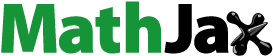
ABSTRACT
This study introduces a comprehensive framework that integrates Multi-objective optimisation with Multiple-Criteria Decision Making (MCDM) techniques to enhance decision-making in sustainable machining processes. Through a systematic literature review, the framework is developed, incorporating sustainability indicators, experimental validation, and multi-objective optimisation algorithms to identify the optimal machining conditions. To address the challenge of subjective judgements and the multiple solutions generated by multi-objective optimisation, MCDM techniques are employed to select the best solution. By considering economic viability, environmental impact reduction, and social commitment, the proposed framework overcomes the limitations of traditional optimisation approaches. It provides an objective and holistic approach to decision-making, promoting sustainability in machining processes. The framework’s effectiveness and applicability are demonstrated through a case study, validating its potential for practical implementation and informed decision-making
1. Introduction
The manufacturing industry plays a significant role in environmental degradation (Rajemi, Mativenga, and Aramcharoen Citation2010), with machines such as milling, lathe, and EDM being major energy consumers, accounting for up to 50% of total energy consumption (Hu et al. Citation2019). To address this issue, improving the sustainability of manufacturing processes has become crucial. Machine parameter optimisation has emerged as a promising approach in recent years, aiming to optimise machining parameters to achieve desired performance while minimising environmental impact (Cai et al. Citation2022). This optimisation technique has shown considerable improvements in machining performance, including reduced tool wear and increased material removal rates, while also reducing energy consumption and carbon emissions (Pawanr, Kant Garg, and Routroy Citation2019).
Machine parameter optimisation is a multi-objective problem, where multiple objectives may conflict with each other, creating trade-offs that need to be considered for determining the best solution. It is important to take into account these trade-offs and consider the optimisation outcomes in a multi-objective context. By employing multi-objective optimisation techniques, a set of optimal solutions can be obtained, each representing different trade-offs between the objectives. The selection of the best solution from this set requires a comprehensive evaluation that considers the inherent trade-offs and takes into account the specific requirements and priorities of the problem at hand. Some of the examples of these tradeoffs are given below:
Surface Finish vs. Material Removal Rate: Achieving a smooth and high-quality surface finish often requires using lower cutting speeds and feeds, which may result in a slower material removal rate. Conversely, maximising material removal rate may lead to rougher surface finishes. Balancing these objectives is crucial to achieve the desired level of surface finish while maintaining productivity.
Tool Life vs. Productivity: Increasing cutting speeds and feeds can enhance productivity by reducing machining time. However, this may also result in increased tool wear and shorter tool life. Optimising machine parameters to extend tool life while maintaining acceptable productivity levels requires finding an optimal balance between these conflicting objectives.
Energy Consumption vs. Machining Time: Minimising energy consumption is an essential aspect of sustainability. However, reducing cutting speeds and feeds to lower energy consumption may lead to longer machining times, which can be detrimental to overall productivity. Finding the optimal parameters that strike a balance between energy efficiency and machining time is crucial for sustainable machining operations.
Dimensional Accuracy vs. Tool Wear: Achieving high dimensional accuracy requires precise control over machining parameters. However, aggressive cutting conditions that ensure dimensional accuracy can accelerate tool wear, leading to increased costs associated with tool replacement and maintenance. Striking a balance between dimensional accuracy and tool wear is necessary to optimise both quality and cost-effectiveness.
Multi-objective optimisation techniques offer several benefits. They facilitate improved decision-making by providing a comprehensive understanding of the trade-offs between different objectives. The outcome of multi-objective optimisation is a set of solutions known as the Pareto front, representing the best trade-offs between conflicting objectives. This provides decision-makers with a range of options to choose from, enhancing flexibility and adaptability. Moreover, by incorporating multiple sustainability objectives, such as energy consumption and tool wear, multi-objective optimisation enables the development of more sustainable machining processes.
However, one limitation of multi-objective optimisation is that it yields multiple solutions, and the selection of the final solution depends on user preferences and experience. This process often involves subjective judgements and trade-offs based on individual expertise and domain knowledge. To overcome this limitation, this study proposes a framework that combines multi-objective optimisation with Multiple-Criteria Decision Making (MCDM) techniques. MCDM techniques, such as Grey Relational Analysis (GRA), Technique for Order of Preference by Similarity to Ideal Solution (TOPSIS), and Analytic Hierarchy Process (AHP), can be employed to select the best solution from the set of optimal solutions provided by multi-objective optimisation. These techniques consider different criteria and assign weights to each criterion, helping to quantify preferences. Therefore, the aim of this study is to propose a framework to improve decision-making in the machining process, the objectives of the framework are as follows:
Design and conduct experiments to collect and calculate sustainability indicators machining process under different machining conditions.
Develop a mathematical model that captures the relationships between the machining conditions and the response variables.
Apply a multi-objective optimisation algorithm to the mathematical model to find the optimal machining conditions that minimise or maximise respective sustainability indicators, thereby improving economic viability and environmental impact.
Evaluate the sustainability of the machining process by analysing the results of the multi-objective optimisation through MCDM.
By addressing these objectives, this research aims to enhance the sustainability of magnesium alloy machining, improving economic feasibility and reducing environmental impact.
2. Literature review
2.1. Sustainability in manufacturing processes
The existing literature on sustainability assessment in manufacturing processes has predominantly focused on product-level sustainability rather than process-level evaluation (Abubakr et al. Citation2020; Bhanot, Rao, and Deshmukh Citation2016; Ingarao et al. Citation2012; Jayal et al. Citation2010; Labuschagne, Brent, and van Erck Citation2005; Linke et al. Citation2013; Lu et al. Citation2012; Mani et al. Citation2014; Olinto Citation2014; Saad, Nazzal, and Darras Citation2019; Singh et al. Citation2009). Several studies have introduced sustainability assessment indices such as the Product Sustainability Index (ProdSI) to evaluate the sustainability of manufactured goods without considering process variables (Hapuwatte et al. Citation2016).
However, limited research has been conducted on assessing sustainability at the process level, particularly for processes other than machining operations (Abbas et al. Citation2019; Bhanot, Rao, and Deshmukh Citation2016; Haq et al. Citation2021; Jamil et al. Citation2021; Khan et al. Citation2020; Linke et al. Citation2013; Singh et al. Citation2020). While studies have proposed frameworks and metrics-based assessment methods for machining operations, processes such as casting, joining, shaping, and forming have received less attention (Sivarupan et al. Citation2021; Wu et al. Citation2020). For example, research on welding processes has utilised sustainability assessment tools like weight space partitioning and life cycle assessment (LCA) to compare different welding methods and evaluate their sustainability performance (Sproesser et al. Citation2016).
Efficient energy and material use in sheet metal forming and die casting processes have also been studied, with researchers conducting sensitivity analyses and developing computer-aided tools for sustainability assessment (Ingarao et al. Citation2012; Singh et al. Citation2009).
Additionally, the evaluation of industrial technical devices in manufacturing processes has been recognised as crucial for enhancing sustainability. These devices offer advantages and opportunities but require critical evaluation to ensure effective sustainability assessment ().
Table 1. Advantages and disadvantages of industrial devices for sustainability in machining processes.
2.2. Sustainability assessment frameworks
The concept of sustainability has gained increasing significance in modern work environments and culture, with manufacturers and consumers alike emphasising sustainable practices and products. However, there is still a lack of consensus among scholars and practitioners regarding the definition of sustainable development (SD) and sustainable manufacturing. The widely accepted definition of sustainable development is provided by the Brundtland commission (undefined.), while the Department of Commerce’s definition is widely recognised for sustainable manufacturing (Kim et al. Citation2015; Sangwan and Mittal Citation2015).
A literature review of sustainability assessment frameworks and initiatives in manufacturing organisations reveals a range of practices and tools employed by researchers. These include the balanced scorecard method, sustainable value stream mapping, multi-criteria decision making, indicator-based assessment, software and mathematical modelling, life cycle analysis, product-service systems, and sustainability indices (Chou, Chen, and Conley Citation2015; Hallstedt Citation2017; Herrmann et al. Citation2014; Rajak and Vinodh Citation2015; Salvado et al. Citation2015).
Various types of sustainability assessment tools have been identified, including financial, biophysical, and indicator tools, each with subcategories. It is recommended to use a well-balanced ‘indicator tool’ that combines financial and biophysical aspects and incorporates stakeholder value orientations. However, a well-organised sustainability assessment framework is still lacking, leading to the use of different evaluation methodologies (Gasparatos and Scolobig Citation2012).
Another study proposes a hierarchical approach to sustainability assessment in the manufacturing sector, drawing inspiration from Maslow’s hierarchy of needs. The framework focuses on addressing complex interactions between elements, performance measures, industry, society, and ecosystems. It incorporates a sustainability cone-based model and includes life cycle stages during the early design phase. The framework encompasses organisational spheres, stakeholder involvement, product and process management, and sustainability dimensions, providing a systematic and holistic evaluation of sustainability performance (Bhakar, Digalwar, and Sangwan Citation2018). The process flow of the framework is shown in .
Figure 1. 5 level sustainability framework (Bhakar, Digalwar, and Sangwan Citation2018).

A proposed framework provides a systematic structure for evaluating sustainability performance in manufacturing processes. It incorporates qualitative and quantitative indicators with weighting methods, conducts quantification and normalisation, and utilises multi-criteria decision-making (MCDM) methods for aggregation. Sensitivity analysis is employed to assess aggregated scores. While the framework offers a structured approach for decision-making, it has limitations. It lacks optimisation algorithms, restricting exploration beyond available datasets, and does not handle complex environments. Integrating optimisation algorithms could enhance its capability, enabling trade-off exploration and handling diverse datasets. Future research should address these limitations to achieve more robust sustainability assessment in manufacturing processes (Saad, Nazzal, and Darras Citation2019). The detailed framework can be seen in .
Figure 2. MCDM based sustainability framework for manufacturing sector (Saad, Nazzal, and Darras Citation2019).

Sustainability in the manufacturing sector requires a multi-dimensional approach, as highlighted in the literature (Gunasekaran and Spalanzani Citation2012). Several studies have presented hierarchical frameworks for achieving sustainability in manufacturing, including five-level hierarchical frameworks (Batterham Citation2006; Mittal and Sangwan Citation2014). The environmental, social, and economic impacts of manufacturing processes have led to an increased emphasis on sustainability. Various frameworks have been developed to measure sustainability in manufacturing, but most lack automated selection of the appropriate solution after global optimisation. To address this gap, this article presents a comprehensive framework consisting of seven levels: identification of sustainability dimensions and indicators, design of experiments, data extraction, data refinement, data modelling and optimisation, decision-making, and result validation. The proposed framework covers the full scope of sustainable manufacturing, considers the global environment, and sets absolute targets for sustainability improvement. Seventeen sustainable development goals are incorporated into the framework, emphasising the importance of people in sustainable development (Transforming Our World: The Citation2030Agenda for Sustainable Development .:. Sustainable Development Knowledge Platform n.d..). The hierarchical approach is adopted to handle complex interactions between elements, performance measures, industry, society, and ecosystems (Batterham Citation2006).
2.3. Machine parameter optimisation for sustainability improvement
Rising manufacturing energy use has contributed to the energy problem and global warming, with manufacturing responsible for a significant portion (36%) of carbon dioxide emissions and energy consumption (Cai et al. Citation2019; Spiering et al. Citation2015; undefined). To address these challenges, optimising manufacturing processes, particularly in machine tools and turning operations, is crucial for reducing energy consumption and improving environmental sustainability (Givehchi, Haghighi, and Wang Citation2017; Hu et al. Citation2015).
Optimisation techniques play a vital role in enhancing sustainability in manufacturing by reducing energy consumption, waste generation, carbon footprint, and raw material usage while improving product quality (Eheliyagoda et al. Citation2022; Obaideen et al. Citation2022; Pérez-Fernández, Sebastián, and González-Gaya Citation2022; Pimenov et al. Citation2022; Roslan et al. Citation2022). Green machining techniques, such as dry machining, cryogenic machining, and minimum quantity lubrication, further contribute to sustainability by minimising environmental impact (Memić, Hasković Džubur, and Avdagić-Golub Citation2022).
