing our knowledge of terrestrial ecosystems and the global carbon cycle (Fayad et al. Citation2021; Narine et al. Citation2019b).
Traditional field-measurement methods can accurately estimate forest height but are very time-consuming and labor-intensive, making them impractical for assessing extensive forested areas. Passive optical and active microwave remote sensing techniques struggle to acquire precise vertical structure information within forest canopies due to their limited canopy penetration capabilities (Moudrý et al. Citation2023). In contrast, Light Detection and Ranging (LiDAR), especially space-borne LiDAR, is the preferred choice for large-scale forest structural parameter monitoring due to its unique ability to penetrate through the forest canopy to reach the ground and its wide coverage area (Davison, Donoghue, and Galiatsatos Citation2020; Gwenzi et al. Citation2016; Lim et al. Citation2003).
The launch of the Ice, Cloud, and Land Elevation Satellite (ICESat) marked the initiation of space-borne LiDAR research for forest height measurement. Many previous studies have utilized ICESat data to achieve forest height extraction at global or regional scales (Simard et al. Citation2011). However, due to the low frequency of laser pulses emitted by ICESat, most of the resulting forest height maps have a resolution of either 500 meters or 1 kilometer. Currently, the launch of Global Ecosystem Dynamics Investigation (GEDI) and ICESat-2 missions have provided unprecedented opportunities for global fine-scale vegetation height modeling and carbon storage monitoring (Narine, Popescu, and Malambo Citation2019a; Potapov et al. Citation2021; Silva et al. Citation2021). GEDI is positioned on the International Space Station and is limited to observing forest vertical structures between latitudes 51.6 degrees north and south. In contrast to GEDI, ICESat-2’s Advanced Topographic Laser Altimeter System (ATLAS) employs micro-pulse, multi-beam photon-counting LiDAR technology with a high pulse repetition frequency of 10 kHz (Zhu et al. Citation2018; Zhu et al. Citation2020a). This advanced technology enables high-resolution 3D along-track sampling, covering nearly global extents (Neuenschwander and Magruder Citation2019a). While ICESat-2's primary mission revolves around monitoring glacier elevation and mass changes, its land, water, and vegetation elevation product (ATL08) have found extensive applications within the realm of forest research. These applications encompass forest height retrieval (Liu, Cheng, and Chen Citation2021; Nie et al. Citation2018; Pang et al. Citation2022), forest height change monitoring (Mulverhill et al. Citation2022; Sun, Qi, and Huang Citation2020), and above-ground biomass estimation (Narine et al. Citation2019c). Furthermore, combining ICESat-2 with other remote sensing data allows for regional or global scale forest height mapping. For instance, Jiang et al. (Citation2021) employed the Random Forest (RF) algorithm, synergizing Sentinel-2 and ICESat-2 data, to achieve continuous mapping of forest height. Their results underscored the exceptional performance of ICESat-2 data in modelling forest height. Liu, Cheng, and Chen (Citation2022) introduced a novel neural network-guided interpolation method that incorporated GEDI, ICESat-2, and Sentinel-2 datasets to create a 30 m forest height map for China.
While previous research has underscored the potential of ICESat-2 data for forest height retrieval and modelling, its accuracy is subject to various influencing factors, including sensor characteristics (strong/weak beams), canopy structure (canopy cover and height), terrain (terrain slope), and external environmental conditions (solar angle and atmosphere scattering). Several studies have thoroughly investigated the impact of these factors on the precision of ICESat-2 data retrieval in forest height analysis. For example, Zhu et al. (Citation2020b) conducted an assessment of ICESat-2 data under different scenarios (strong/weak beams, daytime, and nighttime) for ground elevation and forest height estimation. Their findings indicated that all ICESat-2 data are highly effective for ground elevation extraction, whereas weak beams collected during the daytime are inadequate for forest height estimation. Neuenschwander et al. (Citation2020) performed a comprehensive validation of terrain and canopy heights derived from ICESat-2 data using airborne LiDAR data, revealing a significant correlation between the accuracy of ICESat-2 canopy heights and beam intensity. Furthermore, prior studies have evaluated the impact of canopy cover on forest height retrieval (Malambo and Popescu Citation2021; Moudrý et al. Citation2022; Neuenschwander and Pitts Citation2019b), suggesting that the accuracy of ICESat-2 canopy heights varies with canopy cover, with the lowest errors observed under moderate canopy cover conditions.
In summary, ICESat-2 data is a potent tool for forest height retrieval and modeling, but prior research has mainly focused on sensor characteristics, canopy structure, and terrain slope's impact on accuracy. There has been limited exploration of the influence of seasonal variations (leaf-on/leaf-off) during ICESat-2 data collection. This gap is critical in deciduous forests with varying photon penetration characteristics. To improve accuracy in combining ICESat-2 with other remote sensing data for forest height mapping, we must accumulate a broader set of high-precision ICESat-2 samples. Therefore, optimizing the use of ICESat-2 data under both leaf-on and leaf-off conditions is vital, necessitating an evaluation of their impact on forest height extraction for selecting more high-precision samples.
