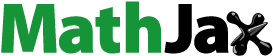
ABSTRACT
We evaluate a non-governmental housing microfinance intervention that attempts to improve housing conditions for low-income populations by simultaneously offering them a labelled loan and non-financial technical support. Using household survey data from Kenya and Uganda, we first show evidence for the successful targeting of our labelled loans because 95% of clients used the loan for the intended housing improvement. Second, our results suggest that access to small, short-term loans enables households to invest in housing upgrades that can significantly improve both the characteristics of their dwelling and their satisfaction with their dwelling.
1. Introduction
Access to adequate, safe, and affordable housing and basic services for all is seen as a human right, and hence is manifested in the 2030 Agenda for Sustainable Development (UN, Citation2015). To meet this objective, Habitat for Humanity International’s Terwilliger Centre for Innovation in Shelter (henceforth Habitat) and the Mastercard Foundation implemented the ‘Building Assets, Unlocking Access’ (BAUA) programme in Kenya and Uganda. With a population growth rate of 3.2% per year, the national housing deficit in Uganda has grown to 1.6 million houses, of which 1.395 million units are in rural areas (The Ministry of Lands, Housing and Urban Development, Citation2016). In Kenya, the deficit has grown to 2 million houses (The World Bank, Citation2017). In both countries, the projected number of people living in temporary shelters or low-quality housing is further increasing (The World Bank, Citation2011; Habitat for Humanity, Citation2019).
The BAUA programme aims to provide adequate housing for people living on less than five dollars a day. By means of small, short-term loans that have affordable payment schedules, clients can complete incremental construction on their homes. In addition, non-financial housing support services (HSS) offer construction advice and linkages to masons, engineers, and building material suppliers. Habitat recognised that its traditional approach of direct housing provision was not sufficient to meet demand en masse, so they started exploring housing microfinance (HMF)Footnote1 as a market-based solution to improve housing. Habitat supported six financial institutions in the two countries to develop HMF products that were supposed to be commercially viable but also attractive for customers. The ultimate goal was to have the financial institutions develop scalable products. The broader objective was to influence the market by proving the business case for HMF and the donor landscape by demonstrating the poverty impacts of housing finance.
This study evaluates the BAUA programme in Kenya and Uganda. Our sample consists of 2,341 existing clients from a deposit-taking microfinance bank, KWFTFootnote2, in Kenya and a commercial bank, Centenary BankFootnote3, in Uganda of whom 1,213 clients were offered the programme. As both banks agreed to withhold product roll-out in some of their branches, we rely on the staggered order – though not random – in which lenders introduced the HMF product across branches. Pre-intervention branch characteristics, however, show that more profitable and/or greater branches were targeted first, which is why we apply a difference-in-differences (DiD) approach on a two-period panel data survey. Relying on a branch-level fixed effects model with robust standard errors clustered at the branch level will further account for distorting baseline dissimilarities across treatment and comparison branches.
Like in other microfinance interventions, take-up during piloting was low (Dahal and Fiala, Citation2020). In both countries, we, therefore, decided to oversample clients who had recently taken out the HMF product. Three out of four clients in our treatment sample were randomly chosen from a small pool of existing bank clients who decided to use the product (‘adopters’). The remaining quarter in our treatment sample is a random sample of existing bank clients who were also offered the product but had not taken up the loan, yet (‘non-adopters’). Households from comparison branches compose a random sample of existing clients who were not offered the HMF product (‘comparison group’). Comparison households were not screened for interest in the HMF product.
Within the treatment branches, the adoption and non-adoption decision is obviously not at random, but clients self-selected into treatment. To account for this selection process, we use the variation in observable baseline characteristics between adopters and non-adopters in treatment branches to identify ‘hypothetical adopters’ in comparison branches using kernel matching. By comparing adopters to hypothetical adopters using kernel propensity weights, we estimate treatment effects on the treated (‘TOT’).
Despite the HMF product being unique in its combination of financial and construction support, at the time of the study, financial services providers (FSPs) in both countries had comparable products in their portfolios. KWFT did not offer a loan for generic house improvements but rather a suite of loan products targeted at specific house improvements, such as loans for water tanks or solar panels. Centenary Bank offered a home improvement product but with higher interest and no HSS or training on basic construction concepts for loan officers. Competing FSPs also offered similar products but again not with dedicated HSS with support of Habitat. While there has generally been growth in finance for housing, markets in Kenya and Uganda are still dominated by commercial institutions that offer formal mortgages. Such services only meet the financial needs of a small percentage of these countries’ populations, typically the middle- to high-income earners. Low-income households are excluded from formal housing finance because they lack formal land tenure documentation – which makes them a relevant target group for the BAUA programme.
A crucial feature of the BAUA programme is the labelled loan – a loan that is linked to housing investments through its name only. Unlike loans that are bundled with the investment and are directly transferred to the producer of the product, a labelled loan can be spent according to the desires of the borrower. The BAUA programme incentivises borrowing for housing improvements with information brochures and construction support. Our initial scoping work in Uganda confirms that labelled financial products were already being offered (meaning that labelling as such is not new). Yet usage and impacts of labelled products for a specific purpose on borrowing and investment decisions remain unclear. While previous studies show the effectiveness of labelled mobile money accounts (Dizon et al., Citation2020) and labelled loans (Augsburg et al., Citation2021), others find little evidence of potential benefits of earmarked accounts because alternative approaches like non-earmarked accounts or subsidies worked better or equally well (Habyarimana and Jack, Citation2018; Lipscomb and Schechter, Citation2018).
We find evidence for the successful targeting of our labelled loans because 95% of clients stated loan usage for the intended housing improvement. These results are in line with the concept of mental accounting, suggesting that once an account is labelled for a specific purpose, households tend to spend their money accordingly (Thaler, Citation1985). Additionally, we also see that loans did not only serve one but multiple purposes, including non-labelled purposes (e.g., school fees, business, or medical expenses). About 40% of clients mentioned at least two purposes, with 30% being unrelated to housing improvements. This may diminish impacts on housing, which is why, in a second step, we look at pre-specified improvements in dwelling characteristics and basic services.
As clients can complete various types of improvements on their housing using the loan, it can improve livelihoods in multiple ways. The methodological implication is that our sample size is too small to have enough statistical power to determine downstream effects of each type of improvement. In contrast to previous studies that focus on specific housing improvements such as sanitation upgrades (Duflo et al., Citation2015; Guiteras et al., Citation2015; Yishay et al., Citation2017; Augsburg et al., Citation2021), we contribute to the literature by analysing the purpose the loan is used for rather than impact heterogeneity by type of improvement.
Our findings suggest that facilitating small, short-term housing microfinance together with non-financial HSS has strong effects on housing conditions. In Kenya, adopters improved their homes in terms of water access, lighting (electricity or gas), cooking (electricity, gas, or solar energy), and rain protection. They invested in better quality material for roofing, flooring, and walls. In Uganda, treatment effects are positive, though not statistically significant. In both countries, the intervention results in improved housing satisfaction.
