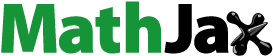
ABSTRACT
Early assessment of dosing requirements should be an integral part of developability assessments for a discovery program. If a very high dose is required to achieve the desired pharmacological effect, it may not be clinically feasible or commercially desirable to develop the biotherapeutic for the selected target unless extra measures are taken to develop a high concentration formulation or maximize yield during manufacturing. A quantitative understanding of the impact of target selection, biotherapeutic format, and optimal drug properties on potential dosing requirements to achieve efficacy can affect many early decisions. Early prediction of dosing requirements for biotherapeutics, as opposed to small molecules, is possible due to a strong influence of target biology on pharmacokinetics and dosing. Mechanistic pharmacokinetic/pharmacodynamic (PK/PD) models leverage knowledge and competitor data available at an early stage of drug development, including biophysics of the target(s) and disease physiology, to rationally inform drug design criteria. Here we review how mathematical mechanistic PK/PD modeling can and has been applied to guide early drug development decisions.
Introduction
Developability for antibody-based biotherapeutics has been defined as “the likelihood of an antibody candidate with suitable functionality to be developed into a manufacturable, stable, safe, and effective drug that can be formulated to high concentrations while retaining a long shelf life.”Citation1 Early integration of developability considerations into the drug discovery process can help to identify potential risks associated with a program and reduce later stage attrition, and the pharmaceutical industry has begun to develop such approaches to the discovery of new molecular entities.Citation2
Developability assessments for therapeutic antibodies have generally focused on issues such as solubility, stability, susceptibility to chemical degradation, aggregation propensity, and immunogenicity. Many early developability assessments rely on in vitro screens to assess various physicochemical properties. In recent years, in silico predictions using machine learning approaches based on the large amount of in vitro data available are becoming more accurate and integrated into developability assessment workflows.Citation1–5 These methods have been recently reviewed by Khetan et al.Citation6
In this review, we make the case that in silico dose predictions are an important aspect of developability assessments. Doses that can be administered are often limited by practical considerations of clinical convenience and patient compliance, as well as manufacturing or formulation limitations, depending on the desired route of administration (e.g., volume limits for subcutaneous administration). An early prediction of whether it is feasible to achieve pharmacological activity for a given target or drug concept, and whether this is achieved at reasonable doses or at a dose close to feasible limit, can inform Go/No-Go and target prioritization decisions and identify potential risks associated with a program. Understanding the quantitative relationship between drug properties, such as valency, affinity, half-life, and dose, can also help direct lead selection and optimization strategies.
Traditionally, dose predictions are performed only after a drug reaches clinical development, when data becomes available. However, a bottom-up in silico approach based on the understanding of the mechanism of action (MOA) can be used to provide quantitative insights into drug properties and dosing requirements for a safe and effective drug at an early stage of development for antibodies, even before the lead molecule becomes available. This is possible because of the relative predictability of pharmacokinetics for biotherapeutics, especially monoclonal antibodies (mAbs)Citation7–9 and their high specificity for the target. One of the most common modeling approaches at this early stage of development is called mechanistic pharmacokinetic/pharmacodynamic (PK/PD) modeling, which is the focus of this review.
Through examples, we demonstrate that leveraging mechanistic PK/PD models at an early stage of drug discovery and development can inform drug developability. These models can be used to answer questions common to early drug discovery and development, such as 1) What is the estimated dose to achieve the intended pharmacology in the clinic and is it feasible to achieve this dose? 2) Is there an optimal target within a pathway? and 3) What are the required drug properties to achieve the desired pharmacology at reasonable doses? Published examples of how modeling can be applied will be presented (summarized in ), along with a discussion of why the adoption of these methods has been slow and how progress might be made.
Table 1. Summary of examples for applications of mechanistic PK/PD models.
Introduction to mechanistic PK/PD modeling
Mechanistic PK/PD modeling links PK to PD through a biophysical description of the therapeutic MOA and relevant target biology. With a mathematical description of these key mechanisms, mechanistic PK/PD models can be used to support decisions across the drug discovery and development pipeline (). The application of mechanistic PK/PD modeling to later stage drug development decisions has been extensively reviewed.Citation10,Citation16–19 To support later drug development and clinical stage decisions, mechanistic PK/PD models rely on integrating data from in vitro and in vivo preclinical studies, as well as clinical PK, biomarker, and response data. This approach has improved extrapolation capabilities to predict drug efficacy and safety in humans compared to empirical PK/PD modeling, therefore it is also commonly used in translational research.Citation11
Figure 1. Schematic of how mechanistic PK/PD models can support drug discovery and development across the pipeline. Different data can be leveraged at each stage (text above the model diagram) to build a mechanistic PK/PD model that can be used to answer questions specific to the stage of development (text below the model diagram). Established early, then updated continuously, the model can seamlessly support decisions from early discovery to clinical. Figure created with BioRender.Com.

At an early stage, mechanistic PK/PD modeling can leverage data available in the literature and quantitative information about the intended pharmacology to answer questions around dosing feasibility and optimal therapeutic design (). These mechanistic PK/PD models can be built using known target biology (e.g., target half-life, expression levels) and drug parameters such as binding affinity, half-life, and bioavailability. A relevant surrogate metric (e.g., target engagement, target inhibition, total target levels, or active trimers formed by a drug engaging two targets) is used to determine effective dosing. Model simulation and analysis can be used to predict the active dose in the clinic, and to inform optimal drug properties and drug property ranges.