This study aims to address this knowledge gap by assessing the effect of canopy conditions (leaf-on/leaf-off) during ICESat-2 data collection on forest height retrieval and modelling. Three specific objectives have been outlined: (1) retrieve terrain and canopy heights from ICESat-2 data under both leaf-on and leaf-off conditions; (2) integrate ICESat-2 and Sentinel-2 data to map forest height using the RF algorithm; (3) evaluate the impact of leaf-on and leaf-off canopy conditions on forest height retrieval and modelling. Through these objectives, our research endeavors to offer valuable insights into the influence of seasonal canopy conditions on forest height estimation, ultimately enhancing the accuracy and applicability of ICESat-2 data for forest monitoring and ecological research.
2. Materials
2.1. Study area
Six sites in the northeastern United States (latitude 35.69°N to 46.23°N, longitude 89.59°W to 71.29°W, see ) were selected as our study area. This choice was driven by the availability of airborne LiDAR data and the presence of extensive deciduous forests. The study area exhibits an altitude range of 160 m to 1978m, with an annual average temperature varying between 4.3 and 13.1°C and annual average precipitation ranging from 797 mm to 1375 mm. Land cover types in this region include forests (deciduous, evergreen, and mixed), shrubs, grasslands, and crops. In this study, we concentrate on deciduous forests, and leverage the seasonal characteristics of deciduous forests to investigate the influence of leaf-on (May-September) and leaf-off (November-March) canopy conditions on ICESat-2 canopy height retrieval and modelling.
Figure 1. Location and distribution of the study sites. The background displays the land cover map from Liu Liangyun's team (Zhang et al. Citation2021).

2.2. ICESat-2 data
NASA launched the ICESat-2 mission as a successor to the ICESat mission (Wang et al. Citation2011). ICESat-2 encompasses 21 standard data products. Several data processing steps, such as noise removal and photon classification, are executed to derive the ATL08 product from ATL03 data. The ATL08 product furnishes terrain and canopy heights at an along-track interval of 100 m. The terrain elevation metrics in ATL08 encompass minimum, maximum, and average terrain heights. Additionally, ATL08 provides various canopy height metrics, including minimum, maximum, average canopy heights, and percentile canopy heights. enumerates the key parameters extracted from the ATL08 data product. For this study, we acquired ATL08 data from November 2018 to September 2021 (excluding the months of April and October each year) through the National Snow and Ice Data Center (NSIDC) website (https://nsidc.org/data/icesat-2).
Table 1. Key parameters extracted from ATL08 data product.
2.3. Sentinel-2 data
Sentinel-2, a component of the European Space Agency's Copernicus program, comprises Sentinel-2A and Sentinel-2B, high-resolution multispectral imaging satellites extensively employed for surface parameter retrieval and disaster monitoring (Li et al. Citation2020). In this study, we accessed Sentinel-2 data via the Google Earth Engine (GEE) platform to extract canopy cover, spectral variables, and vegetation indices for forest height modeling (Drusch et al. Citation2012).
Calculating canopy cover from Sentinel-2 data involved two main steps: (1) We selected only the Sentinel-2 data with less than 10% cloud cover during the vegetation growing season from April to September 2019. (2) Canopy cover was computed using Sentinel-2 data by calculating the Normalized Difference Vegetation Index (NDVI), and then applying the dimidiate pixel model after excluding water bodies. Similar preprocessing steps were applied to Sentinel-2 data. Specifically, we selected cloud-free Sentinel-2 images from April to September 2019 using a de-clouding function, calculated the median value of Sentinel-2 images for each month, and merged them into a single image. These merged images and canopy cover were resampled to 30 m resolution.
2.4. Airborne LiDAR data
For validation purposes, this study utilized airborne LiDAR data obtained through the National Ecological Observatory Network (NEON) project (https://data.neonscience.org/). The NEON Airborne Observation Platform (AOP) is equipped with various remote sensing instruments, including a spectrometer, LiDAR, and a camera, designed to collect valuable data for measuring vegetation biophysical parameters (Kampe et al. Citation2010). NEON processed the airborne LiDAR data to generate several products, including Digital Terrain Models (DTM) and Canopy Height Models (CHM) at a spatial resolution of 1 m. These DTM and CHM products are stored in GeoTIFF format utilize the NAVD88 (Geoid12A) vertical datum (Scholl et al. Citation2020; Wang et al. Citation2022).
2.5. Ancillary data
2.5.1. Global land-cover data product
We employed the GLC_FCS30-1985_2020 global land cover classification product from Prof. Liu Liangyun's team (Zhang et al. Citation2021) to identify the location and extent of deciduous forests within the study area. This product, generated using time-series Landsat images on the Google Earth Engine (GEE) computing platform, encompasses 29 land cover types and is accessible for free at the Earth Big Data Science Project data sharing website (https://data.casearth.cn/).
2.5.2. SRTM DEM
The Shuttle Radar Topography Mission (SRTM) provides high-resolution digital elevation models (DEMs) extensively utilized across various fields, including geology, geomorphology, natural disaster assessment, and vegetation survey (Moudrý et al. Citation2018; Yang, Meng, and Zhang Citation2011). We adopted the 30 m resolution SRTM DEM to compute terrain slope and aspect, crucial inputs for forest height modeling.