By employing optimisation techniques, manufacturing firms can achieve greater process efficiency, cost reduction, and improved sustainability, aligning with the goals of energy efficiency and environmental responsibility (Singh et al. Citation2020; Vats et al. Citation2022). These techniques help identify optimal machining parameters that optimise material removal rate, surface quality, and energy consumption, leading to sustainable manufacturing practices
provides an overview of commonly studied machining processes, their parameters, and responses for optimisation. However, few studies have connected these responses to sustainability dimensions, hindering the quantification of sustainability in machining. To address this, our study aims to develop a framework that links sustainability with optimisation responses in machining. The proposed framework consists of seven levels and aims to calculate a numerical sustainability score. This framework can be applied to any machining process, addressing the need for a systematic approach to assess sustainability performance.
Table 2. Machining parameter for optimisation.
3. Proposed sustainability assessment framework
Sustainability has become an essential aspect of modern manufacturing. To assess the sustainability of a manufacturing process, it is necessary to have a robust and comprehensive framework. This article proposes a sustainability assessment framework that consists of seven levels, as discussed earlier, and an additional feature of best optimal solution selection among various. The proposed framework is designed to help numerically calculate the sustainability of any well-defined process and select the best optimal solution. below shows a graphical representation of the proposed sustainability assessment framework. Moreover, complete process flowchart of the framework can be seen in .
Figure 3. Proposed framework.

Figure 4. Technical representation of proposed framework.

Identification of indicators (Independent and Dependent Variables):
Figure 5. (Continued).

Identify the key machining parameters that significantly affect the machining process of magnesium alloys. These parameters may include cutting speed, feed rate, depth of cut, tool material, tool geometry, and cooling/lubrication techniques (Independent variables). Determine the response variables that reflect the performance and quality of the machining process, such as surface roughness, material removal rate, cutting forces, tool wear, and dimensional accuracy (Dependent Variables). Section 3.1 provides a comprehensive discussion on the various indicators identified in the literature.
Design of Experiment:
Utilise Taguchi orthogonal array to design a set of experiments that efficiently explore the effects of different machining parameters on the response variables. Assign specific levels or values to each parameter in the orthogonal array to cover a wide range of conditions while minimising the number of experimental runs. Section 3.2 elaborates on additional methodologies for experimental design that have been documented in the literature
Data Extraction through Sensor Fusion:
Install suitable sensors and measurement devices to collect data during the machining experiments. Capture relevant process variables, such as temperatures, vibrations, forces, and displacements, using sensor fusion techniques that integrate data from multiple sensors. This fusion process ensures comprehensive and accurate data collection. elaborates on additional methodologies for experimental design that have been documented in the literature. Section 3.3 presents a comprehensive list of the key machining parameters that significantly impact the economic, environmental, and social sustainability of the machining process. Additionally, it discusses the specific measuring devices, such as sensors, that are necessary for monitoring and extracting data related to these parameters.
Data Refinement and Dimension Reduction:
The collected data is refined and analysed to evaluate the effects of different machining parameters on the response variables. A main effect plot is constructed to visualise the impact of each parameter on the response variables and identify the significant factors influencing the machining process. In Section 3.4, the study delves into the intricate steps of the data refinement process. Specifically, it discusses the utilisation of S/N analysis (Signal-to-Noise analysis) and PCA (Principal Component Analysis) as techniques for dimensional reduction in machine parameter optimisation. The section provides a detailed exploration of how these methods are applied to refine and streamline the data, enabling a more focused and efficient optimisation process.
Data Modelling:
Develop mathematical models or utilise machine learning techniques to establish the relationships between the machining parameters and response variables. Use regression analysis or advanced algorithms to create models for each response variable, such as surface roughness prediction, material removal rate estimation, or cutting force optimisation. Tune the efficiency of each model through hyperparameter optimisation to enhance accuracy and predictive capability. Section 3.5 delves into the various data modelling techniques found in literature, employed to develop an efficient model that accurately represents the machining process. These techniques aim to capture the complex relationships between the machining parameters and the corresponding response variables, enabling the creation of a robust and reliable model.
Multi-objective Optimisation of models:
Apply multi-objective optimisation techniques to optimise the machining process for magnesium alloys. Define objective functions considering sustainability and performance aspects, such as minimising energy consumption, reducing tool wear, and maximising material removal rate or surface quality. Adjust hyperparameters of the optimisation process to obtain optimal solutions that strike a balance between conflicting objectives. Section 3.5 explores the diverse range of multi-objective optimisation algorithms utilised in the literature for optimising machining parameters.
Decision Making (Ranking Optimal Solutions using MCDM Techniques):
Rank the obtained optimal solutions achieved through multi-objective optimisation and evaluate their sustainability values using Multiple-Criteria Decision Making (MCDM) techniques. Assess sustainability metrics by considering economic, environmental, and social factors associated with the machining process. Employ MCDM methods to compare and rank the solutions, ultimately selecting the most sustainable alternative. This approach ensures a comprehensive evaluation of the optimal solutions and facilitates informed decision-making based on the identified sustainability criteria. Section 3.6 provides an overview of various classic Multiple-Criteria Decision Making (MCDM) approaches found in the literature that have been applied to similar types of applications.
Validation:
Validate the selected optimal solution by conducting a machining experiment based on the identified machining parameters. Implement the machining process under the optimised conditions and evaluate its performance by comparing the experimental results with the predicted values from the data models. Analyze any discrepancies or deviations, and make necessary adjustments to further optimise the process if required.
By following this specific methodology, the proposed framework aims to achieve sustainable machining of magnesium alloys, considering factors such as efficiency, performance, and sustainability in the overall process. Section 3.7 explores additional validation approaches employed in the literature for similar types of applications.
3.1. Identification of indicators
The proposed sustainability assessment framework begins with the identification of indicators, which are measures of environmental, social, and economic sustainability (Azapagic Citation2004). This involves conducting a literature review, consulting with stakeholders, and evaluating the indicators based on criteria such as relevance and reliability (Dubravská et al. Citation2020; Schaltegger, Bennett, and Burritt Citation2006; Vishnu, Varghese, and Gurumoorthy Citation2023; Welford Citation2016). The selected indicators should cover environmental, social, and economic aspects and can include factors like greenhouse gas emissions, employee health and safety, and profitability. These indicators are then used to assess the sustainability performance of the manufacturing process and track improvements over time. presents a range of indicators used in various systems to assess sustainability, highlighting the importance of sustainable materials and manufacturing processes (ISO 14,040 guidelines, LCA methodology).
Table 3. Sustainability assessment systems.
3.1.1. Environmental indicators
The environmental dimension of sustainability focuses on preserving natural resources, maintaining a balance between resource consumption and regeneration, and avoiding exceeding planetary boundaries (Bag, Panda, and Kumar Sahoo Citation2022; Gerten et al. Citation2019; Jie et al. Citation2023; Oláh et al. Citation2020). Environmental indicators serve as early warning systems and monitoring tools for tracking environmental damage. These indicators include metrics such as wastewater generation, water consumption, and material usage/recycling, as shown in (Estoque et al. Citation2020).
Table 4. List of environmental indicators.
3.1.2. Economic indicators
The economic dimension of sustainability in manufacturing is concerned with the financial well-being of stakeholders and the impact on local and global economic systems (Sartal et al. Citation2020; Simpson and Tamayo Citation2020). It encompasses all economic transactions, including intangible assets and unconventional financial indicators (Roinioti and Koroneos Citation2019). However, defining what needs to be sustained from an economic perspective is not always clear (Lee et al. Citation2020). Compared to the environmental aspect, the economic dimension of sustainability has received less research attention, resulting in a lack of comprehensive indicator frameworks (Kim, Kim, and Lee Citation2013). Economic indicators used in manufacturing operations mainly focus on costs and profits, as shown in .
Table 5. List of economic indicators.
3.1.3. Social indicators
The social dimension of sustainability in manufacturing encompasses equity, accessibility, participation, and other elements that foster societal cohesiveness (Akcali and Cahantimur Citation2022). However, quantifying and incorporating the social dimension into assessments has been challenging, resulting in limited research and practice focus (Padilla-Rivera, Russo-Garrido, and Merveille Citation2020). Few established social indicators exist for the manufacturing industry, despite the importance of considering positive and negative social consequences (Nara et al. Citation2021). More attention is needed to assess intangible qualities such as consumer satisfaction (Morkunas and Rudienė Citation2020). Social indicators, both qualitative and quantitative, provide insights into the social dimension. presents a selection of social indicators reported in the literature, capturing aspects like satisfaction with wages, work-related accidents, and employee turnover rates. To comprehensively evaluate social sustainability, it is essential to utilise a combination of qualitative and quantitative indicators (Mies and Gold Citation2021).
Table 6. List of social indicators.
3.2. Design of experiment
Design of Experiments (DOE) is a statistical tool used to systematically analyse experiments and optimise machining processes. Various DOE methodologies are utilised, including full factorial design, fractional factorial design, response surface methodology (RSM), and the Taguchi method. The selection of the DOE methodology depends on factors such as problem complexity, the number of input variables, and available resources. Full factorial design offers a comprehensive analysis but can be time-consuming and costly (Kechagias et al. Citation2020). Fractional factorial design reduces the number of experiments while providing valuable insights (Horvath, Matyasi, and Dregelyi-Kiss Citation2014). RSM helps identify optimal input combinations for desired outcomes (Cardoso et al. Citation2021). The Taguchi method focuses on robust parameter settings, reducing variability and noise in the process (Yaka Citation2021). The choice of DOE methodology should consider the specific requirements and constraints of the machining problem (Daniel et al. Citation2020).
3.3. Data extraction
Data extraction in machining involves collecting and transforming raw data from various sensors to optimise machining parameters and improve efficiency. Sensor fusion combines data from multiple sensors, such as cutting force (Shah et al. Citation2022; Zarrouk et al. Citation2022), vibration (Kouahla et al. Citation2022; Sahoo et al. Citation2023), acoustic emission (Dixit, Sharma, and Kumar Citation2022), temperature (Delijaicov et al. Citation2023; Soori and Arezoo Citation2023), and power consumption (Li et al. Citation2023), to provide a comprehensive view of the machining process. This data can be used to analyse tool wear (Cheng et al. Citation2023; Nguyen et al. Citation2022), surface quality (Ishfaq, Rehman, and Wang Citation2023; Xie et al. Citation2023), chip formation (Bhuiyan, Choudhury, and Dahari Citation2014), and other factors.
3.4. Data refinement
Data refinement is a critical step in machine parameter optimisation, involving techniques such as data cleaning (Diao, Yan, and Gao Citation2022), transformation (Aydemir, Taskaya Temizel, and Temizel Citation2022), aggregation (Department of Electrical Engineering, Jamia Millia Islamia, New Delhi, India, 110001, Singh, J., din, Z., & Department of Electrical Engineering, Jamia Millia Islamia, New Delhi, India, 110001 Citation2022), sampling (Huang Citation2022), and feature selection (El-Kenawy et al. Citation2023). Data cleaning corrects errors, while transformation standardises or normalises data. Aggregation summarises data, sampling selects subsets for analysis, and feature selection focuses on relevant variables. The most commonly used algorithms for feature selection are Principal Component Analysis (PCA) and Single-to-Noise ratio Analysis (S/N). PCA reduces dimensionality, capturing variance in the data, while S/N ratio identifies influential factors. PCA combines original features, and S/N ratio measures signal strength relative to noise. These techniques enhance the accuracy and reliability of data in machine parameter optimisation.
3.5. Data modelling and optimisation
Data modelling is the process of creating a mathematical or statistical model to represent a real-world system or process. Optimisation, on the other hand, involves finding the best set of input parameters to maximise or minimise a particular output variable based on the model (Qiao et al. Citation2022). In the context of machine parameter optimisation, data modelling and optimisation are used to predict and optimise machining parameters, such as cutting speed, feed rate, and depth of cut, to improve machining efficiency and reduce manufacturing costs. Data modelling and optimisation are important steps in machine parameter optimisation that involve creating a mathematical model of the system and using optimisation algorithms to improve its performance. These steps are often used one after the other to ensure that the model accurately represents the system and that it is optimised to achieve the desired response.
After data refinement and feature selection, the refined data is used to create a mathematical model of the system. Each response is mapped to the most influential machining parameters obtained after S/N or PCA analysis. This helps the model increase its efficiency and avoid disturbances. Once an accurate model is generated, it can be optimised using one of the many algorithms available to obtain the minimum or maximum values of the responses. represents the literature discussing all the algorithms currently used in the field of machine parameter optimisation.
Table 7. Review of modelling and optimisation in machine parameter optimisation.
