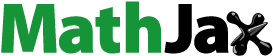
ABSTRACT
A substantial amount of wastewater is generated during the extraction of oil and gas. Wastewater that is generated as a result of oil and gas extraction activities is known as produced water (PW), and managing PW is one of the greatest challenges faced by the oil and gas industry. Effective management of PW requires an understanding of the complex impact it may have on both oil production and water treatment operations. Here, a numerical model has been applied to a real-world case study, that of Kuwait Oil Company (KOC), to examine the relationships among various input and output factors in PW supply chain management. Specifically, the numerical model is a multi-objective optimisation model for the management of PW supply chains that takes into account economic as well as environmental objectives. A global sensitivity analysis has also been performed to assess the economic and operational factors that can influence the management of PW in KOC. The findings of this study show that the operational costs, treatment costs and environmental impacts can be assessed and used to guide the management of the PW supply chain in KOC.
1. Introduction
Due to the world’s growing energy needs and consumption, demand for crude oil is rapidly increasing and the oil and gas industry is continuously increasing production to supply hydrocarbon fluids and gas to the market (Nashawi, Malallah, and Al-Bisharah Citation2010). During oil and gas extraction, large amounts of wastewater are generated, with an estimated global production surpassing oil production at a volumetric ratio of 2:4. This includes the water originally present in the reservoirs, as well as the water injected into the wells (Salem and Thiemann Citation2022). This wastewater is known as produced water (PW) and also referred to as brine and salt water in the oil industry (Alkhudhiri, Darwish, and Hilal Citation2013). PW contains a high level of total dissolved solids (TDS) that can reach concentrations of up to 400,000 mg/L, as well as a number of toxic organic and inorganic compounds (Jiménez et al. Citation2018). . summarises the main components of PW.
Table 1. The content of oilfield-produced water (Ahmad et al. Citation2020; Al-Ghouti et al. Citation2019; Clark and Veil Citation2009; Dudek et al. Citation2020; Fakhru’l-Razi et al. Citation2009; Jiménez et al. Citation2018; Salem and Thiemann Citation2022.).
There are many factors that influence the composition of PW, such as geological formation, lifecycle, and type reservoir of hydrocarbon produced (Simões et al. Citation2020). Additionally, the composition of PW depends on whether it is associated with the production of oil, gas, or coal (Coha et al. Citation2021).
Considering that PW 1) is the largest by-product or waste stream associated with petroleum production, 2) treatment methods can be costly, 3) can have environmental impacts, managing PW is a challenging undertaking (Simões et al. Citation2020). In addition, the treatment and management of PW directly affects the cost drivers for oil producers (Karapataki Citation2012). Recent estimates suggest that the worldwide market for PW treatment will reach USD 8.1 billion in 2020, with an expected compound annual growth rate of 4.8% between 2020 and 2027 (Dawoud et al. Citation2021).
Hence, in the oil and gas industry, there is a vital need to focus more attention on the treatment, utilisation and management of PW. It is necessary to consider specific technical, economic, environmental, and regulatory factors prior to using PW for onsite operations or other beneficial purposes (Hernández Citation2021). Therefore, it is essential that effective management be developed in order to enable the reuse of PW in a responsible manner (Liden et al. Citation2018). Clearly, utilising and managing PW involves understanding and addressing a range of issues, such as PW quality, quantity and transportation (Goodwin Citation2014).
1.1. Utilisation of produced water
Utilisation, or what is referred to as ‘beneficial reuse’, refers to using PW for an alternative purpose By reusing PW, the water cycle for drilling and fracturing operations can be closed, alleviating the strain on freshwater withdrawals, reducing water transportation activities, and reducing pollution (Conrad et al. Citation2020; Echchelh, Hess, and Sakrabani Citation2018; Entrekin et al. Citation2018).
The management of PW by internal re-use (i.e. reinjection into the source oil zone) or disposal is considered a feasible, environmentally acceptable alternative to the disposal of PW into the sea or in desert evaporation ponds, which poses serious environmental risks.
Due to the global water shortage, the large quantities of PW represent potential water sources. However, when selecting specific management schemes, it is necessary to consider both the quality and quantity of PW. In order for PW to be effectively managed, it is critical to choose the right treatment method and disposal method. If improperly handled, PW can cause damage to seawater, aquifer water, soil, aquatic animals and plants, the atmosphere, and even human health
PW management strategies are aimed at minimising environmental impacts and costs. The separation process is typically gravity-based, and the treated PW is discharged into the environment from offshore platforms or injected underground for production enhancement or disposed of directly from onshore platforms, respectively. Using PW in other applications such as industrial applications requires that it be treated according to the requirements of the end user (Erfani, Mokhtar, and Erfani Citation2018; Fakhru’l-Razi et al. Citation2009; Jiménez et al. Citation2018; Saad, Rayes, and Pactor Citation2016).
The treatment method can be determined once the requirements have been identified (Jiménez et al. Citation2018). Concentrations of each component are also important considerations and can result in significant increases in treatment costs (Scanlon et al. Citation2020). The levels of PW treatment required must also be considered (e.g. primary, secondary, and tertiary levels), as well as the desired use of treated PW (Nijhawan and Myers Citation2006).