2.5.3. Worldclim 2 data product
Climatic factors were considered in relation to forest height, and as such, variables like temperature and precipitation were included in this study (Simard et al. Citation2011). The dataset for average annual temperature and precipitation was sourced from the WorldClim 2 data product, made available by WorldClim (https://www.worldclim.org/data/worldclim21.html).
3. Methodology
The technical flowchart for this study is illustrated in , comprising three main steps: (1) ICESat-2 data processing for terrain and canopy height retrievals, (2) forest height modeling, and (3) accuracy assessment and analysis.
3.1. ICESat-2 data processing for terrain and canopy heights retrievals
Accurate retrieval of terrain and canopy heights relies heavily on effective ICESat-2 data selection and processing. This section outlines the various data processing steps employed in this study.
Key parameter extraction: Key parameters extracted from ICESat-2 ATL08 data (see ) are essential for effective data selection, and terrain and canopy height retrievals. These parameters include ‘longitude’ and ‘latitude,’ representing geographic positions, ‘h_canopy_uncertainty’ and ‘h_te_uncertainty’ as quality flags, and ‘h_te_best_fit’ and ‘h_canopy’ used for terrain and canopy height retrieval (Neuenschwander and Pitts Citation2019b).
Effective data selection: To ensure the quality of ICESat-2 data, we initiated the process by discarding data affected by cloud cover, as clouds can compromise data validity and reliability. Specifically, ICESat-2 ATL08 data were considered cloud-free when the absolute difference between their ground elevation and the reference elevation from a global DEM product was less than 50 m. Additionally, ATL08 data with significant uncertainties (‘h_canopy_uncertainty’ = 3.40282E + 38 or ‘h_te_uncertainty’ = 3.40282E + 38) were classified as low-quality data and excluded from subsequent analysis (Yu et al. Citation2022). Furthermore, to minimize the impact of solar background noise on forest height retrieval, we only considered ATL08 data collected at night within deciduous forest areas where airborne LiDAR data was available. Through this process, we identified 2656 valid ICESat-2 samples (870 for the leaf-on season and 1786 for the leaf-off season).
Terrain and canopy heights retrievals: In this study, we employed two key parameters from ATL08 data as proxies for ICESat-2 terrain and canopy heights. Specifically, ‘h_canopy’ from ATL08 represented canopy height, and ‘h_te_best_fit’ represented terrain height.
Vertical datum conversion: Ensuring that all datasets share the same vertical datum is essential for consistent terrain height analysis. While the ATL08 product employs the WGS84 ellipsoidal vertical datum for terrain height, the NEON DTM product uses the North American Vertical Datum NAVD88 (GEOID12A) as its geodetic elevation reference datum. To achieve consistency, we utilized the online version of VDatum software (https://vdatum.noaa.gov/vdatumweb/) (Nwankwo et al. Citation2021) to convert the vertical datum of the ATL08 data. All exported ATL08 terrain height data were replaced with elevation values that matched the LiDAR data. Vertical datum conversion was unnecessary for canopy heights as they represent relative heights.
3.2. Forest height modelling
This section details the construction of a forest height model by integrating ICESat-2 forest height samples, Sentinel-2 images, SRTM DEM, and other multi-source data for forest height modeling under both leaf-on and leaf-off canopy conditions. The process involves three main steps:
3.2.1. Predictor variable extraction
We extracted a series of predictor variables () following the methods of Zhu et al. (Citation2020c) and Simard et al. (Citation2019). These included spectral indices such as the Difference Vegetation Index (DVI), Enhanced Vegetation Index (EVI), and Inverted Red Edge Chlorophyll Index (IRECI) derived from pre-processed Sentinel-2 data. Additionally, topographic variables (elevation, terrain slope, and aspect) were obtained from SRTM DEM data. Climatic variables such as average annual temperature and precipitation were extracted from the WorldClim 2 data product.
Table 2. Predictor variables employed for forest height model construction.
3.2.2. Forest height modeling and modelling
The forest height model was developed using the Random Forest algorithm (Nandy, Srinet, and Padalia Citation2021). The RF algorithm is a nonparametric statistical estimation technique known for analyzing complex nonlinear relationships between dependent and predictor variables. Four key steps were involved in building the forest height models using the RF algorithm:
Setting essential input parameters, including the number of variables attempted at each split node (mtry) and the number of trees (ntree). In this study, ntree was set to 100.
Ranking feature variables by importance and selecting only those with high-ranking importance to establish the forest height extrapolation models.
Employing the RF algorithm to construct the forest height models based on ICESat-2 forest height samples, Sentinel-2 data, and other ancillary data.
Applying the RF models to generate spatially continuous forest height maps for the study area.
3.3. Accuracy assessment and analysis
3.3.1. Accuracy validation
The DTM and CHM served as reference data for validating the accuracies of terrain and forest heights, respectively. Terrain height accuracy was assessed by considering the elevation at the midpoint of the 100-m transect (100 m × 12 m) as a reference value. Forest height accuracy was evaluated by calculating the relative 98th percentile height of the CHM within the 100-m transect. Furthermore, we validated the accuracy of the 30 m resolution forest height map using the relative 98th percentile height of the CHM within a 30 m × 30 m area. To ensure temporal consistency with the ATL08 data, most of the DTM and CHM products were collected in 2019 and downloaded from NEON. Additionally, the coordinate system of DTM and CHM was converted to the UTM projection with datum WGS84 to maintain compatibility with that of ICESat-2.