3.6. Decision making and sustainability assessment
Machine parameter optimisation is a complex problem that involves multiple objectives, and the trade-offs between these objectives lead to multiple sets of optimal solutions. The selection of the most suitable solution among the obtained ones is done manually based on the advice of an expert in the field. However, this issue can be resolved by applying Multi-Criteria Decision Making (MCDM) techniques like TOPSIS (Technique for Order Preference by Similarity to Ideal Solution), GRA (Grey Relational Analysis), and many others (Bhuvanesh Kumar et al. Citation2023; Pinzon Amorocho and Hartmann Citation2022).
MCDM helps identify the most suitable solution based on applied weights and criteria. The weights can be applied using various algorithms available in the literature like Analytic Hierarchy Process (AHP) (Singh Sivam, Rajendran, and Harshavardhana Citation2022), Fuzzy weights (Ye et al. Citation2022), and others. The weight applied to each criterion represents its importance.
Moreover, the result obtained from MCDM can be validated using a sustainability assessment model that considers the economic, social, and environmental aspects of the optimised responses/indicators. The performance of each indicator can be calculated using the Equations 1–3. The graphical representation can be seen in .
Figure 6. Technical indicators to assess sustainability.

3.7. Results validation
To validate the results obtained through parameter optimisation and sustainability assessment, a robust validation process is crucial. This process involves two key aspects: utilising sustainability assessment criteria (Kalateh, Kianifar, and Sardarabadi Citation2022) and conducting experimental validation (Mahfoud et al. Citation2022; Zamfirache et al. Citation2022).
Firstly, the framework emphasises the use of sustainability assessment criteria to evaluate the optimised parameters. These criteria encompass various dimensions, including environmental impact, social responsibility, and economic feasibility. By considering these criteria, the framework ensures that the optimised parameters not only improve the performance of the machining process but also contribute positively to the broader sustainability goals. The criteria can be tailored to specific industries or processes, allowing for a customised and comprehensive evaluation of sustainability.
Secondly, experimental validation is a critical step in the validation process. It involves conducting physical experiments or simulations to verify the performance of the optimised parameters in real-world or simulated scenarios. This validation helps to confirm that the optimised parameters indeed lead to improved machining process performance while aligning with sustainability objectives. By comparing the experimental results with the predicted outcomes from parameter optimisation, the framework ensures the reliability and applicability of the optimised parameters.
4. Case study
The case study presented in this section aims to implement the proposed framework for sustainability assessment of the machining process through machining parameter optimisation technique. The dataset utilised in this study comprises experimental data obtained from runs on a milling machine, conducted under diverse operating conditions. The current case is focused on determining the optimal machining parameters to machine the two materials found in the dataset i.e. cast iron and steel using the propose framework. Within this dataset, a total of 16 cases were examined , each comprising a varying number of runs. The number of runs conducted for each case was contingent upon the degree of flank wear observed between runs. These measurements were taken at irregular intervals up to a predefined wear limit and occasionally extended beyond it.
Table 8. Experimental conditions.
By utilising this dataset, the case study aims to provide readers with a practical illustration of the proposed framework’s application, offering valuable insights into how the framework can be effectively implemented to optimise machining parameters while ensuring sustainability. Through this empirical investigation, readers will gain firsthand knowledge of the framework’s functionalities and its potential to enhance machining process performance and sustainability outcomes.
4.1. Identification of indicators
The dataset utilised in this study encompasses a range of response variables that serve as indicators for computing sustainability in the machining process. These response variables include Flankwear (VB), Machining time (t), Spindle motor AC current consumption (I), Spindle Vibration (V), and Spindle acoustic emission (A). Each of these variables plays a crucial role in assessing different aspects of sustainability.
The computation of economic sustainability involves reducing Flankwear (VB), Machining time (t), Spindle Vibration (V) and Spindle motor AC current consumption (I). These indicators directly impact the economic viability of the machining process by minimising tool wear, optimising the time required for machining, and reducing energy consumption.
Environmental sustainability, on the other hand, focuses on reducing Spindle motor AC current consumption (I). By minimising energy consumption, this indicator contributes to lowering the environmental impact associated with the machining process. The emphasis on energy efficiency aligns with the broader goal of sustainable manufacturing practices.
Additionally, social sustainability is evaluated through the indicator of Spindle acoustic emission (A). This variable allows for the assessment of the impact of machining on worker safety and well-being. By considering acoustic emissions, potential risks and disturbances to the workforce can be identified and mitigated, promoting a socially responsible machining environment.
4.2. Design of experiment
This study employed a factorial design L16 array and conducted 166 experiments, each replicated 16 times, to investigate the impact of machining parameters on sustainability indicators. The factorial design facilitated the simultaneous examination of multiple factors, while replication enhanced data precision and allowed for the evaluation of experimental variability. The sustainability indicators measured included Flankwear (VB), Machining time (t), Spindle motor AC current consumption (I), Spindle Vibration (V), and Spindle acoustic emission (A), representing economic, environmental, and social aspects. The comprehensive dataset obtained from the experiments provided a robust foundation for analysing the relationships between machining parameters and sustainability.
4.3. Data extraction
This study employed specific sensors to extract data during the machining process. The WD 925 acoustic sensor captured acoustic emission, while the 720,150 accelerometer measured vibrations. Additionally, the CTA 213 current sensor monitored spindle motor current. These sensors provided valuable insights into the behaviour, dynamics, and energy consumption of the machining operation. The data extracted from these sensors facilitated a comprehensive analysis of performance, efficiency, and sustainability indicators. By integrating these sensors and data extraction techniques, the study aimed to enhance understanding and optimise machining practices.
4.4. Data refinement
During the data refinement process, a main effect plot was generated to analyse the influence of each machining parameter on the material and responses as shown in . Surprisingly, the main effect plot indicated that every response was significantly affected by every parameter. This implies that there was no specific parameter dominating any particular response. As a result, the reduction in dimension for modelling purposes was deemed not applicable for any of the responses. This finding highlights the complex interdependencies and intricate relationships between the machining parameters and the responses, suggesting that a comprehensive approach considering all parameters may be necessary for optimising the machining process.
4.5. Data modelling
After the data refinement process and determining the necessary dimensions for modelling each response, the next step involves utilising linear regression models to obtain mathematical representations of the responses. These regression models not only consider the individual factors but also incorporate their interactions.
In the table below, the models for each material and their corresponding responses are presented. These models provide a quantitative understanding of the relationship between the machining parameters and the desired outcomes, enabling better control and optimisation of the machining process.
By employing regression analysis, it becomes possible to identify the significant factors and their impact on the responses, facilitating decision-making and process improvements. The accuracy of the prediction serves as an indicator of the reliability and effectiveness of the developed models. The obtained mathematical models serve as valuable tools for further analysis, optimisation, and decision support in the context of machining processes.
4.6. Data optimisation
In order to improve the performance and efficiency of the machining process, the generated data presented in is subjected to an unconstrained optimisation using a classical genetic algorithm. The MATLAB Optimisation Toolbox was utilised to optimise the generated model in . Unconstrained optimisation techniques were employed, leveraging the flexibility provided by the toolbox. Hyperparameters, such as 500 generations and a population size of 50, were carefully tuned to obtain the best optimal solutions.
Table 9. Response regression models for both materials.
The optimisation process aimed to enhance the performance and efficiency of the machining process by exploring the parameter space. The results of the optimisation are presented in the table below in , showcasing the optimal solutions achieved through the MATLAB Optimisation Toolbox for cast iron and steel respectively.
Table 10. Optimal set of solutions for cast iron material.
Table 11. Optimal set of solutions for steel material.
4.7. Decision making and sustainability assessment
The novelty of this framework lies in its incorporation of a multi-criteria decision-making (MCDM) technique to validate and explain the optimal solutions obtained. In this case study, the Grey Relational Analysis (GRA) method is employed for decision-making purposes. The upcoming sections discuss the step-by-step procedure for applying GRA and assessing sustainability within the proposed framework.
To facilitate the decision-making process, the values of the responses are normalised using EquationEquation 4(4)
(4) and EquationEquation 5
(5)
(5) . These equations ensure that the responses are evaluated based on the ‘smaller-the-better’ or ‘larger-the-better’ characteristic. In this particular case study, all the responses, including flankwear, machining time, current consumption, vibrations, and acoustic emission, are expected to be smaller for better performance.
By normalising the dataset, the responses are transformed into a normalised dataset, which is presented below. This normalisation process enables a comprehensive analysis and comparison of the responses to support the MCDM technique’s application and subsequent sustainability assessment within the framework.
The normalised values obtained from are used for calculating the sustainability metrics. In this analysis, the weight of each response is assigned a value of 1, indicating equal importance. The sustainability metrics are calculated based on the following considerations:
Economic Sustainability (ESA): Economic sustainability is evaluated by considering the variables Vb (flank wear), T (machining time), I (spindle motor AC current consumption), and V (spindle vibration). Reducing these parameters leads to cost reduction and improved product quality, thereby enhancing economic sustainability.
Environmental Sustainability (EnSA): Environmental sustainability is assessed by focusing on the variable I (spindle motor AC current consumption). By reducing the current consumption, energy usage is minimised, resulting in lower greenhouse gas emissions and improved environmental sustainability.
Social Sustainability (SSA): Social well-being is linked to the variable A (spindle acoustic emission), which represents noise levels. By reducing acoustic emissions and noise levels, the working environment becomes more conducive, benefiting the well-being and comfort of workers. This contributes to enhanced social sustainability.
Table 12. Normalised solutions.
By considering these factors the values were calculated and shown in for cast iron and steel respectively, the sustainability metrics provide a comprehensive evaluation of the machining process. The goal is to optimise the machining parameters in a way that ensures economic efficiency, environmental responsibility, and social well-being are taken into account.
Table 13. Sustainability assessment of cast iron through normalised responses.
Table 14. Sustainability assessment of steel through normalised responses.
The economic, environmental, and social sustainability metrics obtained previously are further normalised using the larger-the-better characteristic. This normalisation process aims to facilitate a fair and consistent comparison among the different sustainability metrics. The normalised metrics are presented in the .
Table 15. Normalised sustainability metrics.
The performance of each experiment for specific sustainability metrics is evaluated by comparing the processed data values obtained through data pre-processing. The experiment with a value closest to 1 is considered to have the best performance for that response. To facilitate comparison, a reference sequence (X0) is established with reference values for each metric. The deviation sequences in indicate the proximity of the comparability sequences to the reference sequence, enabling the determination of each experiment’s relative performance for different responses.
Table 16. Deviation sequence.
The grey relational coefficient is utilised to assess the proximity between xij and x0j, where a larger coefficient indicates a closer resemblance. The coefficient can be calculated using the equation 6, with representing the distinguishing coefficient ranging from 0 to 1. In this study,
is assumed to be 0.5, reflecting a moderate level of distinguishability. A smaller value of zeta indicates a higher level of distinguishability between the compared values.
The grey relational grade is a measure used to quantify the similarity between a comparability sequence and a reference sequence in the grey relational space. It is computed as a weighted sum of grey relational coefficients, representing the degree of closeness between the sequences. The grey relational grade is determined using the provided equation 7, where n represents the number of responses and wj denotes the weight assigned to each response.
There are various methodologies to calculate the weight of each response like analytical heretical process (AHP), Fuzzy weights, etc. In this study, the equal weight approach is opted and weight of every response is kept at 1. The grey relational grade serves as an indicator of the level of similarity between the comparability sequence and the reference sequence. The experiment with the highest grey relational grade indicates the best choice, as it exhibits the closest resemblance to the reference sequence.
displays the grey relational coefficients and grades for each experiment. Notably, experiment number 14 (Cast Iron) and experiment number 11 steel, highlighted in yellow, demonstrates the best optimal combination of controllable parameters.
Table 17. Grey rational coefficients and grades.
Based on the Grey Relational Analysis (GRA), the optimal machining parameters for cast iron are determined to be a Depth of Cut (DOC) of 0.8 and a feed rate of 0.5. Similarly, for steel, the optimal parameters are a DOC of 1.4 and a feed rate of 0.5. These parameter combinations have been ranked as the top choices among all the optimal solutions obtained based on sustainability metrics.