Mechanistic PK/PD modeling has been successfully used to guide early drug discovery decisions by defining a range of drug properties that are expected to achieve the most robust response with respect to receptor occupancy (RO) and/or target engagement (TE). As described more than a decade ago by Davda and Hansen, mechanistic PK/PD modeling can be used to predict target engagement responses across a range of drug properties, which are then used to guide molecule selection based on binding affinity and drug exposure properties.Citation12 There are many cases in which parameter scans, like those outlined in Marcantonio et al. to assess target feasibility,Citation20 have also been used to identify optimal drug properties. Detailed examples highlighting applications of mechanistic PK/PD models to early discovery decisions follow.
Early-stage questions informed by mechanistic PK/PD modeling
Is there an optimal target within a pathway? Is it better to target the ligand or the receptor?
Models that focus on the upstream interactions of ligand binding to receptors can be leveraged to optimize targeting strategy, as demonstrated in the example of inhibiting IL13-mediated signaling by Tiwari et al.Citation21 IL-13 is a pleiotropic cytokine. It first binds to IL13 receptor α1 (IL13Rα1), then recruits IL4 receptor α to form a ternary complex that triggers downstream signaling. IL-13 can also bind to IL13 receptor α2 (IL13Rα2), a decoy receptor that does not trigger downstream signaling. Two antibodies, IMA-638 and IMA-026, were developed. IMA-638 binds to IL4Rα and prevents the formation of the active ternary complex, but does not interfere with IL-13 binding to IL13Rα2. IMA-026 blocks IL-13 binding to both IL13Rα1 and IL13Rα2. A mechanistic PK/PD model describing the interactions between ligand and antibodies, antibody PK, and clearance of the antibody-IL13 complex was developed and calibrated to PK and total IL-13 data. When used to simulate dosing with each molecule, the model predicted that, despite the lower Kd value of IMA-026 (i.e., more potent binder), the IMA-638 molecule would be more effective at reducing the formation of active receptor complex formation. This result was consistent with the efficacy results. Even though this model was developed retrospectively, it demonstrates the value in building a mechanistic PK/PD model early in the program. Using existing knowledge and an assumption of typical antibody PK, a mechanistic PK/PD model of IL13 and two potential drugs was able to inform which mechanism to focus on, eliminating the need for two parallel programs in parallel to compare the effects of IMA-638 and IMA-026 in clinical studies.
At the target identification and preclinical validation stage, developability should include an assessment of whether the therapeutic concept is feasible or optimal. For example, when a ligand-dependent signaling pathway is identified as a potential target for disease intervention, the team should consider whether it is better to develop an antibody that binds to the ligand or to the receptor as the optimal approach for interrupting the ligand-receptor signaling. Dwivedi et al.Citation22 developed a multiscale model of IL6 signaling for Crohn’s disease (CD) to explore this question for a complex ligand-receptor signaling pathway. Both IL6:IL6Rα:gp130 complex and IL6:soluble IL6Rα:gp130 complex can lead to activation of STAT3 signaling. In this system, various antibodies could be used to disrupt the formation of either complex: an antibody binding anti-IL6, an antibody binding IL6Rα, an antibody binding both IL6 and IL6:IL6R complex, or a gp130-Fc fusion, which competes with IL6 for binding to IL6R. A multi-compartment PK/PD model of CD, including gut and liver as the sites-of-action, was developed to include all IL6, IL6R and gp130 interactions. The model describes the formation of the IL6:IL6R:gp130 ternary complex, where IL6 is present as a soluble ligand, and gp130 and IL6R can be either membrane-bound or soluble. The model was developed using CD patient data, validated against clinical data for tocilizumab, and then used to explore the therapeutic effect of each type of therapeutic described above. Assuming each drug had the same disposition properties, simulations were performed to predict the C-reactive protein (CRP) response for each treatment. The results suggested that an antibody with a dual mechanism, targeting both IL6 and IL6:soluble IL6Rα, would show the best reduction in CRP. This model combined a mechanistic understanding of disease biology and available data characterizing key molecular markers in patients and healthy individuals to computationally explore the optimal targeting strategy.
In more recent examples, mechanistic PK/PD models were used to prospectively compare different mechanisms for antagonizing a target.Citation23 In one example, the team used modeling to explore two slightly different antibody-based approaches to targeting the protease tryptase in the lung for treatment of asthma and allergic airway disease. In the lung interstitial space, the tryptase exists either as a long-lived inactive monomer or an active tetrameric form that can dissociate into monomers quickly. The protein science group was able to design two different types of antibodies with distinct mechanisms, the first binds selectively to the tetrameric form, which stabilizes it, but also blocks its function, while the second binds to both forms and promotes fast dissociation of the tetramer into the long-lived inactive monomer. Despite the concern that the second mechanism involves a high percentage of unproductive binding to the more abundant inactive monomeric form, a mechanistic model describing tetramer formation, dissociation into monomer, binding of drug to the different forms, and clearance of the different species, clearly suggested the advantage of the second mechanism by inducing tetramer dissociation. Furthermore, the model suggested that a weak binder (one with fast antibody-induced tetramer dissociation) would reduce unproductive binding without compromising tetramer inhibition. These simulation results guided the decision to focus on the development of the antibody with the second mechanism (binding both forms) without further affinity maturation.Citation23
These examples demonstrate the power of mechanistic modeling applied in very early stages of development to help choose the best MOA. Research teams can then focus on the mechanisms with the highest chance of success.
Is it feasible to achieve intended pharmacology in the clinic?