The assessment was conducted using statistical indicators, including bias, coefficient of determination (R2), and root mean square error (RMSE).
(1)
(1)
(2)
(2)
(3)
(3) where x represents the ICESat-2 terrain or canopy height, y denotes the reference value derived from the NEON LiDAR-derived DTM or CHM, and
is the average reference value.
3.3.2. Effect of leaf-on and -off canopy conditions
The study evaluated differences in terrain and canopy height accuracies between leaf-on and leaf-off canopy conditions and analyzed the impact of canopy cover on ICESat-2 terrain and canopy height retrievals under varying canopy conditions. Furthermore, the effect of leaf-on and leaf-off canopy conditions on forest height modeling was explored. Different forest height models were established using ICESat-2 data collected during the leaf-off season, the leaf-on season, and both seasons.
4. Results
4.1. ICESat-2 terrain and canopy heights
4.1.1. Terrain heights
The validation results for terrain heights from ICESat-2 ( and ) demonstrate a high level of compatibility with airborne LiDAR-derived terrain heights, irrespective of canopy conditions. The R2 values are close to 1, indicating strong agreement. The RMSE values for ICESat-2 terrain heights are 4.57 and 1.36 m for leaf-on and leaf-off canopy conditions, respectively. Notably, terrain heights from ICESat-2 exhibit higher accuracy under leaf-off canopy conditions compared to leaf-on conditions. illustrates that under leaf-on conditions, ICESat-2 terrain heights tend to be significantly overestimated (bias = 1.73 m), while under leaf-off conditions, they closely match the reference terrain heights derived from NEON airborne LiDAR data.
Figure 3. The frequency histogram of the differences between the ICESat-2 terrain heights and NEON airborne LiDAR terrain heights under (a) leaf-on and (b) leaf-off canopy conditions.

Figure 4. Scatterplots of ICESat-2 terrain heights versus airborne LiDAR-derived terrain heights under (a) leaf-on and (b) leaf-off canopy conditions.

Additionally, reveals a correlation between the accuracy of ICESat-2 terrain heights and canopy cover. Specifically, the RMSE value for ICESat-2 terrain heights is lower under leaf-off conditions for all canopy cover ranges, indicating higher accuracy under leaf-off conditions regardless of canopy cover. Notably, the RMSE values for terrain heights only slightly differ between leaf-on and leaf-off canopy conditions when canopy cover is below 0.75. However, a substantial disparity in RMSE values emerges when canopy cover exceeds 0.75, particularly with a significant increase in RMSE values under leaf-on conditions.
4.1.2. Canopy heights
Validation results of ICESat-2 canopy heights using airborne LiDAR-derived canopy heights under leaf-on and leaf-off canopy conditions are depicted in and . demonstrates R2 values of 0.47 and 0.56 and corresponding RMSE values of 5.62 and 4.51 m for ICESat-2 canopy heights under leaf-on and leaf-off conditions, respectively. Notably, a negative bias exists between canopy height estimates and reference values in both leaf-on and leaf-off conditions, indicating that ICESat-2 consistently underestimates canopy heights. Furthermore, larger bias values are observed under leaf-off canopy conditions compared to leaf-on conditions, suggesting more substantial underestimation of canopy heights under leaf-off conditions. These results also highlight that there is lower consistency between ICESat-2 and airborne LiDAR-derived canopy heights compared to terrain heights.
Figure 6. The frequency histogram of the differences between ICESat-2 canopy heights and airborne LiDAR-derived canopy heights under (a) leaf-on and (b) leaf-off canopy conditions.

Figure 7. Scatterplots of ICESat-2 canopy heights versus NEON airborne LiDAR canopy heights under (a) leaf-on and (b) leaf-off canopy conditions.

wThe impact of canopy cover on canopy height agreement between ICESat-2 and airborne LiDAR is explored in . When canopy cover is less than 0.75, the RMSE curves of canopy height under leaf-on and leaf-off conditions largely overlap. However, a significant difference in RMSE values emerges when canopy cover exceeds 0.75. Furthermore, the results in indicate that RMSE values are substantial at low canopy cover, regardless of leaf-on or leaf-off canopy conditions, indicating that ICESat-2 canopy heights struggle to represent actual forest heights.
4.2. Forest height modelling
The importance ranking of 30 feature variables was calculated, only the top 20 important feature variables were selected based on their significance in the RF model, primarily include spectral bands and vegetation indices from Sentinel-2, topographic variables, and climatic variables. Notably, regardless of canopy conditions, MTCI and B5 consistently rank as the most important feature variables.
RF models are established to map forest heights under six different conditions, as depicted in . Forest heights with significant values are predominantly distributed in the northeast and southwest regions, while regions in the central area exhibit relatively smaller values. These forest height maps are subsequently validated using reference forest heights derived from airborne LiDAR data, as shown in . Notably, forest height maps exhibit the highest accuracy when ICESat-2 data from both leaf-on and leaf-off conditions are combined, excluding the 0–0.25 canopy cover interval. In other words, for optimal forest height modeling, it is advisable to utilize ICESat-2 data from both leaf-on and leaf-off seasons while excluding ICESat-2 data with low canopy cover.