1.2. Kuwait oil company – the case study
Kuwait is renowned for its extensive production of hydrocarbon resources and significant crude oil reserves. As a member of the Organisation of the Petroleum Exporting Countries (OPEC), the nation holds the sixth-largest oil reserves globally, totalling approximately 102 billion barrels. This accounts for about 8.2% of the world’s reserves (U.S. Energy Information Administration Citation2016). Kuwait is reliant on hydrocarbon products for its energy needs, including crude oil, natural gas, and fuel oil. Almost all of Kuwait’s government income is generated through petroleum exports, which account for a significant portion of the country’s economy. It is estimated that petroleum exports accounted for nearly 89% of Kuwait’s total export revenues (Siderius et al. Citation2020).
It is estimated that Kuwait has the world’s lowest level of renewable freshwater resources, with a total renewable water supply of less than 5 m3 per capita per-year (Siderius et al. Citation2019). With the impact of climate change, rapid growth of the population and increasing urbanisation and agriculture, the demand and the availability of freshwater resources in the country is becoming a problem (Freyman Citation2014). A high rate of oil production in Kuwaiti oil fields is expected in the near future, as well as a significant increase in investment in the development of oil and gas production facilities (Alsayegh Citation2021). As Kuwait’s oil fields contain approximately 40% water, Kuwait Oil Company (KOC) produces approximately 2 million barrels of PW per day, compared to approximately 3 million barrels of oil per day. The increasing amount of PW in Kuwait’s oil fields has presented KOC with the challenge of managing the PW supply chain as the rest of the world (AlAnezi et al. Citation2018).
Kuwait relies heavily on aquifers and desalination for its water supply, therefore, PW could be an important water source, at least as an irrigation source, and it should not be underutilised. The reuse of PW could reduce pollution to the environment while also reducing the need to supply fresh water to the population (Alhumoud, Behbehani, and Abdullah Citation2003). Therefore, in Kuwait, control and reuse of treated wastewater is becoming mandatory to meet the population demand and the local water shortage (Al-Jarallah Citation2013; Salem and Thiemann Citation2022). A high oil production rate is expected from Kuwait Oil Company (KOC) in the near future (Al-Abdullah et al. Citation2019; Alsayegh Citation2021). Due to the high amount of PW associated with oil production in KOC, managing this waste is becoming increasingly difficult (AlAnezi et al. Citation2018).
This study is conducted to determine how to best manage PW in the oil field of KOC. As part of this model, the two different objectives: the economic objective function seeks to minimise the overall cost, while the environmental objective function seeks to minimise the emissions throughout the PW supply chain.
The model comprises a supply chain system consisting of crude oil gathering centres, treatment facilities, and disposal and reinjection wells and a water reuse site to generate the most related realistic and practical system (Aledan and Erfani Citation2023).
1.3. Global sensitivity analysis – the validation method
Various uncertainties are involved in the development of modern models in physics, chemistry, environments, economics, risk analysis and other fields (Cheng, Lu, and Zhang Citation2019; Erfani and Erfani Citation2015a). We further investigate how the variations in inputs could impact the outputs of our model. For this, we perform global sensitivity analysis (GSA) to obtain more representative results from the optimisation model. GSA is one of the methods that continues to play an important role in the fields of research that are data-rich. Also, GSA is a mathematical technique that has become increasingly important in the development of environmental models and assessments over the past decade (Ye and Hill Citation2017). The aim of this paper is to evaluate the input parameters (via GSA) and, identify the most effective input parameter.
Moreover, GSA is employed for several purposes, including to validate model parameters and diagnostic evaluation, for dominant control analysis and to support robust decision-making (Y. Wang, Huang, and Tang Citation2020). Also, application of GSA to optimisation models provides insights into the variables that have the greatest influence on their outcomes (M. Wang et al. Citation2013, Erfani and Erfani, Citation2015b).
Similar studies have used the GSA to provide results that may be used by decision makers to reduce the uncertainty in the prediction of system performance (Sin et al. Citation2011). This results in a better understanding of the model behaviour and, thus, better estimates and reduced uncertainty (Wagener and Pianosi Citation2019). A more detailed explanation of GSA is provided under the methodology section of this document.
2. Problem definition
A large amount of PW is generated during the production of oil and gas from wells in Kuwait (Salem and Thiemann Citation2022). The increased production of PW has a variety of impacts on the field, and specific concerns have been raised regarding the treatment capabilities, the transportation methods, the limited capacity of water-handling facilities and the high costs associated with building more water-handling facilities (Al-Ballam et al. Citation2018; Hale et al., Citation2010). Furthermore, due to the high salinity of the PW in KOC fields, it is difficult to treat PW in the area given the limited options available (Salem and Thiemann Citation2022). Thus, KOC will be required to take significant measures in the coming years to effectively manage the anticipated increase in PW production, injection and disposal operations (Ali et al. Citation2013).