Once a target/mechanism has been selected, a related question that arises early in drug discovery is dosing feasibility, which can be defined as whether a desired pharmacology or MOA can be achieved at reasonable doses and dosing regimen. The first step to answering this question is to gain a quantitative understanding of potential dosing requirements for a novel therapeutic. Relatively simple mechanistic PK/PD models that are designed to be informed by literature and other readily available information can be used to predict the effective dose for a biotherapeutic. This was demonstrated recently in Marcantonio et al.,Citation20 where one- and two-compartment PK/target engagement models were used to project the effective dose for a panel of 9 biotherapeutics. Using target engagement and target inhibition criteria based on the drugs’ intended MOAs, the model-predicted effective doses were almost all within 3-fold of clinically approved doses for the biotherapeutics. This type of workflow can be applied to project dosing requirements for novel targets and novel drug concepts and used to assess feasibility, as discussed in the examples below.
By considering the predicted dose in the context of practical considerations like feasible dosing regimen for a given indication and maximum dose from a manufacturing or formulation point of view, the ease of developing a drug can be assessed. This concept is demonstrated here in a retrospective analysis of magrolimab, an anti-CD47 mAb. CD47, a marker of self that can inhibit phagocytosis through interactions with SIRPα, is overexpressed on many cancer cells.Citation24 Magrolimab is currently being evaluated at a dose of 30 mg/kg administered intravenously (IV) on a Q1W schedule in patients with myelodysplastic syndrome and acute myeloid leukemia, a dose and dosing frequency that could be considered prohibitive in other indications and that could provide challenges considering the amount of drug product required for clinical trials and the associated costs.Citation25,Citation26
Using the approach outlined in Marcantonio et al.,Citation20 a model of magrolimab was developed and model simulations were used to predict the dose required to achieve 95% inhibition of CD47-SIRPa interactions. The Monospecific Anti-Receptor with Membrane Ligand Competitor (4-compartment) Model from Applied BioMath Assess™ was used for the simulations. The model includes central, peripheral, and a site-of-action compartment, and describes drug distribution and elimination, target expression, binding to target of interest, and competition with a membrane-associated ligand (). The model was chosen because it accounts for the fact that the receptor-ligand interactions are occurring at a cell-cell interface. Parameters were identified from literature as previously describedCitation20 using information about CD47 expression levels, SIRPa expression levels, cell numbers, and receptor turnover.Citation27–37 All the information/data used to parameterize the model can be obtained prior to the availability of preclinical or clinical PK/PD data. Simulations of magrolimab PK, CD47 receptor occupancy, and CD47:SIRPa inhibition for the range of doses explored in the clinic were performed ().Citation38 Based on these simulations, the model predicted that 9.37 mg/kg IV Q1W is required to sustain 95% target occupancy, while 23.7 mg/kg IV Q1W is required to sustain 95% target (CD47:SIRPa) inhibition, consistent with clinical observations and the current Phase 2 dose (). While this analysis was done retrospectively with magrolimab-specific antibody half-life used for simulations, the results are robust to changes in antibody half-life. The model predicts a dose of 7.6 mg/kg to 10.5 mg/kg IV Q1W would be required to sustain 95% target occupancy for antibody half-life ranging from 10 to 28 days. This modeling approach could have been used to prospectively identify the dosing challenges and at a minimum, suggest prioritization of activities to mitigate the impact of high dosing requirements.
Figure 2. Mechanistic PK/PD model analysis of magrolimab. A) model diagram of the monospecific anti-receptor with membrane ligand competitor (4-compartment) model from Applied BioMath Assess™ used for simulating PK, target engagement and target inhibition of magrolimab. B) Simulations of 7 doses of magrolimab, dosed at 3 to 50 mg/kg IV Q1W. Left panel is simulated drug concentration vs. time in central compartment, middle panel is target engagement vs. time in tumor (disease compartment), and right panel is target inhibition vs. time in tumor. C) from the simulations in B), the dose vs. trough target engagement and trough target inhibition in the tumor is plotted. Doses predicted to achieve 95% target engagement or inhibition are indicated.

A recent application of a mechanistic PK/PD model to test the feasibility of a drug concept was outlined in Kondic et al.Citation23 In this example, Novartis used a mechanistic PK/PD model to test the feasibility of achieving therapeutic levels of GDF15 through administration of a non-antagonist anti-GDF15 antibody. The model used is of similar complexity as in the previous example and describes antibody PK, binding to GDF15, and GDF15 turnover. In this case, the metric of interest was an increase in total (antibody-bound and free) GDF15 levels, with a 100- to 1000-fold increase defined as the target threshold based on comparator analysis. The model was used to explore a range of drug properties and baseline GDF15 concentrations to find the maximum level of GDF15 increase that could be achieved. The final analysis suggested that GDF15 synthesis rates, which were derived from baseline GDF15 level and GDF15 degradation rates, were the limiting factor, and the desired 100- to 1000-fold increase was not feasible. Applied at this early stage, the model was used to inform a No-Go decision. The model was then extended to support an alternative approach of administering anti-GDF15 antibody pre-complexed with recombinant GDF15.