4.8. Validation
In this case study, a framework for sustainability assessment of the machining process through parameter optimisation has been developed and applied to an available dataset. Due to the unavailability of specific testing conditions and environmental factors during data extraction, experimental validation was not conducted. Instead, the validation of the framework was performed using sustainability assessment metrics. The metrics considered economic, environmental, and social sustainability factors to evaluate the performance of the optimised machining parameters. The results obtained through the sustainability assessment metrics provide valuable insights into the sustainability of the optimised solutions. Although experimental validation was not possible, the rigorous analysis and evaluation of the dataset using sustainability metrics ensure the reliability and applicability of the framework. The findings contribute to the advancement of sustainable machining practices and serve as a reference for future research and applications.
4.9. Conclusion
In this case study, the objective was to determine the optimal machining conditions for cast iron and steel based on a provided dataset obtained through milling machining. The dataset consisted of responses such as flankwear, machining time, spindle current consumption, spindle vibration, and acoustic emission, which were linked to sustainability indicators for assessing the economic, environmental, and social sustainability of the machining process.
By following the proposed framework, a set of optimal solutions was obtained. These solutions were then analysed using the Grey Rational Analysis (GRA) which is a MCDM technique, and sustainability metrics were calculated to identify the best optimal solution. The findings revealed that for machining cast iron, a depth of cut (DOC) of 0.8 and a feed rate of 0.5 should be maintained, while for steel, a DOC of 1.4 and a feed rate of 0.5 are recommended. These machining parameters ensure sustainable machining for both materials, contributing to improvements in economic, environmental, and social aspects.
Through the integration of MCDM techniques and sustainability assessment metrics, this case study demonstrates the importance of considering multiple sustainability dimensions in optimising machining processes. The findings provide valuable insights for practitioners in the manufacturing industry to make informed decisions for sustainable machining operations. Further research and implementation of the proposed framework can contribute to the advancement of sustainable manufacturing practices and help mitigate the environmental impacts of machining processes while considering economic viability and social well-being.
5. Conclusion and future directions
This review paper highlights the prevalence of multi-objective optimisation techniques in machine parameter optimisation and sustainability assessment; it is evident that multi-objective optimisation techniques are widely used in this field. However, a key gap identified is the lack of a standardised procedure for selecting the best solution from the set of optimal solutions generated by these techniques. To address this gap, a holistic framework has been developed that incorporates multi-criteria decision-making techniques to evaluate the sustainability of machining processes. This framework enables an objective and comprehensive evaluation of the set of optimal solutions obtained through parameter optimisation.
In addition to the framework, a case study was conducted to validate its effectiveness. The case study focused on determining the optimal machining conditions for cast iron and steel using a provided dataset obtained through milling machining. By following the proposed framework, a set of optimal solutions was obtained. These solutions were then analysed using the Grey Rational Analysis (GRA) which is a MCDM technique, and sustainability metrics were calculated to identify the best optimal solution. The findings revealed that for machining cast iron, a depth of cut (DOC) of 0.8 and a feed rate of 0.5 should be maintained, while for steel, a DOC of 1.4 and a feed rate of 0.5 are recommended. These machining parameters ensure sustainable machining for both materials, contributing to improvements in economic, environmental, and social aspects.
Through the integration of MCDM techniques and sustainability assessment metrics, this case study demonstrates the importance of considering multiple sustainability dimensions in optimising machining processes. The findings provide valuable insights for practitioners in the manufacturing industry to make informed decisions for sustainable machining operations. Further research and implementation of the proposed framework can contribute to the advancement of sustainable manufacturing practices and help mitigate the environmental impacts of machining processes while considering economic viability and social well-being.
Looking ahead, several future directions can advance this field. Firstly, developing more sophisticated and efficient algorithms for multi-objective optimisation, capable of handling a large number of objectives and decision variables, will enhance optimisation outcomes. Additionally, integrating artificial intelligence and machine learning techniques into the framework can improve the accuracy and efficiency of the optimisation process.
Moreover, exploring the applicability of the framework across diverse machining processes and industries will yield valuable insights and expand practical implications. Investigating the economic aspects of sustainable machining, such as cost optimisation and resource efficiency, can provide further avenues for research. Furthermore, considering the social dimension of sustainability, including worker safety, fair labour practices, and community engagement, will contribute to a more holistic approach.
It is important to note that the manufacturing sector’s contributions to sustainable development extend beyond the scope of this research. While manufacturing drives economic growth, it must also embrace sustainability to mitigate environmental impacts and ensure the well-being of its workforce.
Abbreviation
Abbreviation | = | Details |
AHP | = | Analytical Hierarchy Process |
ANN | = | Artificial Neural Network |
ANOVA | = | Analysis of Variance |
AWJM | = | Abrasive water jet machining |
CCD | = | Central Composite Design |
CFRP | = | Carbon Fiber-Reinforced Plastic |
CNC | = | Computer Numeric Control |
CRITIC | = | Criteria Importance through Inter-Criteria |
DEAR | = | Data Envelopment Analysis Ranking |
DOE | = | Design of Experiment |
EDM | = | Electrical Discharge Machining |
EDX | = | Energy Dispersive X-ray Analysis |
FEM | = | Finite Element Analysis |
FSW | = | Friction Stir Welding |
GA | = | Genetic Algorithm |
GRA | = | Grey Rational Analysis |
GRI | = | Global Reporting Initiative |
IEA | = | International Energy Agency |
ISO | = | International Standards Organization |
LCA | = | Life-Cycle Analysis |
MADM | = | Multi-Attribute Decision Making |
MCDM | = | Multi-Criteria Decision Making |
MEC | = | Machining Energy Consumption |
MOGA | = | Multi-Objective Genetic Algorithm |
MOORA | = | Multi-Objective Optimisation by Ratio Analysis |
MOPSO | = | Multi-objective Particle Swarm Optimisation |
MQL | = | Minimum Quantity Lubrication |
NSGA | = | Non-dominated Sorting Genetic Algorithm |
PBOM | = | Production Bills Of Material |
PCA | = | Principal Component Analysis |
PID | = | Proportional-Integral-Derivative |
ProdSI | = | Product Sustainability Index |
RSM | = | Response Surface Methodology |
SDG | = | Sustainable Development Goals |
SEM | = | Scanning Electron Microscope |
TLBO | = | Teaching learning-based optimisation |
TOPSIS | = | Technique for Order Performance by Similarity to Ideal Solution |
WASPAS | = | Weighted Aggregated Sum Product Assessment |
WBGT | = | Wet Bulb Globe Temperature |
WEDM | = | Wire Electrical Discharge Machining |
WEF | = | World Economic Forum |
Acknowledgments
The dataset used in this research paper is the “Milling Data Set” obtained from the BEST lab at UC Berkeley, as acknowledged by A. Agogino and K. Goebel in 2007. This dataset is available in the NASA Ames Prognostics Data Repository, maintained by NASA Ames Research Center in Moffett Field, CA. (Source: http://ti.arc.nasa.gov/project/prognostic-data-repository).
Disclosure statement
‘This work was supported by the Government of Pakistan Planning Commission under Grant Establishment of National Center in Big Data & Cloud Computing (NCBC). The funding provided by the Government of Pakistan Planning Commission was instrumental in enabling the execution of this research project’.
Additional information
Funding
Notes on contributors
Muhammad Atif
Muhammad Atif is currently a PhD student in the field of Computer Science at SZABIST University. He also holds the position of Assistant Professor at the same institution. His research interests encompass a variety of topics including mechanical design, optimal machining, renewable energy, embedded systems, and automation.
Faraz Junejo
Faraz Junejo obtained his PhD (Mechatronics) degree from Loughborough University, UK. He is currently working as Professor in Mechatronics Engineering Department at SZABIST, Karachi. His research interests include Machine Vision, Renewable Energy, Condition monitoring, Robotics and Engineering Mechanics. He is an active member of Higher Education Commission’s National Curriculum review committee for both Mechatronics and Mechanical Engineering.
Imran Amin
Imran Amin is an Professor and Head of Department at SZABIST Institute in Karachi. He has served as the Head for Center of Renewable Energy and Research from 2009 to 2012.
References
- Abas, M., L. Sayd, R. Akhtar, Q. S. Khalid, A. M. Khan, and C. I. Pruncu. 2020. “Optimization of Machining Parameters of Aluminum Alloy 6026-T9 Under MQL-Assisted Turning Process.” Journal of Materials Research and Technology 9 (5): 10916–10940. https://doi.org/10.1016/j.jmrt.2020.07.071.
- Abbas, A. T., M. K. Gupta, M. S. Soliman, M. Mia, H. Hegab, M. Luqman, and D. Y. Pimenov. 2019. “Sustainability Assessment Associated with Surface Roughness and Power Consumption Characteristics in Nanofluid MQL-Assisted Turning of AISI 1045 Steel.” The International Journal of Advanced Manufacturing Technology 105 (1–4): 1311–1327. https://doi.org/10.1007/s00170-019-04325-6.
- Abubakr, M., A. T. Abbas, I. Tomaz, M. S. Soliman, M. Luqman, and H. Hegab. 2020. “Sustainable and Smart Manufacturing: An Integrated Approach.” Sustainability 12 (6): 2280. https://doi.org/10.3390/su12062280.
- Abubakr, M., M. A. Hassan, G. M. Krolczyk, N. Khanna, and H. Hegab. 2021. “Sensors Selection for Tool Failure Detection During Machining Processes: A Simple Accurate Classification Model.” CIRP Journal of Manufacturing Science and Technology 32:108–119. https://doi.org/10.1016/j.cirpj.2020.12.002.
- Akcali, S., and A. Cahantimur. 2022. “The Pentagon Model of Urban Social Sustainability: An Assessment of Sociospatial Aspects, Comparing Two Neighborhoods.” Sustainability 14 (9): 4990. https://doi.org/10.3390/su14094990.
- Al-Naggar, Y. M., N. Jamil, M. F. Hassan, and A. R. Yusoff. 2021. “Condition Monitoring Based on IoT for Predictive Maintenance of CNC Machines.” Procedia CIRP 102:314–318. https://doi.org/10.1016/j.procir.2021.09.054.
- Alshibi, A., A. Nasreldin, and S. Pervaiz. 2023. “Sustainable Vegetable Oil-Based Minimum Quantity Lubrication Assisted Machining of AZ91 Magnesium Alloy: A Grey Relational Analysis-Based Study.” Lubricants 11 (2): 79. https://doi.org/10.3390/lubricants11020079.
- Asgari, A., H. Delavar, and M. Sedighi. 2023. “Microstructure and Surface Integrity of Machined AZ91 Magnesium Alloy.” Journal of Materials Research and Technology 22:735–746. https://doi.org/10.1016/j.jmrt.2022.11.164.
- Ashima, R., A. Haleem, M. Javaid, and S. Rab. 2022. “Understanding the Role and Capabilities of Internet of Things-Enabled Additive Manufacturing Through Its Application Areas.” Advanced Industrial and Engineering Polymer Research 5 (3): 137–142. https://doi.org/10.1016/j.aiepr.2021.12.001.
- Aydemir, A. E., T. Taskaya Temizel, and A. Temizel. 2022. “A Machine Learning Ensembling Approach to Predicting Transfer Values.” SN Computer Science 3 (3): 201. https://doi.org/10.1007/s42979-022-01095-z.
- Azapagic, A. 2004. “Developing a Framework for Sustainable Development Indicators for the Mining and Minerals Industry.” Journal of Cleaner Production 12 (6): 639–662. https://doi.org/10.1016/S0959-6526(03)00075-1.
- Bag, R., A. Panda, and A. Kumar Sahoo. 2022. “A Concise Review on Environmental Sustainable Machining Conditions of Hard Part Materials.” Materials Today: Proceedings 62:3724–3728. https://doi.org/10.1016/j.matpr.2022.04.433.
- Barni, A., C. Capuzzimati, A. Fontana, M. Pirotta, S. Hänninen, M. Räikkönen, and T. Uusitalo. 2022. “Design of a Lifecycle-Oriented Environmental and Economic Indicators Framework for the Mechanical Manufacturing Industry.” Sustainability 14 (5): 2602. https://doi.org/10.3390/su14052602.
- Batterham, R. J. 2006. “Sustainability—The Next Chapter.” Chemical Engineering Science 61 (13): 4188–4193. https://doi.org/10.1016/j.ces.2005.10.016.
- Bhakar, V., A. K. Digalwar, and K. S. Sangwan. 2018. “Sustainability Assessment Framework for Manufacturing Sector – a Conceptual Model.” Procedia CIRP 69:248–253. https://doi.org/10.1016/j.procir.2017.11.101.