3. Methodology
In this study, a mathematical model of multi-objective mixed integer linear programming (MO-MILP) is used based on a case study in Kuwait oil company. The application of the MO-MILP forms the basis of the analysis. The mathematical optimisation technique is used to optimise the trade-off between conflicting objectives, focusing on economic costs and environmental impacts over a five-, ten-, and twenty-year period. By examining these two conflicting criteria, we can obtain a solution that strikes a balance between economic feasibility and environmental sustainability. This method has the advantage of finding the best solution under linear constraints while simultaneously optimising multiple conflicting objectives (Jabarzadeh et al. Citation2020). It is the nature of multi-objective mathematical programming that conflicting objectives must be optimised simultaneously, and no one optimal solution can achieve all objectives at the same time (Cui et al. Citation2017, Erfani et al. Citation2020).
Based on the mathematical optimisation model established to optimise the PW supply chain in real systems related to KOC by Aledan and Erfani (Citation2023), the GSA studies presented in this paper were conducted. GSA is a mathematical technique that has become increasingly important in the development of mathematical models and assessments over the past decade (Ye and Hill Citation2017). GSA is applied to understand the implications of uncertainty and environmental variability on the identification of different system models (Wagener and Pianosi Citation2019). Also, GSA is employed to assess the influence of input variables on model outputs, enhancing the robustness of the findings and recommendations. GSA is performed in this study to obtain more representative results about PW supply chain management.
We focus on constraints (inputs) related to PW quantity, operational costs and environmental impacts. We have also examined the main opportunities that arise from efficient and appropriate PW utilisation.
In this study, when conducting the GSA, four independent parameters are taken into account including discount rate, treatment cost, electricity cost and water capacity (amount of PW that can be treated). Multiple linear regression (MLR) has been used to conduct a GSA in which the influence of different PW supply chain parameters on the total operations cost and environmental impact are assessed. Moreover, MLR has been used to assess the impacts of the model’s inputs on each of its outputs (Pianosi et al. Citation2016; Sin et al. Citation2011).
Discount rates are carefully considered in the oil and gas sector, where investment cycles are long and project timelines reach 20–30 years. Several academic studies have demonstrated the importance of accurately determining the discount rate over long planning periods for projects (Komzolov et al. Citation2021Komzolov et al. Citation2021). The use of discount rates in the oil and gas sector allows decision makers to assess the change in the value of expenses over the course of several years (Harden Citation2014). Costs associated with PW treatment are also important, as large volumes of oil production wastewater are incapable of being discharged directly into the environment (Katz, Bowman, and S Citation2003). The development of a cost-effective treatment process is therefore essential (Liden et al. Citation2018). The cost of PW treatment is closely related to the price of electricity and the quality of the PW (Al-Ghouti et al. Citation2019).
In experiments that require multiple parameters to be analysed, a factorial design is commonly used (Hribernik, Bauman, and Lobnik Citation2009Hribernik, Bauman, and Lobnik Citation2009). In practice, the 2k factorial design is considered for implementing the GSA in our analysis. This is because it can be used to investigate multiple parameters at once (Lu Citation2016). Therefore, the GSA conducted in this study utilised a 2k factorial design. A total of 48 simulations are performed for four parameters over 5-, 10- and 20-year periods.
Multiple linear regression (MLR) has been used to conduct a GSA in which the influence of different PW supply chain parameters on the total operations cost and environmental impact are assessed. Moreover, MLR has been used to assess the impacts of the model’s inputs on each of its outputs (Pianosi et al. Citation2016; Sin et al. Citation2011).
The results of the optimisation model are expressed in terms of the MLR model and represented by EquationEquation (1)(1)
(1) , as follows:
where y is the dependent parameters representing different impacts including transportation, treatment, maintenance, reuse and electricity costs, as well as CO2 eq from chemical additives and electricity usage. A vector of independent parameters, including discount rate, treatment expenditure, electricity expenditures and water capacity is represented by β_n. Interactions between these parameters are considered in EquationEquation (1)(1)
(1) , such as x1×2. Here, the regression coefficient of each independent parameter reflecting the influence of each independent parameter on the dependent parameter. C is the intercept of the equation, and reflects external variables not considered in the model. To verify the validity of the optimisation model results, the robustness of dependent values is evaluated. outlined the case study flowchart modelling process.
Furthermore, there are several research gaps that exist, indicating areas where further investigation is needed. Here are a few key research gaps in the field of PW:
Environmental impact: The environmental impacts of PW are acknowledged in this study; however, further research is required to gain a comprehensive understanding of such impacts.
Water reuse and recycling: There is a lack of understanding of the potential risks, technical challenges, and economic feasibility of large-scale PW reuse projects.
Standards and regulations: For safe and sustainable reuse of PW, further research may focus on developing guidelines, treatment standards, and risk assessment frameworks.
Socioeconomic and policy aspects: PW management has received relatively little attention in terms of socioeconomic and policy dimensions. It is important to conduct more that explore the social and economic impacts of PW on local communities.
3.1. Data collection
To verify the validity of our optimisation model results, we evaluate the robustness of dependent values. Four independent parameters we considered when conducting the MLR analysis:
Discount Rate.
Treatment Cost.
Electricity Cost.
Water Capacity (amount of pw that can be treated).
The variations in the data obtained for each independent parameter are shown in .
Table 2. The independent parameters that resulted from the 2k factorial design, bounded by lower and upper level (Alzahrani and Mohammad Citation2014; Elaila et al. Citation2017; Eltony Citation2008; Kajenthira, Siddiqi, and Anadon Citation2012; Nayar et al. Citation2019; Rodriguez Citation2019).