For immune agonists, the feasibility of achieving a therapeutic window is an important consideration. CD3 T-cell engagers are bispecific biotherapeutics with a tumor-associated antigen (TAA) binding domain and a CD3 binding domain. They are designed to promote interactions between CD3+ T cells and tumor cells in order to activate the T cells and induce immunogenic cell death. For T-cell engagers, assessing the risk for on-target, off-tumor binding associated toxicity can be important in determining if a tumor antigen is an attractive target. Solitomab is an EpCAM/CD3 bispecific T-cell engager that was tested in a Phase 1 clinical trial in patients with relapsed/refractory solid tumors. A maximum tolerated dose of 24 µg/day administered as continuous IV infusion was identified. Dose-limiting toxicities included diarrhea and liver toxicity. Diarrhea severity was dependent on dose, and subsequent analysis was suggestive of on-target toxicity. Adverse events prevented dose escalation to therapeutic levels, although signs of antitumor activity were observed.Citation39
In this retrospective analysis, the T-cell engager model for solid tumors in Applied BioMath Assess™ was once again parameterized from literature and simulated to predict toxic and effective doses and therapeutic index.Citation13–15,Citation27,Citation33,Citation40–43 This model mechanistically describes the binding and crosslinking of TAA and CD3 to form an active trimolecular complex or “trimer”, which is used as the surrogate for activity (). The model was simulated to predict solitomab PK and trimer formation. The toxic dose was projected as the dose required to achieve a time-averaged 500 trimers per T cell in the gastrointestinal compartment, while the effective dose was projected as the dose required to achieve the same 500 trimers per T cell in the tumor compartment. The threshold of 500 trimers was chosen based on experimental data suggesting 200 to 1000 receptors per cell is the minimum antigen density required for CAR-T or T-cell engager activity.Citation44,Citation45 Model simulations predicted a toxic dose of 28 µg per day, comparable to the observed maximum tolerated dose of 24 µg per day. The model predicted an effective dose of 13.5 µg per day, within 2-fold of the toxic dose, suggesting a minimal therapeutic index (). This conclusion was robust across a range of drug affinities and half-lives. If applied prospectively, this analysis could have identified the development risks associated with this target.
Figure 3. Mechanistic PK/PD model analysis of solitomab. A) model diagram of the T cell engager for solid tumors model in Applied BioMath Assess™ used for simulating solitomab PK and trimer formation in the tumor and tox compartments. B) from these simulations, the dose vs. mean trimer in the tumor and tox compartments are plotted. The dose predicted to result in 500 trimers/cell in each compartment is indicated.

These examples highlight the potential for mechanistic PK/PD modeling predicted doses to provide early insights into potential development risks for a biotherapeutic and support prioritization of downstream activities.
What are the required drug properties to achieve desired pharmacology at reasonable doses?
Once target selection and feasibility has been established, a lead generation campaign will be initiated and the lead molecule will be funneled through various selection processes where drug properties will be assessed: binding assays will be used to bin molecules based on a range of binding affinities for the target; preclinical in vivo studies will be performed to assess PK properties of lead molecules; and in vivo target engagement will be evaluated to validate the potential for in vivo pharmacology. Applying mechanistic PK/PD modeling prior to these phases of drug discovery allows researchers to focus on drug properties required for the program to succeed.
In an analysis performed by Tiwari et al.,Citation46 a mechanistic PK/PD model was used to explore the relationship between binding affinity of mAbs and target expression and turnover for both soluble and membrane-bound targets. The model was used to simulate target occupancy for hypothetical targets with various expression levels and turnover rates across a range of doses and drug binding affinities. The researchers evaluated the doses and drug binding affinities needed to achieve 90% coverage and defined rule-of-thumb relationships to roughly define optimal binding affinities by taking into account three factors: 1) baseline target concentrations (for soluble targets), 2) receptor levels and turnover rates (for membrane-bound targets), and 3) receptors expressed in both soluble and membrane forms. For soluble targets, assuming the mAb-target complex elimination is the same as elimination of drug alone, the optimal KD is 10% of the baseline target concentration (S0) (EquationEquation 1(1)
(1) ). In the case of membrane receptors, where the mAb-receptor complex is eliminated at the same rate of the receptor (faster than mAb alone), the optimal KD is a ratio of internalization rate (kelDM) and association rate (kon) of the mAb (EquationEquation 2
(2)
(2) ). If target is expressed as both soluble (but pharmacologically inactive) and membrane-bound, using the same assumptions as above for soluble and membrane receptors, the optimal KD is the same as described in EquationEquation 2
(2)
(2) (when the soluble target concentration, S0, is low compared to membrane receptor, M0), but bounded by target expression when there is a highly soluble target.
To “validate” their approach, the authors used the model to compare the predicted optimal binding affinity to the reported values for a number of marketed therapies. This comparison illustrates that there may still be room for improvement for anti-VEGF therapies (by developing anti-VEGF treatments with higher binding affinities), but that improving binding affinities for anti-TNF and anti-RANKL mAbs will not improve target coverage. For anti-EGFR and anti-Her2 therapies, the predicted optimal affinities were in-line with the marketed therapies and, for one case, that of nimotuzumab, the off-target effects were minimized (as predicted for antibodies with lower binding affinity). This work highlights that mechanistic PK/PD modeling can be used to define the criteria for developability of biologics, in a similar way that Lipinski’s Rule of 5 is applied to the development of small molecules.Citation47
This approach has been applied as part of a comparator analysis to guide candidate selection for an antibody targeting CCL20, a soluble ligand for the treatment of atopic dermatitis. Kapitanov et al.Citation48 developed a mechanistic site-of-action (skin) model for CCL20 and used it to investigate why GSK3050002, a competitor molecule that binds to CCL20 with a Kd = 350 pM, showed poor clinical performance when tested in healthy subjects. In model simulations of GSK3050002, it was predicted that 90% target coverage was not maintained at the site-of-action, even at an IV bolus dose as high as 20 mg/kg. The model was then used to simulate a range of drug properties and dosing regimens of a hypothetical anti-CCL20 mAb, which showed that 90% target coverage was achievable at lower doses (300 mg subcutaneous Q2W) of an anti-CCL20 mAb with a Kd of 35 pM. These results were key in interpreting the clinical results of GSK3050002, as well as defining the optimal binding affinity for a follow-on anti-CCL20 antibody campaign.