Figure 9. Forest height maps under (a) leaf-on conditions; (b) leaf-off conditions; (c) leaf-on and -off conditions; (d) leaf-on conditions excluding 0-0.25 canopy cover interval; (e) leaf-off conditions excluding 0-0.25 canopy cover interval; (f) leaf-on and -off conditions excluding 0-0.25 canopy cover interval.

Figure 10. Scatterplots of forest height maps versus airborne LiDAR canopy heights under (a) leaf-on conditions; (b) leaf-off conditions; (c) leaf-on and -off conditions; (d) leaf-on conditions excluding 0-0.25 canopy cover interval; (e) leaf-off conditions excluding 0-0.25 canopy cover interval; (f) leaf-on and -off conditions excluding 0-0.25 canopy cover interval.

5. Discussion
The results affirm that ICESat-2 data can accurately retrieve terrain and canopy heights. This is consistent with previous studies. Neuenschwander et al. (Citation2020) and Zhu et al. (Citation2020b) have also documented the strong capability of ICESat-2 in terrain elevation extraction and forest height estimation. Moreover, a higher level of agreement is observed between ICESat-2 terrain heights and reference values compared to canopy heights, aligning with the study of Malambo and Popescu (Citation2021), which employed airborne LiDAR data to confirm that terrain heights exhibit greater agreement with ICESat-2 data compared to canopy heights.
In this study, ICESat-2 terrain heights are more accurately extracted under leaf-off conditions than under leaf-on conditions, in line with similar findings in the literature. The seasonal variability in canopy conditions is known to significantly impact the accuracy of ATL08 terrain heights, with errors varying up to 0.8 m (Tian and Shan Citation2021). White et al. (Citation2021) also confirmed that leaf-off Single Photon LiDAR (SPL) data tend to be more accurate than leaf-on SPL data. This discrepancy is attributed to the greater number of returns from the ground surface under leaf-off conditions, resulting in improved terrain height accuracy. Conversely, some ground photons are more likely to be reflected by the canopy rather than the ground surface under leaf-on conditions, leading to terrain height overestimation.
Additionally, the study reveals that ICESat-2 terrain heights are overestimated under leaf-on canopy conditions. This overestimation may be the fact that more photons come from canopy rather than ground reflection leaf-on conditions, and the ATL08 algorithm easily misclassifies near-ground canopy photons as ground photons (Neuenschwander and Pitts Citation2019b). Similarly, the results show that ICESat-2 consistently underestimates canopy heights under both leaf-on and leaf-off canopy conditions, with more pronounced underestimation occurring under leaf-off conditions. This underestimation may be attributed to photons having a lower probability of detecting the canopy top compared to the canopy interior (Liu, Cheng, and Chen Citation2021), particularly under leaf-off conditions when photons are more likely to be reflected from the canopy's interior.
Furthermore, the accuracy of ICESat-2 terrain and canopy heights is closely linked to canopy cover. The study indicates that ICESat-2 terrain height accuracy is lower under leaf-on conditions when canopy cover is high. This observation is consistent with prior research (Neuenschwander and Magruder Citation2016), which highlighted the importance of a sufficient number of photons reflected from the ground surface for accurate terrain height retrieval. The study also found that extremely dense canopy cover results in low terrain height accuracy due to the limited number of ground-reaching photons. In contrast, terrain height accuracy under leaf-off conditions remains consistently high regardless of canopy cover as a sufficient number of photons can be reflected from the ground surface. Additionally, canopy height accuracy is substantially lower under leaf-on and leaf-off conditions when canopy cover is low, mainly because signal photons are more likely to be reflected from the ground surface than the canopy, adversely affecting canopy height estimation.
Previous research has explored the impact of leaf-on and leaf-off canopy conditions on forest parameter estimation using airborne LiDAR data (Davison, Donoghue, and Galiatsatos Citation2020; Hernández-Stefanoni et al. Citation2015; Hill and Broughton Citation2009). However, the prior studies have not systematically investigated the effect of these canopy conditions on forest height modeling using ICESat-2 data. This study pioneers the examination of the influence of ICESat-2 data collected under different canopy conditions (leaf-on or leaf-off) on forest height modeling. The results underscore the advantage of integrating ICESat-2 data from both leaf-on and leaf-off conditions to enhance forest height modeling accuracy. Removing low-quality ICESat-2 forest height samples is also shown to be beneficial for improving modeling accuracy.
6. Conclusion
This study provides a comprehensive evaluation of the impact of changing canopy conditions (leaf-on/leaf-off) during ICESat-2 data collection on terrain and canopy height retrievals and forest height modeling. Key findings include:
ICESat-2 demonstrates the capability to retrieve terrain and canopy heights, with stronger agreement observed for terrain heights compared to canopy heights.
Terrain heights are estimated with higher accuracy during leaf-off canopy conditions, as they allow for a greater acquisition of ground photons. Conversely, leaf-on conditions often lead to an overestimation of terrain heights. Canopy heights consistently exhibit underestimation under both leaf-on and leaf-off conditions, with a more significant underestimation observed during leaf-off conditions.
The accuracy of ICESat-2 terrain and canopy heights is closely related to canopy cover. High canopy cover adversely affects terrain height accuracy under leaf-on conditions, while leaf-off conditions exhibit higher accuracy regardless of canopy cover. Canopy height accuracy is significantly reduced under both canopy conditions when canopy cover is low.