However, data access to comprehensive and reliable information on PW characteristics, volumes, and treatment technologies may be limited, as well as availability and quality of information may vary based on industry, region, and regulatory requirements. In addition, economic data, such as energy prices and treatment cost, as well as environmental indicators, such as emissions may be limited or vary across different sources. These limitations can affect the accuracy and reliability of the model outputs and the subsequent analysis of trade-offs.
We collected data on the tested independent parameters from existing case studies that had been conducted under similar conditions to our case study. More information about the tested independent parameters from exiting case studies are available in the supplementary file. Some of economic and environmental data needed for the mathematical model were collected from Aledan and Erfani (Citation2023) (Aledan and Erfani Citation2023) study of the trading-off the economic cost and environmental impact in Kuwait oil company and can be found in the supplementary file section 1 under ‘Case study details’ including Figure S1 and Table S1. Other economic and figures needed for the GSA model were collected from different sources that can be found section 2 under ‘GSA Data’ including Table S2 and Table S3. Section 3 is intended for ‘Other data’.
The description of the dependent parameters used in the analysis is given below, we use short terms instead of full names of the dependent parameters in our whole analysis.
4. Results and discussion
This paper has examined PW supply chain modal sensitivity against different independent parameters including discount rate, treatment and electricity prices and water capacity. Using a case study of an oil and gas company in Kuwait, GSA method is used to estimate the modal sensitivity. Modal sensitivity can provide insight into the extent to which PW supply chain changes may have an economic and ecological impact. Based on these results, we are able to determine the importance and contribution of each of the selected independent parameters in our model. Furthermore, the results demonstrate the effect of each independent parameter on each dependent parameter in the PW supply chain model.
Figure 1. Flowchart of the modelling process used in this study.

Since the independent parameters have different units of measure, we converted the values to dimensionless values by normalising them to values between 0 and 1. Then, the MLR results obtained for the different periods (5, 10 and 20 years) could be used to illustrates the effects of the different independent parameters on the dependent parameters. The data resulting from the GSA conducted for the 5-, 10- and 20-year periods are presented in respectively.
Figure 2. Effect of independent parameters on dependent parameters: 5-year periods.

Figure 3. Effect of independent parameters on dependent parameters:10-year periods.

Figure 4. Effect of independent parameters on dependent parameters:20-year periods.

The independent parameters are listed in the first row, and the dependent parameters are listed in the first column in each table. The zero values indicate the situations in which the independent parameters don’t affect the dependent parameters. In cases where an independent parameter is found to be affected by dependent parameters, the coefficient is highlighted with a different colours as red indicates high negative correlation and green indicates high positive indication.
In The coefficient values shown represent the rate of change in the dependent parameters (when a unit change occurs in the independent parameters (discount rate, treatment and electricity prices and water capacity). For example, in , −0.027 is the average change in Total Cost (dependent parameters) when a unit change occurs in discount rate (independent parameters). Also, shows that 1 is the average change in Total Env. (dependent parameters) when a unit change occurs in treatment cost (independent parameters).
Similarly, in , 0.114 is the average change in Total Cost when a unit change occurs in Electricity Cost. While 1 is the average change in Total Env. (dependent parameters) when a unit change occurs in treatment cost (independent parameters). In for 20 years of operations, 0.825 is the average change in Total Cost when a unit change occurs in Treatment Cost.
Furthermore, The interaction effects of the independent parameters on the dependent parameters shown in for the 5-, 10-, and 20-year periods. Only the parameters that are found to have an interaction effect shown in . In each panel, the parameters are listed on the x-axis, and the magnitude of the respective effects of the independent parameters are represented on the y-axis and the vertical line shows the effect of different parameter interactions. As shown in , among the independent parameters (i.e. discount rate, electricity cost, treatment cost and water capacity), treatment cost is found to have the greatest impact on the two dependent parameters of total cost and total environment. It had the greatest impact on total environment, with a value of 1, and total cost the treatment cost is the highest among all. Total environment is the highest value among individuals which is 1. This indicates that pw treatment costs play an important role in determining the total cost and environmental impact of pw management. Electricity cost and discount rate have the second- and third-greatest impact, respectively. Water capacity has zero effect on both total cost and total environment. The two categories of interactions that affect the dependent parameters are discount rate x treatment cost and discount rate x electricity cost.
Figure 5. The interaction between the independent and dependent parameters in the produced water supply chain model.

shows the interaction effect of discount rate x treatment cost and discount rate x electricity cost for the periods of 5, 10 and 20 years. It can be observed in that between the two categories examined (discount rate x treatment cost and discount rate x electricity cost), discount rate x treatment cost recorded the highest interaction in each analysis (5 years = −0.115, 10 years= −0.222 and 20 years = −0.3626). However, in terms of total environment, both discount rate x treatment cost and discount rate x electricity cost recorded values zero.
Figure 6. The impact of two categories of interactions on the dependent parameters over the 5-,10- and 20- year periods.

These results provide critical insights into the input parameters (via GSA) and identified the most effective input parameters. The major findings, indicate that discount rate, treatment and electricity prices significantly influence PW supply chain system.