Another example of application of mechanistic PK/PD modeling from Yu et al.Citation49 demonstrates how a model of an anti-cytomegalovirus (CMV) antibody was used to define affinity requirements prior to the initiation of lead selection. A model was developed that included viral infection dynamics (using components similar to those for HIV or hepatitis C virus), along with antibody disposition kinetics and binding to the glycoprotein complex. Using standard antibody PK parameters, dose-response simulations were performed across a range of binding affinities and dosing regimens to determine the binding affinity needed to treat a CMV infection at the recommended dosing interval. The results demonstrated that an antibody dose in the 1–5 mg/kg range and a binding affinity of 30 to 100 pM would be needed to achieve efficacy with Q4W dosing. These criteria were used for candidate selection.
These examples demonstrate that mechanistic PK/PD models can be leveraged in the early stages of lead identification to highlight key drug properties and provide a rationale for project termination if the optimal drug cannot be identified.
Conclusions
In conclusion, through multiple examples, we illustrated how mechanistic PK/PD models can be used to address questions in early discovery that affect the developability of biotherapeutics, such as predicted efficacious dose, the optimal target, and the optimal drug property. Importantly, mathematical modeling has the potential to enable an early no-go decision and integrate development considerations into early decision-making that will reduce late-stage attrition. While most of the examples described antibody-based therapeutics, mechanistic PK/PD models can be applied to other biotherapeutics, such as recombinant proteins and peptides. This is especially important when working with novel modalities or in biological space that is not yet well understood. In the absence of competitor data and prior knowledge, it is important to invest in the associated experiments first to enable the development of the mechanistic PKPD models. Developability decisions for a first-in-class molecule can still leverage mechanistic PK/PD modeling.
While the use of mechanistic modeling is expanding, the application in the early stages of drug discovery and development is trailing behind the application in clinical stages. This is evident in a recent survey which shows that quantitative systems pharmacology models (including mechanistic PK/PD models) are more frequently applied to address questions on dose selection and clinical trial design than on candidate selection and target validation.Citation50 Part of the reason for not applying modeling earlier in drug discovery may be the early discovery team’s lack of familiarity with mechanistic modeling compared to the clinical teams who have substantial experience with PK/PD or population PK modeling. In addition, since PK/PD models and population PK models are data-driven, the team may hesitate to use modeling in early discovery programs, as molecule-specific data are not yet available. The examples discussed in here serve to demonstrate that models based on literature information and target biology-related information can be very useful in making early predictions when applied appropriately.
A second challenge is the need for a quantitative understanding of the target biology, such as target expression pattern, expression level and turnover rates, to build a robust mechanistic PK/PD model. For established targets, target-related information either already exists in the literature or can be derived from available clinical data for competitor molecules. It is critical, however, these types of data are collected in early discovery programs for novel targets where literature information is sparse. Internal teams may be needed to generate these data for model development. Advancements in technologies enabling quantitative protein measurements, such as quantitative flow cytometryCitation51 and absolute protein quantitation using mass spectrometry,Citation52,Citation53 will also make data more readily available.
This early-stage mathematical modeling is often just the beginning of a learn-and-confirm cycle of model development to support a successful program. There will be caveats in the interpretation of model results, especially for novel approaches. Unavoidable sources of uncertainty will need to be taken into consideration, sometimes due to limited knowledge of targets across species, or biological mechanisms that are not captured by the model. Even though the model is intended to describe disease conditions, teams often rely on contrived in vitro systems to inform model parameters early on, which may not be directly translatable to in vivo disease conditions. However, the model can, and should, be continuously developed and refined with increased understanding and increased data availability (). Specifically, the mechanistic PK/PD model developed at an early stage using literature data can be updated with specific information for the lead molecule, such as binding potency and PK, to make the model more specific for the molecule of interest and reduce the uncertainty in model prediction. Additional downstream biology and associated mechanisms may be added to further improve accuracy and translatability. Models calibrated with preclinical PK and PD data, can then be translated to predict human PK and PD and support clinical dose selection. With a model that is already established, the flexibility to apply it along the preclinical development process and into the clinical becomes seamless, and oftentimes a critical tool for dosing decisions in the clinic. As the model is continuously updated, it can support the entire development program and provide value beyond the developability assessment (). For example, differences in target burden and/or kinetics across species may result in target-mediated drug disposition effects that were not observed preclinically, but may still significantly affect the drug half-life in humans. This paradigm shift of applying PK/PD modeling across an entire portfolio at every critical decision point will help the industry identify the failures much earlier and focus on informing, accelerating, and developing potentially best-in-class new therapies, saving substantial time and money, and ultimately improving the lives of patients.
Abbreviations
CD | = | Crohn’s disease |
CMV | = | cytomegalovirus |
CRP | = | C-reactive protein |
IV | = | intravenous |
mAb | = | monoclonal antibody |
MOA | = | mechanism of action |
PK/PD | = | pharmacokinetic/pharmacodynamic |
RO | = | receptor occupancy |
TAA | = | tumor-associated antigen |
TE | = | target engagement |
Acknowledgments
Authors would like to acknowledge the scientific contributions of Sarah DiBartolo, David Flowers, Carter Johnson, and Saheli Sarkar to some of the case studies described in the manuscript. Thanks also to Andrew Matteson, David Hagen, Victor Chang for Applied BioMath Assess™ support.