Combining ICESat-2 data from leaf-on and leaf-off conditions, excluding data with low canopy cover, enhances forest height modeling accuracy. Additionally, the removal of low-quality ICESat-2 forest height samples contributes to improved modeling accuracy.
Acknowledgements
The authors would like to thank NASA for providing ICESat-2 data and NEON for providing airborne LiDAR data. We also thank the anonymous reviewers for their constructive comments on the manuscript.
Disclosure statement
No potential conflict of interest was reported by the author(s).
Data availability statement
The data that support the findings of this study are available from the corresponding author upon reasonable request.
Additional information
Funding
References
- Davison, S., D. N. Donoghue, and N. Galiatsatos. 2020. “The Effect of Leaf-on and Leaf-off Forest Canopy Conditions on LiDAR Derived Estimations of Forest Structural Diversity.” International Journal of Applied Earth Observation and Geoinformation 92:102160. https://doi.org/10.1016/j.jag.2020.102160.
- Drusch, M., U. Del Bello, S. Carlier, O. Colin, V. Fernandez, F. Gascon, B. Hoersch, et al. 2012. “Sentinel-2: ESA's Optical High-Resolution Mission for GMES Operational Services.” Remote Sensing of Environment 120:25–36. https://doi.org/10.1016/j.rse.2011.11.026.
- Fayad, I., N. N. Baghdadi, C. A. Alvares, J. L. Stape, J. S. Bailly, H. F. Scolforo, M. Zribi, and G. Le Maire. 2021. “Assessment of GEDI's LiDAR Data for the Estimation of Canopy Heights and Wood Volume of Eucalyptus Plantations in Brazil.” IEEE Journal of Selected Topics in Applied Earth Observations and Remote Sensing 14:7095–7110. https://doi.org/10.1109/JSTARS.2021.3092836.
- Gwenzi, D., M. A. Lefsky, V. P. Suchdeo, and D. J. Harding. 2016. “Prospects of the ICESat-2 Laser Altimetry Mission for Savanna Ecosystem Structural Studies Based on Airborne Simulation Data.” ISPRS Journal of Photogrammetry and Remote Sensing 118:68–82. https://doi.org/10.1016/j.isprsjprs.2016.04.009.
- Hernández-Stefanoni, J. L., K. D. Johnson, B. D. Cook, J. M. Dupuy, R. Birdsey, A. Peduzzi, and F. Tun-Dzul. 2015. “Estimating Species Richness and Biomass of Tropical dry Forests Using LIDAR During Leaf-on and Leaf-off Canopy Conditions.” Applied Vegetation Science 18 (4): 724–732. https://doi.org/10.1111/avsc.12190.
- Hill, R. A., and R. K. Broughton. 2009. “Mapping the Understorey of Deciduous Woodland from Leaf-on and Leaf-off Airborne LiDAR Data: A Case Study in Lowland Britain.” ISPRS Journal of Photogrammetry and Remote Sensing 64 (2): 223–233. https://doi.org/10.1016/j.isprsjprs.2008.12.004.
- Jiang, F., F. Zhao, K. Ma, D. Li, and H. Sun. 2021. “Mapping the Forest Canopy Height in Northern China by Synergizing ICESat-2 with Sentinel-2 Using a Stacking Algorithm.” Remote Sensing 13 (8): 1535. https://doi.org/10.3390/rs13081535.
- Kampe, T. U., B. R. Johnson, M. A. Kuester, and M. Keller. 2010. “NEON: The First Continental-Scale Ecological Observatory with Airborne Remote Sensing of Vegetation Canopy Biochemistry and Structure.” Journal of Applied Remote Sensing 4 (1): 043510. https://doi.org/10.1117/1.3361375.
- Li, W., Z. Niu, R. Shang, Y. Qin, L. Wang, and H. Chen. 2020. “High-resolution Mapping of Forest Canopy Height Using Machine Learning by Coupling ICESat-2 LiDAR with Sentinel-1, Sentinel-2 and Landsat-8 Data.” International Journal of Applied Earth Observation and Geoinformation 92:102163. https://doi.org/10.1016/j.jag.2020.102163.
- Lim, K., P. Treitz, M. Wulder, B. St-Onge, and M. Flood. 2003. “LiDAR Remote Sensing of Forest Structure.” Progress in Physical Geography: Earth and Environment 27 (1): 88–106. https://doi.org/10.1191/0309133303pp360ra.
- Liu, A., X. Cheng, and Z. Chen. 2021. “Performance Evaluation of GEDI and ICESat-2 Laser Altimeter Data for Terrain and Canopy Height Retrievals.” Remote Sensing of Environment 264: 112571. https://doi.org/10.1016/j.rse.2021.112571.
- Malambo, L., and S. C. Popescu. 2021. “Assessing the Agreement of ICESat-2 Terrain and Canopy Height with Airborne Lidar Over US Ecozones.” Remote Sensing of Environment 266:112711. https://doi.org/10.1016/j.rse.2021.112711.