These improvements might ultimately lead to further downstream impacts on physical and psychological health outcomes (Evans, Citation2003; Thomson et al., Citation2009), but also on human capital, feelings of security, social cohesion, well-being, and asset accumulation (Cattaneo et al., Citation2009; Legovini et al., Citation2011; Galiani et al., Citation2017). However, given the sample size and hence power restrictions, we only explore potential changes on key development indicators. We find no effects in development indicators such as health, education, and pride associated with the HMF intervention. Similarly, we cannot attribute any income or wealth effects to the intervention.
In sum, the HMF product helps clients to improve the physical structure of their houses. This yields important insights for various stakeholders such as policymakers, FSPs, and donors who are interested in alleviating the supply-side challenges that restrict access to housing microfinance. We provide evidence for labelling as an effective tool for home improvements.
More specifically, our findings also offer important insights for microfinance organisations that are interested in targeting loans to specific purposes. We provide a proof-of-concept that labelling the loan can be enough to make customers use the loan for the desired purpose. Since bundled loans have obvious bureaucratic disadvantages like monitoring and operational costs that come with the sale, supply, transportation, and installation of the specific product, labelling loans can thus be an attractive alternative. As such the labelled loan is also less difficult and less costly to scale-up than bundled loans. In fact, the HMF product in this study was rolled-out and provided to clients just like the business-as-usual scenario. Two years after endline data collection, both banks still provide the HMF as part of their loan portfolio, also suggesting that the product jointly developed with Habitat is sustainable.
The remainder of the manuscript is structured as follows: Section 2 summarises the existing evidence on housing improvements; Section 3 describes the intervention and the conceptual framework; Section 4 provides the data and empirical strategy; Sections 5 and 6 provide results and robustness; and Section 7 concludes.
2. Previous evidence on housing improvements
This paper contributes to a housing literature that goes far beyond (i) the physical structure of houses, but also covers (ii) relocation of households, (iii) tenure security, and (iv) maintenance or upgrading of existing household structures and infrastructure, typically water and sanitation.
One of the most significant findings in terms of physical home improvements is that they can synergistically exploit socio-economic and health outcomes. In a quasi-experimental study, Cattaneo et al. (Citation2009) study a Mexican government programme (Piso firme) that replaced dirt floors by cement floors. They find large positive impacts on adult welfare, measured by higher satisfaction with housing and quality of life. Results also show significantly improved child health (reduction in parasitic infections, diarrhoea, and anaemia) and cognitive development. More recently, Galiani et al. (Citation2017) look at the effects of providing better houses to slum dwellers in El Salvador, Mexico, and Uruguay. The better physical housing structure led to children’s health improvements in El Salvador and Mexico, improved perception of safety and security in El Salvador, and overall satisfaction with housing and life.
Despite positive economic and non-economic impacts of relocation programmes, a major problem with this kind of interventions is people’s disconnection from previous informal networks and jobs. Legovini et al. (Citation2011) look at different informal settlement upgrading interventions in South Africa. In Limpopo Province, relocating households from an informal, unserviced home to a fully serviced Reconstruction and Development Programme (RDP) house led to increased household sizes, household upgradingFootnote4, satisfaction levels, and asset accumulation. Households were also more involved in community organisations. On the downside, the programme resulted in higher unemployment rates, which increased people’s dependency on government grants. Barnhardt et al. (Citation2017) study the effects of randomly relocating slum dwellers into improved housing on the outskirts of Ahmedabad, India. 14 years later, many beneficiaries had never moved to their new houses and those who moved did not show any significant differences to the control group. If anything, it led people to disconnect from their families and caste networks and reduced their access to informal insurance.
Research has consistently shown that greater security of tenure can increase human capital. In Peru, Field (Citation2007) uses variation in ownership rights arising from a large-scale, urban land titling programme. She finds an increase in total labour force hours that is also reflected in fewer hours spent on housework, and a substitution of adult for child labour. Similarly, Galiani and Schargrodsky (Citation2010) use a natural experiment that led to an exogenous allocation of land titles in a poor suburban area of Buenos Aires. Entitled families substantially reduced their household size, increased housing and human capital investments for their children. Although land titling allows the poor to access credit markets, the authors argue that land titling can decrease poverty by means of better education. They estimate that the benefits in terms of improved school performance are comparable to OportunidadesFootnote5, a social inclusion programme in Mexico that provides cash transfers to families.
More recently, a new literature has emerged, providing evidence of water and appropriate sanitary facilities as effective, preventive health investments. In Morocco, Devoto et al. (Citation2012) first show that households have large willingness to pay for a private water connection. The combination of less time spent collecting water and less intra-household conflicts on water matters can lead to sustained improvements in overall well-being. Second, they randomly offer credit to finance a water connection at home and find a significant improvement in self-reported well-being and social integration. On the contrary, public health effects or income gains are low. A study by Duflo et al. (Citation2015) estimates the effects of the combination of a sanitation and water system upgrade in slums in rural India. This integrated approach shows strong long-term health effects, particularly reduction of diarrhoea by 30% to 50%. Guiteras et al. (Citation2015) show that sanitation subsidies cause better uptake of sanitation ownership in comparison with community motivation and information or supply-side interventions. An alternative to encourage investments in sanitation are microloans. Using a randomised controlled trial (RCT), Yishay et al. (Citation2017) find that facilitating access to microloans significantly raises people’s willingness to pay for improved latrines. Augsburg et al. (Citation2021) study a cluster RCT in India and find that labelled loans for sanitation increase the uptake of new toilets.
3. The BAUA programme
3.1. Low-quality housing and targeting
Low-quality housing can be described by the materials used for construction and the available infrastructure, such as water, sanitation, and electricity. These characteristics usually reflect the socio-economic situation of the household and have a direct bearing on health and welfare of each household member.
In rural Kenya, the materials used for flooring range from earth (43.1%) over cement (28.4%) to dung (26.9%). One fifth of rural households have access to own toilet facilities, while almost 47.7% must rely on pit latrines without slabs/open pits. Only 12.6% of rural households have access to the electricity grid, relative to 68.4% of urban households. Similarly, in Uganda, only about a quarter of rural dwellings have a cemented floor, while 73.1% remain earthen. 60.8% of houses have walls which are made of bricks and more than two thirds of houses have iron roofs (68.6%). In urban areas, this number increases to 92.6%. While almost three quarter of the rural population have access to an improved water sourceFootnote6, 85.9% of households still use a pit latrine. Less than 10% of households are connected to the electricity grid. The number of rooms used for sleeping provides a good indication for the risk of contracting infectious diseases within the household. The more people sleep in one room, the higher the risk of respiratory infections and skin diseases. 53% of Kenyan households use one room for sleeping. In rural Uganda, 40.6% of houses have one room, shared by 2.4 people on average. In addition, the place and the type of fuel used for cooking influence indoor air quality and the degree to which household members are exposed to the risk of respiratory infections and other diseases. In Kenya, an overwhelming majority of households (84.2%) use wood as cooking fuel. While 61.1% of Kenyans cook in a separate building, about a third cooks in the house with limited ventilation (30.4%) (Kenya National Bureau of Statistics, Citation2015). In Uganda, firewood usage for cooking has decreased by 8.6 percentage points since the 2012/13 Uganda National Household Survey (UNHS) but remains the most common source for cooking at 80.8% (Uganda Bureau of Statistics, Citation2018).