Disclosure statement
JG, FH, JA, JB, and DM were all employees of Applied BioMath, LLC during the course of this research.
Additional information
Funding
References
- Wolf Pérez A-M, Lorenzen N, Vendruscolo M, Sormanni P. Assessment of therapeutic antibody developability by combinations of in vitro and in silico methods. Methods Mol Biol. 2022;2313:57–11.
- Saxena V, Panicucci R, Joshi Y, Garad S. Developability assessment in pharmaceutical industry: an integrated group approach for selecting developable candidates. J Pharm Sci. 2009;98(6):1962–79. doi:10.1002/jps.21592.
- Jarasch A, Koll H, Regula JT, Bader M, Papadimitriou A, Kettenberger H. Developability assessment during the selection of novel therapeutic antibodies. J Pharm Sci. 2015;104(6):1885–98. doi:10.1002/jps.24430.
- Xu Y, Wang D, Mason B, Rossomando T, Li N, Liu D, Cheung JK, Xu W, Raghava S, Katiyar A, et al. Structure, heterogeneity and developability assessment of therapeutic antibodies. MAbs. 2019;11(2):239–64. doi:10.1080/19420862.2018.1553476.
- Negron C, Fang J, McPherson MJ, Wb S Jr, McCluskey AJ. Separating clinical antibodies from repertoire antibodies, a path to in silico developability assessment. MAbs. 2022;14(1):2080628. doi:10.1080/19420862.2022.2080628.
- Khetan R, Curtis R, Deane CM, Hadsund JT, Kar U, Krawczyk K, Kuroda D, Robinson SA, Sormanni P, Tsumoto K, et al. Current advances in biopharmaceutical informatics: guidelines, impact and challenges in the computational developability assessment of antibody therapeutics. MAbs. 2022;14(1):2020082. doi:10.1080/19420862.2021.2020082.
- Deng R, Iyer S, Theil FP, Mortensen DL, Fielder PJ, Prabhu S. Projecting human pharmacokinetics of therapeutic antibodies from nonclinical data: what have we learned? MAbs. 2011;3(1):61–66. doi:10.4161/mabs.3.1.13799.
- Dong JQ, Salinger DH, Endres CJ, Gibbs JP, Hsu C-P, Stouch BJ, Hurh E, Gibbs MA. Quantitative prediction of human pharmacokinetics for monoclonal antibodies: retrospective analysis of monkey as a single species for first-in-human prediction. Clin Pharmacokinet. 2011;50(2):131–42. doi:10.2165/11537430-000000000-00000.
- Betts A, Keunecke A, van Steeg Tj, van der Graaf Ph, Avery LB, Jones H, Berkhout J, van Steeg TJ, van der Graaf PH. Linear pharmacokinetic parameters for monoclonal antibodies are similar within a species and across different pharmacological targets: a comparison between human, cynomolgus monkey and hFcrn Tg32 transgenic mouse using a population-modeling approach. MAbs. 2018;10(5):751–64. doi:10.1080/19420862.2018.1462429.
- Agoram BM, Martin SW, van der Graaf PH. The role of mechanism-based pharmacokinetic-pharmacodynamic (PK-PD) modelling in translational research of biologics. Drug Discov Today. 2007;12(23–24):1018–24. doi:10.1016/j.drudis.2007.10.002.
- Danhof M, de Jongh J, De Lange ECM, Della Pasqua O, Ploeger BA, Voskuyl RA. Mechanism-based pharmacokinetic-pharmacodynamic modeling: biophase distribution, receptor theory, and dynamical systems analysis. Annu Rev Pharmacol Toxicol. 2007;47:357–400. doi:10.1146/annurev.pharmtox.47.120505.105154.
- Davda JP, Hansen RJ. Properties of a general PK/PD model of antibody-ligand interactions for therapeutic antibodies that bind to soluble endogenous targets. MAbs. 2010;2(5):576–88. doi:10.4161/mabs.2.5.12833.
- Chen J, Eshghi ST, Bova GS, Li QK, Li X, Zhang H. Epithelium percentage estimation facilitates epithelial quantitative protein measurement in tissue specimens. Clin Proteomics. 2013;10(1). Internet. doi:10.1186/1559-0275-10-18.
- Munz M, Fellinger K, Hofmann T, Schmitt B, Gires O. Glycosylation is crucial for stability of tumour and cancer stem cell antigen EpCAM. Front Biosci. 2008;13(13):5195–201. doi:10.2741/3075.
- Liu H, Rhodes M, Wiest DL, Vignali DA. On the dynamics of TCR: cD3 complex cell surface expression and downmodulation. Immunity. 2000;13(5):665–75. doi:10.1016/S1074-7613(00)00066-2.
- Hu L, Hansen RJ. Issues, challenges, and opportunities in model-based drug development for monoclonal antibodies. J Pharm Sci. 2013;102(9):2898–908. doi:10.1002/jps.23504.
- Zou H, Banerjee P, Leung SSY, Yan X. Application of pharmacokinetic-pharmacodynamic modeling in drug delivery: development and challenges. Front Pharmacol. 2020;11:997. doi:10.3389/fphar.2020.00997.
- Danhof M, de Lange Ecm, Della Pasqua OE, Ploeger BA, Voskuyl RA, Delange E. Mechanism-based pharmacokinetic-pharmacodynamic (PK-PD) modeling in translational drug research. Trends Pharmacol Sci. 2008;29(4):186–91. doi:10.1016/j.tips.2008.01.007.