5. Conclusion and future work
In the oil and gas industry, PW is one of the largest waste streams, and PW management poses a number of economic and environmental challenges. It is therefore essential to find a method of PW management that is sustainable, environmentally friendly, and cost-effective. In this study, the KOC’s PW supply chain management is presented to address this need. We investigate the short, medium, and long-term planning periods of the KOC-PW management. To reduce the uncertainty associated with modelling of KOC’s PW supply chain and to assess the effects of different parameters on output, we develop and test an optimisation model using four major parameters: 1) Discount Rate, 2) Cost of Treatment, 3) Cost of Electricity and 4) Water Supply Capacity. We investigate, the sensitivity of the optimisation model’s parameters using the GSA method. Conducting a GSA is found to enhance the accuracy of the model, reduce uncertainty in the estimation of values and increase understanding of the entire system.
Our findings show that all the investigated parameters significantly influence KOC’s PW supply chain system, except Water Supply Capacity. We also find a negative correlation exists between Discount Rates and Total Cost and Total Environment, whereas Electricity Cost and Treatment Costs are found to positively correlate with Total Cost and Total Environment in KOC’s PW management system. Various interactions occur among the independent parameters; specifically, interactions exist between the Electricity Cost and Discount Rate, between Treatment Cost and Discount Rate, leading to an inverse relationship with Total Cost.
Using a real case study, we facilitate the presentation of comparable results of operational costs, treatment costs, and transportation costs as well as environmental impact results for PW management to assess and guide managerial decisions.
Future studies could incorporate additional factors into the optimisation model we have established, such as exchange rates, pipeline costs and well locations. In addition, based on the GSA framework used in this study, future studies should assess the impact of the different parameters including the pipeline material, pipeline length, salinity and electricity consumption level under different conditions on the reuse and manage PW. Through this study, we focused on the most input parameters that ultimately contributing to PW supply chain system in KOC. According to the results of the study, the proposed input parameters should be taken into consideration during the management of PW.
In conclusion, by incorporating the approaches of MO-MILP and GSA, the model can effectively address the trade-off between economic and environmental sustainability. It allows decision-makers to explore different scenarios, quantify the economic and environmental implications, identify optimal solutions, and make informed decisions that balance both objectives. In addition, our findings confirm and emphasise the importance of conducting a GSA for parameters selection for gaining an understanding of the sensitivity of selected independent parameters. Also, as a result of these findings, stakeholders, policymakers, and industry professionals can make more informed decision, which in turn will support the management of the PW supply chain system. This study can guide decision-making, inform policy development, and contribute to the overall sustainability of produced water management practices. The research results and expected outcomes enable KOC to balance economic profitability with environmental responsibility, promoting a more sustainable and resilient approach to PW management. The novel information generated by this research serves as a valuable resource for improving produced water management, guiding planning and prioritisation within Kuwait Oil Company and contributing to sustainable practices in the oil and gas industry as a whole.
Abbreviations
PW | = | Produced water. |
KOC | = | Kuwait oil company. |
GSA | = | Global sensitivity analysis. |
Supplemental Material
Download MS Word (116 KB)Disclosure statement
No potential conflict of interest was reported by the author(s).
Data availability statement
The authors confirm that the data supporting the findings of this study are available within the article [and/or] its supplementary materials.
Supplementary material
Supplemental data for this article can be accessed online at https://doi.org/10.1080/19397038.2024.2324006
Additional information
Notes on contributors
Tohid Erfani
Dr Tohid Erfani is an Associate Professor in computational modelling and infrastructure systems engineering and serves as Co-Director of the Centre for Urban Sustainability and Resilience at UCL. He also leads the team at the United Nations University Sustainability Nexus AID Programme on Infrastructure Resilience. Leading the Environmental Systems, Data and Analytics group at UCL, and through collaborations with government and infrastructure sector stakeholders, his work bridges advanced methodologies with practical applications. Dr Erfani specialises in developing tailored models using mathematical and statistical analyses, graph theory, and artificial intelligence to solve environmental system challenges. His UKRI project involvement spans water security, water quality trading for food security, and the development of adaptive strategies for net zero emission infrastructure planning under uncertainty. His approach to adaptive water infrastructure planning under uncertainty was recognised with the American Geological Union’s Water Resource Research Editor’s Award in 2020.
Afrah AlEdan
Dr Afrah AlEdan has a Ph.D. in Civil and Environmental Engineering from University College London (2023) and a Bachelor’s degree in Industrial Engineering from Kuwait University (2007). She is currently a Senior Projects Engineer at Kuwait Oil Company, where she works on water management projects in the oil field. Her research interests include water management, environmental management, cost optimisation, and system optimisation
References
- Ahmad, N. A., P. S. Goh, L. T. Yogarathinam, A. K. Zulhairun, and A. F. Ismail. 2020. “Current Advances in Membrane Technologies for Produced Water Desalination.” Desalination 493 (May): 114643. https://doi.org/10.1016/j.desal.2020.114643.
- Al-Abdullah, Y. M., Al-Ragom, F., Al-Sayegh, O and Heaps, C. 2019. Kuwait Energy Outlook Sustaining Prosperity Through Strategic Energy Management. Kuwait. https://www.undp.org/content/dam/rbas/doc/EnergyandEnvironment/KEOreportEnglish.pdf.