3.2. The housing microfinance products
In February 2014, Habitat partnered with KWFT and Centenary Bank to support the development of viable housing products for low-income families, who were not being served by each of the bank’s existing loan products. As a result of this partnership, the local banks developed new products, called the ‘Nyumba Smart Loan’ in Kenya and the ‘Cente-Home Loan’ in Uganda, both of which include a labelled loan and non-financial HSS.
For comparison, lists the characteristics of the two loans. At the time of the study, both loans targeted rural and peri-urban residents. While the minimum and maximum loan limits were fairly similar across countries, average loan sizes differed with UGX 7,300,000 (USD 2,000 or $PPP 6,803) in Uganda and KES 76,000 (USD 700 or $PPP 1,984) in Kenya.Footnote7 Both loans had a payment period of up to 60 months. The average length of repayment was higher for the ‘Cente-Home Loan’ (a difference of six months), which corresponds to the higher average value of the loan. Clients had to provide collateral and could access the HMF product individually or in groups. In our sample, most clients are individually liable for their loan.
A common element between the two countries is the labelled loan. All funds are disbursed directly to clients who can then spend the borrowed money as they see fit. Loan use checks by product officers are not incentivised or sanctioned. If a client applies for a follow-up housing loan, the new loan is indeed linked to the loan usage of the previous housing loan. In this case, the loan should be used to finance construction, improvement, renovations, or repairs of houses on an incremental basis, or the improvement of tenure. Approval of other types of loans do not depend on loan use and are linked to loan repayment history.
Another similarity is the provision of non-financial services in form of HSS. HSS are construction advice or technical assistance that is provided to clients as part of the housing loan package. Loan takers receive information brochures and are also linked to selected masons, surveyors, engineers, and building material suppliers.
3.3. Theory of change
Habitat provides the main inputs. The programme entails Habitat selecting FSPs to partner with and then providing these financial partners with technical assistance to design a product combining microfinance and HSS components. FSPs input time to perform market research and develop the product. In a next step, FSPs begin marketing the HMF products, as they would any new microfinance product. That includes training loan officers on the product features but also on basic housing construction features. In treatment branches, loan officers then advertise the product through home visits and branches advertise the product through brochures and posters.
The product is relevant to people who want to improve their housing conditions but require financing to do so. Both the labelling of the loan and additional incentives in form of HSS can nudge clients to complete incremental housing improvements, but ultimately, they were free to use the loan as needed. If they do allocate the finance towards housing improvements, outcomes can be realised through better dwelling characteristics and/or services. We, therefore, assess the basic structure of a respondents’ dwelling, including materials used for roof, walls, and flooring, number of rooms, a separate kitchen, a chimney, and quality of available services such as water and sanitationFootnote8 as primary outcomes. All the above will aid in the overall welfare of the households and their level of satisfaction with their houses.
Households’ utility may benefit directly from these improved housing conditions. They can immediately enhance safety and protection against environmental hazards and reduce exposure to health threats such as parasites or smoke. Open defaecation and exposure to pathogenic organisms such as mosquitoes and parasitic worms can cause morbidity and mortality. Improved sanitation arising from the installation of latrines and running water in households can reduce prevalence of such diarrhoeal diseases and thus result in better health security for the households’ inhabitants (Environmental Health Project, Citation2004). Additionally, households with improved heating, lighting, and cooking facilities have lower risks of serious health hazards such as indoor air pollution and the probability of fires (Martin et al., Citation2014). A measurable reduction in the prevalence of diseases, however, takes considerable time – perhaps even intergenerationally.
Interventions that influence people’s feelings about the areas they reside can bring stability to a person’s life. It is, therefore, relevant to understand if this project affects perceived health and mental well-being of its beneficiaries. We hypothesise that someone is happier and less stressed living in a cleaner, warmer, more aesthetically pleasing environment. Like Cattaneo et al. (Citation2009), we use the ‘Perceived Stress Scale’ (PSS) developed by Cohen et al. (Citation1983) to measure the impact on mental health.Footnote9
Additionally, access to electricity and thus appropriate lighting can extend children’s study hours and ultimately improve their educational performance. Empirically, there is no consensus about the relationship between time spent on homework and whether a household has electricity (Khandker et al., Citation2012; Barron and Torero, Citation2017), especially for the African context (Bensch et al., Citation2011; Peters and Sievert, Citation2016). This study looks at days absent from school, school expenses and hours spent on schooling to assess the project’s impact on education. summarises these transmission channels from product development to an increase in people’s well-being.
4. Data and empirical approach
Given the commercial nature of both partner banks, the study design had to be as unobtrusive as possible. The banks did not want to deny their clients access to a product based on random selection because they were concerned about their client relationships and reputation. Instead, both banks agreed to a staggered roll-out and to withhold the product from a small number of branches, which they claimed they had selected to be highly comparable in terms of their geographic locations with branches that did receive the product but were far enough to avoid unintended spillover effects. presents the geographical spread of the final branches that were selected to be part of this study.
4.1. Data collection
summarises the timeline of the development of the HMF products and the impact evaluation in both countries. In Kenya, the bank provided us with a non-random list of eight branches to be part of the evaluation: four treatment and four comparison branches. In June 2015, after a six-month piloting phase, the partner bank rolled out the ‘Nyumba Smart Loan’ in all but four of its 222 branches across Kenya. In Uganda, after a piloting phase of nine months, the partner bank started to roll out the ‘Cente-Home Loan’ to 15 branches every six months until all branches had been served. In August 2016, the product was offered to the first 15 branches across Uganda. Clients from these branches constitute the treatment group. The bank provided us with a list of further 17 branches in which the phased roll-out would only take place after the follow-up survey. Clients from these branches serve as the comparison group. Of these 32 branches, we randomly selected 17 to be part of this study (9 belonging to the treatment group and 8 belonging to the comparison group). This selection was done to facilitate logistics and to reduce data collection costs.
To ensure a representative sample in each branch, at baseline, we stratified the number of clients per branch proportional to the total number of clients in that branch. For both banks, to become a client and open an account it is required to possess a government-issued ID. During piloting in both countries, take-up among bank clients was low, perhaps also due to the specific purpose advertised by this product. Hence, to ensure a powerful sample of people who are interested in the BAUA programme, we decided to oversample customers who had recently taken out the HMF product. In both countries, three out of four customers in our treatment sample were randomly chosen from a pool of customers who decided to use the product (‘adopters’). The remaining quarter in our treatment sample is a random sample of existing bank clients who were also offered the product but had not taken up the loan, yet (‘non-adopters’). The comparison group is a random sample of existing bank clients from branches without access to the BAUA programme. Take-up statistics throughout the project validate our approach. In Kenya, between baseline and endline survey, only 8% among non-adopters decided to take up the HMF product. In Uganda, only 5%. We have no reason to believe that contamination in comparison branches is an issue because take-up in those branches was zero.