- Bhanot, N., P. V. Rao, and S. G. Deshmukh. 2016. “An Assessment of Sustainability for Turning Process in an Automobile Firm.” Procedia CIRP 48:538–543. https://doi.org/10.1016/j.procir.2016.03.024.
- Bhuiyan, M. S. H., I. A. Choudhury, and M. Dahari. 2014. “Monitoring the Tool Wear, Surface Roughness and Chip Formation Occurrences Using Multiple Sensors in Turning.” Journal of Manufacturing Systems 33 (4): 476–487. https://doi.org/10.1016/j.jmsy.2014.04.005.
- Bhuvanesh Kumar, M., J. Antony, E. Cudney, S. L. Furterer, J. A. Garza-Reyes, and S. M. Senthil. 2023. “Decision-Making Through Fuzzy TOPSIS and COPRAS Approaches for Lean Tools Selection: A Case Study of Automotive Accessories Manufacturing Industry.” International Journal of Management Science and Engineering Management 18 (1): 26–35. https://doi.org/10.1080/17509653.2022.2064356.
- Bonilla Hernández, A. E., T. Lu, T. Beno, C. Fredriksson, and I. S. Jawahir. 2019. “Process Sustainability Evaluation for Manufacturing of a Component with the 6R Application.” Procedia Manufacturing 33:546–553. https://doi.org/10.1016/j.promfg.2019.04.068.
- Butola, R., V. Sharma, S. Kanwar, L. Tyagi, M. Singari, and M. Tyagi. 2020. “Optimizing the Machining Variables in CNC Turning of Aluminum Based Hybrid Metal Matrix Composites.” SN Applied Sciences 2 (8): 1356. https://doi.org/10.1007/s42452-020-3155-8.
- Cai, W., K. Lai, C. Liu, F. Wei, M. Ma, S. Jia, Z. Jiang, and L. Lv. 2019. “Promoting Sustainability of Manufacturing Industry Through the Lean Energy-Saving and Emission-Reduction Strategy.” Science of the Total Environment 665:23–32. https://doi.org/10.1016/j.scitotenv.2019.02.069.
- Cai, W., L. Wang, L. Li, J. Xie, S. Jia, X. Zhang, Z. Jiang, and K. Lai. 2022. “A Review on Methods of Energy Performance Improvement Towards Sustainable Manufacturing from Perspectives of Energy Monitoring, Evaluation, Optimization and Benchmarking.” Renewable and Sustainable Energy Reviews 159:112227. https://doi.org/10.1016/j.rser.2022.112227.
- Camposeco-Negrete, C. 2021. “Analysis and Optimization of Sustainable Machining of AISI O1 Tool Steel by the Wire-EDM Process.” Advances in Manufacturing 9 (2): 304–317. https://doi.org/10.1007/s40436-021-00353-2.
- Cardoso, L. G., D. S. Madeira, T. E. P. A. Ricomini, R. A. Miranda, T. G. Brito, and E. J. Paiva. 2021. “Optimization of Machining Parameters Using Response Surface Methodology with Desirability Function in Turning Duplex Stainless Steel UNS S32760.” The International Journal of Advanced Manufacturing Technology 117 (5–6): 1633–1644. https://doi.org/10.1007/s00170-021-07690-3.
- Chate, G. R., M. P. G C, H. M. Harsha, S. U. Urankar, S. A. Sanadi, A. P. Jadhav, S. Hiremath, and A. S. Deshpande. 2021. “Sustainable Machining: Modelling and Optimization Using Taguchi, MOORA and DEAR Methods.” Materials Today: Proceedings 46:8941–8947. https://doi.org/10.1016/j.matpr.2021.05.365.
- Cheng, Y., X. Gai, R. Guan, Y. Jin, M. Lu, and Y. Ding. 2023. “Tool Wear Intelligent Monitoring Techniques in Cutting: A Review.” Journal of Mechanical Science and Technology 37 (1): 289–303. https://doi.org/10.1007/s12206-022-1229-9.
- Chou, C.-J., C.-W. Chen, and C. Conley. 2015. “An Approach to Assessing Sustainable Product-Service Systems.” Journal of Cleaner Production 86:277–284. https://doi.org/10.1016/j.jclepro.2014.08.059.
- Daniel, S. A. A., S. V. Ananth, A. Parthiban, and S. Sivaganesan. 2020. “Optimization of Machining Parameters in Electro Chemical Machining of Al5059/SiC/MoS2 Composites Using Taguchi Method.” Materials Today: Proceedings 21:738–743. https://doi.org/10.1016/j.matpr.2019.06.750.
- Daniyan, I. A., I. Tlhabadira, O. O. Daramola, and K. Mpofu. 2019. “Design and Optimization of Machining Parameters for Effective AISI P20 Removal Rate During Milling Operation.” Procedia CIRP 84:861–867. https://doi.org/10.1016/j.procir.2019.04.301.
- Delijaicov, S., G. C. Alves, É. C. Bordinassi, and E. J. D. Silva. 2023. “Optimization of Machining Parameters Applied in Hard Turning of Large Components for Cooling Lubrication Methods.” Proceedings of the Institution of Mechanical Engineers, Part E: Journal of Process Mechanical Engineering 237 (2): 580–588. https://doi.org/10.1177/09544089221116414.
- Department of Electrical Engineering, Jamia Millia Islamia, New Delhi, India, 110001, Singh, J., din, Z., & Department of Electrical Engineering, Jamia Millia Islamia, New Delhi, India, 110001. 2022. “Energy Efficient Data Aggregation and Density-Based Spatial Clustering of Applications with Noise for Activity Monitoring in Wireless Sensor Networks.” Engineered Science https://doi.org/10.30919/es8d694.
- Diao, Y., L. Yan, and K. Gao. 2022. “A Strategy Assisted Machine Learning to Process Multi-Objective Optimization for Improving Mechanical Properties of Carbon Steels.” Journal of Materials Science & Technology 109:86–93. https://doi.org/10.1016/j.jmst.2021.09.004.
- Dixit, N., V. Sharma, and P. Kumar. 2022. “Experimental Investigations into Ultrasonic Assisted Magnetic Abrasive Flow Machining Process.” Materials and Manufacturing Processes 38 (10): 1291–1306. https://doi.org/10.1080/10426914.2022.2146712.
- Dubravská, M., M. Marchevská, P. Vašaničová, and R. Kotulič. 2020. “Corporate Social Responsibility and Environmental Management Linkage: An Empirical Analysis of the Slovak Republic.” Sustainability 12 (13): 5431. https://doi.org/10.3390/su12135431.
- EEA indicators—European Environment Agency. (n.d.). [Page]. Accessed January 8, 2023, from https://www.eea.europa.eu/data-and-maps/indicators/about/eea-indicators.
- Eheliyagoda, D., J. Li, Y. Geng, and X. Zeng. 2022. “The Role of China’s Aluminum Recycling on Sustainable Resource and Emission Pathways.” Resources Policy 76:102552. https://doi.org/10.1016/j.resourpol.2022.102552.
- El-Kenawy, E.-S. M., S. Mirjalili, N. Khodadadi, A. A. Abdelhamid, M. M. Eid, M. El-Said, and A. Ibrahim. 2023. “Feature Selection in Wind Speed Forecasting Systems Based on Meta-Heuristic Optimization.” PLOS ONE 18 (2): e0278491. https://doi.org/10.1371/journal.pone.0278491.
- Errigo, A., J.-K. Choi, and K. Kissock. 2022. “Techno-Economic-Environmental Impacts of Industrial Energy Assessment: Sustainable Industrial Motor Systems of Small and Medium-Sized Enterprises.” Sustainable Energy Technologies and Assessments 49:101694. https://doi.org/10.1016/j.seta.2021.101694.
- Estoque, R. C., M. Ooba, X. T. Seposo, T. Togawa, Y. Hijioka, K. Takahashi, and S. Nakamura. 2020. “Heat Health Risk Assessment in Philippine Cities Using Remotely Sensed Data and Social-Ecological Indicators.” Nature Communications 11 (1): 1581. https://doi.org/10.1038/s41467-020-15218-8.
- Feng, C., H. Guo, J. Zhang, Y. Huang, and S. Huang. 2022. “A Systematic Method of Optimization of Machining Parameters Considering Energy Consumption, Machining Time, and Surface Roughness with Experimental Analysis.” The International Journal of Advanced Manufacturing Technology 119 (11–12): 7383–7401. https://doi.org/10.1007/s00170-022-08772-6.
- Gasparatos, A., and A. Scolobig. 2012. “Choosing the Most Appropriate Sustainability Assessment Tool.” Ecological Economics 80:1–7. https://doi.org/10.1016/j.ecolecon.2012.05.005.
- General Motors Metrics for Sustainable Manufacturing—LABORATORY FOR SUSTAINABLE BUSINESS. (n.d.). Accessed January 8, 2023, from https://www.readkong.com/page/general-motors-1822704.
- Gerten, D., V. Heck, J. Jägermeyr, and B. Bodirsky, I. Fetzer, M. Jalava, M. Kummu, W. Lucht, J. Rockström, S. Schaphoff, & H. Schellnhuber, (Ed.), 2019. Model Output For: “Feeding ten Billion People is Possible within Four Terrestrial Planetary boundaries” (P. 2 Files) [Application/octet-Stream, application/octet-Stream]. GFZ Data Services. https://doi.org/10.5880/PIK.2019.021
- Givehchi, M., A. Haghighi, and L. Wang. 2017. “Cloud-DPP for Distributed Process Planning of Mill-Turn Machining Operations.” Robotics and Computer-Integrated Manufacturing 47:76–84. https://doi.org/10.1016/j.rcim.2016.11.007.
- GRI - Standards. (n.d.). Accessed January 8, 2023, from https://www.globalreporting.org/standards/.
- Gunasekaran, A., and A. Spalanzani. 2012. “Sustainability of Manufacturing and Services: Investigations for Research and Applications.” International Journal of Production Economics 140 (1): 35–47. https://doi.org/10.1016/j.ijpe.2011.05.011.
- Gupta, M. K., Q. Song, Z. Liu, M. Sarikaya, M. Jamil, M. Mia, A. K. Singla, A. M. Khan, N. Khanna, and D. Y. Pimenov. 2021. “Environment and Economic Burden of Sustainable Cooling/Lubrication Methods in Machining of Inconel-800.” Journal of Cleaner Production 287:125074. https://doi.org/10.1016/j.jclepro.2020.125074.
- Hallstedt, S. I. 2017. “Sustainability Criteria and Sustainability Compliance Index for Decision Support in Product Development.” Journal of Cleaner Production 140:251–266. https://doi.org/10.1016/j.jclepro.2015.06.068.
- Hapuwatte, B., K. D. Seevers, F. Badurdeen, and I. S. Jawahir. 2016. “Total Life Cycle Sustainability Analysis of Additively Manufactured Products.” Procedia CIRP 48:376–381. https://doi.org/10.1016/j.procir.2016.03.016.
- Haq, M. A. U., S. Hussain, M. A. Ali, M. U. Farooq, N. A. Mufti, C. I. Pruncu, and A. Wasim. 2021. “Evaluating the Effects of Nano-Fluids Based MQL Milling of IN718 Associated to Sustainable Productions.” Journal of Cleaner Production 310:127463. https://doi.org/10.1016/j.jclepro.2021.127463.
- He, B., and K.-J. Bai. 2021. “Digital Twin-Based Sustainable Intelligent Manufacturing: A Review.” Advances in Manufacturing 9 (1): 1–21. https://doi.org/10.1007/s40436-020-00302-5.
- Hegab, H. A., B. Darras, and H. A. Kishawy. 2018. “Towards Sustainability Assessment of Machining Processes.” Journal of Cleaner Production 170:694–703. https://doi.org/10.1016/j.jclepro.2017.09.197.
- Herrmann, C., M. Hauschild, T. Gutowski, and R. Lifset. 2014. “Life Cycle Engineering and Sustainable Manufacturing: Life Cycle Engineering and Sustainable Manufacturing.” Journal of Industrial Ecology 18 (4): 471–477. https://doi.org/10.1111/jiec.12177.
- Horvath, R., G. Matyasi, and A. Dregelyi-Kiss. 2014. “Optimization of Machining Parameters for Fine Turning Operations Based on the Response Surface Method.” ANZIAM Journal 54:250. https://doi.org/10.21914/anziamj.v55i0.7865.