- AlAnezi, K., M. Al-Samhan, M. Belkharchouche, W. Abuhaimed, S. Alali, K. Alenizi, A. Alfuraij, et al. 2018. “Comparative Analysis of Produced Water Collected from Different Oil Gathering Centers in Kuwait.” Journal of Environmental Protection 9 (06): 736–750. https://doi.org/10.4236/jep.2018.96046.
- Al-Ballam, S., Pandey, D., Pallath, G., & Kuijvenhoven, C. 2018. “A Cost-Effective Water Management Strategy for Developing Large Heavy Oil Field in Northern Kuwait.” Society of Petroleum Engineers - SPE. https://doi.org/10.2118/193736-MS.
- Aledan, A. B., and T. Erfani. 2023. “Sustainable Produced Water Supply Chain Design and Optimisation: Trading-Off the Economic Cost and Environmental Impact in Kuwait Oil Company.” Journal of Cleaner Production 391 December 2022:136185. https://doi.org/10.1016/j.jclepro.2023.136185.
- Al-Ghouti, M. A., M. A. Al-Kaabi, M. Y. Ashfaq, and D. A. Da’na. 2019. “Produced Water Characteristics, Treatment and Reuse: A Review.” Journal of Water Process Engineering 28 September 2018:222–239. https://doi.org/10.1016/j.jwpe.2019.02.001.
- Alhumoud, J. M., H. S. Behbehani, and T. H. Abdullah. 2003. “Wastewater reuse practices in Kuwait.” The Environmentalist 23 (2): 117–126. https://doi.org/10.1023/A:1024831503569.
- Ali, F. M., Al Wazzan, R., Robinson, M., & Thawer, R. 2013. “Efficient Upgrading Philosophy to Manage Future Effluent Water in the Kuwait Oil Company, Greater Burgan Field.” SPE Middle East Oil and Gas Show and Conference, 2: 1465–1471. https://doi.org/10.2118/164336-ms.
- Al-Jarallah, R. 2013. “The Potential for Reusing Grey Water and Its Generation Rates for Sustainable Potable Water Security in Kuwait.” Journal of Engineering Research 1 (1): 97–112.
- Alkhudhiri, A., N. Darwish, and N. Hilal. 2013. “Produced Water Treatment: Application of Air Gap Membrane Distillation.” Desalination 309: 46–51. https://doi.org/10.1016/j.desal.2012.09.017.
- Alsayegh, O. A. 2021. “Barriers Facing the Transition Toward Sustainable Energy System in Kuwait.” Energy Strategy Reviews 38 (November): 100779. https://doi.org/10.1016/j.esr.2021.100779.
- Alzahrani, S., and A. W. Mohammad. 2014. “Challenges and Trends in Membrane Technology Implementation for Produced Water Treatment: A Review.” Journal of Water Process Engineering 4 (C): 107–133. https://doi.org/10.1016/j.jwpe.2014.09.007.
- Cheng, K., Z. Lu, and K. Zhang. 2019. “Multivariate Output Global Sensitivity Analysis Using Multi-Output Support Vector Regression.” Structural and Multidisciplinary Optimization 59 (6): 2177–2187. https://doi.org/10.1007/s00158-018-2184-z.
- Clark, C. E., and J. A. Veil. 2009. Produced Water Volumes and Management Practices in the United States. United State: U.S. Department of Energy.
- Coha, M., G. Farinelli, A. Tiraferri, M. Minella, and D. Vione. 2021. “Advanced Oxidation Processes in the Removal of Organic Substances from Produced Water: Potential, Configurations, and Research Needs.” Chemical Engineering Journal 414 September 2020:128668. https://doi.org/10.1016/j.cej.2021.128668.
- Conrad, C. L., Y. Ben Yin, T. Hanna, A. J. Atkinson, P. J. J. Alvarez, T. N. Tekavec, M. A. Reynolds, et al. 2020. “Fit-For-Purpose Treatment Goals for Produced Waters in Shale Oil and Gas Fi Elds.” Water Research 173:115467. https://doi.org/10.1016/j.watres.2020.115467.
- Cui, Y., Z. Geng, Q. Zhu, and Y. Han. 2017. “Review: Multi-Objective Optimization Methods and Application in Energy Saving.” Energy 125:681–704. https://doi.org/10.1016/j.energy.2017.02.174.
- Dawoud, H. D., H. Saleem, N. A. Alnuaimi, and S. J. Zaidi. 2021. “Characterization and Treatment Technologies Applied for Produced Water in Qatar.” Water (Switzerland) 13 (24): 3573. https://doi.org/10.3390/w13243573.
- Dudek, M., E. A. Vik, S. V. Aanesen, and G. Øye. 2020. “Colloid Chemistry and Experimental Techniques for Understanding Fundamental Behaviour of Produced Water in Oil and Gas Production.” Advances in Colloid and Interface Science 276:102105. https://doi.org/10.1016/j.cis.2020.102105.
- Echchelh, A., T. Hess, and R. Sakrabani. 2018. “Reusing Oil and Gas Produced Water for Irrigation of Food Crops in Drylands.” Agricultural Water Management 206 (July 2017): 124–134. https://doi.org/10.1016/j.agwat.2018.05.006.