Consequently, we conducted retrospective baseline surveys shortly after take-up. Baseline data collection was conducted between April to June 2016 in Kenya, and between June to July 2017 in Uganda. Given that there is likely to be a delay between output delivery activities and their measurable effect on outcome performance indicators, the baseline study still provides an accurate estimate of pre-operation conditions even after the project has begun. Even if participants would immediately start construction work at their homes, we expect housing improvement impacts to surface only over the medium or longer run so that impacts are not visible two weeks after loan-granting. In the very unlikely event that this may happen, we at most underestimate the effects of the HMF product at endline.
In total, the baseline sample includes 3,020 individuals across the two countries, 1,546 KWFT clients in Kenya (771 from the comparison group and 775 from the treatment group) and 1,474 Centenary Bank clients in Uganda (673 from the comparison group and 801 from the treatment group). All participants were revisited for a follow-up survey about 18 months later before the BAUA programme began in comparison branches (Kenya: September to November 2017, Uganda: October to November 2018). 2,341, or 77.5%, could be reached. In Kenya, the field teams completed 1,242 interviews during this follow-up survey, out of which 633 respondents could access the HMF product. In Uganda, 1,099 respondents participated at follow-up; 581 of them were offered the HMF product. shows that attrition was not significantly associated with treatment status, which implies no selective attrition.
4.2. Bank clients and loan usage
Baseline surveys contain a wide range of information on household and individual characteristics, in addition to house ownership, dwelling’s age, time lived in house and outcome data. Respondents were asked to recall the condition of their houses before the provision of the loan. We expect this information to be reasonably accurate because dwelling characteristics and major home improvements are easy to remember. Other information, however, may become more difficult to recall, including variables such as perceived health, mental well-being, or monetary values. Like Lachaud et al. (Citation2018), we cannot completely rule out systematic recollection errors at baseline and report results (especially on health and education) with caution.
displays baseline characteristics for bank clients in treatment branches, alongside the difference vis-à-vis comparison branches separated by the two countries.Footnote10 On average, participants are about 40 years old and married. Households consist of five members, including the respondent and two children. In both samples, many households possess livestock (cows, goats, pigs, poultry), while vehicles are less commonly owned. House ownership is very common. 42% have been living in their house for ten or more years. Houses are on average ten years old, but twelve per cent of the sample do not know when their house was built. We still consider this covariate to be important for loan take-up and, hence, include a missingness dummy as an additional covariate and recode missing values to zero. While less than 30% of participants have completed secondary education or more in Kenya, the Ugandan sample is significantly more educated. Also, more participants state ‘subsistence farming’ as their main occupation in Kenya than in Uganda.
Table 1. Household balancing (baseline values).
Interestingly, the share of Kenyan households owning livestock, land or a house is significantly higher in the treatment group compared to the comparison group. This implies a target group of wealthier households in Kenya. Among treatment households in Uganda, significantly less people in treatment branches were married than in comparison branches. Further, having non-agricultural employment, a greater likelihood for television and fridge ownership and less time lived in generally younger houses are variables correlated with treatment status. In the Kenya sample, the value of the F-statistic for the test that the covariates jointly predict assignment to treatment (’omnibus test’) is 8.48 (p = 0.000). Like in Kenya, the p-value for an omnibus test of balance is 0.000 in Uganda.
shows that 95% of those who took up the HMF product in treatment branches used the loan for the intended housing improvement. The loan was commonly dedicated to improving the physical structure of the houses (roofs, floors, doors/windows), to paint the exterior, or to buy building materials for the construction of new houses. A less common usage was to improve basic services such as sanitation, water, or electrification. The category ‘other’ mainly refers to non-labelled purposes such as school fees, business, or medical expenses. As multiple response options were possible, we also see that about 40% of clients mentioned at least two purposes, with on average 30% being unrelated to housing improvements. This suggests that some households are either insensitive to the loan label or that there are other more pressing needs and constraints, respectively.
4.3. Empirical strategy
4.3.1. Identification strategy
This study identifies causal impacts of the combination of the financial and the non-financial component of the BAUA programme. The empirical analysis makes use of two-period panel data surveys in Kenya and Uganda. More specifically, we take advantage of the delayed provision of the BAUA programme in some branches and collected household data of existing bank clients in branches with access and without access to the BAUA programme. One challenge of this evaluation is selection into treatment that happens on two levels: (i) on the branch level, and (ii) once a branch offers the HMF product on the household level.
First, we observe that certain branches were given priority by the lenders to offer the BAUA programme. shows p-values and normalised differences for branch characteristics at baseline. In Kenya, none mean-equality test is significant using the conventional p-values in column (5). Given the low number of branches per country, column (6) additionally shows the normalised difference that is the difference in means between the treatment and comparison groups, divided by the square root of the average of the sample variances of the two groups, following Imbens (Citation2015). This measure is not affected by scale or sample size and thus accounts for the low number of branches. The lowest normalised difference obtained equates to 0.390, and so does not pass the Imbens (Citation2015) cut-off criterion of 0.3. In both countries, all branch characteristics lie well above this value and need to be considered imbalanced. We argue that this results most likely from a roll out strategy that targeted more profitable and/or greater branches first because treatment branches have more clients, more credit officers and hold a higher collective value of loans (‘loan book’) than comparison branches. Consequently, and to achieve comparability between both groups, we include branch-level fixed effects with standard errors clustered at the branch level into our regression models.
Table 2. Balance across branches.
Second, we account for self-selection on the household level by using the variation between adopters and non-adopters in treatment branches to identify those clients in the comparison branches that would be likely to take up the HMF product if it was available (Bensch et al., Citation2011; Lenz et al., Citation2017).Footnote11 For this purpose, we first only consider clients in treatment branches and run a probit model with take-up of the HMF product as the dependent variable, including several baseline covariates that have predictive power for loan take-up and impact indicators. reveals some precisely estimated coefficients. In Kenya, the probability of take-up increases with land and house ownership and decreases with the age of the dwelling. In Uganda, males are more likely to take up the HMF product than females. Those who are older, better off, own a television or a house have a higher probability of taking up the loan. Owning livestock or having lived in a house for a long period decreases this probability. Looking at the pseudo-r-squared, the predictive power of the conditioning variables is much higher in Uganda (0.160) than in Kenya (0.050).
Table 3. Probit regression of loan take-up on baseline characteristics (treatment sample only).