- Hu, L., R. Tang, W. Cai, Y. Feng, and X. Ma. 2019. “Optimisation of Cutting Parameters for Improving Energy Efficiency in Machining Process.” Robotics and Computer-Integrated Manufacturing 59:406–416. https://doi.org/10.1016/j.rcim.2019.04.015.
- Hu, L., R. Tang, K. He, and S. Jia. 2015. “Estimating Machining-Related Energy Consumption of Parts at the Design Phase Based on Feature Technology.” International Journal of Production Research 53 (23): 7016–7033. https://doi.org/10.1080/00207543.2014.944281.
- Huang, D. 2022. SVR Modeling and Parameter Optimization for Financial Time Series Forecasting. 2022 IEEE Conference on Telecommunications, Optics and Computer Science (TOCS), 1126–1130. https://doi.org/10.1109/TOCS56154.2022.10016054
- Imad, M., C. Hopkins, A. Hosseini, N. Z. Yussefian, and H. A. Kishawy. 2022. “Intelligent Machining: A Review of Trends, Achievements and Current Progress.” International Journal of Computer Integrated Manufacturing 35 (4–5): 359–387. https://doi.org/10.1080/0951192X.2021.1891573.
- Ingarao, G., G. Ambrogio, F. Gagliardi, and R. Di Lorenzo. 2012. “A Sustainability Point of View on Sheet Metal Forming Operations: Material Wasting and Energy Consumption in Incremental Forming and Stamping Processes.” Journal of Cleaner Production 29-30:255–268. https://doi.org/10.1016/j.jclepro.2012.01.012.
- Ishfaq, K., M. Rehman, and Y. Wang. 2023. “Toward the Targeted Material Removal with Optimized Surface Finish During EDM for the Repair Applications in Dies and Molds.” Arabian Journal for Science and Engineering 48 (3): 2653–2669. https://doi.org/10.1007/s13369-022-07006-x.
- Jamil, M., W. Zhao, N. He, M. K. Gupta, M. Sarikaya, A. M. Khan, S. Siengchin, and D. Y. Pimenov. 2021. “Sustainable Milling of Ti–6Al–4V: A Trade-Off Between Energy Efficiency, Carbon Emissions and Machining Characteristics Under MQL and Cryogenic Environment.” Journal of Cleaner Production 281:125374. https://doi.org/10.1016/j.jclepro.2020.125374.
- Jamwal, A., R. Agrawal, M. Sharma, and A. Giallanza. 2021. “Industry 4.0 Technologies for Manufacturing Sustainability: A Systematic Review and Future Research Directions.” Applied Sciences 11 (12): 5725. https://doi.org/10.3390/app11125725.
- Jayal, A. D., F. Badurdeen, O. W. Dillon, and I. S. Jawahir. 2010. “Sustainable Manufacturing: Modeling and Optimization Challenges at the Product, Process and System Levels.” CIRP Journal of Manufacturing Science and Technology 2 (3): 144–152. https://doi.org/10.1016/j.cirpj.2010.03.006.
- Jie, H., I. Khan, M. Alharthi, M. W. Zafar, and A. Saeed. 2023. “Sustainable Energy Policy, Socio-Economic Development, and Ecological Footprint: The Economic Significance of Natural Resources, Population Growth, and Industrial Development.” Utilities Policy 81:101490. https://doi.org/10.1016/j.jup.2023.101490.
- Kalateh, M. R., A. Kianifar, and M. Sardarabadi. 2022. “A Three-Dimensional Numerical Study of the Effects of Various Twisted Tapes on Heat Transfer Characteristics and Flow Field in a Tube: Experimental Validation and Multi-Objective Optimization via Response Surface Methodology.” Sustainable Energy Technologies and Assessments 50:101798. https://doi.org/10.1016/j.seta.2021.101798.
- Kam, M., A. İ̇pekçi, and K. Argun. 2022. “Experimental Investigation and Optimization of Machining Parameters of Deep Cryogenically Treated and Tempered Steels in Electrical Discharge Machining Process.” Proceedings of the Institution of Mechanical Engineers, Part E: Journal of Process Mechanical Engineering 236 (5): 1927–1935. https://doi.org/10.1177/09544089221078133.
- Kandavalli, S. R., A. M. Khan, A. Iqbal, M. Jamil, S. Abbas, R. A. Laghari, and Q. Cheok. 2023. “Application of Sophisticated Sensors to Advance the Monitoring of Machining Processes: Analysis and Holistic Review.” The International Journal of Advanced Manufacturing Technology 125 (3–4): 989–1014. https://doi.org/10.1007/s00170-022-10771-6.
- Kang, T. 2020. “BIM-Based Human Machine Interface (HMI) Framework for Energy Management.” Sustainability 12 (21): 8861. https://doi.org/10.3390/su12218861.
- Kechagias, J. D., K.-E. Aslani, N. A. Fountas, N. M. Vaxevanidis, and D. E. Manolakos. 2020. “A Comparative Investigation of Taguchi and Full Factorial Design for Machinability Prediction in Turning of a Titanium Alloy.” Measurement 151:107213. https://doi.org/10.1016/j.measurement.2019.107213.
- Khan, A. M., M. K. Gupta, H. Hegab, M. Jamil, M. Mia, N. He, Q. Song, Z. Liu, and C. I. Pruncu. 2020. “Energy-Based Cost Integrated Modelling and Sustainability Assessment of Al-GnP Hybrid Nanofluid Assisted Turning of AISI52100 Steel.” Journal of Cleaner Production 257:120502. https://doi.org/10.1016/j.jclepro.2020.120502.
- Kim, D. B., S.-J. Shin, G. Shao, and A. Brodsky. 2015. “A Decision-Guidance Framework for Sustainability Performance Analysis of Manufacturing Processes.” The International Journal of Advanced Manufacturing Technology 78 (9–12): 1455–1471. https://doi.org/10.1007/s00170-014-6711-9.
- Kim, S., G.-H. Kim, and Y.-D. Lee. 2013. “Sustainability Life Cycle Cost Analysis of Roof Waterproofing Methods Considering LCCO2.” Sustainability 6 (1): 158–174. https://doi.org/10.3390/su6010158.
- Kittali, P., V. Kalwa, D. Athith, K. P. Prashanth, and B. K. Venkatesh. 2022. “Optimization of Machining Parameters in Turning Operation to Minimize the Surface Roughness Using Taguchi Technique for EN1A Alloy Steel.” Materials Today: Proceedings 54:463–467. https://doi.org/10.1016/j.matpr.2021.10.323.
- Kosarac, A., C. Mladjenovic, M. Zeljkovic, S. Tabakovic, and M. Knezev. 2022. “Neural-Network-Based Approaches for Optimization of Machining Parameters Using Small Dataset.” Materials 15 (3): 700. https://doi.org/10.3390/ma15030700.
- Kouahla, I., M. A. Yallese, S. Belhadi, K. Safi, and M. Nouioua. 2022. “Tool Vibration, Surface Roughness, Cutting Power, and Productivity Assessment Using RSM and GRA Approach During Machining of Inconel 718 with PVD-Coated Carbide Tool.” The International Journal of Advanced Manufacturing Technology 122 (3–4): 1835–1856. https://doi.org/10.1007/s00170-022-09988-2.
- Kravchenko, M., D. C. Pigosso, and T. C. McAloone. 2019. “Towards the Ex-Ante Sustainability Screening of Circular Economy Initiatives in Manufacturing Companies: Consolidation of Leading Sustainability-Related Performance Indicators.” Journal of Cleaner Production 241:118318. https://doi.org/10.1016/j.jclepro.2019.118318.
- Kumar Debta, M., R. Mishra, and M. Masanta. 2020. “Experimental Investigation on the Machining Performance of AZ91D (90% Mg) Alloy by Wire-Cut EDM.” Materials Today: Proceedings 33:5557–5560. https://doi.org/10.1016/j.matpr.2020.03.540.
- Kumar, P., A. Chaudhary, A. Kuradiya, R. Mordia, and M. Bhandari. 2022. “Implementation of Sustainable Manufacturing in Production Systems: A Systematic Review of Past Decades Data.” Applied Ecology and Environmental Sciences 10 (7): 488–497. https://doi.org/10.12691/aees-10-7-7.
- Kumar Parida, A., and K. Maity. 2019. “Modeling of Machining Parameters Affecting Flank Wear and Surface Roughness in Hot Turning of Monel-400 Using Response Surface Methodology (RSM).” Measurement 137:375–381. https://doi.org/10.1016/j.measurement.2019.01.070.
- Kuntoğlu, M., E. Salur, M. K. Gupta, M. Sarıkaya, and D. Y. Pimenov. 2021. “A State-Of-The-Art Review on Sensors and Signal Processing Systems in Mechanical Machining Processes.” The International Journal of Advanced Manufacturing Technology 116 (9–10): 2711–2735. https://doi.org/10.1007/s00170-021-07425-4.
- Labuschagne, C., A. C. Brent, and R. P. G. van Erck. 2005. “Assessing the Sustainability Performances of Industries.” Journal of Cleaner Production 13 (4): 373–385. https://doi.org/10.1016/j.jclepro.2003.10.007.
- Lakshmana Kumar, S., M. Subburaj, A. Kulandaivel, P. Manoj Kumar, A. Thanikasalam, and R. Kumar. 2022. “Multiple response optimisation of machining parameters in turning nimonic C263 using TOPSIS approach.” Materials Today: Proceedings 59:1414–1419. https://doi.org/10.1016/j.matpr.2021.12.106.
- Lee, W. J., H. Wu, H. Yun, H. Kim, M. B. G. Jun, and J. W. Sutherland. 2019. “Predictive Maintenance of Machine Tool Systems Using Artificial Intelligence Techniques Applied to Machine Condition Data.” Procedia CIRP 80:506–511. https://doi.org/10.1016/j.procir.2018.12.019.
- Lee, Y., A. Resiga, S. Yi, and C. Wern. 2020. “The Optimization of Machining Parameters for Milling Operations by Using the Nelder–Mead Simplex Method.” Journal of Manufacturing and Materials Processing 4 (3): 66. https://doi.org/10.3390/jmmp4030066.
- Li, R., C. He, W. Xu, and X. Wang. 2023. “Modeling and Optimizing the Specific Cutting Energy of Medium Density Fiberboard During the Helical Up-Milling Process.” Wood Material Science & Engineering 18 (2): 464–471. https://doi.org/10.1080/17480272.2022.2049867.
- Li, Z., Y. Wang, K. Wang, and J. Li. 2019. “HDPS-BPSO Based Predictive Maintenance Scheduling for Backlash Error Compensation in a Machining Center.” In Advanced Manufacturing and Automation VIII, edited by K. Wang, Y. Wang, J. O. Strandhagen, and T. Yu, Vol. 484, 71–77. Springer Singapore. https://doi.org/10.1007/978-981-13-2375-1_11.
- Linke, B. S., G. J. Corman, D. A. Dornfeld, and S. Tönissen. 2013. “Sustainability Indicators for Discrete Manufacturing Processes Applied to Grinding Technology.” Journal of Manufacturing Systems 32 (4): 556–563. https://doi.org/10.1016/j.jmsy.2013.05.005.
- Lu, T., G. Rotella, S. C. Feng, F. Badurdeen, O. W. Dillon, K. Rouch, and I. S. Jawahir. 2012. “Metrics-Based Sustainability Assessment of a Drilling Process.” In Sustainable Manufacturing, edited by G. Seliger, 59–64. Berlin Heidelberg: Springer. https://doi.org/10.1007/978-3-642-27290-5_8.
- Mahesh, K., J. T. Philip, S. N. Joshi, and B. Kuriachen. 2021. “Machinability of Inconel 718: A Critical Review on the Impact of Cutting Temperatures.” Materials and Manufacturing Processes 36 (7): 753–791. https://doi.org/10.1080/10426914.2020.1843671.
- Mahfoud, S., A. Derouich, A. Iqbal, and N. El Ouanjli. 2022. “ANT-colony optimisation-direct torque control for a doubly fed induction motor: An experimental validation.” Energy Reports 8:81–98. https://doi.org/10.1016/j.egyr.2021.11.239.
- Maneiah, D., M. Shunmugasundaram, A. Raji Reddy, and Z. Begum. 2020. “Optimization of Machining Parameters for Surface Roughness During Abrasive Water Jet Machining of Aluminium/Magnesium Hybrid Metal Matrix Composites.” Materials Today: Proceedings 27:1293–1298. https://doi.org/10.1016/j.matpr.2020.02.264.