- Ait-Oudhia S, Ovacik MA, Mager DE. Systems pharmacology and enhanced pharmacodynamic models for understanding antibody-based drug action and toxicity. MAbs. 2017;9(1):15–28. doi:10.1080/19420862.2016.1238995.
- Marcantonio DH, Matteson A, Presler M, Burke JM, Hagen DR, Hua F, Apgar JF. Early feasibility assessment: a method for accurately predicting biotherapeutic dosing to inform early drug discovery decisions. Front Pharmacol. 2022:13. Internet. doi:10.3389/fphar.2022.864768.
- Tiwari A, Kasaian M, Heatherington AC, Jones HM, Hua F. A mechanistic PK/PD model for two anti-IL13 antibodies explains the difference in total IL-13 accumulation observed in clinical studies. MAbs. 2016;8(5):983–90. doi:10.1080/19420862.2016.1172151.
- Dwivedi G, Fitz L, Hegen M, Martin SW, Harrold J, Heatherington A, Li C. A multiscale model of interleukin-6-mediated immune regulation in Crohn’s disease and its application in drug discovery and development. CPT: Pharmacometrics & Systems Pharmacology. 2014;3(1):e89. doi:10.1038/psp.2013.64.
- Kondic A, Bottino D, Harrold J, Kearns JD, Musante CJ, Odinecs A, Ramanujan S, Selimkhanov J, Schoeberl B. Navigating between right, wrong, and relevant: the use of mathematical modeling in preclinical decision making. Front Pharmacol. 2022;13:860881. doi:10.3389/fphar.2022.860881.
- Oldenborg P-A. CD47: a cell surface glycoprotein which regulates multiple functions of hematopoietic cells in health and disease. ISRN Hematol. 2013;2013:614619. doi:10.1155/2013/614619.
- Latham P, Kaltenbrunner O, Curling J, Levine H, Rathore A. Costing issues in the production of biopharmaceuticals. BioPharm Int [Internet]. 2004 [[accessed 2022 Nov 20];17(2): https://www.biopharminternational.com/view/costing-issues-production-biopharmaceuticals.
- Contract Development and Manufacturing Costs During Clinical Development of a New Drug. 2013. Applied Clinical Trials Online [Internet]. [accessed 2022 Nov 22]. https://www.appliedclinicaltrialsonline.com/view/contract-development-and-manufacturing-costs-during-clinical-development-new-drug
- Bianconi E, Piovesan A, Facchin F, Beraudi A, Casadei R, Frabetti F, Vitale L, Pelleri MC, Tassani S, Piva F, et al. An estimation of the number of cells in the human body. Ann Hum Biol. 2013;40(6):463–71. doi:10.3109/03014460.2013.807878.
- Sender R, Fuchs S, Milo R. Revised estimates for the number of human and bacteria cells in the body. PLoS Biol. 2016;14(8):1–14. doi:10.1371/journal.pbio.1002533.
- Trepel F. Number and distribution of lymphocytes in man. A critical analysis. Klin Wochenschr. 1974;52(11):511–15. doi:10.1007/BF01468720.
- Puro RJ, Bouchlaka MN, Hiebsch RR, Capoccia BJ, Donio MJ, Manning PT, Frazier WA, Karr RW, Pereira DS. Development of AO-176, a next-generation humanized anti-CD47 antibody with novel anticancer properties and negligible red blood cell binding. Mol Cancer Ther. 2020;19(3):835–46. doi:10.1158/1535-7163.MCT-19-1079.
- Martinez-Torres A-C, Quiney C, Attout T, Boullet H, Herbi L, Vela L, Barbier S, Chateau D, Chapiro E, Nguyen-Khac F, et al. CD47 agonist peptides induce programmed cell death in refractory chronic lymphocytic leukemia B cells via PLCγ1 activation: evidence from mice and humans. PLoS Med. 2015;12(3):e1001796. doi:10.1371/journal.pmed.1001796.
- Willingham SB, Volkmer J-P, Gentles AJ, Sahoo D, Dalerba P, Mitra SS, Wang J, Contreras-Trujillo H, Martin R, Cohen JD, et al. The CD47-signal regulatory protein alpha (SIRPa) interaction is a therapeutic target for human solid tumors. Proc Natl Acad Sci U S A. 2012;109(17):6662–67. doi:10.1073/pnas.1121623109.
- Del Monte U. Does the cell number 10(9) still really fit one gram of tumor tissue? Cell Cycle. 2009;8(3):505–06. doi:10.4161/cc.8.3.7608.
- Brooke G, Holbrook JD, Brown MH, Barclay AN. Human lymphocytes interact directly with CD47 through a novel member of the signal regulatory protein (SIRP) family. J Immunol. 2004;173(4):2562–70. doi:10.4049/jimmunol.173.4.2562.
- Jalil AR, Andrechak JC, Discher DE. Macrophage checkpoint blockade: results from initial clinical trials, binding analyses, and CD47-SIRPα structure-function. Antib Ther. 2020;3(2):80–94. doi:10.1093/abt/tbaa006.
- Leclair P, Liu C-C, Monajemi M, Reid GS, Sly LM, Lim CJ. CD47-ligation induced cell death in T-acute lymphoblastic leukemia. Cell Death Dis. 2018;9(5):544. doi:10.1038/s41419-018-0601-2.
- Andrejeva G, Capoccia BJ, Hiebsch RR, Donio MJ, Darwech IM, Puro RJ, Pereira DS. Novel SIRPα antibodies that induce single-agent phagocytosis of tumor cells while preserving T cells. J Immunol. 2021;206(4):712–21. doi:10.4049/jimmunol.2001019.