Coefficients from this probit model are then used to predict the probability of taking up the loan for each client in both country subsamples, including clients in comparison branches. Based on these predicted propensity scores, we identify hypothetical adopters in comparison branches using the kernel matching algorithm with a Gaussian kernel and bandwidth of 0.05. Kernel matching can be seen as a weighted regression of the counterfactual outcome. It takes each adopter and matches them to a weighted average of all clients in comparison branches. The weights depend on the distance between clients in comparison branches and adopters in treatment branches. Those clients in comparison branches who are close in terms of propensity score of an adopter receive more weight when calculating the treatment effect for an adopter and those who are more distant comparison clients are down-weighted. Results are also robust to the application of alternative matching algorithms such as using a threshold of 0.5 to be considered a hypothetical adopter as in Bensch et al. (Citation2011), radius matching, and nearest neighbour matching (see Section 6).
The advantage of this approach is that we use the variation of adoption in treatment branches to identify hypothetical adopters in comparison branches. Because the latter were recruited from branches that had no access to the BAUA programme, they could not self-select into treatment. This is likely to substantially reduce selection biases. Both pairs of graphs in suggest that kernel matching improves balance.
Figure 4. Propensity scores histograms of adopters and comparison households. Adopters are households in treatment branches that took up the product, while comparison households are existing clients in non-treated branches.

The matching approach builds on the conditional independence assumption. We here rely on variables observed after the intervention but for which we assume to affect the take-up decision and are not affected by the loan: gender, age, inelastic dwelling characteristics (age, years inhabited) and relatively inelastic wealth indicators that capture pre loan take-up wealth.Footnote12
Additionally, reemphasises that the kernel matching process can balance most covariates that are used to calculate propensity scores. Indeed, differences in means between adopters and hypothetical adopters decrease substantially and become less significant or insignificant. For the Ugandan sample, there are two exceptions – being a woman and married – but overall balance improves. Running the probit model again using adopters and hypothetical adopters reduces the pseudo-R2 to 0.008, compared with 0.050 using the households from treatment branches only (see ). Together with the respective chi-squared statistic, which does not show joint significance anymore, both tests confirm the success of the matching procedure in Kenya. In Uganda, the pseudo-R2 of a probit regression of take-up on socio-economic and housing baseline characteristics also reduces from 0.160 to 0.039. While the substantial decrease is supporting the success of the matching procedure, the respective chi-squared statistic still shows a joint significant influence of the covariates. In sum, the weighted sample of hypothetical adopters yield a more reasonable comparison group in Uganda, although slight discrepancies remain as indicated by the non-balancing on gender and marital status. As a result, we condition on this set of covariates for the impact analysis to further improve the balancing.
Table 4. Pre- and post-matching balancing.
4.3.2. Difference-in-differences estimation
Using a DiD approach, we next estimate the TOT by comparing adopters in treatment branches to matched clients in comparison branches – hypothetical adopters. Non-adopters in treatment branches are excluded from our analysis. The DiD approach accounts for potentially remaining distorting dissimilarities between treatment and comparison branches but also builds on key assumptions.
The first assumption underlying the DiD approach is that, in the absence of the BAUA programme, the average outcomes for the adopters and hypothetical adopters would have followed parallel paths over time. Although the DiD approach controls for group-specific and time-constant unobservables, this assumption may be implausible if pre-treatment characteristics that are thought to be associated with outcome variables are unbalanced between both groups. Despite the lack of longer pre-treatment periods that prevents us from extending pre-trend tests to, for instance, event-study methods, the good baseline balancing shown in makes strong secular trends unlikely. Nevertheless, the caveat of imbalanced branches shown in remains. Even though, we have found quite similar households in both treatment and comparison branches, we cannot completely rule out that they have not moved in parallel over time.
Second, the DiD estimator also requires that the outcome of one unit is unaffected by the treatment status of other units. Throughout this study, we implicitly assume that this Stable Unit Treatment Value Assumption (SUTVA) holds. This assumption will be violated when externalities are present and spillover effects exist. For this evaluation, we assume that if there are spillover effects, they only exist at a local level and not across branches. Because we vary exposure to the treatment at the branch level, it is unlikely that spillover effects on untreated households undermine our identification strategy. Nevertheless, Appendix A investigates spillover effects on non-adopters in treatment branches.
Third, a causal interpretation requires the absence of anticipation effects. The restriction could fail in practice if respondents change their behaviour in anticipation of the treatment. We believe this to be unlikely because our survey team assessed all primary outcomes on site. Hence, adopters could not lie about outcomes such as dwelling characteristics and services that would benefit their application for future housing loans. The same holds for non-adopters which is only relevant when thinking about spillover effects as they are excluded from our main analysis. In comparison branches, the HMF was withhold and not marketed at all.
If the identifying assumptions hold, the treatment effect can be estimated in a regression framework. Ti ∈ {0, 1} indicates whether or not individual, i, is treated (Ti = 1), i.e. took up the HMF product, and Postt ∈ {0, 1} indicates the post treatment period (Postt = 1), i.e. during follow-up surveys. We further interact a linear time trend with the treatment dummy. Because the regressor of interest only varies at the branch level, we also include branch-level fixed effects. These control for any time-invariant small-sample differences, e.g., certain types of branches were given priority for treatment selection by banks. Additionally, we cluster errors at the branch level in all specifications. To further reduce the risk of confounding factors, a set of relevant pre-treatment covariates that are relevant for the development of outcomes or product take-up enter the regression:
where Yit denotes the individual outcome variable i at time t, Bj are branch dummies and Xi denotes a vector of baseline covariates used for calculating the propensity score in the kernel matching procedure. ϵijt represents the error-term. The coefficients, β and δ are to be estimated. The coefficient of interest, β3, is the DiD estimator for the impact of the intervention on adopters i.
5. Results
presents results from EquationEquation (1)(1)
(1) , studying the differential effects of the HMF product on adopters versus hypothetical adopters. We counteract the problem of multiple hypothesis testing by adjusting the rejection criteria for each of the individual hypotheses according to the Holm-Bonferroni method (Holm, Citation1979). All outcomes are self-reported, though, if possible, double checked by our survey team when conducting the interview. Variable definitions are provided in .
Table 5. Primary treatment effects in Kenya and Uganda.
Looking at dwelling characteristics, our analysis finds contrasting effects between both country subsamples. In Kenya, beneficiaries of the ‘Nyumba Smart Loan’ experienced a positive impact on the overall housing conditions. As a result of loan take-up, they added more rooms to their houses, and improved roofing, walls, and flooring. Improved walls and flooring, however, remain the only significant outcomes when correcting for multiple hypotheses. In Uganda, we find no impact on the reported dwelling characteristics. In line with the modest to zero treatment effects, shows that spillover effects on non-adopters’ housing in treatment areas (e.g., from higher awareness of the benefits of better housing conditions) are very unlikely to undermine our identification strategy.