- Mani, M., J. Madan, J. H. Lee, K. W. Lyons, and S. K. Gupta. 2014. “Sustainability Characterisation for Manufacturing Processes.” International Journal of Production Research 52 (20): 5895–5912. https://doi.org/10.1080/00207543.2014.886788.
- Marakini, V., S. P. Pai, U. K. Bhat, D. T. Singh, and B. P. Achar. 2022. “High Speed Machining for Enhancing the AZ91 Magnesium Alloy Surface Characteristics Influence and Optimisation of Machining Parameters.” Defence Science Journal 72 (1): 105–113. https://doi.org/10.14429/dsj.72.17049.
- Mawson, V. J., and B. R. Hughes. 2020. “Thermal Modelling of Manufacturing Processes and HVAC Systems.” Energy 204:117984. https://doi.org/10.1016/j.energy.2020.117984.
- McAfee, M., M. Kariminejad, A. Weinert, S. Huq, J. D. Stigter, and D. Tormey. 2022. “State Estimators in Soft Sensing and Sensor Fusion for Sustainable Manufacturing.” Sustainability 14 (6): 3635. https://doi.org/10.3390/su14063635.
- Memić, B., A. Hasković Džubur, and E. Avdagić-Golub. 2022. “Green IoT: Sustainability environment and technologies.” Science, Engineering and Technology 2 (1): 24–29. https://doi.org/10.54327/set2022/v2.i1.25.
- Mercy, J. L., P. Sivashankari, M. Sangeetha, K. R. Kavitha, and S. Prakash. 2022. “Genetic Optimization of Machining Parameters Affecting Thrust Force During Drilling of Pineapple Fiber Composite Plates – an Experimental Approach.” Journal of Natural Fibers 19 (5): 1729–1740. https://doi.org/10.1080/15440478.2020.1788484.
- Mies, A., and S. Gold. 2021. “Mapping the Social Dimension of the Circular Economy.” Journal of Cleaner Production 321:128960. https://doi.org/10.1016/j.jclepro.2021.128960.
- Mittal, V. K., and K. S. Sangwan. 2014. “Prioritizing Barriers to Green Manufacturing: Environmental, Social and Economic Perspectives.” Procedia CIRP 17:559–564. https://doi.org/10.1016/j.procir.2014.01.075.
- Mohsin, M., A. K. Rasheed, H. Sun, J. Zhang, R. Iram, N. Iqbal, and Q. Abbas. 2019. “Developing Low Carbon Economies: An Aggregated Composite Index Based on Carbon Emissions.” Sustainable Energy Technologies and Assessments 35:365–374. https://doi.org/10.1016/j.seta.2019.08.003.
- Morkunas, M., and E. Rudienė. 2020. “The Impact of Social Servicescape Factors on Customers’ Satisfaction and Repurchase Intentions in Mid-Range Restaurants in Baltic States.” Journal of Open Innovation: Technology, Market, and Complexity 6 (3): 77. https://doi.org/10.3390/joitmc6030077.
- Nagalingam, A. P., B. L. Toh, and S. H. Yeo. 2023. “Surface Polishing of Laser Powder Bed Fused Inconel 625 Surfaces Using Multi-Jet Hydrodynamic Cavitation Abrasive Finishing: An Emerging Class of Cleaner Surface Enhancement Process.” International Journal of Precision Engineering and Manufacturing-Green Technology 10 (3): 637–657. https://doi.org/10.1007/s40684-022-00471-7.
- Nara, E. O. B., M. B. da Costa, I. C. Baierle, J. L. Schaefer, G. B. Benitez, L. M. A. L. Do Santos, and L. B. Benitez. 2021. “Expected Impact of Industry 4.0 Technologies on Sustainable Development: A Study in the Context of Brazil’s Plastic Industry.” Sustainable Production and Consumption 25:102–122. https://doi.org/10.1016/j.spc.2020.07.018.
- Nguyen, A.-T., V.-H. Nguyen, T.-T. Le, N.-T. Nguyen, and S. Ramesh. 2022. “Multiobjective Optimization of Surface Roughness and Tool Wear in High-Speed Milling of AA6061 by Machine Learning and NSGA-II.” Advances in Materials Science and Engineering 2022:1–21. https://doi.org/10.1155/2022/5406570.
- Nor, F. M., F. A. Jafar, A. Jamri, W. N. I. Wan Md Hatta, and M. H. Abu Bakar 2020. “PLC Based Automated Coolant Supply System for Machining AISI 304L in CNC Milling”. In Intelligent Manufacturing and Mechatronics, Eds., 569–579. Springer Singapore. https://doi.org/10.1007/978-981-13-9539-0_54
- Obaideen, K., N. Shehata, E. T. Sayed, M. A. Abdelkareem, M. S. Mahmoud, and A. G. Olabi. 2022. “The role of wastewater treatment in achieving sustainable development goals (SDGs) and sustainability guideline.” Energy Nexus 7:100112. https://doi.org/10.1016/j.nexus.2022.100112.
- Oláh, J., N. Aburumman, J. Popp, M. A. Khan, H. Haddad, and N. Kitukutha. 2020. “Impact of Industry 4.0 on Environmental Sustainability.” Sustainability 12 (11): 4674. https://doi.org/10.3390/su12114674.
- Olinto, A. C. 2014. “Vector Space Theory of Sustainability Assessment of Industrial Processes.” Clean Technologies and Environmental Policy 16 (8): 1815–1820. https://doi.org/10.1007/s10098-014-0729-4.
- Ortega Del Rosario, M. D. L. Á., K. Beermann, and M. Chen Austin. 2023. “Environmentally Responsive Materials for Building Envelopes: A Review on Manufacturing and Biomimicry-Based Approaches.” Biomimetics 8 (1): 52. https://doi.org/10.3390/biomimetics8010052.
- Padilla-Rivera, A., S. Russo-Garrido, and N. Merveille. 2020. “Addressing the Social Aspects of a Circular Economy: A Systematic Literature Review.” Sustainability 12 (19): 7912. https://doi.org/10.3390/su12197912.
- Pata, U. K. 2021. “Linking Renewable Energy, Globalization, Agriculture, CO2 Emissions and Ecological Footprint in BRIC Countries: A Sustainability Perspective.” Renewable Energy 173:197–208. https://doi.org/10.1016/j.renene.2021.03.125.
- Pathapalli, V. R., V. R. Basam, S. K. Gudimetta, and M. R. Koppula. 2019. “Optimization of Machining Parameters Using WASPAS and MOORA.” World Journal of Engineering 17 (2): 237–246. https://doi.org/10.1108/WJE-07-2019-0202.
- Paturi, U. M. R., A. Yash, S. Teja Palakurthy, and N. S. Reddy. 2022. “Modeling and Optimization of Machining Parameters for Minimizing Surface Roughness and Tool Wear During AISI 52100 Steel Dry Turning.” Materials Today: Proceedings 50:1164–1172. https://doi.org/10.1016/j.matpr.2021.08.047.
- Pawanr, S., G. Kant Garg, and S. Routroy. 2019. “Multi-Objective Optimization of Machining Parameters to Minimize Surface Roughness and Power Consumption Using TOPSIS.” Procedia CIRP 86:116–120. https://doi.org/10.1016/j.procir.2020.01.036.
- Payal, H., E. Fekadu, A. Bhattacharya, S. Priyadarshini, and A. Mishra. 2023. “Optimization of Machining Parameters in EDM of Inconel 625 Using Taguchi Approach.” Materials Today: Proceedings 80:S1422–1428. https://doi.org/10.1016/j.matpr.2023.01.223.
- Pérez-Fernández, L., M. A. Sebastián, and C. González-Gaya. 2022. “Methodology to Optimize Quality Costs in Manufacturing Based on Multi-Criteria Analysis and Lean Strategies.” Applied Sciences 12 (7): 3295. https://doi.org/10.3390/app12073295.
- Peter, A. P., K. S. Khoo, K. W. Chew, T. C. Ling, S.-H. Ho, J.-S. Chang, and P. L. Show. 2021. “Microalgae for Biofuels, Wastewater Treatment and Environmental Monitoring.” Environmental Chemistry Letters 19 (4): 2891–2904. https://doi.org/10.1007/s10311-021-01219-6.
- Pimenov, D. Y., M. Mia, M. K. Gupta, Á. R. Machado, G. Pintaude, D. R. Unune, N. Khanna, et al. 2022. “Resource Saving by Optimization and Machining Environments for Sustainable Manufacturing: A Review and Future Prospects.” Renewable and Sustainable Energy Reviews 166:112660. https://doi.org/10.1016/j.rser.2022.112660.
- Pinto, L. 2020. “Green Supply Chain Practices and Company Performance in Portuguese Manufacturing Sector.” Business Strategy and the Environment 29 (5): 1832–1849. https://doi.org/10.1002/bse.2471.
- Pinzon Amorocho, J. A., and T. Hartmann. 2022. “A Multi-Criteria Decision-Making Framework for Residential Building Renovation Using Pairwise Comparison and TOPSIS Methods.” Journal of Building Engineering 53:104596. https://doi.org/10.1016/j.jobe.2022.104596.
- Qiao, K., K. Yu, B. Qu, J. Liang, H. Song, and C. Yue. 2022. “An Evolutionary Multitasking Optimization Framework for Constrained Multiobjective Optimization Problems.” IEEE Transactions on Evolutionary Computation 26 (2): 263–277. https://doi.org/10.1109/TEVC.2022.3145582.
- Radha Krishnan, B., and M. Ramesh. 2020. “Optimization of Machining Process Parameters in CNC Turning Process of IS2062 E250 Steel Using Coated Carbide Cutting Tool.” Materials Today: Proceedings 21:346–350. https://doi.org/10.1016/j.matpr.2019.05.460.
- Rahmani, R., J. Karimi, P. R. Resende, J. C. C. Abrantes, and S. I. Lopes. 2023. “Overview of Selective Laser Melting for Industry 5.0: Toward Customizable, Sustainable, and Human-Centric Technologies.” Machines 11 (5): 522. https://doi.org/10.3390/machines11050522.
- Rajak, S., and S. Vinodh. 2015. “Application of Fuzzy Logic for Social Sustainability Performance Evaluation: A Case Study of an Indian Automotive Component Manufacturing Organization.” Journal of Cleaner Production 108:1184–1192. https://doi.org/10.1016/j.jclepro.2015.05.070.
- Rajemi, M. F., P. T. Mativenga, and A. Aramcharoen. 2010. “Sustainable Machining: Selection of Optimum Turning Conditions Based on Minimum Energy Considerations.” Journal of Cleaner Production 18 (10–11): 1059–1065. https://doi.org/10.1016/j.jclepro.2010.01.025.
- Ram Prasad, A. V. S., K. Ramji, M. Kolli, and G. Vamsi Krishna. 2019. “Multi-Response Optimization of Machining Process Parameters for Wire Electrical Discharge Machining of Lead-Induced Ti-6Al-4V Alloy Using AHP–TOPSIS Method.” Journal of Advanced Manufacturing Systems 18 (2): 213–236. https://doi.org/10.1142/S0219686719500112.
- “Report of the World Commission on Environment and Development.” n.d.-a. Accessed March 14, 2023 from https://digitallibrary.un.org/record/139811?ln=en.
- Roinioti, A., and C. Koroneos. 2019. “Integrated Life Cycle Sustainability Assessment of the Greek Interconnected Electricity System.” Sustainable Energy Technologies and Assessments 32:29–46. https://doi.org/10.1016/j.seta.2019.01.003.
- Roslan, M. F., M. A. Hannan, P. J. Ker, M. Mannan, K. M. Muttaqi, and T. I. Mahlia. 2022. “Microgrid Control Methods Toward Achieving Sustainable Energy Management: A Bibliometric Analysis for Future Directions.” Journal of Cleaner Production 348:131340. https://doi.org/10.1016/j.jclepro.2022.131340.
- Saad, M. H., M. A. Nazzal, and B. M. Darras. 2019. “A General Framework for Sustainability Assessment of Manufacturing Processes.” Ecological Indicators 97:211–224. https://doi.org/10.1016/j.ecolind.2018.09.062.
- Sahoo, A. K., S. K. Sahoo, S. Pattanayak, and M. K. Moharana. 2023. “Ultrasonic Vibration Assisted Turning of Inconel 825: An Experimental Analysis.” Materials and Manufacturing Processes 38 (12): 1600–1614. https://doi.org/10.1080/10426914.2023.2165675.