- Elaila, S. Z., Elred, A., Al Makseed, N. H., Al-Banai, M. K., & Al-Mutairi, S. N. 2017. “Subsurface Disposal of Produced Water and Simultaneous Increased Oil Production Achieved within the Same Wellbore Using Inverted ESP - North Kuwait Case Study.” Society of Petroleum Engineers - SPE Kuwait Oil and Gas Show and Conference 2017. https://doi.org/10.2118/187624-ms.
- Eltony, M. N. 2008. “Estimating Energy Price Elasticities for the Non-Oil Manufacturing Industries in Kuwait.” OPEC Energy Review 32 (2): 184–195. https://doi.org/10.1111/j.1753-0237.2008.00149.x.
- Entrekin, S., A. Trainor, J. Saiers, L. Patterson, K. Maloney, J. Fargione, J. Kiesecker, et al. 2018. “Water Stress from High-Volume Hydraulic Fracturing Potentially Threatens Aquatic Biodiversity and Ecosystem Services in Arkansas, United States.” Environmental Science and Technology 52 (4): 2349–2358. https://doi.org/10.1021/acs.est.7b03304.
- Erfani, T., and R. Erfani. 2015a. “An Evolutionary Approach to Solve a System of Multiple Interrelated Agent Problems.” Applied Soft Computing Journal 37:40–47. https://doi.org/10.1016/j.asoc.2015.07.049.
- Erfani, T., and R. Erfani. 2015b. Fair Resource Allocation Using Multi-Population Evolutionary Algorithm. Applications of Evolutionary. Computation: Springer International Publishing.
- Erfani, T., H. Mokhtar, and R. Erfani. 2018. “Self-Adaptive Agent Modelling of Wind Farm for Energy Capture Optimisation.” Energy Systems 9 (1): 209–222. https://doi.org/10.1007/s12667-017-0243-y.
- Fakhru’l-Razi, A., A. Pendashteh, L. C. Abdullah, D. R. A. Biak, S. S. Madaeni, and Z. Z. Abidin. 2009. “Review of Technologies for Oil and Gas Produced Water Treatment.” Journal of Hazardous Materials 170 (2–3): 530–551. https://doi.org/10.1016/j.jhazmat.2009.05.044.
- Freyman, M. 2014. “Water Demand by the Numbers.”
- Goodwin, S. 2014. Dissertation Water Management and Reuse Strategies for Unconventional Oil and Gas Fields. Colorado State University.
- Hale, C., R. Erfani, and K. Kontis. 2010. “Multiple Encapsulated Electrode Plasma Actuators to Influence the Induced Velocity: Further Configurations.” 40th Fluid Dynamics Conference and Exhibit.
- Harden, C. J. 2014. “SPE Hydrocarbon economics and evaluation symposium.” Discount Rate Development in Oil and Gas Valuation.
- Hernández, E. A. A. 2021. Management of Produced Water from the Oil and Gas Industry: Treatment, Disposal and Beneficial Reuse. Politecnico di torino. https://webthesis.biblio.polito.it/19969/.
- Hribernik, A., M. Bauman, and A. Lobnik. 2009. “Aplication of 2k Factorial Design in Wastewater Decolorization Research.” 19th IMEKO World Congress 2009.
- Jabarzadeh, Y., H. Reyhani Yamchi, V. Kumar, and N. Ghaffarinasab. 2020. “A Multi-Objective Mixed-Integer Linear Model for Sustainable Fruit Closed-Loop Supply Chain Network.” Management of Environmental Quality: An International Journal 31 (5): 1351–1373. https://doi.org/10.1108/MEQ-12-2019-0276.
- Jiménez, S., M. M. Micó, M. Arnaldos, F. Medina, and S. Contreras. 2018. “State of the Art of Produced Water Treatment.” Chemosphere 192:186–208. https://doi.org/10.1016/j.chemosphere.2017.10.139.
- Kajenthira, A., A. Siddiqi, and L. D. Anadon. 2012. “A New Case for Promoting Wastewater Reuse in Saudi Arabia: Bringing Energy into the Water Equation.” Journal of Environmental Management 102:184–192. https://doi.org/10.1016/j.jenvman.2011.09.023.
- Karapataki, C. 2012. “Techno-economic analysis of water management options for unconventional shale gas developments in the Marcellus shale.” Master’s Thesis. Massachusetts institute of technology.
- Katz, L. E., R. S. Bowman, and E. J. S. 2003. “Treatment of Produced Oil and Gas Waters with Surfactant- Modified Zeolite.”
- Komzolov, A., T. Kirichenko, O. Kirichenko, Y. Nazarova, and N. Shcherbakova. 2021. “The Problem of Determining Discount Rate for Integrated Investment Projects in the Oil and Gas Industry.” MDPI-Mathematics 9 (24): 1–13. https://doi.org/10.3390/math9243327.
- Liden, T., I. C. Santos, Z. L. Hildenbrand, and K. A. Schug. 2018. “Treatment Modalities for the Reuse of Produced Waste from Oil and Gas Development.” Science of the Total Environment 643:107–118. https://doi.org/10.1016/j.scitotenv.2018.05.386.