Similarly, for services and rain protection, the HMF product had greater impact in Kenya than in Uganda. In Kenya, there is an improvement in the type of fuel used for lighting and cooking (electricity, gas, solar energy) and protection against rain that is consistently significant also when adjusting the p-values. On average, beneficiaries also report improved access to water of about 3.7 percentage points, though not significant using the adjusted p-value. Adopters mainly stated an increase in access to piped water connections and water tanks. In Uganda, we see a positive impact on rain protection on the five percent significance level but cannot confirm further impacts under this outcome category for Ugandan households.
In both countries, positive impacts on the physical housing structure have resulted in an improved housing satisfaction amongst users of the HMF product. Our analyses show a significant increase in satisfaction with the quality of floors, walls, roofs, and the overall housing condition. Results are similar for both countries and among estimation strategies. We do not see any statistically significant impact on pride. Pride indicates whether the person reported to be proud of their house and would, for instance, hold a social event at their house.
The paper does come with some limitations inherent to the variety of housing improvements the HMF product can be used for. The methodological implication is that our sample size is too small to have enough statistical power to determine downstream effects of each type of improvement – secondary outcomes. Hence, we view results presented in as exploratory and statistically underpowered. The absence of an effect in our analysis might well be related to our sample size.
Table 6. Secondary treatment effects in Kenya and Uganda.
In terms of personal finances (current and future finances, savings, borrowings, and earnings), we see no changes in households’ financial behaviour in Kenya. Ugandan households are more likely to be optimistic about their overall financial situation in comparison with their peers. From the theory of change, the improvements made to housing are expected to lead to an improvement in the health of the occupants. And although both countries consistently show negative coefficients on symptoms, we cannot confidently attribute mental or physical health impacts to the HMF product take-up. The same is true for educational outcomes.Footnote13
6. Additional analyses
6.1. Sensitivity to alternative matching algorithms
To account for statistical differences between pre-treatment characteristics in the treatment and comparison branches, we use a combination of kernel matching algorithm and DiD to strengthen our evaluation design and to ensure that credible results of impact can still be produced while the parallel trend assumption is more likely. This section further augments our assumptions and employs three robustness exercises on our primary outcome results. First, we follow Bensch et al. (Citation2011) and assume that those comparison households that exhibit a propensity score larger than 0.5 to be hypothetical adopters. Using this threshold, we estimate DiD regressions. Next, we apply two alternative matching algorithms, radius caliper and nearest neighbour matching, to verify our results are robust to the matching algorithm applied. All regression models control for the set of baseline covariates used for calculating the propensity score in the matching approach. This corrects for any residual differences in the covariates that may be present in the weighted sample.
Columns five to twelve in , and A8 present different estimation methods and confirm our main results for materials used for housing, services, and satisfaction. Although impacts on materials used are stronger in Kenya than in Uganda, shows a similar positive impact on satisfaction in both countries. This suggests that the HMF product in Uganda is being used for purposes other than improving the building materials or services but that make customers similarly happy, such as plastering or painting walls.
6.2. Intention to treat effects
We also estimate effects considering actual assignment to treatment, regardless of whether the client had taken out the HMF product or not. Biases because of self-selection into treatment on the household level should be less concerning as we compare means over the whole (unmatched) sample, including adopters and non-adopters in treatment branches and all comparison households.
Compared to TOT effects, these intentions to treat effects are similar in magnitude and still only happening for Kenya when looking at dwelling characteristics (columns one to four in ). The first four columns in confirm that most results presented in our main analyses are robust to this approach.
6.3. Sensitivity to statistical power
Results of pre-intervention power calculations for the individual countries are presented in . Columns four to six show the intra-class correlation as well as the minimum detectable effect (MDE) size in percentage points for outcome indicators. We focus on discussing MDEs for dwelling characteristics. Because samples in both countries vary a lot, we do not pool data from both countries to further increase the statistical power. The probability of making a false statement if a null hypothesis is rejected is 5% and the probability of making a false statement if the null is not rejected is 20%. The last column reports the size of that effect relative to the baseline comparison mean in percent. In both countries, we assume a take-up rate of 75% because 75% of clients in our treatment sample had taken out the HMF product. This attempt to increase statistical power yields MDEs that vary across outcomes, ranging between 3% and 60% in Kenya and 7% to 74% in Uganda.
MDEs remain robust when we adjust the number of observations to the actual sample size reached at endline. The reason is likely to be the constant number of clusters between baseline and endline: 8 branches in Kenya and 17 branches in Uganda. With clustered data, the statistical power is determined by the combination of the number of clusters and the cluster size. That means even if we consider attrition for power calculations, the cluster size or the number of clients per cluster remains high at endline. In both countries, MDEs are largest for the number of rooms. If we assume the average hypothetical adopter to have three rooms at endline, adding one room to the house can have a massive impact. The same is true for other housing improvements such as better roofing or flooring, which indicates that dwelling characteristics as outcomes seem to be less demanding in terms of sample size or measuring precision than, for instance, profits or sales. However, the evidence on housing finance is still scarce and no meta-analysis suggests average impacts, yet.
In Uganda, our coefficients are generally quite small, for most dwelling characteristics below ten percent. This emphasises the point that not finding a significant effect on dwelling characteristics of the BAUA programme in Uganda does not mean that the BAUA programme had no effect. Additionally, we strategically oversampled clients who were interested in the housing loan. A random selection of existing bank clients from the actual population would have resulted in extremely low take-up rates (or low compliance rates) and ultimately in extremely large MDEs (Dahal and Fiala, Citation2020). Moreover, those who take up the loan are likely to not use the entire money on their house, but on other unrelated purposes such as school fees, business, or medical expenses (see Section 4.2). The bottom line is that under actual compliance rates, these type of microfinance evaluations face significant power challenges. Dahal and Fiala (Citation2020) discuss some solutions to overcome these challenges, e.g., collecting data at multiple periods, better targeting, and randomisation at the individual level.
7. Discussion and conclusion
Remaining true to Habitat’s original principles of self-help and sustainability, through the BAUA programme, Habitat focused on improving systems that enable families to achieve affordable housing without needing ongoing direct support. This meant supporting local firms and expanding innovative and client-responsive services, products, and financing so that households can improve their housing more effectively. This study evaluates the final products of the BAUA programme in Kenya and Uganda that is a combination of a labelled loan and non-financial housing support services.
In both countries, our findings suggest that the HMF product has generally improved the lives of those who have taken up the loan, as well as their families, because coefficients are not only statistically but also economically significant. We conclude that our results are robust as inconsistencies using various matching procedures are rare. This suggests that labelling is sufficient to nudge clients to invest in their homes without restricting their choice set. It is, however, this wide range of use options that complicates the evaluation of health or educational impacts. Although all coefficients go into the intended direction, this study does not entail the sufficient power and (potentially) time frame to observe ultimate effects on poverty indicators.