- Salvado, M., S. Azevedo, J. Matias, and L. Ferreira. 2015. “Proposal of a Sustainability Index for the Automotive Industry.” Sustainability 7 (2): 2113–2144. https://doi.org/10.3390/su7022113.
- Sangwan, K. S., and V. K. Mittal. 2015. “A Bibliometric Analysis of Green Manufacturing and Similar Frameworks.” Management of Environmental Quality: An International Journal 26 (4): 566–587. https://doi.org/10.1108/MEQ-02-2014-0020.
- Sanjeevi, R., G. A. Kumar, and B. R. Krishnan. 2021. “Optimization of Machining Parameters in Plane Surface Grinding Process by Response Surface Methodology.” Materials Today: Proceedings 37:85–87. https://doi.org/10.1016/j.matpr.2020.04.075.
- Sartal, A., R. Bellas, A. M. Mejías, and A. García-Collado. 2020. “The Sustainable Manufacturing Concept, Evolution and Opportunities within Industry 4.0: A Literature Review.” Advances in Mechanical Engineering 12 (5): 168781402092523. https://doi.org/10.1177/1687814020925232.
- Saxena, P., P. Stavropoulos, J. Kechagias, and K. Salonitis. 2020. “Sustainability Assessment for Manufacturing Operations.” Energies 13 (11): 2730. https://doi.org/10.3390/en13112730.
- Schaltegger, S., M. Bennett, and R. Burritt (Eds.). (2006). Sustainability Accounting and Reporting. Springer.
- Schmidt, W.-P. 2006. “Life Cycle Tools within Ford of Europe’s Product Sustainability Index. Case Study Ford S-MAX & Ford Galaxy (8 Pp).” The International Journal of Life Cycle Assessment 11 (5): 315–322. https://doi.org/10.1065/lca2006.08.267.
- Senthilkumar, K. M., R. Thirumalai, T. A. Selvam, A. Natarajan, and T. Ganesan. 2021. “Multi Objective Optimization in Machining of Inconel 718 Using Taguchi Method.” Materials Today: Proceedings 37:3466–3470. https://doi.org/10.1016/j.matpr.2020.09.333.
- Shah, D. R., N. Pancholi, H. Gajera, and B. Patel. 2022. “Investigation of Cutting Temperature, Cutting Force and Surface Roughness Using Multi-Objective Optimization for Turning of Ti-6Al-4 V (ELI).” Materials Today: Proceedings 50:1379–1388. https://doi.org/10.1016/j.matpr.2021.08.285.
- Shandilya, P., A. K. Rouniyar, and D. Saikiran. 2020. “Multi-Objective Parametric Optimization on Machining of Inconel-825 Using Wire Electrical Discharge Machining.” Proceedings of the Institution of Mechanical Engineers, Part C: Journal of Mechanical Engineering Science 234 (20): 4056–4068. https://doi.org/10.1177/0954406220917706.
- Sharma, R. C., V. Dabra, G. Singh, R. Kumar, R. P. Singh, and S. Sharma. 2022. “Multi-Response Optimization While Machining of Stainless Steel 316L Using Intelligent Approach of Grey Theory and Grey-TLBO.” World Journal of Engineering 19 (3): 329–339. https://doi.org/10.1108/WJE-06-2020-0226.
- Sharma, S., U. K. Vates, and A. Bansal. 2021. “Optimization of Machining Characteristics for EDM of Different Nickel-Based Alloys by Embodying of Fuzzy, Grey Relational and Taguchi Technique.” World Journal of Engineering 18 (1): 23–36. https://doi.org/10.1108/WJE-07-2020-0262.
- Simpson, A., and A. Tamayo. 2020. “Real Effects of Financial Reporting and Disclosure on Innovation.” Accounting and Business Research 50 (5): 401–421. https://doi.org/10.1080/00014788.2020.1770926.
- Singh, A. K., A. Kumar, V. Sharma, and P. Kala. 2020. “Sustainable Techniques in Grinding: State of the Art Review.” Journal of Cleaner Production 269:121876. https://doi.org/10.1016/j.jclepro.2020.121876.
- Singh, R. K., H. R. Murty, S. K. Gupta, and A. K. Dikshit. 2009. “An Overview of Sustainability Assessment Methodologies.” Ecological Indicators 9 (2): 189–212. https://doi.org/10.1016/j.ecolind.2008.05.011.
- Singh Sivam, S. P. S., R. Rajendran, and N. Harshavardhana. 2022. “An Investigation of Hybrid Models FEA Coupled with AHP-ELECTRE, RSM-GA, and ANN-GA into the Process Parameter Optimization of High-Quality Deep-Drawn Cylindrical Copper Cups.” Mechanics Based Design of Structures and Machines 1–25. https://doi.org/10.1080/15397734.2022.2120497.
- Sivarupan, T., N. Balasubramani, P. Saxena, D. Nagarajan, M. El Mansori, K. Salonitis, M. Jolly, and M. S. Dargusch. 2021. “A Review on the Progress and Challenges of Binder Jet 3D Printing of Sand Moulds for Advanced Casting.” Additive Manufacturing 40:101889. https://doi.org/10.1016/j.addma.2021.101889.
- Somasundaram, M., J. Pradeep Kumar, and M. Mathan. 2020. “Investigation on Surface Integrity, Machining and Form Tolerance Characteristics Using TOPSIS Approach for Electrical Discharge Machining of Biocompatible Material.” Materials Today: Proceedings 22:1853–1860. https://doi.org/10.1016/j.matpr.2020.03.020.
- Soori, M., and B. Arezoo. 2023. “Minimization of Surface Roughness and Residual Stress in Abrasive Water Jet Cutting of Titanium Alloy Ti6Al4V.” Proceedings of the Institution of Mechanical Engineers, Part E: Journal of Process Mechanical Engineering 095440892311579. https://doi.org/10.1177/09544089231157972.
- Spiering, T., S. Kohlitz, H. Sundmaeker, and C. Herrmann. 2015. “Energy Efficiency Benchmarking for Injection Moulding Processes.” Robotics and Computer-Integrated Manufacturing 36:45–59. https://doi.org/10.1016/j.rcim.2014.12.010.
- Sproesser, G., S. Schenker, A. Pittner, R. Borndörfer, M. Rethmeier, Y.-J. Chang, and M. Finkbeiner. 2016. “Sustainable Welding Process Selection Based on Weight Space Partitions.” Procedia CIRP 40:127–132. https://doi.org/10.1016/j.procir.2016.01.077.
- Tracking Industrial Energy Efficiency and CO2 Emissions – Analysis—IEA. (n.d.-b.). Accessed March 15, 2023, from https://www.iea.org/reports/tracking-industrial-energy-efficiency-and-co2-emissions
- Transforming our World: The 2030 Agenda for Sustainable Development .:. Sustainable Development Knowledge Platform. (n.d.). Accessed March 14, 2023, from https://sustainabledevelopment.un.org/post2015/transformingourworld/publication
- Vats, P., T. Singh, V. Dubey, and A. Kumar Sharma. 2022. “Optimization of Machining Parameters in Turning of AISI 1040 Steel Using Hybrid MCDM Technique.” Materials Today: Proceedings 50:1758–1765. https://doi.org/10.1016/j.matpr.2021.09.188.
- Verl, A., A. Valente, S. Melkote, C. Brecher, E. Ozturk, and L. T. Tunc. 2019. “Robots in Machining.” CIRP Annals 68 (2): 799–822. https://doi.org/10.1016/j.cirp.2019.05.009.
- Vishnu, V. S., K. G. Varghese, and B. Gurumoorthy. 2023. “A Data-Driven Digital Twin Framework for Key Performance Indicators in CNC Machining Processes.” International Journal of Computer Integrated Manufacturing 1–19. https://doi.org/10.1080/0951192X.2023.2177741.
- Welford, R., Ed. 2016. Corporate Environmental Management 1: Systems and Strategies. 0 ed. Routledge. https://doi.org/10.4324/9781315825120.
- Wong, C. 2014. “Sustainable Development Indicators.” In Encyclopedia of Quality of Life and Well-Being Research, edited by A. C. Michalos, 6504–6507. Springer Netherlands. https://doi.org/10.1007/978-94-007-0753-5_2948.
- Wu, T.-Y., and C.-C. Lin. 2021. “Optimization of Machining Parameters in Milling Process of Inconel 718 under Surface Roughness Constraints.” Applied Sciences 11 (5): 2137. https://doi.org/10.3390/app11052137.
- Wu, Y.-F., S. M. S. Kazmi, M. J. Munir, Y. Zhou, and F. Xing. 2020. “Effect of Compression Casting Method on the Compressive Strength, Elastic Modulus and Microstructure of Rubber Concrete.” Journal of Cleaner Production 264:121746. https://doi.org/10.1016/j.jclepro.2020.121746.
- Xiao, G., Y. He, Y. Huang, Y. Liu, W. Dai, and L. Quan. 2019. “Integration of Heterogeneous System Information in Machining-Detection Logistics for Titanium Alloy Blade with Adaptive Belt Grinding.” International Journal of Computer Integrated Manufacturing 32 (9): 900–917. https://doi.org/10.1080/0951192X.2019.1644479.
- Xie, S., Z. He, C. Wang, C. Liu, and X. Ke. 2023. “A Generic Evolutionary Ensemble Learning Framework for Surface Roughness Prediction in Manufacturing.” International Journal of Computer Integrated Manufacturing 36 (11): 1572–1594. https://doi.org/10.1080/0951192X.2023.2204486.
- Yadegaridehkordi, E., M. Hourmand, M. Nilashi, E. Alsolami, S. Samad, M. Mahmoud, A. A. Alarood, A. Zainol, H. D. Majeed, and L. Shuib. 2020. “Assessment of Sustainability Indicators for Green Building Manufacturing Using Fuzzy Multi-Criteria Decision Making Approach.” Journal of Cleaner Production 277:122905. https://doi.org/10.1016/j.jclepro.2020.122905.
- Yaka, H. 2021. “MEASUREMENT OF SURFACE QUALITY AND OPTIMIZATION OF CUTTING PARAMETERS IN SLOT MILLING OF GFRP COMPOSITE MATERIALS WITH DIFFERENT FIBER RATIOS PRODUCED BY PULTRUSION METHOD.” Surface Review and Letters 28 (10): 2150095. https://doi.org/10.1142/S0218625X21500955.
- Yale Center For Environmental Law And Policy-YCELP-Yale University, Center For International Earth Science Information Network-CIESIN-Columbia University, & World Economic Forum-WEF. 2006. Pilot 2006 Environmental Performance Index (EPI) [ Data set]. Palisades, NY:NASA Socioeconomic Data and Applications Center (SEDAC). https://doi.org/10.7927/H44M92GX
- Yan, X., S. Dong, X. Li, Z. Zhao, S. Dong, and L. An. 2021. “Optimization of Machining Parameters for Milling Zirconia Ceramics by Polycrystalline Diamond Tool.” Materials 15 (1): 208. https://doi.org/10.3390/ma15010208.
- Ye, W., W. Shen, Z. Qian, and J. Zheng. 2022. “Robust Longitudinal Motion Control of Underground Mining Electric Vehicles Based on Fuzzy Parameter Tuning Sliding Mode Controller.” Computers & Electrical Engineering 98:107683. https://doi.org/10.1016/j.compeleceng.2022.107683.
- Zamfirache, I. A., R.-E. Precup, R.-C. Roman, and E. M. Petriu. 2022. “Reinforcement Learning-Based Control Using Q-Learning and Gravitational Search Algorithm with Experimental Validation on a Nonlinear Servo System.” Information Sciences 583:99–120. https://doi.org/10.1016/j.ins.2021.10.070.
- Zarrouk, T., M. Nouari, J.-E. Salhi, H. Makich, M. Salhi, S. Atlati, and N. Salhi. 2022. “Optimization of the Milling Process for Aluminum Honeycomb Structures.” The International Journal of Advanced Manufacturing Technology 119 (7–8): 4733–4744. https://doi.org/10.1007/s00170-021-08495-0.
- Zhou, G., Q. Lu, Z. Xiao, C. Zhou, and C. Tian. 2019. “Cutting Parameter Optimization for Machining Operations Considering Carbon Emissions.” Journal of Cleaner Production 208:937–950. https://doi.org/10.1016/j.jclepro.2018.10.191.