- Lu, J. 2016. “On Randomization-Based and Regression-Based Inferences for 2K Factorial Designs.” Statistics and Probability Letters 112:72–78. https://doi.org/10.1016/j.spl.2016.01.010.
- Nashawi, I. S., A. Malallah, and M. Al-Bisharah. 2010. “Forecasting World Crude Oil Production Using Multicyclic Hubbert Model.” Energy and Fuels 24 (3): 1788–1800. https://doi.org/10.1021/ef901240p.
- Nayar, K. G., J. Fernandes, R. K. McGovern, K. P. Dominguez, A. McCance, B. S. Al-Anzi, J. H. Lienhard, et al. 2019. “Cost and Energy Requirements of Hybrid RO and ED Brine Concentration Systems for Salt Production.” Desalination 456 (June 2018): 97–120. https://doi.org/10.1016/j.desal.2018.11.018.
- Nijhawan, N., and J. E. Myers. 2006. “Constructed Treatment Wetlands for the Treatment and Reuse of Produced Water in Dry Climates.” 8th SPE International Conference on Health, Safety and Environment in Oil and Gas Exploration and Production 2006, 2, 553–560. https://doi.org/10.2118/98567-ms.
- Pianosi, F., K. Beven, J. Freer, J. W. Hall, J. Rougier, D. B. Stephenson, T. Wagener, et al. 2016. “Sensitivity Analysis of Environmental Models: A Systematic Review with Practical Workflow.” Environmental Modelling and Software 79:214–232. https://doi.org/10.1016/j.envsoft.2016.02.008.
- Rodriguez, A. N. Z. 2019. Assessment of Produced Water Treatment Technologies for Beneficial. New Mexico State University.
- Saad, M. A., S. M. E. Rayes, and J. M. Pactor. 2016. “Plant Modification to Re-Use the Produced Water from the de-Hydration Unit at NGL Plant for Irrigation.” 4 (April): 1–6.
- Salem, F., and T. Thiemann. 2022. “Produced Water from Oil and Gas Exploration—Problems, Solutions and Opportunities.” Journal of Water Resource and Protection 14 (2): 142–185. https://doi.org/10.4236/jwarp.2022.142009.
- Scanlon, B. R., R. C. Reedy, P. Xu, M. Engle, J. P. Nicot, D. Yoxtheimer, Q. Yang, et al. 2020. “Can We Beneficially Reuse Produced Water from Oil and Gas Extraction in the U.S?” Science of the Total Environment 717 (February): 137085. https://doi.org/10.1016/j.scitotenv.2020.137085.
- Siderius, C., Conway, D., Yassine, M., Murken, L., & Lostis, P. L. 2019. Characterising the Water-Energy-Food Nexus in Kuwait and the Gulf Region Middle East Centre. www.lse.ac.uk/grantham/.
- Siderius, C., D. Conway, M. Yassine, L. Murken, P.-L. Lostis, and C. Dalin. 2020. “Multi-Scale Analysis of the Water-Energy-Food Nexus in the Gulf Region.” Environmental Research Letters 15 (9): 094024. https://doi.org/10.1088/1748-9326/ab8a86.
- Simões, A., R. Macêdo-Júnior, B. Santos, L. Silva, D. Silva, and D. Ruzene. 2020. “Produced Water: An Overview of Treatment Technologies.” International Journal for Innovation Education and Research 8 (4): 207–224. https://doi.org/10.31686/ijier.vol8.iss4.2283.
- Sin, G., K. V. Gernaey, M. B. Neumann, M. C. M. van Loosdrecht, and W. Gujer. 2011. “Global Sensitivity Analysis in Wastewater Treatment Plant Model Applications: Prioritizing Sources of Uncertainty.” Water Research 45 (2): 639–651. https://doi.org/10.1016/j.watres.2010.08.025.
- Tohid, E., K. Pachos, and J. J. Harou. 2020. “Decision-Dependent Uncertainty in Adaptive Real-Options Water Resource Planning.” Advances in Water Resources 136: 103490.
- U.S. Energy Information Administration. 2016 Kuwait: Country Analysis Brief. http://www.eia.gov/beta/international/analysis_includes/countries_long/Nigeria/nigeria.pdf.
- Wagener, T., and F. Pianosi. 2019. “What Has Global Sensitivity Analysis Ever Done for Us? A Systematic Review to Support Scienti Fi C Advancement and to Inform Policy-Making in Earth System Modelling.” Earth-Science Reviews 194 (April): 1–18. https://doi.org/10.1016/j.earscirev.2019.04.006.
- Wang, Y., J. Huang, and H. Tang. 2020. “Global Sensitivity Analysis of the Hydraulic Parameters of the Reservoir Colluvial Landslides in the Three Gorges Reservoir Area, China.” Landslides 17 (2): 483–494. https://doi.org/10.1007/s10346-019-01290-9.
- Wang, M., Wright, J., Buswell, R., & Brownlee, A. 2013. “A Comparison of Approaches to Stepwise Regression for Global Sensitivity Analysis Used with Evolutionary Optimization.” Proceedings of BS 2013: 13th Conference of the International Building Performance Simulation Association, 2551–2558.
- Ye, M., and M. C. Hill. 2017. Sensitivity Analysis in Earth Observation Modelling. https://doi.org/10.1016/B978-0-12-803011-0.00010-0.