This study has nothing to say about impacts on marginal borrowers. This clearly has implications for external validity as impacts on borrowers at the external margin can differ compared with impacts presented in this study (Wydick, Citation2016). Even though the impact on the inframarginal borrower is considered the policy-relevant parameter in our study context, it may well be that results change once the banks extend their services to new areas and individuals not already being served. Our findings, therefore, represent a close approximation on existing bank clients who are interested in the BAUA programme in Kenya and Uganda because we sampled multiple branches across the countries, not limited to one area. Additionally, we have no reason to believe that these effects are affected by special care problems because the BAUA programme was provided under business-as-usual conditions. Each bank must understand, which products are profitable – meaning that both banks in our study had no further incentive to track this product differently than other products. In fact, both banks still offer the HMF product as part of their loan portfolio.
The general evidence shows that most micro-loans are actually used for other purposes than they were initially intended for, e.g., wedding expenses, religious festivals, consumption, or repayment of other debts. Given our findings, however, we can confidently say that the HMF product was (at least partially) used for the purpose it was officially intended for (which is admittedly a broad range of purposes). As such the labelling is sufficient to nudge clients towards housing improvements, but we cannot claim any causal changes on poverty indicators as a result of it. This therefore presents an opportunity to advance the conversation around FSPs investing, scaling, and replicating HMF products in other contexts. More data and time may be needed to fully establish the potential of housing microfinance to synergistically exploit socio-economic, health and environmental goals, as decent and affordable housing remains a critical component to anti-poverty interventions.
Acknowledgment
The data underlying this research were collected for an impact evaluation commissioned by Habitat for Humanity’s Terwilliger Centre for Innovation in Shelter. We are grateful for valuable comments by Nathan Fiala, Thorben Korfhage, Lise Masselus, Jörg Peters, and Maximiliane Sievert. Annekathrin Schoofs gratefully acknowledges the support of a special grant (Sondertatbestand) from the German Federal Ministry for Economic Affairs and Energy and the Ministry of Innovation, Science, and Research of the State of North Rhine-Westphalia, and financial support by the Transatlantic Programme of the Federal Republic of Germany and the European Recovery Programme of the Federal Ministry of Economics and Technology (BMWi) as well as the RWI Transatlantic Research Exchange Programme.
Disclosure statement
No potential conflict of interest was reported by the author(s).
Additional information
Funding
Notes
1. In the following, we use the ‘BAUA programme’ and ‘HMF product’ interchangeably, keeping in mind that the HMF product includes financial and non-financial services.
2. KWFT is one of the largest deposit-taking microfinance institutions in Kenya in terms of market share, number of branches, and size of loan portfolio.
3. Centenary Bank is a leading microfinance commercial bank in Uganda serving over 1,400,000 customers, whose services can be accessed through 72 branches across the country.
4. Households move from having an average of one bedroom in informal shacks to an average of two bedrooms in an RDP home. This reduces the percentage of households that use their kitchen as a sleeping area from 73% to 4% (Legovini et al., Citation2011).
5. In 2014, the programme has been renamed to Prospera.
6. The Ugandan Bureau of Statistics uses the definition by the WHO/UNICEF Joint Monitoring Programme (JMP). ‘An improved drinking water source is one that, by nature of its construction and when properly used, adequately protects the source from outside contamination, particularly faecal matter. Water sources considered as improved include piped water, public taps, boreholes, protected springs/wells, gravity flow schemes, rainwater, and bottled water. Unprotected wells/springs, rivers/lakes/streams, vendors, and tanker trucks were considered unimproved water sources’ (Uganda Bureau of Statistics, Citation2018, p. 140).
7. The purchasing power parity adjusted exchange rate in 2014 was 38.3 KES/1 USD in Kenya and 1,073.7 UGX/1 USD in Uganda.
8. We present this only for Kenya because sanitation improvements were incorrectly translated in Uganda.
9. This ten-item scale captures the degree to which members find their lives to be unpredictable, uncontrollable, and overloaded. Participants were asked to report how frequently they felt a certain way in the past six months. See for PSS questions.
10. We follow Lin and Green (Citation2016) in treating missing covariates. If no more than 10% of the covariate’s values are missing, we recode the missing values to the overall country mean at baseline. If more than 10% of the covariate’s values are missing, we include a missingness dummy as an additional covariate and recode missing values to zero. We test sensitivity of estimates to these approaches. Results are available upon request.
11. Bensch et al. (Citation2011); Lenz et al. (Citation2017) apply a similar approach to study the decision to connect to an electricity grid in Rwanda.
12. Despite a careful selection of covariates, households that decide to take up the HMF product may do so for reasons that are potentially unobservable to the researcher and that, at the same time, affect the outcomes of interest (e.g., employees’ specific characteristics at the branch level that can affect customers’ choice of HMF products). We cannot completely rule out that, even after matching, such differences remain and that they could bias our findings.
13. Despite this, qualitative interviews undertaken with beneficiaries revealed that improvements made to their physical housing structure, such as adding extra rooms, have made the households more conducive for children to spend more time on their homework.
References
- Augsburg, B., B. Caeyers, S. Giunti, B. K. Malde, and S. Smets (2021). Labelled Loans and Human Capital Investments. IFS Working Paper W21/09.
- Barnhardt, S., E. Field, and R. Pande. 2017. “Moving to Opportunity or Isolation? Network Effects of a Randomized Housing Lottery in Urban India.” American Economic Journal: Applied Economics 9 (1): 1–32.
- Barron, M., and M. Torero. 2017. “Household Electrification and Indoor Air Pollution.” Journal of Environmental Economics and Management 86: 81–92. doi:10.1016/j.jeem.2017.07.007.
- Bensch, G., J. Kluve, and J. Peters (2011). Impacts of Rural Electrification in Rwanda, Journal of Development Effectiveness3(4): 567–588.
- Cattaneo, M. D., S. Galiani, P. J. Gertler, S. Martinez, and R. Titiunik. 2009. “Housing, Health, and Happiness.” American Economic Journal: Economic Policy 1 (1): 75–105.
- Cohen, S., T. Kamarck, and R. Mermelstein (1983). A Global Measure of Perceived Stress, Journal of Health and Social Behavior pp. 385–396.
- Dahal, M., and N. Fiala. 2020. “What Do We Know About the Impact of Microfinance? The Problems of Statistical Power and Precision.” World Development 128: 104773. doi:10.1016/j.worlddev.2019.104773.
- Devoto, F., E. Duflo, P. Dupas, W. Parient´e, and V. Pons. 2012. “Happiness on Tap: Piped Water Adoption in Urban Morocco.” American Economic Journal: Economic Policy 4 (4): 68–99.
- Dizon, F. F., E. Gong, and K. Jones. 2020. “The Effect of Promoting Savings on Informal Risk-Sharing: Experimental Evidence from Vulnerable Women in Kenya.” The Journal of Human Resources 55 (3): 963–998. doi:10.3368/jhr.55.3.0917-9077R2.
- Duflo, E., M. Greenstone, R. Guiteras, and T. Clasen (2015). Toilets Can Work: Short and Medium Run Health Impacts of Addressing Complementarities and Externalities in Water and Sanitation. National Bureau of Economic Research Working Paper No. 21521.