- Moudrý, V., A. F. Cord, L. Gábor, G. V. Laurin, V. Barták, K. Gdulová, M. Malavasi, et al. 2023. “Vegetation Structure Derived from Airborne Laser Scanning to Assess Species Distribution and Habitat Suitability: The way Forward.” Diversity and Distributions 29 (1): 39–50. https://doi.org/10.1111/ddi.13644.
- Moudrý, V., K. Gdulová, L. Gábor, E. Šárovcová, V. Barták, F. Leroy, O. Špatenková, D. Rocchini, and J. Prošek. 2022. “Effects of Environmental Conditions on ICESat-2 Terrain and Canopy Heights Retrievals in Central European Mountains.” Remote Sensing of Environment 279:113112. https://doi.org/10.1016/j.rse.2022.113112.
- Moudrý, V., V. Lecours, K. Gdulová, L. Gábor, L. Moudrá, J. Kropáček, and J. Wild. 2018. “On the Use of Global DEMs in Ecological Modelling and the Accuracy of New Bare-Earth DEMs.” Ecological Modelling 383:3–9. https://doi.org/10.1016/j.ecolmodel.2018.05.006.
- Mulverhill, C., N. C. Coops, T. Hermosilla, J. C. White, and M. A. Wulder. 2022. “Evaluating ICESat-2 for Monitoring, Modeling, and Update of Large Area Forest Canopy Height Products.” Remote Sensing of Environment 271:112919. https://doi.org/10.1016/j.rse.2022.112919.
- Nandy, S., R. Srinet, and H. Padalia. 2021. “Mapping Forest Height and Aboveground Biomass by Integrating ICESat-2, Sentinel-1 and Sentinel-2 Data Using Random Forest Algorithm in Northwest Himalayan Foothills of India.” Geophysical Research Letters 48 (14): e2021GL093799. https://doi.org/10.1029/2021GL093799.
- Narine, L. L., S. C. Popescu, and L. Malambo. 2019a. “Synergy of ICESat-2 and Landsat for Mapping Forest Aboveground Biomass with Deep Learning.” Remote Sensing 11 (12): 1503. https://doi.org/10.3390/rs11121503.
- Narine, L. L., S. Popescu, A. Neuenschwander, T. Zhou, S. Srinivasan, and K. Harbeck. 2019b. “Estimating Aboveground Biomass and Forest Canopy Cover with Simulated ICESat-2 Data.” Remote Sensing of Environment 224:1–11. https://doi.org/10.1016/j.rse.2019.01.037.
- Narine, L. L., S. Popescu, T. Zhou, S. Srinivasan, and K. Harbeck. 2019c. “Mapping Forest Aboveground Biomass with a Simulated ICESat-2 Vegetation Canopy Product and Landsat Data.” Annals of Forest Research 62 (2): 69–86. https://doi.org/10.15287/afr.2018.1163.
- Neuenschwander, A., E. Guenther, J. C. White, L. Duncanson, and P. Montesano. 2020. “Validation of ICESat-2 Terrain and Canopy Heights in Boreal Forests.” Remote Sensing of Environment 251:112110. https://doi.org/10.1016/j.rse.2020.112110.
- Neuenschwander, A. L., and L. A. Magruder. 2016. “The Potential Impact of Vertical Sampling Uncertainty on ICESat-2/ATLAS Terrain and Canopy Height Retrievals for Multiple Ecosystems.” Remote Sensing 8 (12): 1039. https://doi.org/10.3390/rs8121039.
- Neuenschwander, A. L., and L. A. Magruder. 2019a. “Canopy and Terrain Height Retrievals with ICESat-2: A First Look.” Remote Sensing 11 (14): 1721. https://doi.org/10.3390/rs11141721.
- Neuenschwander, A., and K. Pitts. 2019b. “The ATL08 Land and Vegetation Product for the ICESat-2 Mission.” Remote Sensing of Environment 221:247–259. https://doi.org/10.1016/j.rse.2018.11.005.
- Nie, S., C. Wang, X. Xi, S. Luo, G. Li, J. Tian, and H. Wang. 2018. “Estimating the Vegetation Canopy Height Using Micro-Pulse Photon-Counting LiDAR Data.” Optics Express 26 (10): A520–A540. https://doi.org/10.1364/OE.26.00A520.
- Nwankwo, U. C., S. Howden, D. Wells, and B. Connon. 2021. “Validation of VDatum in Southeastern Louisiana and Western Coastal Mississippi.” Marine Geodesy 44 (1): 1–25. https://doi.org/10.1080/01490419.2020.1846644.
- Pang, S., G. Li, X. Jiang, Y. Chen, Y. Lu, and D. Lu. 2022. “Retrieval of Forest Canopy Height in a Mountainous Region with ICESat-2 ATLAS.” Forest Ecosystems 9:100046. https://doi.org/10.1016/j.fecs.2022.100046.
- Potapov, P., X. Y. Li, A. Hernandez-Serna, A. Tyukavina, M. C. Hansen, A. Kommareddy, A. Pickens, et al. 2021. “Mapping Global Forest Canopy Height Through Integration of GEDI and Landsat Data.” Remote Sensing of Environment 253:112165. https://doi.org/10.1016/j.rse.2020.112165.
- Scholl, V. M., M. E. Cattau, M. B. Joseph, and J. K. Balch. 2020. “Integrating National Ecological Observatory Network (NEON) Airborne Remote Sensing and in-Situ Data for Optimal Tree Species Classification.” Remote Sensing 12 (9): 1414. https://doi.org/10.3390/rs12091414.