- Sikic BI, Lakhani N, Patnaik A, Shah SA, Chandana SR, Rasco D, Colevas AD, O’rourke T, Narayanan S, Papadopoulos K, et al. First-in-human, first-in-class phase i trial of the anti-CD47 antibody Hu5F9-G4 in patients with Advanced Cancers. J Clin Oncol. 2019;37(12):946–53. doi:10.1200/JCO.18.02018.
- Kebenko M, Goebeler ME, Wolf M, Hasenburg A, Seggewiss-Bernhardt R, Ritter B, Rautenberg B, Atanackovic D, Kratzer A, Rottman JB, et al. A multicenter phase 1 study of solitomab (MT110, AMG 110), a bispecific EpCAM/CD3 T-cell engager (BiTE®) antibody construct, in patients with refractory solid tumors. Oncoimmunology. 2018;Internet. doi:10.1080/2162402X.2018.1450710.
- Brischwein K, Schlereth B, Guller B, Steiger C, Wolf A, Lutterbuese R, Offner S, Locher M, Urbig T, Raum T, et al. MT110: a novel bispecific single-chain antibody construct with high efficacy in eradicating established tumors. Mol Immunol. 2006;43(8):1129–43. doi:10.1016/j.molimm.2005.07.034.
- García-Martínez E, Gil GL, Benito AC, González-Billalabeitia E, Conesa MAV, García García T, García-Garre E, Vicente V, Ayala de la Peña F. Tumor-infiltrating immune cell profiles and their change after neoadjuvant chemotherapy predict response and prognosis of breast cancer. Breast Cancer Res. 2014;16(6):488. doi:10.1186/s13058-014-0488-5.
- Thibault G, Bardos P. Compared TCR and CD3 epsilon expression on alpha beta and gamma delta T cells. Evidence for the association of two TCR heterodimers with three CD3 epsilon chains in This information is current as the TCR/CD3 complex. J Immunol. 1995;154:3814–20. doi:10.4049/jimmunol.154.8.3814.
- Mahadeva S, Wyatt JI, Howdle PD. Is a raised intraepithelial lymphocyte count with normal duodenal villous architecture clinically relevant? J Clin Pathol. 2002;55(6):424–28. doi:10.1136/jcp.55.6.424.
- Watanabe K, Terakura S, Martens AC, van Meerten T, Uchiyama S, Imai M, Sakemura R, Goto T, Hanajiri R, Imahashi N, et al. Target antigen density governs the efficacy of anti–CD20-CD28-CD3 ζ chimeric antigen receptor–modified effector CD8+ T Cells. Journal of Immunology. 2015;194(3):911–20. doi:10.4049/jimmunol.1402346.
- Ellerman D. Bispecific T-cell engagers: towards understanding variables influencing the in vitro potency and tumor selectivity and their modulation to enhance their efficacy and safety. Methods. 2019;154:102–17. doi:10.1016/j.ymeth.2018.10.026.
- Tiwari A, Abraham AK, Harrold JM, Zutshi A, Singh P. Optimal affinity of a monoclonal antibody: guiding principles using mechanistic modeling. Aaps J. 2017;19(2):510–19. doi:10.1208/s12248-016-0004-1.
- Singh AP, Krzyzanski W, Martin SW, Weber G, Betts A, Ahmad A, Abraham A, Zutshi A, Lin J, Singh P. Quantitative prediction of human pharmacokinetics for mAbs exhibiting target-mediated disposition. Aaps J. 2015;17(2):389–99. doi:10.1208/s12248-014-9690-8.
- Kapitanov GI, Chabot JR, Narula J, Roy M, Neubert H, Palandra J, Farrokhi V, Johnson JS, Webster R, Jones HM. A mechanistic site-of-action model: a tool for informing right target, right compound, and right dose for therapeutic antagonistic antibody programs. Frontiers in Bioinformatics. 2021;1:1. Internet. doi:10.3389/fbinf.2021.731340.
- Yu J, Karcher H, Feire AL, Lowe PJ. From target selection to the minimum acceptable biological effect level for human study: use of mechanism-based PK/PD modeling to design safe and efficacious biologics. Aaps J. 2011;13(2):169–78. doi:10.1208/s12248-011-9256-y.
- Lemaire V, Bassen D, Reed M, Song R, Khalili S, Lien YTK, Huang L, Singh AP, Stamatelos S, Bottino D, et al. From cold to hot: changing perceptions and future opportunities for quantitative systems pharmacology modeling in cancer immunotherapy. Clin Pharmacol Ther [Internet]. 2022. doi:10.1002/cpt.2770.
- Mizrahi O, Ish Shalom E, Baniyash M, Klieger Y. Quantitative flow cytometry: concerns and recommendations in clinic and research. Cytometry B Clin Cytom. 2018;94(2):211–18. doi:10.1002/cyto.b.21515.
- Pan S, Aebersold R, Chen R, Rush J, Goodlett DR, McIntosh MW, Zhang J, Brentnall TA. Mass spectrometry based targeted protein quantification: methods and applications. J Proteome Res. 2009;8(2):787–97. doi:10.1021/pr800538n.
- Cid-Barrio L, Calderón-Celis F, Abásolo-Linares P, Fernández-Sánchez ML, Costa-Fernández JM, Encinar JR, Sanz-Medel A. Advances in absolute protein quantification and quantitative protein mapping using ICP-MS. Trends Analyt Chem. 2018;104:148–59. doi:10.1016/j.trac.2017.09.024.