- Environmental Health Project (2004). The Hygiene Improvement Framework: A Comprehensive Approach for Preventing Childhood Diarrhea. Prepared by EHP, UNICEF/WES, USAID, World Bank/WSP, WSSCC Accessed 7 July 2022. https://www.ehproject.org/PDF/JointPublications/JP008-HIF.pdf
- Evans, G. W. (2003). The Built Environment and Mental Health, Journal of Urban Health 10.1093/jurban/jtg063 80(4): 536–555.
- Field, E. 2007. “Entitled to Work: Urban Property Rights and Labor Supply in Peru.” The Quarterly Journal of Economics 122 (4): 1561–1602. doi:10.1162/qjec.2007.122.4.1561.
- Galiani, S., P. J. Gertler, R. Undurraga, R. Cooper, S. Martínez, and A. Ross. 2017. “Shelter from the Storm: Upgrading Housing Infrastructure in Latin American Slums.” Journal of Urban Economics 98: 187–213. doi:10.1016/j.jue.2016.11.001.
- Galiani, S., and E. Schargrodsky. 2010. “Property Rights for the Poor: Effects of Land Titling.” Journal of Public Economics 94(9–10): 700–729. doi:10.1016/j.jpubeco.2010.06.002.
- Guiteras, R., J. Levinsohn, and A. M. Mobarak. 2015. “Encouraging Sanitation Investment in the Developing World: A Cluster-Randomized Trial.” Science 348 (6237): 903–906. doi:10.1126/science.aaa0491.
- Habitat for Humanity (2019). The Housing Need in Uganda. Accessed 7 July 2022. https://www.habitat.org/where-we-build/uganda
- Habyarimana, J., and W. Jack. 2018. High Hopes: Experimental Evidence on Saving and the Transition to High School in Kenya. Georgetown University Initiative on Innovation Development and Evaluation (gui2de) Working Paper No. 4.
- Holm, S. 1979. “A Simple Sequentially Rejective Multiple Test Procedure.” Scandinavian Journal of Statistics 65–70.
- Imbens, G. W. 2015. “Matching Methods in Practice: Three Examples.” The Journal of Human Resources 50 (2): 373–419. doi:10.3368/jhr.50.2.373.
- Kenya National Bureau of Statistics (2015). Kenya Demographic and Health Survey 2014. Nairobi.
- Khandker, S. R., D. F. Barnes, and H. A. Samad. 2012. “The Welfare Impacts of Rural Electrification in Bangladesh.” The Energy Journal 33 (1): 187–206. doi:10.5547/ISSN0195-6574-EJ-Vol33-No1-7.
- Lachaud, M. A., B. E. Bravo-Ureta, N. Fiala, and S. P. Gonzalez. 2018. “The Impact of Agri-Business Skills Training in Zimbabwe: An Evaluation of the Training for Rural Economic Empowerment (TREE) Programme.” Journal of Development Effectiveness 10 (3): 373–391. doi:10.1080/19439342.2018.1464494.
- Legovini, A., S. Martinez, and N. Krishnan. 2011. Measuring Success in Human Settlements Development: An Impact Evaluation Study of the Upgrading of Informal Settlements Pro- Gramme in Selected Projects in South Africa. South Africa: The Department of Human Settlements.
- Lenz, L., A. Munyehirwe, J. Peters, and M. Sievert. 2017. “Does Large-Scale Infrastructure Investment Alleviate Poverty? Impacts of Rwanda’s Electricity Access Roll-Out Program.” World Development 89: 88–110. doi:10.1016/j.worlddev.2016.08.003.
- Lin, W., and D. P. Green. 2016. “Standard Operating Procedures: A Safety Net for Pre-Analysis Plans.” PS: Political Science & Politics 49 (3): 495–500.
- Lipscomb, M., and L. Schechter. 2018. “Subsidies versus Mental Accounting Nudges: Har- Nessing Mobile Payment Systems to Improve Sanitation.” Journal of Development Economics 135: 235–254. doi:10.1016/j.jdeveco.2018.07.007.
- Martin, W. J., J. W. Hollingsworth, and V. Ramanathan. 2014. “Household Air Pollution from Cookstoves: Impacts on Health and Climate.” In Global Climate Change and Public Health, pp. 237–255. New York: Humana Press.
- Peters, J., and M. Sievert. 2016. “Impacts of Rural Electrification Revisited–the African Context.” Journal of Development Effectiveness 8(3): 327–345.
- Thaler, R. 1985. “Mental Accounting and Consumer Choice.” Marketing Science 4 (3): 199–214. doi:10.1287/mksc.4.3.199.
- The Ministry of Lands, Housing and Urban Development (2016). The Uganda National Housing Policy. Accessed 7 July 2022. https://mlhud.go.ug/wp-content/uploads/2015/10/National-Housing-Policy-May-2016.pdf
- The World Bank (2011). Developing Kenya’s Mortgage Market. Accessed 7 July 2022. https://housingfinanceafrica.org/app/uploads/Kenya Expanding Mortgage FinanceSM.pdf
- The World Bank (2017). Kenya Economic Update: Housing - Unavailableand Unaffordable. Accessed 7 July 2022. https://documents1.worldbank.org/curated/en/988191491576935397/pdf/114115-REVISED-PUBLIC-KenyaEconomicUpdateFINALFINALMay.pdf
- Thomson, H., S. Thomas, E. Sellstrom, and M. Petticrew. 2009. “The Health Impacts of Hous- Ing Improvement: A Systematic Review of Intervention Studies from 1887 to 2007.” American Journal of Public Health 99 (S3): 681–692. doi:10.2105/AJPH.2008.143909.
- Uganda Bureau of Statistics. 2018. Uganda National Household Survey 2016/17. Vol. 6. Kampala.
- UN (2015). Transforming Our World: The 2030 Agenda for Sustainable Development. UN, General Assembly A/RES/70/1, 21 October.
- Wydick, B. 2016. “Microfinance on the Margin: Why Recent Impact Studies May Understate Average Treatment Effects.” Journal of Development Effectiveness 8 (2): 257–265. doi:10.1080/19439342.2015.1121512.
- Yishay, A. B., A. Fraker, R. Guiteras, G. Palloni, N. B. Shah, S. Shirrell, and P. Wang. 2017. “Microcredit and Willingness to Pay for Environmental Quality: Evidence from a Randomized-Controlled Trial of Finance for Sanitation in Rural Cambodia.” Journal of Envi- Ronmental Economics and Management 86: 121–140. doi:10.1016/j.jeem.2016.11.004.
Appendix Appendix A.
Additional figures and tables
Table A1. Comparison of loans.
Table A2. ‘Perceived Stress Scale’ questions.
Table A3. Sample attrition.
Table A4. Variable descriptions.
Table A5. Impacts on non-adopters in treatment branches.
Table A6. Robustness: Dwelling characteristics.
Table A7. Robustness: Services & protection.
Table A8. Robustness: Satisfaction & pride.
Table A9. Minimum detectable effects for dwelling characteristics.