- Silva, C. A., L. Duncanson, S. Hancock, A. Neuenschwander, N. Thomas, M. Hofton, L. Fatoyinbo, et al. 2021. “Fusing Simulated GEDI, ICESat-2 and NISAR Data for Regional Aboveground Biomass Mapping.” Remote Sensing of Environment 253:112234. https://doi.org/10.1016/j.rse.2020.112234.
- Simard, M., L. Fatoyinbo, C. Smetanka, V. H. Rivera-Monroy, E. Castañeda-Moya, N. Thomas, and T. Van der Stocken. 2019. “Mangrove Canopy Height Globally Related to Precipitation, Temperature and Cyclone Frequency.” Nature Geoscience 12 (1): 40–45. https://doi.org/10.1038/s41561-018-0279-1.
- Simard, M., N. Pinto, J. B. Fisher, and A. Baccini. 2011. “Mapping Forest Canopy Height Globally with Spaceborne Lidar.” Journal of Geophysical Research: Biogeosciences 116:G4. https://doi.org/10.1029/2011JG001708.
- Sun, T., J. Qi, and H. Huang. 2020. “Discovering Forest Height Changes Based on Spaceborne Lidar Data of ICESat-1 in 2005 and ICESat-2 in 2019: A Case Study in the Beijing-Tianjin-Hebei Region of China.” Forest Ecosystems 7 (1): 1–12. https://doi.org/10.1186/s40663-019-0212-0.
- Tian, X., and J. Shan. 2021. “Comprehensive Evaluation of the ICESat-2 ATL08 Terrain Product.” IEEE Transactions on Geoscience and Remote Sensing 59 (10): 8195–8209. https://doi.org/10.1109/TGRS.2021.3051086.
- Wang, X., X. Cheng, P. Gong, H. Huang, Z. Li, and X. Li. 2011. “Earth Science Applications of ICESat/GLAS: A Review.” International Journal of Remote Sensing 32 (23): 8837–8864. https://doi.org/10.1080/01431161.2010.547533.
- Wang, C., A. J. Elmore, I. Numata, M. A. Cochrane, S. Lei, C. R. Hakkenberg, Y. Li, Y. Zhao, and Y. Tian. 2022. “A Framework for Improving Wall-to-Wall Canopy Height Mapping by Integrating GEDI LiDAR.” Remote Sensing 14 (15): 3618. https://doi.org/10.3390/rs14153618.
- White, J. C., M. Woods, T. Krahn, C. Papasodoro, D. Bélanger, C. Onafrychuk, and I. Sinclair. 2021. “Evaluating the Capacity of Single Photon Lidar for Terrain Characterization Under a Range of Forest Conditions.” Remote Sensing of Environment 252:112169. https://doi.org/10.1016/j.rse.2020.112169.
- Yang, L., X. Meng, and X. Zhang. 2011. “SRTM DEM and its Application Advances.” International Journal of Remote Sensing 32 (14): 3875–3896. https://doi.org/10.1080/01431161003786016.
- Yu, J., S. Nie, W. Liu, X. Zhu, D. Lu, W. Wu, and Y. Sun. 2022. “Accuracy Assessment of ICESat-2 Ground Elevation and Canopy Height Estimates in Mangroves.” IEEE Geoscience and Remote Sensing Letters 19:1–5. https://doi.org/10.1109/LGRS.2021.3107440.
- Zhang, X., L. Liu, X. Chen, Y. Gao, S. Xie, and J. Mi. 2021. “GLC_FCS30: Global Land-Cover Product with Fine Classification System at 30 m Using Time-Series Landsat Imagery.” Earth System Science Data 13 (6): 2753–2776. https://doi.org/10.5194/essd-13-2753-2021.
- Zhu, X., S. Nie, C. Wang, and X. Xi. 2020b. “The Performance of ICESat-2's Strong and Weak Beams in Estimating Ground Elevation and Forest Height.” In IGARSS 2020-2020 IEEE International Geoscience and Remote Sensing Symposium. IEEE: 6073–6076. https://doi.org/10.1109/IGARSS39084.2020.9323094.
- Zhu, X., S. Nie, C. Wang, X. Xi, and Z. Hu. 2018. “A Ground Elevation and Vegetation Height Retrieval Algorithm Using Micro-Pulse Photon-Counting Lidar Data.” Remote Sensing 10 (12): 1962. https://doi.org/10.3390/rs10121962.
- Zhu, X., S. Nie, C. Wang, X. Xi, J. Wang, D. Li, and H. Zhou. 2020a. “A noise removal algorithm based on OPTICS for photon-counting LiDAR data.” IEEE Geoscience and Remote Sensing Letters 18 (8): 1471-1475. https://doi.org/10.1109/LGRS.2020.3003191.l.
- Zhu, X., C. Wang, S. Nie, F. Pan, X. Xi, and Z. Hu. 2020c. “Mapping Forest Height Using Photon-Counting LiDAR Data and Landsat 8 OLI Data: A Case Study in Virginia and North Carolina, USA.” Ecological Indicators 114:106287. https://doi.org/10.1016/j.ecolind.2020.106287.